The Largest Open Source Robotics Dataset! DeepMind Teamed up With 21 Institutions to Integrate 60 Datasets and Released Open X-Embodiment, Ushering in the Era of Embodied Intelligence
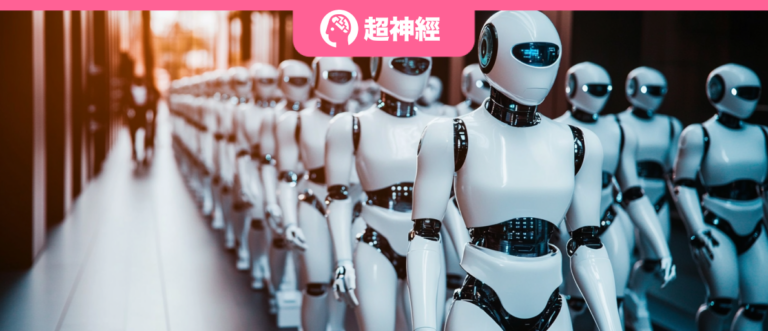
Recently, a video of a robot dog acting as a porter on Mount Tai has gone viral on the Internet.This "robot dog" can not only carry heavy materials easily, but also "walk briskly" on the steep mountain road of Mount Tai, from the foot of the mountain to the top of the mountain in just two hours! Chinese Vice Foreign Minister Hua Chunying also praised it: "The robot dog acts as a porter on the majestic Mount Tai, and technology benefits mankind."

It is understood that this robot dog introduced by the property management company of Taishan Cultural Tourism Group is mainly responsible for clearing garbage and transporting goods. In the past, due to the special terrain of Taishan Scenic Area, garbage cleaning could only rely on manual transportation, and during peak passenger flow periods, it was often impossible to clean and transport garbage. This "robot dog" participating in the test not only has strong terrain adaptability, high stability, and high balance ability, but can also easily cross obstacles, with a load capacity of up to 120 kilograms, which solves the problem of garbage removal and improves work efficiency.
Google RT-2, NVIDIA GR00T, Figure 02 robot, Tesla Optimus, Xiaomi CyberOne... In recent years, with the emergence of more and more robots, embodied intelligence using these robots as carriers has also become a hot topic.These robots, which can perceive, learn and interact with their surroundings and directly participate in human real life, have great potential in the market.
Whether it is inspection, welding, handling, sorting and assembly in the industrial field, housekeeping services and elderly care in the home scene, surgical assistance and daily care in the medical field, as well as planting, fertilizing and harvesting in agriculture, the application scenarios of embodied intelligence are wide and diverse.
However, although current robots perform well in certain specific tasks, they are still lacking in versatility, which means that we need to train a model for each task, each robot, and even each application scenario. Drawing on the successful experience of fields such as natural language processing and computer vision, we may be able to let robots learn from a wide range of diverse data sets, thereby training more general robots. However,Existing robotics datasets often focus on a single environment, a set of objects, or a specific task. Large and diverse datasets for robotic interaction are difficult to obtain.
In response to this, Google DeepMind teamed up with 21 internationally renowned institutions including Stanford University, Shanghai Jiaotong University, NVIDIA, New York University, Columbia University, University of Tokyo, RIKEN, Carnegie Mellon University, ETH Zurich, and Imperial College London to integrate 60 existing robot datasets.Created an open, large-scale, standardized robot learning dataset - Open X-Embodiment.

It is understood that the Open X-Embodiment dataset covers various environments and robot changes and is currently open to the research community. In order to facilitate users to download and use it, the researchers converted datasets from different sources into a unified data format. In the future, they plan to cooperate with the robot learning community to jointly promote the growth of this dataset. HyperAI has launched the "Open X-Embodiment Real Robot Dataset" on its official website, which can be downloaded with one click!
Open X-Embodiment Real Robot Dataset:
https://go.hyper.ai/JAeHn

The largest open source robotics dataset to date
It is reported that Open X-Embodiment is the largest open source real robot dataset to date.It covers 22 different robot types, from single-arm robots to dual-arm robots to quadruped robots, with more than 1 million robot trajectories and 527 skills (160,266 tasks). The researchers demonstrated that models trained on multiple robot type data performed better than models trained on only a single robot type data.

The distribution of the Open X-Embodiment dataset is shown in the figure below.Figure (a) shows the distribution of 22 robot data sets, among which Franka is the most common. Figure (b) shows the distribution of robots in different scenarios, with Franka being the dominant robot, followed by Google Robot. Figure (c) shows the distribution of trajectories for each robot, with xArm and Google Robot contributing the most trajectories. Figures (d, e) show the skills that robots have and the objects they use, including picking, moving, pushing, placing, etc., and a wide variety of objects including household appliances, food, and tableware.

