The Matching Accuracy Rate Increased by 187.9%! The CGCL Laboratory of Huazhong University of Science and Technology Uses Self-supervised Learning to Assist Capsule Endoscopy Image Stitching, and the "Sky Eye" Can Also See Gastrointestinal Health
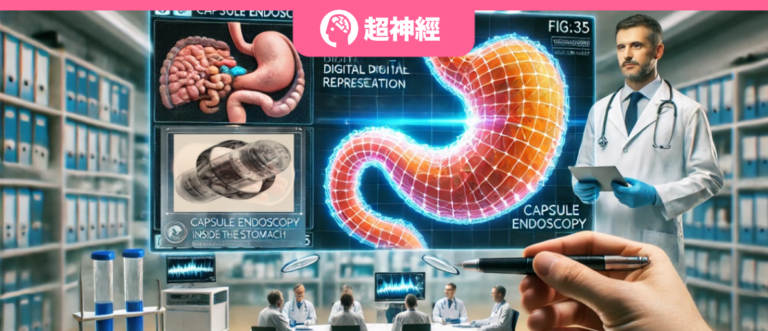
Globally, gastrointestinal diseases are becoming a serious public health challenge. According to statistics from the World Health Organization's International Agency for Research on Cancer, the incidence of gastric diseases in the population is as high as 80%. In China, the number of patients with gastrointestinal diseases has reached 120 million, and there is a clear trend of younger people.It is urgent to pay attention to gastrointestinal health.
In this context, microcapsule endoscopy (MCCE), as an advanced diagnostic tool, has attracted widespread attention due to its non-invasive, painless and cross-infection-free characteristics.Specifically, MCCE has a wireless camera built into the capsule. The patient only needs to swallow the capsule, which will pass through the esophagus, stomach, and then enter the small intestine. During this process, it will take tens of thousands of images and record them in the patient's belt hard drive. Finally, the capsule will be naturally excreted from the body with the patient's feces. Based on the images taken, doctors can quickly identify gastrointestinal diseases or abnormalities, greatly reducing the patient's medical pain.
However, since the movement of capsule endoscopes mainly depends on gastrointestinal motility, the shooting range is limited. MCCE is difficult to effectively capture the specific area that doctors want to focus on (i.e., the area of interest), and can only capture a large number of fragmented images with unstable viewing angles. These images usually have problems such as weak texture, large changes in viewing angle, and deformation when shot at close range.This poses great challenges to image stitching and positioning, and also increases the difficulty of accurately detecting the lesion area.
In response to this, Lu Feng's team from Huazhong University of Science and Technology, in collaboration with Sheng Bin from Shanghai Jiao Tong University, South-Central University for Nationalities, Hong Kong University of Science and Technology (Guangzhou), Hong Kong Polytechnic University, and the University of Sydney, proposed a self-supervised, fragment matching-based capsule endoscopy image stitching method called S2P-Matching.This method simulates the shooting behavior of a capsule endoscope in the gastrointestinal tract, enhances the original data, uses contrastive learning to extract local features of the image, and performs patch-level matching of the image through the Transformer model. Ultimately, the matching can be refined to the pixel level, which can significantly improve the accuracy and success rate of image stitching and enhance the ability to detect and diagnose gastrointestinal diseases at an early stage.
This result, titled "S2P-Matching: Self-supervised Patch-based Matching Using Transformer for Capsule Endoscopic Images Stitching", has been accepted for publication in IEEE Transactions on Biomedical Engineering, a top international journal in the field of biomedical engineering.
Research highlights:
* Compared with other existing methods, S2P-Matching performs better in actual MCCE image matching, especially in solving the parallax and weak texture problems of gastrointestinal images. The matching accuracy and success rate are improved by 187.9% and 55.8% respectively.
* S2P-Matching generates a simulated image dataset by simulating the shooting behavior of a capsule endoscope, which can help the model learn image features from different perspectives
* The researchers proposed the S2P-Matching method, which fills the gap that traditional endoscopes cannot achieve accurate stitching and positioning, helping doctors to observe the gastrointestinal tract more comprehensively and clearly, improving the efficiency of gastrointestinal disease screening, and thus promoting the wider application of non-invasive endoscopic technology in clinical practice

