Combining Supercomputing and Intelligent Computing, the Chinese Academy of Sciences Team Built a Photovoltaic Multi-time Scale Power Prediction Model That Can Integrate Meteorological Data
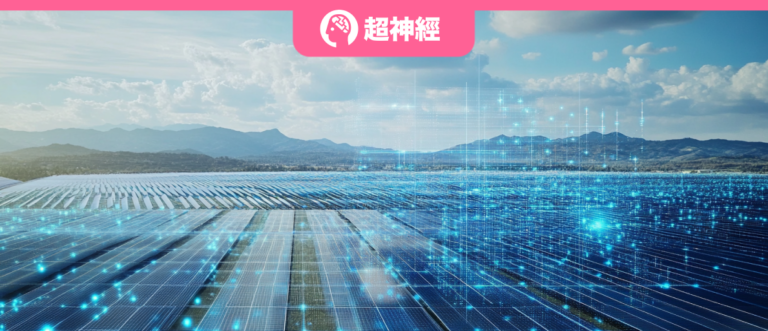
At the 20th CCF National High Performance Computing Academic Annual Conference (CCF HPC China 2024) - Intelligent Supercomputing Fusion Technology Forum in Numerical Simulation Engineering Applications,Wan Meng, an engineer from the Artificial Intelligence Department of the Computer Network Information Center of the Chinese Academy of Sciences, shared the team's practical applications and methodologies accumulated in photovoltaic power generation and power load, as well as cutting-edge research trends in deep learning in the field of time series prediction, providing new technical ideas and methods for new energy prediction.

HyperAI has compiled and summarized this in-depth sharing without violating the original intention.It is divided into four parts: time series, new energy background overview, research basis, and research progress.The following is the transcript of the speech.
Time series applications are extensive and cover many aspects
The time series we study aims to make scientific predictions and judgments on the future development trends or status of specific objects.In our real life, time series are widely used, covering many aspects such as traffic flow, financial economics, meteorological weather, virus transmission, and energy.

The current research directions of time series are mainly divided into four parts.Part 1 is time series future prediction,We can predict future sequences based on our known historical sequences, including photovoltaic forecasts, weather forecasts, stock forecasts, etc.The second part is the time series gap filling.It includes public opinion monitoring, sensor failure, industrial equipment maintenance, etc. For example, sensor failure in industrial scenarios may lead to the loss of some operating data.The third part is the anomaly detection of time series (time series abnormal traffic).This is also a very common phenomenon in network traffic, such as abnormal network attacks, abnormal environment monitoring, and financial fraud identification.Part 4 is the classification of time series.For example, electrocardiogram classification, speech classification, and earthquake monitoring in medicine.

Next, we will explore the significant differences between time series and traditional language sequences. Human language sequences are usually composed of sentences, which are discrete representations with high semantic density. In contrast,Time series are mostly composed of natural signals, which may contain continuous numerical points and their main feature is relatively low semantic density.

The contradiction between the difficulty of absorbing electricity during the lunch break and the tight supply of electricity during the evening peak is prominent, and the new energy forecast faces three major challenges
When discussing how to solve this problem, I will focus on our team's research progress in the direction of time series new energy, specifically the prediction of photovoltaic power generation output.
Since photovoltaic power generation has the characteristics of high power generation in the afternoon and almost no power output in the evening, it increases the difficulty of regulating the entire power system. Especially in the afternoon, it becomes more difficult to absorb new energy, and the power supply during the evening peak period is also facing tension. This contradiction is particularly prominent. Therefore, for the formulation of the day-ahead power generation plan, the adjustment of the intraday power balance, and the operation of the power market, the demand for the accuracy of photovoltaic power generation forecasts is unprecedentedly strong.

