Breaking the 1200°C High Temperature Performance Limit! Beijing University of Science and Technology Uses Machine Learning to Synthesize 24 Refractory High Entropy Alloys With Excellent Room Temperature Ductility
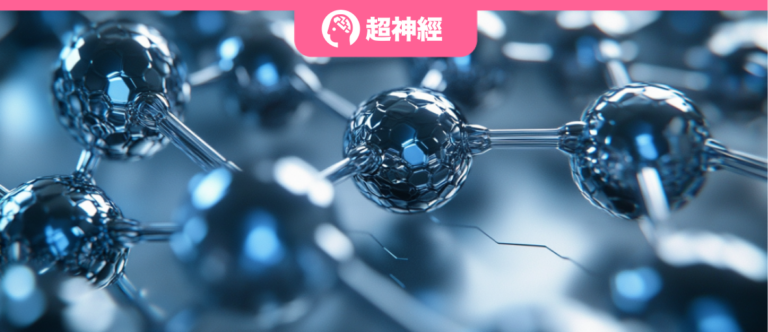
In engineering applications, such as gas turbines, nuclear reactors, and aviation propulsion systems, there is a strong demand for metal alloys with excellent high-temperature mechanical properties. Due to the inherent limitations of the material's melting point, the temperature resistance of traditional nickel-based (Ni) high-temperature alloys has reached its limit. In order to meet the demand for the development of high-temperature structural materials,Refractory high entropy alloys (RHEAs) were proposed in 2010 and have attracted much attention due to their ability to maintain high strength at temperatures of 1000°C and above.
By adding different high-melting-point refractory elements, some RHEAs have shown high-temperature strength comparable to that of high-temperature alloys. In addition, the structural stability brought about by the high entropy effect makes RHEAs have great application potential at high temperatures. However, although the addition of refractory elements to RHEAs will increase their high-temperature strength, their room-temperature ductility will be greatly reduced.For example, the compressive fracture strain of most RHEAs at room temperature is less than 10%, making them difficult to further process.
In order to develop RHEAs with both good high temperature strength and room temperature ductility,Many studies have been conducted. In the past, RHEAs were designed by adjusting the composition of certain elements, but this design mostly relied on the experience and intuition of researchers and had great uncertainty. In addition, the possible composition space of RHEAs is large, containing billions of candidate components. This complex composition and its huge search space severely limit our rapid discovery of promising alloys.
In recent years, the use of machine learning (ML) to solve complex problems in materials science has received widespread attention.Su Yanjing's team at the University of Science and Technology Beijing designed a multi-objective optimization (MOO) framework that combines ML, genetic search, cluster analysis, and experimental feedback, and targeted the RHEAs composition space to search for alloys with optimal high-temperature strength and room-temperature ductility.
Specifically, the research team synthesized 24 RHEAs and determined that the ZrNbMoHfTa alloy has potential for high-temperature applications.0.13Nb0.27Mo0.26Hf0.13Ta0.21 The alloy exhibits excellent mechanical strength, with a yield strength of nearly 940 MPa at 1200°C and a room temperature fracture strain of 17.2%. The alloy's remarkable heat resistance and good structural stability indicate its potential for structural applications at high temperatures, while room temperature ductility improves the alloy's processing performance.
The related results were published in Engineering under the title “Machine-Learning-Assisted Compositional Design of Refractory High-Entropy Alloys with Optimal Strength and Ductility”.
Research highlights:
* This study proposes a method to accelerate the discovery of RHEAs with high-temperature strength and room-temperature ductility by integrating ML, genetic search, cluster analysis, and experimental feedback
* Zr0.13Nb0.27Mo0.26Hf0.13Ta0.21 The high yield strength of the alloy at 1200°C exceeds all reported RHEAs, and 1200°C also breaks the service temperature limit of nickel-based high-temperature alloys.
* This work lays a foundation for the optimization of multiple properties of RHEAs and can also be further applied to the composition design of other alloys or material systems