Paper address:
http://dx.doi.org/10.1109/TBME.2024.3462502
Follow the official account and reply "Capsule Endoscopy" to get the complete PDF
The open source project "awesome-ai4s" brings together more than 100 AI4S paper interpretations and provides massive data sets and tools:
https://github.com/hyperai/awesome-ai4s
Dataset: Covering 20,000+ clinical shooting data, accurately labeled by professional doctors
The researchers focused on analyzing a series of continuous images of areas of interest to medical experts in actual clinical scenarios, and selected capsule endoscopy examination records from a domestic hospital from 2016 to 2019.To verify the effectiveness and accuracy of S2P-Matching, they selected images taken continuously by capsule endoscopes during relatively stable periods as training and test data sets. These images were taken every 0.5 seconds, and the spatial resolution of each image was 480×480 pixels.
Specifically, to ensure random grouping and optimize the comparison of stitching effects, the researchers randomly selected samples from the data of 213 patients, and extracted n×10 consecutive frames (n was between 5 and 15) from the image sequence of each patient, obtaining a total of 21,526 images. After strict screening, 20,862 high-quality images were finally retained. On this basis, the researchers selected 528 images to form a test set and invited two collaborating doctors to accurately annotate the matching points on these images.
Model architecture: Patch Transformation, Self-Supervised Transformer takes you on a seamless journey of capsule endoscopy
S2P-Matching introduces an improved self-supervised contrastive learning method, which uses a dual-branch encoder to extract local features and uses these features to train a Transformer model for patch-level image matching, which is finally further refined to pixel-level matching through the Patch-to-Pixel method.The main process includes five parts: Data Augmentation, Deep Feature Descriptor Extraction, Patch Level Matching, Refine to Pixel-level Matching, and Correct Correspondences Filtering, as shown in the following figure:

* Data Augmentation:The role of this part is data enhancement, that is, to simulate the behavior of the capsule endoscope camera in the gastrointestinal tract through affine transformation, generate multi-view reference images, help the model learn image features from different viewpoints, and avoid the complexity of manual labeling.
* Feature extraction (Deep Feature Descriptor Extraction):The role of this part is to extract deep feature descriptors, that is, to extract local features using improved contrastive learning techniques. Specifically, a dual-branch encoder is used to extract features from image patches and background patches respectively, and these features are combined to form deep feature descriptors for matching.
* Patch-level Matching:This part uses a Transformer-based model for image patch-level matching. The model expands its receptive field through a multi-head self-attention mechanism to effectively identify different patch matching pairs in the image. In addition, a matching probability matrix is generated through a dual-softmax operation to determine the confidence of the patch pairing.
* Refine to Pixel-level Matching:This part refines the image from patch-level matching to pixel-level matching. That is, based on patch-level matching, the Patch-to-Pixel method is used to refine the matching to the pixel level to further improve the stitching accuracy.
* Correct Correspndence Filtering:Determine the correct matching pairs, that is, use the MAGSAC algorithm to filter out incorrect matching pairs to ensure accurate pixel-level matching results.
Combining data augmentation, contrastive learning, Transformer networks, and pixel-level matching,S2P-Matching can effectively improve the matching and stitching accuracy of endoscopic images, especially in cases of weak texture, close-up shooting and rotation, which provides potential application value for gastrointestinal screening based on MCCE.In the future, researchers will further expand the application scenarios of this method, such as dealing with complex lighting conditions, bubbles, blur and occlusion.
Experimental conclusion: Matching + splicing, S2P-Matching is a versatile method in capsule endoscopy images
To evaluate the performance of the S2P-Matching method, the researchers compared its image matching effects with other current advanced image matching algorithms (such as CAPS, ASIFT, DeepMatching, R2D2, SuperPoint, etc.). The dataset used for the experiment contains capsule endoscopy images collected from 2016 to 2019, covering a variety of complex scenes, such as weak textures, close-up shots, and large-angle rotations.
As shown in the table below, the research results show that in all experimental types (weak texture, close-up, large-angle rotation), S2P-Matching exhibits the highest NCM (number of correctly matched points) and SR (success rate) scores, with an average NCM of 311 and an average SR of 81.7%.Compared with traditional algorithms, the matching accuracy of S2P-Matching is significantly improved.

The researchers selected three groups of images from different datasets (weak texture, close-up, and large-angle rotation). The matching results of different methods are compared in the following visual graph. Each pair of input images includes two capsule endoscopy images taken at 0.5 second intervals. The three pairs of images in each column are taken at very close positions and have rotation variance. The white lines represent the corresponding pairs, i.e. the matching results. The image matching results obtained by different methods are shown in the following visual graph:

It can be seen that from the first row to the third row, as the texture becomes weaker and the number of repeated areas increases, the number of matching pairs obtained by various methods decreases to varying degrees. For example, CAPS and ASIFT can only extract a small number of matching pairs, and there are incorrect matching pairs, resulting in errors in the final image stitching. DeepMatching can also only extract a limited number of matching pairs. R2d2 and SuperPoint have a large number of matches, but there are many inaccurate matching pairs. SuperGlue, LoFTR, and TransforMatcher have fewer correct matches. Compared with other methods,S2P-Matching achieves the best feature matching performance and can extract a sufficient number of important matching pairs without interference from impurities and obvious transformations, thus ensuring the final image stitching.
In clinical applications, the limited range of images captured by capsule endoscopes each time makes it difficult for doctors to observe the region of interest in a wider field of view, which may affect the accuracy of diagnosis. Usually, a complete region of interest involves multiple continuous images with partial overlap. Therefore, it is very important to achieve continuous stitching of capsule endoscope images.
As shown in the figure below, researchers compared different methods for stitching continuous frames of capsule endoscopy images and found that:S2P-Matching has the most natural stitching effect and the highest stitching accuracy, and can effectively deal with problems such as weak texture and rotation of images. Compared with other algorithms, this method generates the most matching pairs, and the stitching results have no obvious problems such as texture misalignment, overscaling and texture connection.

Furthermore, the researchers conducted ablation experiments to study the impact of different modules on the final results. The results showed that the S2P-Matching framework that combines image derivatives and deep feature descriptors can significantly improve the accuracy of image matching, especially when processing complex capsule endoscopy images. In addition, S2P-Matching performs well when processing images taken at different angles, and can adapt well to image matching tasks with large angle rotations, with better accuracy than other methods.
In summary, S2P-Matching has achieved higher matching accuracy and better stitching effect in complex capsule endoscopy image matching tasks, especially in complex situations such as weak texture, rotation and close-range shooting.
The leader in smart healthcare
With the advancement of medical technology, capsule endoscopes have become a "small lens" for exploring the internal world of the human body. With the support of AI, this non-invasive examination method not only reduces the pain of patients, but also provides doctors with valuable diagnostic basis.
It is worth mentioning that the first author of the paper, Professor Lu Feng of Huazhong University of Science and Technology, continues to pay attention to the application of AI in disease diagnosis and treatment.In addition to the above research, she also collaborated with the University of Sydney team to publish a paper titled "Fine-Grained Lesion Classification Framework for Early Auxiliary Diagnosis" in IEEE/ACM Transactions on Computational Biology and Bioinformatics, proposing a fine-grained lesion classification framework for capsule endoscopy. This framework can accurately identify candidate lesions of different sizes from medical images from capsule endoscopy, assisting doctors in early diagnosis.
Original paper:
https://ieeexplore.ieee.org/abstract/document/10077722
Professor Lu Feng has achieved fruitful results in his research.He has published more than 30 academic papers in top international journals and conferences such as Nat Med, IEEE Network, TBME, TCBB, TIOT, AAAI, and has won many domestic and international patents and technical awards.

Lu Feng's personal homepage:
http://faculty.hust.edu.cn/lufeng2/zh_CN/index.htm
Her research team is affiliated with the CGCL Laboratory of Huazhong University of Science and Technology.The laboratory is an innovation team in key areas of the Ministry of Science and Technology, the leading unit of the innovation team of the Ministry of Education's "Changjiang Scholars and Innovation Team Development Program", and an innovation team of the Hubei Natural Science Foundation. It has undertaken nearly 400 scientific research projects and has rich medical data resources and computing resources. It is one of the very few laboratories in the world that can conduct industrial-level large-scale data analysis and intelligent medical research.
Home page of CGCL Laboratory of Huazhong University of Science and Technology:
https://grid.hust.edu.cn/
Professor Lu Feng's team has not only achieved remarkable results with its own excellent technology and abundant resources, but also actively sought cooperation with top universities at home and abroad. For example, in the research of this article, Lu Feng's team cooperated with Professor Sheng Bin, a senior scholar in the field of AI medicine.Professor Sheng Bin has long been concerned about the application of AI in medicine and has published a series of research results in this field. For example, he built the world's first visual-large language model integrated system DeepDR-LLM for diabetes diagnosis and treatment, providing grassroots doctors with personalized diabetes management advice and auxiliary diagnosis results for diabetic retinopathy.
More details: The world's first! Tsinghua University/Shanghai Jiaotong University and others jointly built a visual-language model for diabetes diagnosis and treatment, published in Nature
In the future, with the joint efforts of these outstanding researchers, we look forward to achieving more accurate and efficient medical diagnosis and effectively improving patients' medical experience.
References:
1.https://gleneagles.hk/sc/medical-treatments/capsule-endoscopy
2.https://m.21jingji.com/article/20240409/herald/244d34d9d0c815096fa8f3a25ca5cced_zaker.html
