AI Helps RNA Virus Research Achieve Historic Breakthroughs; Sun Yat-sen University and Others Use Deep Learning Models to Discover More Than 160,000 New Viruses
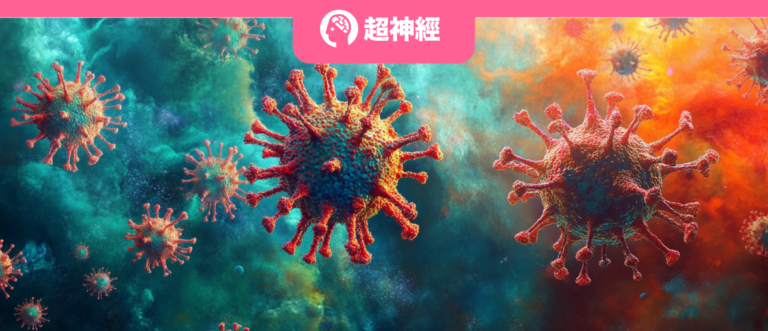
In early 2020, the shadow of the coronavirus quickly enveloped the world. In this race against time, we have witnessed countless brave individuals and teams stepping forward, the social system has experienced severe tests time and time again, and it has also sounded the alarm for the global public health field.
The reason why coronavirus is so feared is largely because it is an RNA virus. This type of virus lacks error correction mechanisms during replication and is prone to mutation. This mutation ability not only allows RNA viruses to spread across species and expand their host range, but may also cause changes in pathogenicity. Once a virus that is originally harmless to humans mutates, it may become pathogenic and cause disease. Since humans generally lack immunity to such mutant viruses, once the virus mutates, it may quickly cause a large-scale epidemic.
Although viruses are closely related to human health, there are only about 5,000 known confirmed virus species, which is just the tip of the iceberg. Traditional RNA virus identification methods rely heavily on sequence homology comparison, that is, identification by comparing the sequence similarity of unknown viruses with known viruses. However,Since RNA viruses are numerous and highly differentiated, traditional methods are difficult to capture "dark matter viruses" that lack homology or have very low homology.This limits the efficiency of new virus discovery.
In the past 10 years, artificial intelligence-related methods, especially deep learning algorithms, have had a significant impact on various research fields in the field of life sciences. The combination of AI and virology research is providing new methods for humans to overcome the difficulty of RNA virus identification.
recently,Professor Shi Mang from the School of Medicine of Sun Yat-sen University, in collaboration with Zhejiang University, Fudan University, China Agricultural University, City University of Hong Kong, Guangzhou University, University of Sydney, Alibaba Cloud Feitian Laboratory, etc., proposed a new deep learning model LucaProt.The model uses cloud computing and AI technology to discover 180 supergroups and more than 160,000 new RNA viruses, which is nearly 30 times the number of known viruses, greatly improving the industry's understanding of RNA virus diversity and viral evolution history. The study also discovered the longest RNA virus genome to date, with a length of 47,250 nucleotides, marking a major breakthrough in the field of RNA virus identification.
The study was published in the international academic journal Cell under the title "Using artificial intelligence to document the hidden RNA virosphere".
Research highlights:
* AI-driven metagenomic mining technology has achieved unprecedented expansion of global RNA virus diversity
* Through precise identification, the existence of 161,979 potential RNA virus species and 180 viral supergroups were revealed
* The study found the longest RNA virus genome to date, which may have modular structural characteristics

Paper address:
https://doi.org/10.1016/j.cell.2024.09.027
Follow the official account and reply "RNA virus identification" to get the full PDF
The open source project "awesome-ai4s" brings together more than 100 AI4S paper interpretations and provides massive data sets and tools:
https://github.com/hyperai/awesome-ai4s
Dataset: Covering various ecosystems around the world, RNA viruses are diverse
This study first conducted a systematic search of databases such as NCBI SRA and CNGBdb, aiming to conduct in-depth research on the diversity of RNA viruses in various ecosystems around the world.
As shown in Figure A below, the research team screened a total of 10,487 data sets from global biological environment samples.The total sequencing data involved reached 51 TB, generating more than 1.3 billion fragments and 872 million predicted proteins.Using these large datasets, the researchers revealed and validated potential viral RdRPs and cross-validated them using 2 different strategies.

By combining the results of the two search strategies,The study found 513,134 viral genomes representing 161,979 potential viral species and 180 RNA virus supergroups.This discovery significantly expands the study's understanding of the RNA virus supergroup, increasing it by about 9 times and the number of viral species by about 30 times.
As shown in Figure C below, this study compared the RdRP protein sequences in other studies.A total of 70,458 newly identified potentially unique viral species were revealed.

