Event Review丨Shanghai Jiaotong University/Zhejiang University/Tsinghua University/OpenBayes Experts, Covering Medical/geographic Information/urban Complex Systems/new Scientific Research Paradigms
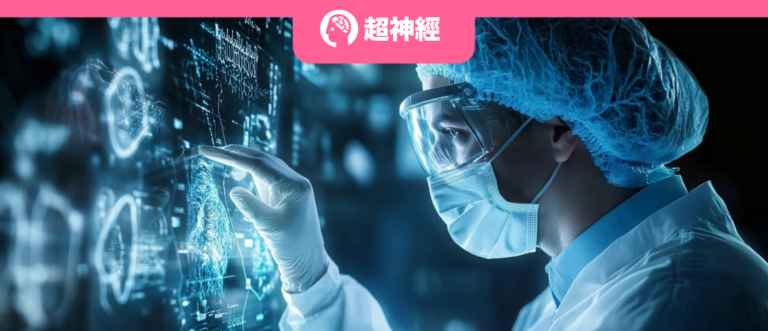
This year's Nobel Prize's "preference" for AI has once again brought AI for Science to the public's attention. It can even be said to be a milestone event, indicating that a new scientific research paradigm has become a general trend. Looking back at the development of science, from experimental science to theoretical science, and then to computational science and data-intensive science, each paradigm shift has greatly promoted the progress of human civilization, and in the entire iterative process, the core role of data has never changed.
Now, in the era of AI for Science, the value of data can be further explored.What innovations will the basic scientific research field usher in? How can researchers in vertical fields embrace AI?
Facing the development trend of AI for Science, HyperAI has promoted the development of AI4S in China through interpreting cutting-edge achievements, reporting on typical enterprises, and holding academic activities, and has built a communication platform for domestic researchers.As a co-production community, HyperAI held an open source AI forum on AI for Science during the COSCon'24 9th China Open Source Annual Conference and the 10th Anniversary Carnival of Open Source Society.
We are honored to have invited Wang Chenhan, founder and CEO of OpenBayes Bayesian Computing, Qi Jin, a specially-appointed researcher at the School of Earth Sciences of Zhejiang University, Xie Weidi, a tenured associate professor at Shanghai Jiao Tong University and a young scientist at the Shanghai Artificial Intelligence Laboratory, and Ding Jingtao, a postdoctoral researcher at the Center for Urban Science and Computing, Department of Electronic Engineering, Tsinghua University.
In this forum, four lecturers shared their insights on topics such as medical artificial intelligence (AI4Health), geographic information artificial intelligence (GeoAI), scientific research intelligent cloud platform, and AI-driven urban complex systems from the perspectives of knowledge popularization, case introduction, and trend analysis.
Then,We will further report the key points of each lecturer's sharing in the form of text records and videos.Stay tuned!
A new paradigm of scientific research driven by AI: a comprehensive upgrade of statistical methods by artificial intelligence
OpenBayes is a leading artificial intelligence service provider in China. In the process of empowering top universities and research institutions in China, it also has a deep insight into the development of AI for Science. Regarding the value of machine learning in promoting the development of cutting-edge research,The company's founder and CEO Wang Chenhan proposed an innovative formula: Scale data X model structure = AI scientific research achievements – traditional research.
That is, in the scientific research process, by applying large-scale data to effective model structures, it is possible to significantly surpass traditional methods in practical research topics in any industrial field. This is an important reason why AI-driven scientific research has achieved a 2-5 times growth in the past two years.

At the same time, Wang Chenhan also emphasized that if the model structure remains unchanged and the amount of data is increased blindly, marginal effects may occur, making it difficult to improve performance; similarly, when the data scale is certain, the model parameters are not necessarily the larger the better.Only when the data scale and parameter scale are increased equally, the prediction error rate will drop to a lower level.
In addition, he focused on comparing the differences between traditional research methods and AI research methods. Among them, traditional research methods are highly dependent on the characteristics and problem definition capabilities of researchers themselves, and only use "small data", which is questionable in terms of generalization and expansion capabilities.The AI research method requires the introduction of large-scale, high-quality data and the use of machine learning for feature extraction, so that the scientific research results produced are still effective in real-world problems.
Finally, Wang Chenhan also introduced how OpenBayes Bayesian computing enables AI for Science——Encapsulate scientific research data elements such as open source data sets, AI/HPC tutorials, open source/private models, etc. into a cluster software.Help scientific researchers achieve one-stop connection in model construction, model reasoning, industrial software computing, etc.
GeoAI and its interdisciplinary geoscience applications
In the field of geographic information science, the development of stereoscopic observation technologies in the air, space, land and underground has promoted a data explosion, giving rise to the concept of spatiotemporal big data. However, the massive data generated by spatiotemporal processes of different scales is also a major challenge for information mining.
Dr. Qi Jin, a researcher at the School of Earth Sciences at Zhejiang University, said:Geographic relationship regression analysis is a hot topic in geographic modeling research.Developing new spatial regression analysis methods and improving the ability to analyze and mine geographical relationships have important theoretical value and practical significance for understanding social processes and geographical phenomena.

