From Harvard Philosophy Department to Protein Design Master, David Baker: AlphaFold Made Me Deeply Aware of the Power of Deep Learning
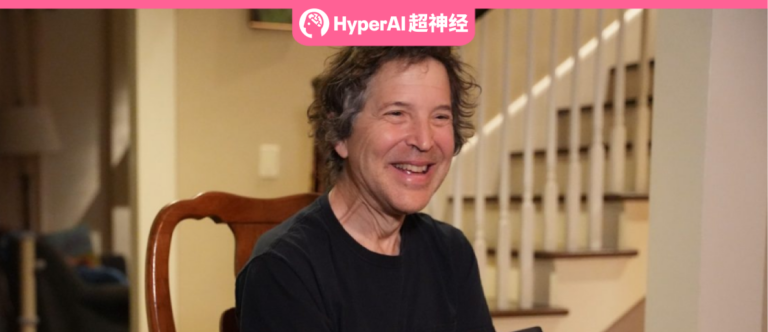
Professor David Baker of the University of Washington is a world-class expert in protein design. As a top expert in the field, Baker has published more than 700 research papers on proteins, with more than 177,000 citations. In October this year, Baker was awarded the 2024 Nobel Prize in Chemistry for his outstanding contributions to protein design.His influence in the academic world is evident.
However, Baker's influence goes far beyond this. In the industrial world, his name is also well-known.According to the official website of the Institute for Protein Design at the University of Washington, there are 21 companies in which Baker is directly involved as a founder. In April this year, Xaira Therapeutics, an AI pharmaceutical company he co-founded, not only attracted the 2022 Nobel Prize in Chemistry winner Carolyn Bertozzi to join, but also received a huge financing of US$1 billion, ranking first in the global Q2 financing list. Even investment giants such as Sequoia Capital and ARCH Venture Partners have endorsed it.
David Baker is a scientist who has many students in academia and has achieved extraordinary success in industry. What is his growth process and secret of success?

Image source: Institute for Protein Design
Starting from interest, we gather strength from all over the world to overcome difficulties
David Baker was born on October 6, 1962 in Seattle, Washington, USA, to a Jewish family. His parents were a physicist and a geophysicist. Despite this, Baker was not interested in science at first. He majored in philosophy and social studies at Harvard University, but now he thinks that "it was a complete waste of time. Many conversations were meaningless."
In his last year of college, Baker took a developmental biology course, where he witnessed a miraculous experiment: after adding protein denaturants, RNase lost its ability to cut RNA, but when the denaturants in the solution evaporated, the activity of RNase miraculously recovered. How do proteins find the correct conformation and function in an instant?This pursuit of clear answers to scientific questions excited him more than the ambiguity of philosophy.So he began to read the classic textbook "Molecular Biology of the Cell" and became more and more fascinated by biology.
Baker then joined the laboratory of Nobel Prize winner in Physiology or Medicine Randy Schekman and received his Ph.D. in biochemistry from the University of California, Berkeley in 1989.
After graduating with a Ph.D., Baker entered the laboratory of Professor David Agard at the University of California, San Francisco and began his postdoctoral research.There, he tried to use computers to analyze crystal structures and came up with the idea of using computers to predict protein structures.“There was a room in the structural biology lab where I worked as a postdoc, dedicated to solving crystal structures, and everyone was busy at a computer terminal, matching amino acid chains with electron density maps. I sat down and tried to do it for three minutes, and I had a splitting headache. That made me realize that I couldn’t do this, and I wanted to use computers to do something more meaningful.”
With this question in mind, in 1993, Baker returned to his hometown of Seattle, the University of Washington, and began to develop a software that could predict protein structure based on amino acid sequence, which later became the popular Rosetta. In addition, Baker also met his wife Hannele Ruohola-Baker at the University of Washington, a professor of biochemistry at the University of Washington, and the two have a son and a daughter.