The study also revealed 60 previously unrecognized and underexplored supergroups,These supergroups have received only limited attention so far. Of particular note, as shown in Figure D below, the study found that 23 of these supergroups could not be identified by traditional sequence homology methods, which are called the "dark matter" of the virosphere.

LucaProt: A data-driven deep learning model that opens up a new paradigm for virology research
The study developed a data-driven deep learning model, LucaProt. As shown in Figure E below, LucaProt consists of five core modules: Input, Tokenizer, Encoder, Pooling, and Output:
* Input:Mainly responsible for receiving amino acid sequences;
* Tokenizer:Mainly responsible for converting the original sequence into a format that the model can understand. This module includes building a corpus consisting of viral RdRP sequences and non-viral RdRP sequences, and using the BPE algorithm to create a vocabulary to decompose protein sequences into single amino acids to extract structural information;
* Encoder:It is mainly responsible for converting data into two representation forms, one is the sequence representation matrix generated by Transformer-Encoder, and the other is the structure representation matrix generated by the structure prediction model ESMFold. This dual-track representation method not only solves the problem of scarce 3D structure data, but also improves computational efficiency;
* Pooling:It is mainly responsible for converting the sequence matrix and structure matrix into 2 vectors through the value-level attention pooling method (VLAP), reducing the dimension and selecting features for effective classification.
* Output:It is mainly responsible for converting these vectors into a probability value, indicating the possibility that the sample is a viral RdRP. Through the sigmoid function, the sequence is classified as viral RdRP or non-viral RdRP.

final,The study carefully prepared a dataset containing 235,413 samples.Designed to improve the accuracy and generalization of the model, this dataset consists of 5,979 well-studied viral RdRPs (positive samples) and 229,434 non-viral RdRPs (negative samples). Based on the Transformer framework and large model characterization technology, combined with protein sequence and intrinsic structural features, it outperforms traditional methods in terms of accuracy, efficiency, and detected virus diversity.
More importantly, LucaProt integrates not only sequence data but also structural information, which is crucial for accurate prediction of protein function.
Identification of a genome structure beyond previous knowledge, the longest RNA virus genome ever discovered
In order to comprehensively evaluate the performance of LucaProt, the study conducted in-depth analysis from multiple angles to ensure comprehensive verification of its accuracy and efficiency:
* LucaProt performance evaluation
* Verify and confirm whether the newly discovered supergroup of viruses is an RNA virus
* Analysis of modularity and flexibility of RNA virus genome structure
* Analysis of RNA virus phylogenetic diversity
* Analysis of the ecological structure of global RNA viruses
Five methods were jointly evaluated for performance, and LucaProt performed the most comprehensively
To evaluate the performance of LucaProt, the study benchmarked it against four other virus discovery tools. The results show that, as shown in Figure A,LucaProt exhibits the highest recall while maintaining a relatively low false positive rate.

In terms of computational efficiency, as shown in Figure E, LucaProt takes an average of 6 datasets to process datasets of different lengths.Demonstrated more reasonable efficiency.

Finally, the advanced Transformer architecture integrated in LucaProt allows parallel processing of longer amino acid sequences, as shown in Figures FH.This architecture is more effective at capturing relationships between distant parts of the sequence space than the CNN/RNN encoders commonly used in other bioinformatics tools.

Validation and structural characterization of a newly discovered RNA virus supergroup, most of which show sequence similarity to existing RdRPs
The research team extracted and sequenced DNA and RNA from 50 environmental samples to verify the presence of 115 viral supergroups identified in these samples. As shown in Figure B, only RNA sequencing reads were successfully mapped to sequences associated with viral RdRP, while RNA and DNA sequencing reads were mapped to sequences associated with DNA viruses, retroviruses (RT), and cellular organisms, respectively.
Furthermore, as shown in Figure C, by applying the more sensitive RT-PCR method, the research team further confirmed 17 of the 115 viral supergroups. In these supergroups, DNA extraction failed to detect sequences encoding viral RdRP.This further confirms that these viral supergroups are indeed RNA organisms.

Longest RNA virus genome ever discovered
In an in-depth analysis of the composition and structure of putative RNA virus genomes, the study found that although the length of most genomes was concentrated at approximately 2,131 nucleotides, the length of the genomes or genome fragments encoding RdRP varied significantly among different supergroups. In particular, the study identified extremely long RNA virus genomes from soil samples, as shown in Figure C, one of which was 47.3 kb long.It is one of the longest RNA viruses known.In this ultra-long genome, the study discovered an additional ORF located between the 50th end and the RdRP coding region, but its function needs further study.