However, there are currently three major challenges in forecasting new energy.First, numerical weather forecasts currently do not meet the needs of high-precision predictions for photovoltaic power stations; second, centralized photovoltaic power station models are not sufficient to describe power fluctuations and are difficult to adapt to prediction needs under multiple times and weather conditions; third, distributed photovoltaic power stations lack ground irradiance data, and the distribution of temporal and spatial characteristics is insufficient, making it impossible to meet multi-time scale predictions.
Constructing a multi-time scale power prediction model for centralized photovoltaic power plants and distributed photovoltaic power plants
Faced with a series of challenges, we proposed a number of model research plans to build a multi-time scale power prediction model for centralized photovoltaic power stations and distributed photovoltaics.We first collected meteorological data from multiple sources, covering data of different time scales and meteorological types,Including satellite cloud images, numerical weather forecast data, ground measurement data and measurement data of photovoltaic power stations.
Secondly, based on these data, we built an irradiance prediction model on the previous layer.Used to guide ultra-short-term forecasts for centralized and distributed photovoltaic power plants.On this basis, we further constructed forecasting models for ultra-short-term, medium-term, short-term and other time scales. Finally, we built a forecasting platform for all time scales.
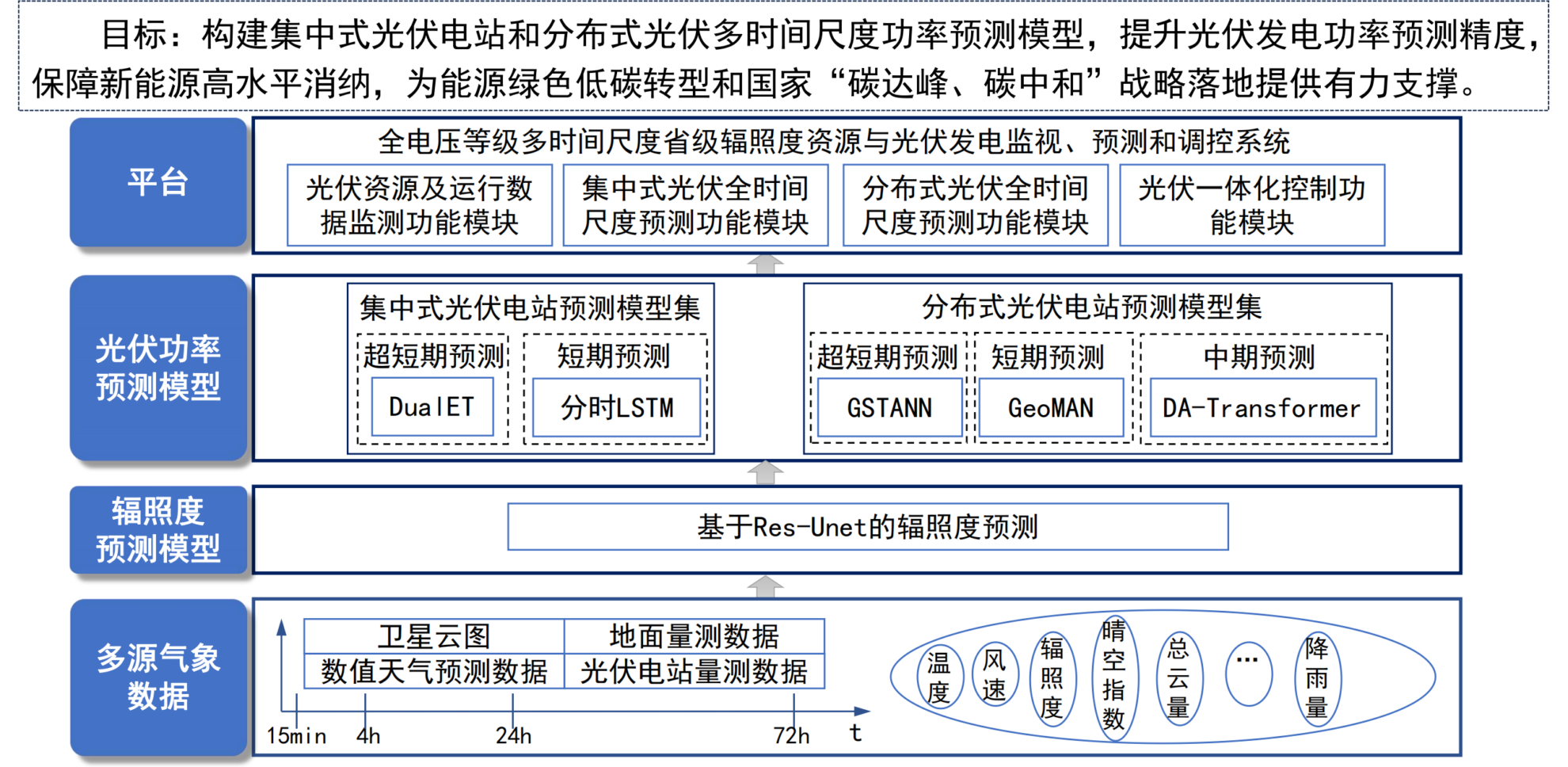
Multi-source meteorological data