In 1998, Rosetta was officially released.Based on the principles of physics, Rosetta can perform energy minimization calculations on protein conformations to predict the most stable three-dimensional structure, that is, a stable conformation of the protein close to its natural state. In order to verify Rosetta's performance in protein structure prediction, the Baker team actively participated in the CASP competition. In this competition, contestants will conduct blind test predictions on a batch of protein structures that have been experimentally resolved but not yet made public, in order to evaluate the accuracy of different algorithms. Since then, Rosetta has gradually emerged in CASP and made history at CASP6 in 2004. For the target protein T0281, Rosetta achieved ab initio protein structure prediction with near-atomic accuracy for the first time, and once became a leader in the field of protein structure prediction.
Rosetta Address:https://levitate.bio/rosetta
However, more accurate predictions mean more computing resources. "When we started doing protein structure prediction, we found that this work required a lot of computing resources. We kept buying new computers, which was not only very expensive, but we soon ran out of space to put them.Therefore, we launched the Rosetta@home project.Invite people from all over the world to use their idle computing power to calculate protein structures. This is a screen saver, and when the computer is calculating, the screen will show the protein being folded. "Baker said.
Today, Rosetta has been widely adopted in academic and industrial environments and has become a standard tool for structural biology and drug discovery. In order to continuously improve the Rosetta software,Baker also created an academic community, Rosetta Commons.This community brings together scholars from more than 60 institutions around the world, covering fields such as chemistry, biology, physiology, physics, engineering, mathematics and computer science. Every year, the community holds meetings for members to share results and exchange ideas. Today, Rosetta Commons has become a large-scale international cooperation project.
Rosetta@home Address:https://boinc.bakerlab.org

Inspired by the Rosetta@home project, Baker deeply realized the importance of "human wave tactics". If one wants to quickly make breakthrough progress in an unknown field, win-win cooperation is the long-term way. In 2008, Baker's team officially launched Foldit, an online puzzle game about protein folding that professionals and non-professionals can participate in. Baker said: "Our dream is that people around the world work together to make significant contributions to science and global health."
In Foldit,Players use the tools in the game to fold the selected protein structure as perfectly as possible. The highest-scoring solutions will be analyzed by researchers to evaluate their applicability in reality and then applied to targeted therapy, etc. It is worth mentioning that Foldit has attracted more than 400,000 participants, and some players are listed as contributors in Baker's paper. For example, in a paper accepted by Nature in 2011, Foldit players helped crack the crystal structure of the M-PMV retroviral protease, which has troubled scientists for 15 years. In just 10 days, the players built a sufficiently accurate 3D model of the enzyme to successfully perform molecular replacement and subsequent structural determination.
Foldit Address:https://fold.it

In the years that followed, Rosetta and Foldit became very popular in the field of protein structure. If this trend had continued, the other half of this year's Nobel Prize in Chemistry "for contributions to protein structure prediction" might not have been awarded to Demis Hassabis and John Jumper. The turning point of everything came at the end of 2020.
Responding to AlphaFold2 with open source
At the 14th CASP competition held in November 2020, AlphaFold2 "came out of nowhere". As a major achievement selected as one of the top ten breakthroughs of the year by Science, AlphaFold2's accuracy in predicting protein structure directly crushed all other teams, and Rosetta brought by Baker's team was "far behind". The organizers even directly announced that AlphaFold 2 successfully solved a problem that had plagued scientists for 50 years.

Unlike Rosetta, which focuses more on methods based on physical principles and predicts protein structure by minimizing calculated energy, AlphaFold2 combines deep learning with knowledge in related fields such as physics and biology to achieve end-to-end prediction of protein three-dimensional structure information.This achievement caused a huge sensation in the scientific community and was hailed as a milestone in protein research. However, DeepMind did not disclose the specific details of AlphaFold2 at the time.
In this regard, Baker said, "Everyone was stunned. There was a lot of media coverage at first, and then there was no news. It was strange that we could not continue to develop on this basis when our field made great progress."
Like his teacher Randy Schekman, Baker advocates open source and sharing of science. His teacher chose to "declare war" on the three major journals.Baker is determined to develop an open source model that can compete with AlphaFold2.
*Randy Schekman advocates open and free access to scientific literature, strongly criticizes closed access journals such as Nature, Science, and Cell, and announces that he will never submit to these journals