Paper address:
https://www.sciencedirect.com/science/article/pii/S2095809924005113
Follow the official account and reply "Refractory High Entropy Alloy" to get the complete PDF
The open source project "awesome-ai4s" brings together more than 100 AI4S paper interpretations and provides massive data sets and tools:
https://github.com/hyperai/awesome-ai4s
Datasets: Use statistical methods to build more datasets
To build a machine learning model, the researchers collected sample data of alloys containing refractory metal elements from Group 4 (Ti, V, Cr), Group 5 (Zr, Nb, Mo), Group 6 (Hf, Ta, W) and aluminum (Al) from the literature. All alloys were prepared by arc melting to reduce performance differences caused by material processing. The data entries of the initial data set included reported composition (ci) and mechanical properties (y), and RHEAs with added interstitial elements (such as oxygen, nitrogen, and carbon) were not considered. The collected cast alloys contained single-phase or multi-phase structures. Accordingly,Two independent data sets of 54 and 145 alloy samples were assembled for the prediction of the two target properties, high temperature strength and room temperature ductility, respectively.
Given that the search space of the RHEAs system is very large, it is not enough to rely solely on predictions based on ML models trained on small data to find materials with the best performance. A utility function can be defined to select some alloys for experiments to maximize the expected utility. In this study, the researchers used the target performance expected improvement (EI) as a utility metric to balance exploration (aimed at improving the prediction model) and exploitation (aimed at finding the best prediction results). Specifically, the well-known statistical method "bootstrap" was used for sampling with replacement to construct more data sets, which were used to train different ML models.
Model architecture: MOO strategy integrating ML, genetic search, cluster analysis, and experimental design
The figure below (a) shows the MOO strategy used in this study for the optimal design of RHEAs.The overall workflow is divided into 3 parts:
* First, machine learning: As shown in the left figure below, select the ML model to calculate the expected improvement (EI) value of a given alloy target property
* Second, Genetic search: As shown in the middle of the figure below, the non-dominated sorting genetic algorithm (NSGA) II is used to search for candidate alloy compositions based on the expected improvement value of the target performance.
* Third, Experimental feedback: As shown in the right side of the figure below, experimental feedback on alloy selection and experimental verification through cluster analysis

Machine Learning: SVR. R Model for Estimating High-Temperature Yield Strength and Room-Temperature Fracture Strain
As shown in the figure above, the researchers trained the ML model to predict the target properties of the alloy by establishing a relationship between composition and properties. The molar composition of the 10 elements involved in the collected alloy was directly used as input features, and the two target properties (high temperature yield strength and room temperature fracture strain) were the outputs of the ML model.
The study considered 9 commonly used regression models, and used root mean square error (RMSE), mean absolute error (MAE) and Pearson correlation coefficient r² to evaluate model performance. Based on the results of the study, the svr. r model was selected as the final model for evaluating high temperature yield strength and room temperature fracture strain in subsequent genetic searches.
Genetic Search: Searching for Candidate Alloy Compositions
Genetic search has been previously used for the composition design of high entropy alloys (HEAs) and nickel-based superalloys. Here, the EI values calculated based on ML predictions are used as input to the NSGA-II algorithm for a heuristic search. After selection, crossover, and mutation, the Pareto front (PF) and the front of the dominating solution are generated at the end of each genetic iteration.
More specifically, after the population is initialized, the researchers used bootstrap sampling to construct additional data sets based on the training data. After training the model with bootstrap samples, the average value of the performance and its associated uncertainty can be obtained to calculate the EI value of each alloy. After several generations of selection, crossover, and mutation, a PF (Pareto font of EI) of the EI results is obtained. Finally, after 100 generations and 100 randomly selected initial populations, the results are summarized to obtain the converged optimal PF.
Experimental feedback: including alloy selection and experimental verification through cluster analysis
To guide the synthesis of unknown compounds, the researchers performed cluster analysis on the PF and used the k-means method to select alloy candidates from the cluster centers, as shown in Figure (c) below. This step enabled iterative improvement of the ML model by incorporating the measurement results into the training dataset.

Research results: ZrNbMoHfTa alloy system confirmed to have potential for high temperature applications
Using the methods mentioned above, the researchers synthesized and characterized 24 predicted alloy compositions.As shown in the figure below, the study found that the high temperature yield strength and room temperature fracture strain of four of the alloys reached an excellent combination of 714-1061 MPa and 17.2%-50.0% respectively. The researchers compared the alloy properties in the training data set (ie, T data, P1-P7) with the results after MOO optimization (E24, E19, E17 and E21) as follows:

First, let’s look at the performance improvement of RHEAs after MOO optimization: To illustrate the results of the MOO strategy, the researchers compared the two target properties of the alloy in the original Pareto frontier (PF) and the new PF, as shown in the table above.After optimization, the performance of both targets has been significantly improved.
Specifically, considering alloys with high ductility (>50%), the high temperature yield strength (HT) of E24 alloy is almost 2.5 times that of P1 (i.e., a typical TaNbHfZrTi alloy, whose high temperature yield strength is only 295 MPa); similarly, considering alloys with high yield strength (>1000 MPa) at 1000 °C, the fracture strain of E21 is almost 3 times that of P6; alloys P2, P3, P4 and P5 are also dominated by multiple optimized materials. Compared with P2, the yield strength of E24 increased by 41.7%, while its fracture strain also increased by more than 54.3%. E19 and E17 alloys also showed improvements in high temperature strength and room temperature ductility.
In summary, compared with the typical NbMoTaW alloy (high temperature yield strength of 548 MPa, fracture strain of 2.6%) and NbMoTaWV alloy (high temperature yield strength of 842 MPa, fracture strain of 1.7%),Most of the newly designed RHEAs have shown significant improvements in both high temperature yield strength and room temperature ductility.
Next, let's look at the structure and softening resistance of the optimized alloy: The researchers further studied the phases of the optimized RHEAs before and after compression deformation at 1000°C to explore their structural stability in potential high-temperature engineering applications. According to the XRD results shown in Figures (a) and (b) below, the phases of the cast alloys E24, E19, and E17 are composed of disordered body-centered cubic (BCC) solid solutions, while alloy E21 exhibits a BCC structure accompanied by a small amount of Laves phase. The XRD spectrum shows thatThe phase structure of the optimized RHEAS before and after high-temperature deformation is basically consistent, showing the good structural stability of the optimized RHEAS.

(a, b) Phase structure; (a) before heat treatment and deformation and (b) after heat treatment and deformation
The researchers also compared the yield stress of Alloy E21 under high temperature deformation with data from the literature, showing a significant improvement in softening resistance. The improvements in multiple properties suggest that these RHEAs have the potential to replace traditional high-temperature alloys.
In short, the researchers identified a promising alloy system, ZrNbMoHfTa, specifically the composition Zr0.13Nb0.27Mo0.26Hf0.13Ta0.21, exhibiting a yield strength close to 940 MPa at 1200°C and good room temperature ductility with a fracture strain of 17.2%.The high yield strength of this composition at 1200°C exceeds that of reported RHEAs, and 1200°C is beyond the service temperature limit of nickel-based superalloys. The heat resistance and good structural stability of this alloy indicate that it has considerable potential in structural applications at extreme temperatures.
Artificial intelligence has great application value in materials science
Materials science can be said to be one of the pillar disciplines for the rapid development of modern industry. Finding new materials with target properties through as few experiments as possible is the key to accelerating material discovery. However, due to the complex composition, structure, and process of materials, the space for candidate materials is huge, making the efficient design of new materials difficult to achieve. In the past, scientists searched for new crystal structures by adjusting known crystals or trying new combinations of elements. This is an expensive and time-consuming trial-and-error process that usually takes several months to obtain limited results.Today, AI has completely changed this situation.
At the end of November 2023, Google's DeepMind published a blockbuster paper in Nature magazine, claiming that they had developed an artificial intelligence reinforcement learning model for materials science, Graph Networks for Materials Exploration (GNoME), and through this model and high-throughput first-principles calculations, they found more than 380,000 thermodynamically stable crystalline materials, which is equivalent to "adding 800 years of intellectual accumulation to mankind", greatly accelerating the speed of discovering new materials. In December of the same year, Microsoft released MatterGen, an artificial intelligence generation model in the field of materials science, which can predict new material structures on demand based on the required material properties.
In June 2024, scientists from the UK and Japan, including Akiyasu Yamamoto, used machine learning technology to design a research system that combines researcher-driven and data-driven methods, and successfully manufactured the world's strongest known iron-based superconducting magnet. The latest research is expected to promote the development of a new generation of magnetic resonance imaging (MRI) technology and future electrified transportation technology. The related paper, titled "Superstrength permanent magnets with iron-based superconductors by data- and researcher-driven process design", has been published in Nature's subsidiary journal NPG Asia Materials.

In summary, by combining machine learning and deep learning, scientists can better predict the performance of materials, simulate the structure and properties of molecules, optimize the design and synthesis of materials, explore the relationship between the microstructure and macroscopic properties of materials, etc. These applications will not only help improve the research level of materials science, but also bring more innovation opportunities to fields such as materials design and manufacturing.
References:
1.https://www.sciencedirect.com/science/article/pii/S2095809924005113
2.https://phys.org/news/2024-09-machine-discovery-high-temperature-alloys.html
3.https://m.huxiu.com/article/2748177.html
4.https://www.sohu.com/a/808673682_120136032