First, the ultra-short-term prediction model of surface solar irradiance for photovoltaic power stations is developed.The main problem at present is that numerical weather forecasts are usually updated every 12 hours, and their spatial resolution and accuracy are low, which makes it difficult to meet the spatial and temporal resolution requirements of photovoltaic power station predictions.
To solve this problem,We combined Himawari-8 satellite cloud images with numerical weather forecast data.The cloud images of Sunflower-8 have a spatial resolution of 4km*4km and a temporal resolution of 10 minutes, but there is a 20-minute delay. The temporal resolution of numerical weather forecasts is 15 minutes, the spatial resolution is 9km*9km, and the update frequency is once every 12 hours.
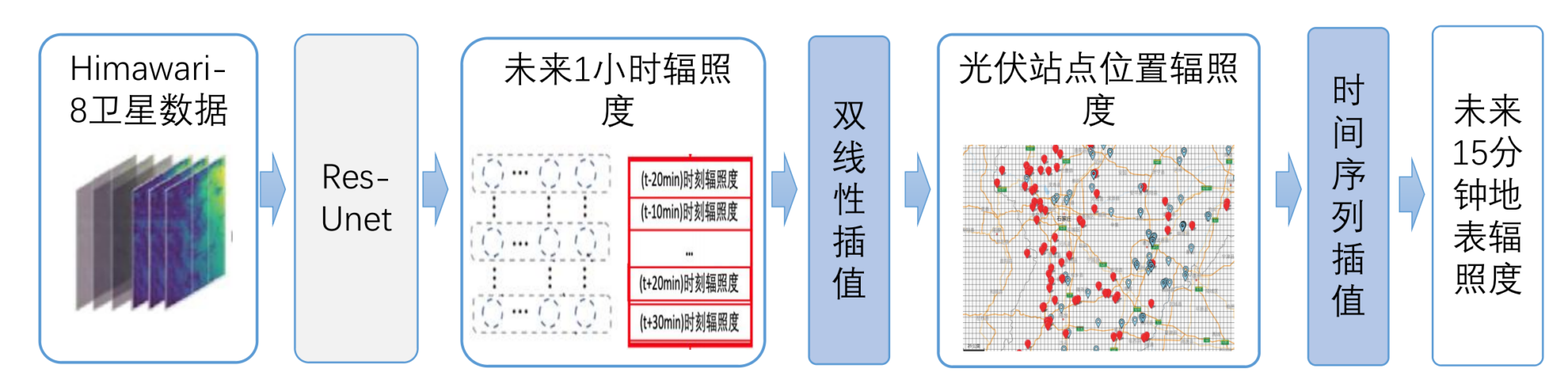
Radiosity prediction model
In the face of these differences, we developed an ultra-short-term prediction model and used a variety of interpolation methods to parallel align multi-source meteorological data to solve the problem of data delay.Through the surface shortwave irradiance prediction method based on Res-UNet and bilinear interpolation, we reduced the MAE and RMSE predicted under various meteorological conditions by an average of 31.31% and 22.18% respectively. The lower right figure shows an actual case of the Donghuang CDI station in Shexian County, Hebei Province. The results show that Res-UNet can more accurately predict the jitter and peak value of irradiance than NWP and UNet.
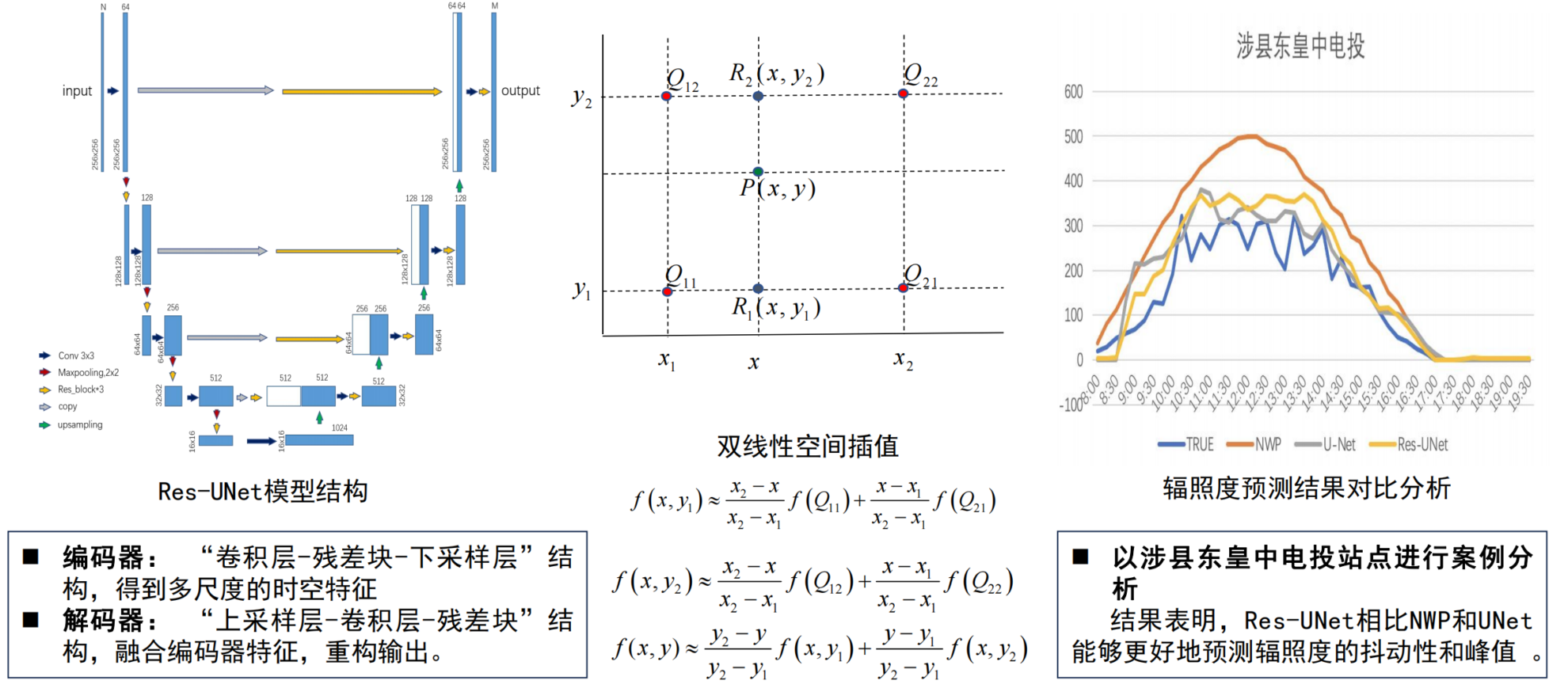
Photovoltaic power prediction model