Drawing on AlphaFold2, Baker and other members of the lab worked hard for several months and released the deep learning model RoseTTAFold. RoseTTAFold uses a unique three-track neural network architecture that can simultaneously consider the sequence pattern, amino acid interactions, and possible three-dimensional structure of proteins, in which one-dimensional, two-dimensional, and three-dimensional information flow into each other, allowing the neural network to infer the relationship between the chemical composition of the protein and its folded structure. Using RoseTTAFold, researchers have calculated hundreds of new protein structures, including many unknown proteins in the human genome, and they have also generated proteins that are directly related to human health, such as those associated with inflammatory diseases and cancer cell growth.
It is worth mentioning that RoseTTAFold's computing energy consumption and time are lower than AlphaFold2. With only an RTX 2080 graphics card, it can calculate the protein structure within 400 amino acid residues in just 10 minutes. The researchers pointed out that "without using this kind of software, it may take a team of scientists several years to determine a protein structure." Baker understood that it was time to make RoseTTAFold public.
RoseTTAFold open source address:https://github.com/RosettaCommons/RoseTTAFold
In June 2021, Baker published a preprint paper detailing the RoseTTAFold technology roadmap. A few days later, DeepMind CEO Demis Hassabis announced on Twitter that they would release the AlphaFold2 paper and source code. On July 15 of the same year, the RoseTTAFold and AlphaFold2 papers were published in Science and Nature respectively. Science magazine also named RoseTTAFold and AlphaFold as the 2021 Breakthrough Technology of the Year.This competition between academia and business finally ended perfectly.

Do something challenging! Introducing deep learning into protein design
After the news of this year's Nobel Prize in Chemistry was announced, relevant personnel conducted a brief telephone interview with Baker. When asked how he viewed the competitive relationship between RoseTTAFold and AlphaFold, Baker said that he himself never felt that he was a competitor to DeepMind.

Image source: Institute for Protein Design, University of Washington
"For many years, we have been developing physics-based protein structure prediction and design methods. But when John and Demis developed AlphaFold2, I deeply realized the power of deep learning. They are great inspirers of the power of deep learning." Of course, with this power,Baker not only used deep learning for protein structure prediction and launched RoseTTAFold, but also used it for protein design.
Baker's student Shen Hao believes that his teacher "has a spirit of innovation and taking big steps forward", focusing on doing important and challenging things, such as designing new proteins. In Baker's view, humans are facing many new and urgent problems, such as new diseases caused by extended life span and environmental pollution. If we wait for natural evolution to solve the problems, it may take millions of years, but through protein design, we can quickly develop new proteins to solve current problems.
In fact, Baker's team thought a long time ago that since amino acid sequences can be input into Rosetta to predict protein structures, is it possible to use the software in reverse, input a desired protein structure, obtain the corresponding amino acid sequence suggestions, and introduce the designed sequence genes into bacteria to enable the bacteria to produce the desired protein?
Based on this,In 2003, Baker's team successfully designed the world's first new protein, Top7.This groundbreaking discovery has greatly inspired research in related fields.

Similarly, after realizing the great potential of deep learning for protein design, Baker also began to think: Can deep learning be used in reverse to generate amino acid sequences for designing new functional proteins? Around this topic, he led the team to develop a series of results.
Baker published a paper titled "De novo design of protein structure and function with RFdiffusion" in the journal Nature. The researchers fine-tuned the RoseTTAFold structure prediction network in the protein structure denoising task.A generative model RFdiffusion was developed.The model performs well in protein binder design, enzyme active site scaffold design, etc. More importantly, the model has excellent versatility and is open source.
RFdiffusion project address:https://github.com/RosettaCommons/RFdiffusion
At the same time, in order to expand the capabilities of RFdiffusion,Baker also developed a protein sequence design method ProteinMPNN based on deep learning.ProteinMPNN takes protein structure as input and generates a new amino acid sequence that can fold into the corresponding skeleton in 1 second. Combined with structure generation tools like RFdiffusion, it can be used to design proteins with unprecedented sequences, structures and functions. In addition, the study also showed that on the natural protein skeleton, the sequence recovery rate of ProteinMPNN is 52.4%, while the physical design based on Rosetta in the past was only 32.9%. The study was accepted by Science with the title "Robust deep learning-based protein sequence design using ProteinMPNN".
ProteinMPNN project address:https://github.com/dauparas/ProteinMPNN
In addition, Baker's team also optimized the previously mentioned structure prediction tools Rosetta and Foldit.By introducing new modules and algorithms into the software, both are not limited to protein structure prediction, but also expanded to antibody design, enzyme design and small molecule docking. In this regard, Baker said, "Foldit was originally created for protein structure prediction, but now it has turned to protein design. We will continue to update levels for players, and it will continue to change as our research interests change."

