Containing 284 Data Sets, Covering 18 Clinical Tasks, Shanghai AI Lab and Others Released the Multimodal Medical Benchmark GMAI-MMBench
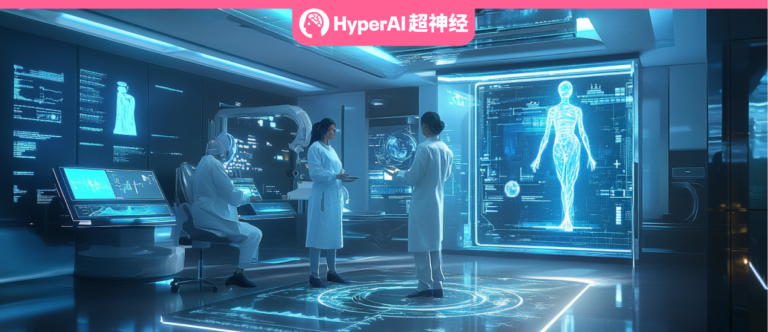
"With such an intelligent medical device, patients can complete the entire process of scanning, diagnosis, treatment and repair by just lying on it, thus achieving a healthy restart." This is a plot in the 2013 science fiction movie "Elysium".

Nowadays, with the rapid development of artificial intelligence technology, the medical scenes shown in science fiction movies are expected to become a reality. In the medical field, large visual language models (LVLMs) can process multiple data types such as imaging, text and even physiological signals, such as DeepSeek-VL, GPT-4V, Claude3-Opus, LLaVA-Med, MedDr, DeepDR-LLM, etc., showing great development potential in disease diagnosis and treatment.
However, before LVLMs are truly put into clinical practice, benchmarks need to be established to evaluate the effectiveness of the model. However, current benchmarks are usually based on specific academic literature and mainly focus on a single field, lacking different perceptual granularity, making it difficult to comprehensively evaluate the effectiveness and performance of LVLMs in real clinical scenarios.
In response to this, the Shanghai Artificial Intelligence Laboratory, together with the University of Washington, Monash University, East China Normal University and other research institutions, proposed the GMAI-MMBench benchmark. GMAI-MMBench is built from 284 downstream task datasets from around the world, covering 38 medical imaging modalities, 18 clinical-related tasks, 18 departments, and 4 perceptual granularities in the visual question answering (VQA) format, with complete data structure classification and multi-perceptual granularity.
The related research, titled "GMAI-MMBench: A Comprehensive Multimodal Evaluation Benchmark Towards General Medical AI", was selected for the NeurIPS 2024 Dataset Benchmark and published as a preprint on arXiv.

Paper address:
https://arxiv.org/abs/2408.03361v7
The "GMAI-MMBench Medical Multimodal Evaluation Benchmark Dataset" is now available on HyperAI's official website and can be downloaded with one click!
Dataset download address:
https://go.hyper.ai/xxy3w
GMAI-MMBench: The most comprehensive and open-source general medical AI benchmark to date
The overall construction process of GMAI-MMBench can be divided into 3 main steps:
First, the researchers searched hundreds of datasets from global public datasets and hospital data. After screening, unifying image formats, and standardizing label expressions, they retained 284 datasets with high-quality labels.
It is worth mentioning that these 284 datasets cover a variety of medical imaging tasks such as 2D detection, 2D classification, and 2D/3D segmentation, and are annotated by professional doctors, ensuring the diversity of medical imaging tasks as well as high clinical relevance and accuracy.

Next, the researchers classified all labels into 18 clinical VQA tasks and 18 clinical departments, making it possible to comprehensively evaluate the pros and cons of LVLMs in various aspects, which is convenient for model developers and users with specific needs.
Specifically, the researchers designed a classification system called a lexical tree structure, which divides all cases into 18 clinical VQA tasks, 18 departments, 38 modalities, etc. "Clinical VQA tasks", "departments", and "modalities" are words that can be used to retrieve the required evaluation cases. For example, the oncology department can select oncology-related cases to evaluate the performance of LVLMs in oncology tasks, which greatly improves the flexibility and ease of use for specific needs.
Finally, the researchers generated question-answer pairs based on the question and option pool corresponding to each label.Each question must contain image modality, task hint, and corresponding annotation granularity information. The final benchmark is obtained through additional validation and manual screening.

50 models evaluated, which one comes out on top in the GMAI-MMBench benchmark
In order to further promote the clinical application of AI in the medical field,The researchers evaluated 44 open source LVLMs (including 38 general models and 6 medical-specific models) and commercial closed-source LVLMs such as GPT-4o, GPT-4V, Claude3-Opus, Gemini 1.0, Gemini 1.5, and Qwen-VL-Max on GMAI-MMBench.