The problems with the ultra-short-term prediction model for centralized photovoltaic power stations mainly lie in its heavy reliance on numerical weather forecasts and its lack of accuracy.To address this problem, we propose a centralized photovoltaic ultra-short-term power prediction method based on dual-coded converters.Combining ground observation data with cloud change characteristic data in satellite cloud images breaks the limitation of photovoltaic prediction relying solely on numerical weather forecast data.
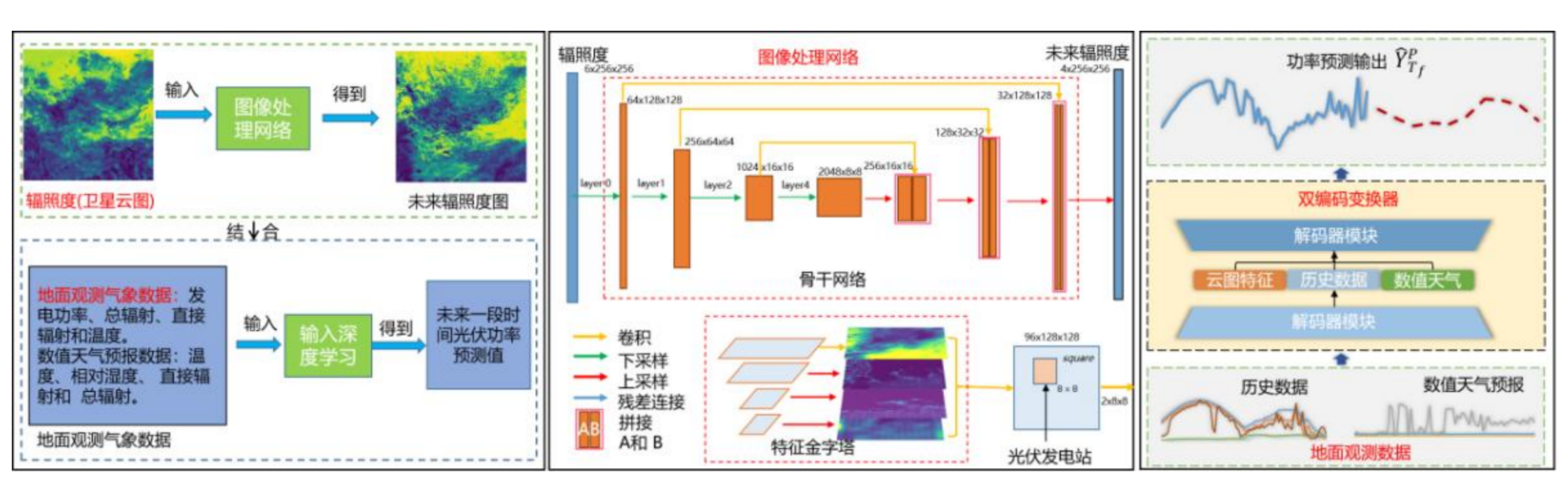
In terms of short-term power forecasting of centralized photovoltaic power stations, the prediction error of a single model is large and is easily affected by sudden weather events.To this end, we proposed a centralized photovoltaic short-term power prediction method based on time-sharing-long short-term memory network.The comprehensive use of historical meteorological data such as irradiance, ambient temperature, humidity and the time correlation characteristics of photovoltaic power generation solves the problem that a single prediction model is difficult to adapt to complex and changeable meteorological conditions, and effectively improves the prediction accuracy under complex meteorological conditions.
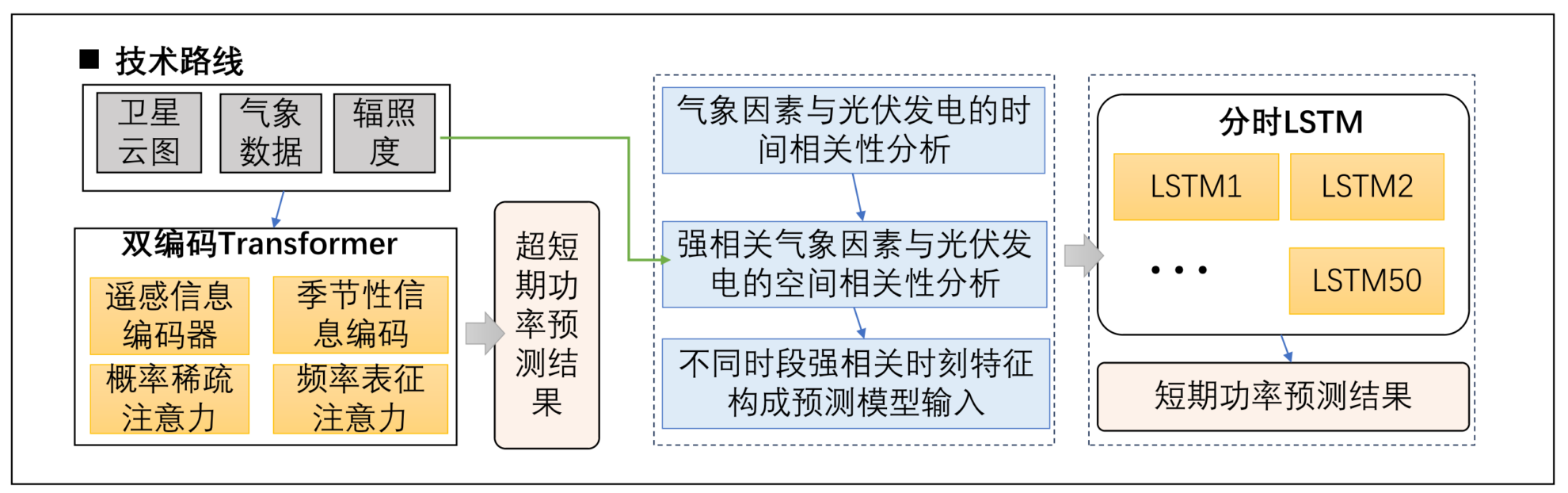
For medium- and long-term forecasts, the main challenge is how to capture the seasonal, cyclical and long-term trend changes in photovoltaic power generation.To solve this problem, we propose a centralized photovoltaic medium-term power forecasting method based on a dual attention encoder, which comprehensively uses periodic, seasonal and trend meteorological characteristic data.It has realized the accurate capture of different seasonal and continuous multi-time features, and has taken the lead in realizing the automatic extraction of time series cycles and trends. The relevant results have been published at the AAAI conference.
Paper address:
https://ojs.aaai.org/index.php/AAAI/article/view/25845

