The Benchmark Test in the Medical Field Surpasses Llama 3 and Is Close to GPT-4. The Shanghai Jiaotong University Team Released a Multilingual Medical Model Covering 6 Languages
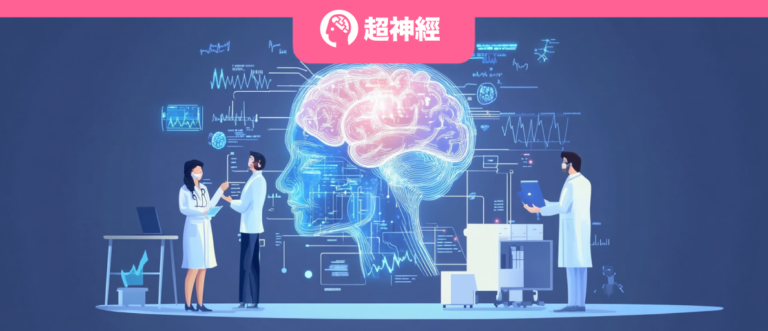
With the popularization of medical information technology, medical data has achieved different degrees of improvement in terms of scale and quality. Since entering the era of big models, various big models for different scenarios such as precision medicine, diagnostic assistance, and doctor-patient interaction have emerged in an endless stream.
But it is worth noting that, just as the universal model faces the problem of multilingual competence lag,Most large medical models rely on English-based models and are also limited by the scarcity and dispersion of multilingual medical professional data, which results in poor performance of the models when handling non-English tasks.Even medical-related open source text data is mainly in high-resource languages, and the number of supported languages is very limited.
From the perspective of model training, multilingual medical models can more comprehensively utilize global data resources, and even expand to multimodal training data, thereby improving the quality of the model's representation of other modal information. From the application level, multilingual medical models can help alleviate language communication barriers between doctors and patients, and improve the accuracy of diagnosis and treatment in multiple scenarios such as doctor-patient interaction and remote diagnosis.
Although the current closed-source models have shown strong multilingual performance, there is still a lack of multilingual medical models in the open source field.The team of Professor Wang Yanfeng and Professor Xie Weidi from Shanghai Jiao Tong University created a multilingual medical corpus MMedC containing 25.5 billion tokens, developed a multilingual medical question-and-answer evaluation standard MMedBench covering 6 languages, and built an 8B base model MMed-Llama 3, which surpassed existing open source models in multiple benchmark tests and is more suitable for medical application scenarios.
The relevant research results were published in Nature Communications under the title "Towards building multilingual language model for medicine".
It is worth mentioning thatThe tutorial section of HyperAI's official website is now online "One-click deployment of MMed-Llama-3-8B".Interested readers can visit the following address to quickly get started ↓. We have also prepared a detailed step-by-step tutorial for you at the end of the article!
One-click deployment address:
🎁 Insert a benefit
Coinciding with "1024 Programmers' Day", HyperAI has prepared computing power benefits for everyone!New users who register OpenBayes.com with the invitation code "1024" will receive 20 hours of free use of a single A6000 card.Valued at 80 yuan, the resource is valid for 1 month. Only available today, resources are limited, first come first served!
Research highlights:
* MMedC is the first corpus built specifically for the multilingual medical field and is also the most extensive multilingual medical corpus to date.
* Autoregressive training on MMedC helps improve model performance. Under full fine-tuning evaluation, the performance of MMed-Llama 3 is 67.75, while Llama 3 is 62.79
* MMed-Llama 3 achieves state-of-the-art performance on English benchmarks, significantly outperforming GPT-3.5

Paper address:
https://www.nature.com/articles/s41467-024-52417-z
Project address:
https://github.com/MAGIC-AI4Med/MMedLM
Follow the official account and reply "Multilingual Medical Big Model" to download the original paper
Multilingual Medical Corpus MMedC: 25.5 billion tokens, covering 6 major languages
The multilingual medical corpus MMedC (Multilingual Medical Corpus) created by researchers,Covering 6 languages: English, Chinese, Japanese, French, Russian and Spanish.Among them, English accounts for the largest proportion, which is 42%, Chinese accounts for about 19%, and Russian accounts for the smallest proportion, which is only 7%.

Specifically, the researchers collected 25.5 billion medical-related tokens from four different sources.
first,The researchers designed an automatic pipeline to filter medically relevant content from an extensive multilingual corpus;Secondly,The team collected a large number of medical textbooks in different languages and converted them into text through methods such as optical character recognition (OCR) and heuristic data filtering;third,To ensure the breadth of medical knowledge, researchers collected texts from open-source medical websites in multiple countries to enrich the corpus with authoritative and comprehensive medical information;at last,The researchers integrated existing small medical corpora to further enhance the breadth and depth of MMedC.
The researchers said,MMedC is the first pre-trained corpus built specifically for the multilingual medical domain and is also the most extensive multilingual medical corpus to date.
MMedC one-click download address:
https://go.hyper.ai/EArvA
MMedBench: a multilingual medical question answering benchmark containing more than 50,000 pairs of medical multiple-choice questions and answers
In order to better evaluate the performance of multilingual medical models,The researchers further proposed the multilingual medical question and answering benchmark MMedBench (multilingual medical Question and Answering Benchmark).We summarized the existing medical multiple-choice questions in the six languages covered by MMedC, and used GPT-4 to add attribution analysis to the QA data.
Finally, MMedBench contains 53,566 QA pairs, spanning 21 medical fields.For example, internal medicine, biochemistry, pharmacology, and psychiatry. The researchers divided it into 45,048 pairs of training samples and 8,518 pairs of test samples. At the same time, in order to further test the reasoning ability of the model, the researchers selected a subset of 1,136 pairs of QA, each with a manually verified reasoning statement, as a more professional reasoning evaluation benchmark.
MMedBench one-click download address:
https://go.hyper.ai/D7YAo
It is worth noting thatThe reasoning part of the answer consists of 200 tokens on average.This larger number of tokens helps train the language model by exposing it to longer reasoning processes, while also enabling it to evaluate the model’s ability to generate and understand lengthy and complex reasoning.