The results show that there are still five major deficiencies in the current LVLMs, as follows:
* There is still room for improvement in clinical applications: Even the best-performing model, GPT-4o, has met the requirements for practical clinical applications, but its accuracy is only 53.96%, which shows that current LVLMs are insufficient in dealing with medical professional problems and there is still huge room for improvement.
* Comparison of open source models with commercial models: Open source LVLMs such as MedDr and DeepSeek-VL-7B have an accuracy of about 44%, which is better than commercial models Claude3-Opus and Qwen-VL-Max on some tasks, and is comparable to Gemini 1.5 and GPT-4V. However, there is still a significant performance gap compared to the best performing GPT-4o.
* Most medical-specific models struggle to reach the general performance level of general-purpose LVLMs (about 30% accuracy), with the exception of MedDr, which achieves 43.69% accuracy.
* Most LVLMs perform unevenly across different clinical VQA tasks, departments, and perceptual granularities. In particular, in experiments with different perceptual granularities, the box-level annotation accuracy is always the lowest, even lower than the image-level annotation.
* The main factors leading to performance bottlenecks include perception errors (such as misidentification of image content), lack of medical domain knowledge, irrelevant answer content, and refusal to answer questions due to security protocols.
In summary, these evaluation results indicate that the performance of current LVLMs in medical applications still has much room for improvement and needs to be further optimized to meet actual clinical needs.
Gathering open-source medical data sets to help advance smart healthcare
In the medical field, high-quality open source datasets have become an important driving force for the advancement of medical research and clinical practice. To this end, HyperAI has selected some medical-related datasets for you, which are briefly introduced as follows:
PubMedVision Large-Scale Medical VQA Dataset
PubMedVision is a large-scale and high-quality medical multimodal dataset created in 2024 by a research team from Shenzhen Big Data Research Institute, the Chinese University of Hong Kong, and the National Health Data Institute, containing 1.3 million medical VQA samples.
In order to improve the alignment of graphic and text data, the research team used the large visual model (GPT-4V) to re-describe the images and constructed dialogues in 10 scenarios, rewriting the graphic and text data into a question-and-answer format, enhancing the learning of medical visual knowledge.
Direct use:https://go.hyper.ai/ewHNg
MMedC Large-Scale Multilingual Medical Corpus
MMedC is a multilingual medical corpus built by the Smart Healthcare Team of the School of Artificial Intelligence of Shanghai Jiao Tong University in 2024. It contains approximately 25.5 billion tokens covering 6 major languages: English, Chinese, Japanese, French, Russian and Spanish.
The research team also open-sourced the multilingual medical base model MMed-Llama 3, which performed well in multiple benchmarks, significantly surpassing existing open source models and is particularly suitable for customized fine-tuning in the medical vertical field.
Direct use:https://go.hyper.ai/xpgdM
MedCalc-Bench medical computing dataset
MedCalc-Bench is a dataset specifically designed to evaluate the medical computing capabilities of large language models (LLMs). It was jointly released in 2024 by nine institutions including the National Library of Medicine of the National Institutes of Health and the University of Virginia. This dataset contains 10,055 training instances and 1,047 test instances, covering 55 different computing tasks.
Direct use:https://go.hyper.ai/XHitC
OmniMedVQA Large-Scale Medical VQA Evaluation Dataset
OmniMedVQA is a large-scale visual question answering (VQA) evaluation dataset focusing on the medical field. This dataset was jointly launched by the University of Hong Kong and the Shanghai Artificial Intelligence Laboratory in 2024. It contains 118,010 different images, covering 12 different modalities, involving more than 20 different organs and parts of the human body, and all images are from real medical scenes. It aims to provide an evaluation benchmark for the development of large medical multimodal models.
Direct use:https://go.hyper.ai/1tvEH
MedMNIST medical image dataset
MedMNIST was released by Shanghai Jiao Tong University on October 28, 2020. It is a collection of 10 public medical datasets, containing a total of 450,000 28*28 medical multimodal image data, covering different data modes, which can be used to solve problems related to medical image analysis.
Direct use:https://go.hyper.ai/aq7Lp
The above are the datasets recommended by HyperAI in this issue. If you see high-quality dataset resources, you are welcome to leave a message or submit an article to tell us!
More high-quality datasets to download:https://go.hyper.ai/jJTaU
References:
https://mp.weixin.qq.com/s/vMWNQ-sIABocgScnrMW0GA