The main problem with the full-time scale prediction model for distributed photovoltaic power stations is that they are small in scale, widely distributed, and lack accurate on-site meteorological observation data. The current prediction model does not fully consider the spatiotemporal fusion of multi-source data, resulting in insufficient accuracy.To this end, we proposed distributed photovoltaic power station power prediction models for the ultra-short term, short term and medium term respectively.
We also proposed a multi-layer graph attention mechanism,Automatically extract the spatiotemporal correlation between massive distributed photovoltaic power plants and the measured meteorological data and satellite cloud images of surrounding centralized photovoltaic power plants;A distributed photovoltaic short-term power prediction model based on dual attention network is proposed.Through the intra-station and inter-station attention mechanism, the numerical weather forecast data of distributed power stations and the ground measurement data of centralized photovoltaic power stations are integrated to achieve the fusion of geographic spatial features in the prediction model;A distributed photovoltaic medium-term power forecasting method based on geo-aware multi-layer attention mechanism is proposed.The strongly correlated centralized photovoltaic power stations are screened through grey correlation analysis, and the inter-station and intra-station multi-level fine-grained attention mechanism is adopted to automatically extract the spatiotemporal correlation between the meteorological characteristics of centralized photovoltaic power stations and the power of distributed photovoltaic power stations.

Comprehensive platform
Finally, we developed a provincial irradiance resource and photovoltaic power generation monitoring, prediction and control system platform with full voltage levels and multiple time scales, including photovoltaic resource and operation data monitoring function module, centralized photovoltaic full time scale prediction function module, distributed photovoltaic full time scale prediction function module and photovoltaic integrated control function module.
* Photovoltaic resource and operation data monitoring function module: realizes the measurement and display of meteorological data, and panoramic monitoring of centralized photovoltaic power stations and massive low-voltage distributed photovoltaics.
* Centralized photovoltaic full-time scale prediction function module: realizes real-time monitoring, abnormal warning, model self-learning, etc. of photovoltaic full-time scale.
* Distributed photovoltaic full-time scale prediction function module: realizes NWP data management, photovoltaic station measured data, basic data management, regional power prediction, photovoltaic station power prediction, system management and other functions.
* Photovoltaic integrated control function module: realizes comprehensive data monitoring and coordinated optimization scheduling, combines centralized and distributed prediction, automatic adjustment and optimization, anomaly detection and alarm, to ensure safe and stable operation of the power grid and high-level absorption of new energy.

