How to Fine-tune the Protein Language Model With a Small Amount of Wet Experimental Data? The Results of the Zhejiang University Team Were Selected for NeurIPS 2024, and the First Author of the Paper Explained the Design Ideas in Detail
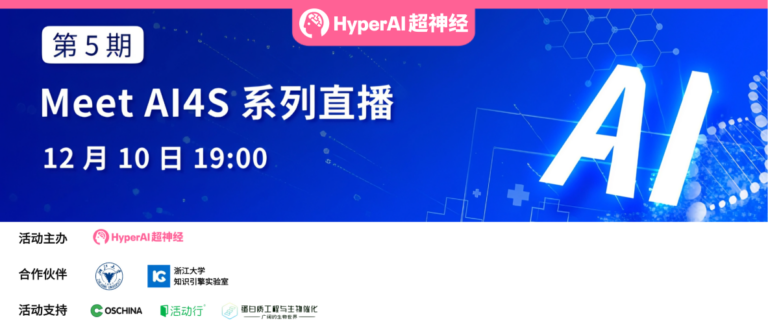
The fifth episode of the "Meet AI4S" live broadcast series will be broadcast on time at 19:00 on December 10. HyperAI is honored to invite Wang Zeyuan, a doctoral student from the Knowledge Engine Laboratory of Zhejiang University. The theme of his sharing this time is "Using the diffusion denoising process to help large models optimize proteins."
Professor Chen Huajun, Researcher Zhang Qiang, Dr. Wang Zeyuan and others from Zhejiang University proposed a new denoising protein language model (DePLM).The evolutionary information captured by the protein language model can be viewed as a mixture of information that is relevant and irrelevant to the target property, where irrelevant information is considered "noise" and eliminated, thereby predicting the protein adaptive landscape and helping protein optimization.
Research has shown that DePLM outperforms existing methods in predicting the effects of protein mutations and has strong generalization capabilities for new proteins. This achievement has been selected for the top conference NeurIPS 2024. In this live broadcast, Dr. Wang Zeyuan will explain the innovative ideas of this paper in detail.
HyperAI has also specially prepared super-value computing power benefits for everyone.Participate in the live broadcast lucky draw and you will have a chance to win 10 hours of NVIDIA RTX A6000, worth 40 yuan, and the resource is valid for 1 month.Come and make an appointment for the live broadcast!
Click to schedule a live broadcast:
Scan the QR code and remark "AI4S" to join the discussion group⬇️

Guest Introduction

Share the topic
Using diffusion denoising to help large models optimize proteins
Introduction
Our research group proposed a method to combine the large model with the diffusion denoising model. Through fine-tuning with a small amount of wet experimental data, the accuracy of the large model in protein adaptive landscape prediction tasks was improved while maintaining the model's own good generalization ability.
Audience benefits
1. Understand the methods, datasets and indicators for predicting protein fitness landscape
2. Understand how the Diffusion Model Enhanced Language Model (DePLM) can be used for adaptive landscape prediction
3. Explore how to combine evolutionary information, wet experiment and other data for AI model training
Paper Review
HyperAI has previously interpreted the research paper "DePLM: Denoising Protein Language Models for Property Optimization" with Dr. Wang Zeyuan as the first author.
Research highlights
* DePLM can effectively filter out information irrelevant to the target properties and improve protein optimization by optimizing the evolutionary information contained in PLM
* DePLM not only outperforms the current state-of-the-art models in predicting mutation effects, but also demonstrates strong generalization capabilities to new proteins
* This study designs a sorting-based forward process in the denoising diffusion framework, extending the diffusion process to the sorting space of mutation possibilities, while changing the learning objective from minimizing numerical error to maximizing sorting relevance, promoting dataset-independent learning and ensuring strong generalization capabilities of the model
Dataset acquisition
The study selected the ProteinGym protein mutation dataset, and after excluding the overly long wild-type protein dataset, ultimately retained 201 deep mutation screening (DMS) datasets.
The dataset is used directly:
https://hyper.ai/datasets/32818
Model Architecture
As shown in the left figure below, DePLM uses the evolution likelihood derived from PLM as input and generates a denoised likelihood for a specific attribute to predict the impact of mutations; in the middle and right sides of the figure below, the denoising module uses the feature encoder to generate a representation of the protein, taking into account the primary and tertiary structures, which are then used to filter the noise in the likelihood through the denoising module.

In order to achieve dataset-independent learning and ensure strong model generalization ability, the researchers performed a diffusion process in the ranking space of feature values and replaced the traditional objective of minimizing numerical error with maximizing ranking relevance.
Zhejiang University Knowledge Engine Laboratory
The Knowledge Engine Laboratory is based on the School of Computer Science and Technology, School of Software, etc. of Zhejiang University.We are committed to academic research, open source, and industrial innovation and application in the fields of knowledge graphs, large language models, and AI for Science. We have jointly built the Zhejiang University-Ant Group Knowledge Graph Joint Research and Development Center and the Zhejiang University-Alibaba Knowledge Engine Joint Laboratory.
The team is recruiting outstanding postdoctoral fellows, 100 experts, R&D engineers and other full-time researchers. Everyone is welcome to join~
Laboratory Github homepage:
Meet AI4S Live Series
HyperAI (hyper.ai) is China's largest search engine in the field of data science. It focuses on the latest scientific research results of AI for Science and tracks academic papers in top journals such as Nature and Science in real time. So far, it has completed the interpretation of nearly 200 AI for Science papers.
In addition, we also operate the only AI for Science open source project in China, awesome-ai4s.
* Project address:
https://github.com/hyperai/awesome-ai4s
In order to further promote the popularization of AI4S, further reduce the dissemination barriers of scientific research results of academic institutions, and share them with a wider range of industry scholars, technology enthusiasts and industrial units, HyperAI has planned the "Meet AI4S" video column, inviting researchers or related units who are deeply engaged in the field of AI for Science to share their research results and methods in the form of videos, and jointly discuss the opportunities and challenges faced by AI for Science in the process of scientific research progress and promotion and implementation, so as to promote the popularization and dissemination of AI for Science.
So far, we have successfully held 4 Meet AI4S live broadcasts, covering the fields of geographic information science, life science, and protein engineering.
We welcome efficient research groups and research institutions to participate in our live events!Scan the QR code to add "Neural Star" WeChat for details↓