Combining AI techniques with physical methods, Baker's lab has created many new proteins.For example, proteins that can neutralize viruses, target cancer cells, or even act as catalysts for chemical reactions. In addition, Baker is designing proteins that can bind to inorganic materials and exploring the possibility of using proteins to regulate the growth of inorganic crystals. This research is expected to be applied to fields such as semiconductor manufacturing.
Promote technology implementation by establishing a company
Baker's teacher David Agard once commented, "David Baker's work has almost single-handedly promoted the development of the field of protein design." Indeed, before the end of 2024, Baker has published more than 110 papers, which is an incredible number of achievements. But what is even more surprising is that every time Baker thinks that the technology he is studying is basically mature, he will set up a new company or invest in a company he founded in the past to incubate it, thereby promoting the industrialization of the technology. According to the official website of the Institute for Protein Design at the University of Washington,Baker has been directly involved as a founder in 21 companies, and he also serves as a consultant to others.

David Baker Founder/Co-founder/Scientific Co-founder
Specifically, Xaira Therapeutics, a company that was established in April this year, applied the aforementioned RFdiffusion and ProteinMPNN.The company is committed to redesigning and developing drugs through emerging AI technologies. Dr. Marc Tessier-Lavigne, former president of Stanford University, serves as CEO and Baker is a co-founder. It is worth noting that several scientists from Baker's laboratory have also joined Xaira full-time.
Xaira can train models with high quality by integrating massive amounts of data on biological features related to molecules and human diseases. In addition, the company has established an industrialized dry and wet experimental platform that can test the adhesion of proteins to specific cell targets in the laboratory and evaluate key properties such as stability. The data obtained is quickly fed back into the protein model, thereby realizing the next iteration of molecular design.
Xaira official website:https://xaira.com
Archon Biosciences, founded in 2023, is committed to designing a new type of biological drug - antibody cage (AbC) through generative AI.AbC combines AI design with structural control to fully control the direction, binding domain valency, size, shape and stiffness of antibodies. This structural control enables precise biodistribution and target engagement on cells, and combined with internal clinical data, it can quickly verify the effectiveness of antibodies. The company has received support from many companies including NVIDIA, and the technology used is based on the results recognized by Baker in the 2024 Nobel Prize in Chemistry.
Archon official website:https://www.archon.bio

In addition, Monod Bio launched the world's first completely de novo protein product, LuxSit™ Pro, a luciferase for life science research and diagnostics, in July this year.In this regard, Baker said: "This is an important milestone in biology and computer science. I believe that in the next few months or years, we will see more proteins designed from scratch transformed into mature commercial products." The technology originated from a paper published by Baker in Nature in 2023.

There are also companies such as Arzeda, founded in 2009, Cyrus Biotech, founded in 2014, and A-Alpha Bio, founded in 2018, which have actively introduced Baker's latest AI technology, hoping to develop more new proteins for the manufacture of new drugs, vaccines, disease treatments, and even new materials.
Arzeda official website:https://arzeda.com/
Cyrus Biotech official website:https://cyrusbio.com/
A-Alpha Bio official website:https://www.aalphabio.com/
From the initial philosophical exploration to the current protein design "magician", every step of Baker is full of desire for the unknown and persistence in innovation. He has always insisted that win-win cooperation is the long-term solution, and has inspired countless researchers and science enthusiasts around the world to devote themselves to the development of this field with the spirit of openness and sharing. His research results have not only made great breakthroughs in academia, but also moved from the laboratory to the industry, empowering disease treatment, food production, materials science and other fields, bringing more possibilities to human life.
References:
1.https://news.bioon.com/article/9068e156469f.html
2.https://news.qq.com/rain/a/20241010A02IB300
3.https://zh.wikipedia.org/zh-cn/Rosetta@home
4.https://www.ipd.uw.edu/2021/07/rosettafold-accurate-protein-structure-prediction-accessible-to-all/
5.https://news.qq.com/rain/a/20241010A04VNA00
6.https://m.thepaper.cn/newsDetail_forward_28994096
7.https://www.nobelprize.org/prizes/chemistry/2024/baker/interview/
8.https://finance.sina.com.cn/tech/roll/2024-10-10/doc-incsarnm2004532.shtml
9.https://news.qq.com/rain/a/20241011A02XB000