Two major advances in time series in the non-new energy field
Next, I will mainly introduce the progress of time series work in non-new energy fields.On the one hand, we proposed a general lossless compression framework based on semantic enhancement and multi-stream pipeline, which mainly includes three research contents: byte stream semantic enhancement, multi-stream pipeline acceleration, and video memory optimization.
In terms of byte stream semantic enhancement, we have developed a series of new methods to obtain complex semantic information, including patch dimension fusion and adaptive sliding window techniques.
In terms of multi-stream pipeline acceleration, we have developed a multi-stream acceleration module for GPU multi-replication engines and a queue model for CPU multi-cores.

In terms of video memory optimization, we first proposed a video memory optimization strategy in multi-stream scenarios. Specifically, we first used a profiler to analyze the order of memory allocation and release to identify memory blocks that can be shared. We focused on those memory blocks that are few but occupy most of the memory to maximize memory reuse.
Based on this, we design a shared pool strategy to manage shared memory blocks between streams. The large continuous memory blocks it uses are released back to the shared pool and marked as reserved blocks; the next blocked stream can access these reserved blocks by adjusting its pointer to the address of these blocks. When S2 requests new memory space, it searches for available reserved blocks in the shared pool. If suitable blocks are found, malloc reuses them in the next stream, saving memory significantly.