Multilingual Medical Model MMed-Llama 3: Small but Beautiful, Surpassing Llama 3 and Approaching GPT-4
Based on MMedC, the researchers further trained multilingual models anchoring medical domain knowledge, namely MMedLM (based on InternLM), MMedLM 2 (based on InternLM 2), and MMed-Llama 3 (based on Llama 3). Subsequently, the researchers evaluated the model performance on the MMedBench benchmark.
First, in the multilingual multiple-choice question and answer task,Large models for the medical field often show high accuracy in English, but their performance in other languages is reduced. This phenomenon is improved after autoregressive training on MMedC. For example,Under full fine-tuning evaluation, MMed-Llama 3 achieves a performance of 67.75%, while Llama 3 achieves 62.79.

Similar observations apply to the PEFT (Parameter Efficient Fine Tuning) setting, where LLMs perform better in the later stages.Training on MMedC leads to significant gains.Therefore, MMed-Llama 3 is a highly competitive open source model.Its 8B parameters are close to GPT-4’s 74.27 accuracy.

In addition, the study also formed a five-person review group to further manually evaluate the explanations of the answers generated by the model. The review group members were from Shanghai Jiao Tong University and Peking Union Medical College.
It is worth noting thatMMed-Llama 3 achieved the highest score in both human evaluation and GPT-4 evaluation.Especially in the GPT-4 rating, its performance is significantly better than other models, 0.89 points higher than the second-ranked model InternLM 2.

Orange is the manual evaluation score, pink is the GPT-4 score
To fairly compare with existing large language models on English benchmarks, the researchers also fine-tuned MMed-Llama 3 on English instructions and evaluated it on four commonly used medical multiple-choice question answering benchmarks, namely MedQA, MedMCQA, PubMedQA, and MMLU-Medical.
The results show thatMMed-Llama 3 achieves state-of-the-art performance on English benchmarks.The performance gains of 4.5%, 4.3% and 2.2% were obtained on MedQA, MedMCQA and PubMedQA respectively.On MMLU, it even far exceeds GPT-3.5,The specific data is shown in the figure below.

One-click deployment of MMed-Llama 3: Breaking through language barriers and accurately answering common sense medical questions
Today, big models have been maturely applied in multiple sub-scenarios such as medical image analysis, personalized treatment, and patient services. Focusing on the patient's usage scenarios, facing practical problems such as difficulty in registration and long diagnosis cycle, coupled with the continuous improvement of the accuracy of medical models, more and more patients will seek help from "big model doctors" when they feel slight discomfort. They only need to clearly and clearly input the symptoms, and the model will be able to provide corresponding medical guidance. The MMed-Llama 3 proposed by Professor Wang Yanfeng and Professor Xie Weidi's team further enriches the model's medical knowledge through a massive and high-quality medical corpus, while also breaking through language barriers and supporting multi-language question and answer.
The tutorial section of HyperAI Super Neural is now online "One-click deployment of MMed-Llama 3". The following is a detailed step-by-step tutorial to teach you how to create your own "AI family doctor".
One-click deployment of MMed-Llama-3-8B:
https://hyper.ai/tutorials/35167
Demo Run
1. Log in to hyper.ai, on the Tutorial page, select One-click deployment of MMed-Llama-3-8B, and click Run this tutorial online.


2. After the page jumps, click "Clone" in the upper right corner to clone the tutorial into your own container.

3. Click "Next: Select Hashrate" in the lower right corner.

4. After the page jumps, select "NVIDIA GeForce RTX 4090" and "PyTorch" image, and click "Next: Review". New users can register using the invitation link below to get 4 hours of RTX 4090 + 5 hours of CPU free time!
HyperAI exclusive invitation link (copy and open in browser):
https://openbayes.com/console/signup?r=Ada0322_QZy7

5. After confirmation, click "Continue" and wait for resources to be allocated. The first clone will take about 3 minutes. When the status changes to "Running", click the jump arrow next to "API Address" to jump to the Demo page. Please note that users must complete real-name authentication before using the API address access function.
Because the model is too large, after the container shows that it is running, you need to wait for about 1 minute before opening the API address, otherwise BadGateway will be displayed.



Effect display
After opening the Demo interface, we can directly describe the symptoms and click Submit. As shown in the figure below, when asking whether the symptoms of "sore throat, sneezing" are a cold, the model will first introduce the common symptoms of a cold and provide a diagnosis based on the self-described symptoms.It is worth noting that the model will also remind users that "the answers cannot replace the information or treatment of professional doctors."

However, it should be noted that unlike commercial models that have undergone strict instruction fine-tuning, preference alignment, and safety control, MMed-Llama 3 is more of a base model, more suitable for task-specific fine-tuning in combination with downstream task data rather than direct zero-sample consultation. When using it, please be sure to pay attention to the model's usage boundaries to avoid related direct clinical use.