The expansion rate of RNA virus species is alarming, and more highly differentiated RNA viruses may exist in environmental samples
The study also found that, as shown in the figure below, the number of RNA virus species increased 55.9 times compared to the virus species defined by the International Committee on Taxonomy of Viruses (ICTV) and increased 1.4 times compared to all previously described RdRP sequences. This expansion is particularly evident in the increased diversity of known virus groups.

Notably, some groups previously represented by only a limited number of genomes, such as AstroPoty, Hypo, Yan, and several newly discovered supergroups, exhibited high levels of phylogenetic diversity. For example, SG023 contained 1,232 viruses, SG025 contained 466 viruses, and SG027 contained 475 viruses.This suggests that there may be more highly differentiated RNA viruses in environmental samples.Waiting for us to discover.
RNA viruses still have diversity in extreme environments
The study showed that RNA viruses are found in 1,612 locations and 32 ecosystems around the world.As shown in Figure A, even in ecological samples that have been studied many times, LucaProt still found a new virus group of 5-33.3%.This indicates that the diversity of RNA viruses has not been fully explored, especially in soil and aquatic environments.
The study also compared the alpha diversity and abundance of RNA viruses in different ecosystems. As shown in Figures CD, alpha diversity was highest in leaf litter, wetlands, freshwater, and wastewater environments, while abundance was highest in Antarctic sediments, marine sediments, and freshwater ecosystems. Diversity and abundance were lowest in rock salt and underground environments, consistent with low numbers of host cells. Extreme ecological subtypes such as hot springs and hydrothermal vents had low diversity but moderate abundance of RNA viruses.

From academia to industry, AI's revolutionary progress and future prospects in RNA virus research
In fact, the application of AI in the field of RNA virus research has become a powerful trend in scientific exploration. A research team led by Professor Shi Mang of Sun Yat-sen University has made breakthrough progress using AI technology and discovered more than 160,000 new RNA viruses. This achievement marks an important milestone in the field.
But as early as 2022, an international research team, in collaboration with scientists from the United States, France, Switzerland and other countries,AI machine learning technology has been used to identify 5,500 new RNA viruses from seawater samples around the world.This study not only broadened the scope of ecological research, but also deepened people's understanding of the evolution of RNA viruses and provided new clues for exploring the evolution of early life on Earth.
The research results have been published in the journal Science under the title "Cryptic and abundant marine viruses at the evolutionary origins of Earth's RNA virome".
* Paper link:
https://doi.org/10.1126/science.abm5847
Of course, the application of AI in RNA virus research is not limited to the exploration of unknown areas, but is also essential for in-depth research in known areas. For example, as an RNA virus, COVID-19 has nearly 16 million genome sequences in the globally shared GISAID database. These data provide rich information for research, but also require a lot of computing and human resources to analyze the evolution and history of COVID-19.
To address this challenge, in early 2024, scientists at the University of Manchester and the University of Oxford developed an AI framework that is capable of identifying and tracking new and relevant COVID-19 variants, which may help tackle other infections in the future.The framework combines dimensionality reduction techniques with a new interpretable clustering algorithm, CLASSIX, developed by mathematicians at the University of Manchester, to quickly identify potentially risky viral genomes.The study, published in the Proceedings of the National Academy of Sciences, provides a new approach to tracking viral evolution and may have an impact on traditional methods of tracking viral evolution.
In the industry, the exploration of RNA virus research is also active. Due to the high mutation rate of RNA viruses during replication, the development of vaccines for RNA viruses has always been a difficult problem. In the first half of 2023, the application of AI-assisted drug development has increased day by day.Scientists at Baidu's California branch used AI to deeply optimize the mRNA vaccine, improving not only the sequence but also the structure, thereby increasing the stability of the molecule.This allows it to remain active in the human body for a longer period of time. If this technology is proven to be safe, it will become a powerful tool for the development of a new generation of RNA vaccines and may also provide new ideas for the development of RNA drugs.
In the second half of 2023, Deep Genomics released "An RNA foundation model enables discovery of disease mechanisms and candidate therapeutics", introducing its unique artificial intelligence foundation model BigRNA. BigRNA is the first Transformer neural network for RNA biology and therapeutics, with nearly 2 billion tunable parameters and trained on thousands of datasets containing 1 trillion genomic signals.It represents a new generation of deep learning AI that can be applied to a variety of different RNA therapeutic discovery tasks.
Looking ahead, AI has a promising future in RNA virus research. With the increase in computing power and the improvement of algorithms, AI may be able to process larger data sets and identify more unknown virus populations, as well as their hosts and transmission pathways. This will not only deepen people's understanding of the role of RNA viruses in the ecosystem, but also provide strong support for the prevention and control of future epidemics.
In addition, the application of AI in vaccine design and drug development indicates that people may soon usher in more personalized and precise medical solutions, bringing new hope for global public health security.