Our main achievements are:For deep learning compressors, the average compression rate is more than 3% and the compression speed is enhanced by more than 35% on images, texts, audio, video, and heterogeneous mixed data. When combined with the PAC compressor, it reaches the current SOTA; the semantic enhancement method can be extended to tasks such as time series to further improve the accuracy of time series prediction; multi-stream pipeline acceleration can be extended to fields such as lossy compression to improve the overall compression speed.

On the other hand, we propose a multi-scale model CSIformer for general time series.First, we designed an adaptive patch partitioning network to automatically adapt traditional hyperparameters by learning the center point and left and right boundaries. In addition, we proposed an adaptive stride strategy to use a mask matrix to adjust the stride of different semantic density blocks. Finally, in order to enhance the capture and perception of long sequence information, we also designed a pyramid fusion strategy to improve the performance of the model in long sequences.

In terms of multi-level sequence decomposition models for PV forecasting, we focus on the wavelet decomposition unit (WTDU), seasonal trend decomposition unit (STDU) and SEEDTrans architecture.It is noteworthy that the model improves the prediction accuracy by more than 40% compared with the traditional ARIMA model in 6 power stations in Hebei, China.

About Wanmeng
Wan Meng is a PhD candidate at the University of Science and Technology Beijing and is currently an engineer at the Artificial Intelligence Department of the Computer Network Information Center of the Chinese Academy of Sciences. He received his bachelor's and master's degrees in software engineering from Beijing University of Posts and Telecommunications and the University of Southampton in the UK, respectively.

He is mainly engaged in time series prediction, artificial intelligence platform and other related research, including photovoltaic power generation processing prediction, polymer material calculation and simulation, ecological carbon cycle, etc. He has participated in projects such as "Construction of China Science and Technology Cloud Software Resource Pool" and "Innovative Application of Artificial Intelligence".
Wan Meng's email address:wanmengdamon@cnic.cn