In response to this, Dr. Qi Jin and his team integrated the spatial weighting idea with the neural network model.A geographic neural network weighted regression model (GNNWR) is proposed.The spatial regression method has expanded its ability to fit and explain nonlinear relationships between geoscientific elements.The team also developed an open source model library based on PyTorch - spatiotemporal intelligent regression model.Its methodology system has supported more than 30 studies in geography, geology, oceanography, atmosphere and other fields.
In terms of application, he introduced the performance of the GNNWR model in scenarios such as urban housing price prediction, air pollution analysis, and offshore ecological environment modeling:
* Establishing spatiotemporal relationships between sparsely sampled points and unknown points along the coast and solving for spatiotemporal non-stationary weights to obtain high spatiotemporal resolution distribution of dissolved silicate (DSi) in coastal waters;
* GNNWR can accurately describe the spatial non-stationarity in urban environments, thereby enabling regression modeling of urban geographic processes such as housing prices;
* Using processed AOD, DEM, climate factor data and PM2.5 data collected by substations, establish spatial non-stationary regression relationships and estimate PM2.5 concentrations;
* Integrating Shapley's interpretability theory into GNNWR enables accurate prediction and interpretation of geological mineralization in complex spatial environments.
The team's primary goal: to build a general medical artificial intelligence system
Xie Weidi, a tenured associate professor at Shanghai Jiao Tong University and a young scientist at the Shanghai Artificial Intelligence Laboratory, has been deeply involved in computer vision. After returning to China in 2022, he has devoted himself to the research of medical artificial intelligence. In this forum,He shared the team's achievements from multiple perspectives including open source dataset construction and model development.
Professor Xie Weidi introduced that most of the knowledge in medicine, especially evidence-based medicine, is summarized from human experience. If a beginner can exhaust all medical books, he can at least become a medical expert in theory. Therefore,During the model training process, we also hope to inject all medical knowledge into it.

However, in the medical field, high-quality data is relatively scarce due to privacy issues.So after returning to China, Professor Xie Weidi and his team began to build a large-scale medical data set.Specifically:
* Collected 1.6 million large-scale image-caption pairs from PubMed Central and constructed the PMC-OA dataset;
* Generated 227,000 medical visual question-answer pairs from PMC-OA to form PMC-VQA;
* A Rad3D dataset was constructed by collecting 53,000 cases and 48,000 multiple image-caption pairs from the Radiopaedia species.
* PubMed Central (PMC) is a free, full-text database created and maintained by the National Center for Biotechnology Information of the United States, specializing in open access scholarly articles in the fields of biomedicine and life sciences.
* Radiopaedia provides high-quality and free access to radiology and medical imaging knowledge and is a collaborative, open editorial platform where radiologists/students and other healthcare professionals can contribute cases, articles, and imaging examples.
In terms of model construction,He mainly introduced the medical-specific language model or visual-language model developed by the team.For example, PMC-LLaMA, multilingual medical model MMedLLaMA, and general segmentation models such as SAT.
A spatiotemporal generative modeling approach for complex urban systems
Dr. Jingtao Ding from the Center for Urban Science and Computational Research, Department of Electronic Engineering, Tsinghua University, focuses on the generative modeling and application of AI-driven spatiotemporal complex systems.Dr. Jingtao Ding focused on introducing spatiotemporal generative AI for modeling complex urban systems.
Dr. Ding Jingtao introduced that the main difficulties currently faced in modeling complex urban systems include the dominance of high-dimensional, multimodal spatiotemporal data; the huge scale of the system, and the interaction between various elements cannot be ignored; the data distribution of each system is very different, making it impossible to use a universal model, etc.

In response to this, he and his team began to explore spatiotemporal generative AI for modeling complex urban systems.A diffusion model guided by physical knowledge is proposed for crowd flow simulation; a diffusion model enhanced by network dynamics is proposed for system resilience prediction; and a spatiotemporal GPT enhanced by prompt learning is proposed for general spatiotemporal prediction.
Specifically:
* The pedestrian flow simulation model SPDiff is based on a real pedestrian flow dataset, achieving a performance improvement of 6.5%-37.2 and better generalization ability under small samples;
* The system resilience prediction model generates observation samples of resilient/non-resilient systems based on the diffusion model, using only 20 (2%) labeled samples and maintaining a prediction accuracy of 87% (F1 score);
* The universal spatiotemporal prediction model UniST collects 20+ spatiotemporal data sets and more than 130 million spatiotemporal sample points. It uses an external spatiotemporal memory network to store valid spatiotemporal patterns and generate prompt vectors to achieve migration generalization.
Final Thoughts
As one of the earliest open source communities paying attention to the development of AI for Science, HyperAI will continue to pay attention to cutting-edge innovative achievements at home and abroad, and provide everyone with practical interpretations and reports. At the same time, we are also building a platform for communication and exchange for researchers through a variety of online live broadcasts and offline academic forums. Research groups engaged in related research are welcome to submit articles to us or share their latest research results!