Bringing together top institutional resources to promote widespread application of robotics technology
The Open X-Embodiment dataset is composed of 60 independent datasets.HyperAI has specially selected some data sets for you, which are briefly introduced as follows:
Berkeley Cable Routing Multi-stage Robotic Cable Routing Task Dataset
The Berkeley Cable Routing dataset was released by the University of California, Berkeley and the Intrinsic Innovation LLC research team. It is 27.92 GB in total and is used to study multi-stage robot manipulation tasks.Especially used for cable routing tasks.This task represents a complex multi-stage robotic manipulation scenario where the robot must thread a cable through a series of clamps, handle deformable objects, close a visual perception loop, and process extended behaviors consisting of multiple steps.
Direct use:https://go.hyper.ai/igi9x

CLVR Jaco Play Dataset Remote Control Robot Clip Dataset
CLVR Jaco Play Dataset is a dataset focusing on the field of remote control robots.The 14.87 GB dataset, released by a research team from the University of Southern California and KAIST, provides 1,085 clips of the remote-controlled robot Jaco 2 with corresponding language annotations. This dataset is a valuable resource for scientists and developers studying robot remote control, natural language processing, and human-computer interaction.
Direct use:https://go.hyper.ai/WPxG8
RT-1 Robot Action Real World Robot Dataset
Google researchers proposed the multi-task model Robotics Transformer (RT-1), which achieved significant improvements in zero-shot generalization on new tasks, environments, and objects, demonstrating excellent scalability and pre-trained model characteristics.
The RT-1 model was trained on a large-scale real-world robotics dataset (RT-1 Robot Action Dataset), using 13 EDR robotic arms, each equipped with a 7-DOF arm, a two-finger gripper, and a mobile base.130,000 clips were collected in 17 months.A total of 111.06 GB, each clip is annotated with a text description of the robot's execution instructions. The high-level skills covered in the dataset include picking up and placing objects, opening and closing drawers, taking out and putting objects in drawers, placing slender objects vertically, pushing down objects, pulling napkins, and opening cans.Over 700 tasks using many different objects are covered.
Direct use:https://go.hyper.ai/V9gL0

Language-Table Robot language label trajectory dataset
The Google Robotics team at Google has proposed a framework for building robots that can interact in real time in the real world through natural language instructions. By training on a dataset containing hundreds of thousands of trajectories with language annotations, the researchers found that the resulting strategy can execute 10 times more instructions than before, which describe end-to-end visual, auditory and motor skills in the real world. The researchers have open-sourced the Language-Table dataset used in the study.The dataset contains nearly 600,000 trajectories with language labels.Used to promote the development of more advanced, capable robots that can interact with natural language.
Direct use:https://go.hyper.ai/9zvRk

BridgeData V2 Large-Scale Robot Learning Dataset
UC Berkeley, Stanford University, Google DeepMind, and CMU jointly released the BridgeData V2 dataset, which is dedicated to promoting scalable robotics research.Contains 60,096 robot trajectories collected in 24 different environments.Among them, 24 environments are divided into 4 categories. Most of the data comes from different toy kitchens, including sinks, stoves, and microwaves, while the rest of the environments include various desktops, toy sinks, toy laundry, etc. The tasks included in the dataset are picking and placing, pushing and sweeping, opening and closing doors and drawers, as well as more complex tasks such as stacking blocks, folding clothes, and sweeping granular media. Some data fragments contain a combination of these skills.
To improve the robot's generalization ability, the researchers collected a large amount of task data in a variety of environments with different objects, camera positions, and workspace positioning.Each trajectory is accompanied by natural language instructions corresponding to the robot's task.The skills learned from this data can be applied to new objects and environments, and even used across institutions, making this dataset a great resource for researchers.
Direct use:https://go.hyper.ai/mGXA1

BC-Z Robot Learning Dataset
The BC-Z dataset was jointly released by Google, Everyday Robots, the University of California, Berkeley, and Stanford University. This large-scale robot learning dataset aims to promote the development of the field of robot imitation learning, especially to support zero-sample task generalization, that is, to allow robots to perform new operation tasks through imitation learning without prior experience.
The dataset contains more than 25,877 different operation task scenarios, covering 100 diverse tasks.These tasks were collected through expert teleoperation and shared autonomy processes involving 12 robots and 7 operators, with a total of 125 hours of robot operation time. The dataset can be used to train a 7-DOF multi-task policy that can be adjusted to perform specific tasks based on a verbal description of the task or a video of a human operating it.
Direct use:https://go.hyper.ai/MdnFu

The above are the datasets recommended by HyperAI in this issue. If you see high-quality dataset resources, you are welcome to leave a message or submit an article to tell us!
More high-quality datasets to download:https://go.hyper.ai/P5Mtc