Li Benxia From the National Marine Environmental Forecast Center: The Country's First Intelligent Wave Forecasting System Has Been Put Into Commercial Operation, and AI Has Helped Achieve Multiple Breakthroughs in Wave Forecasting
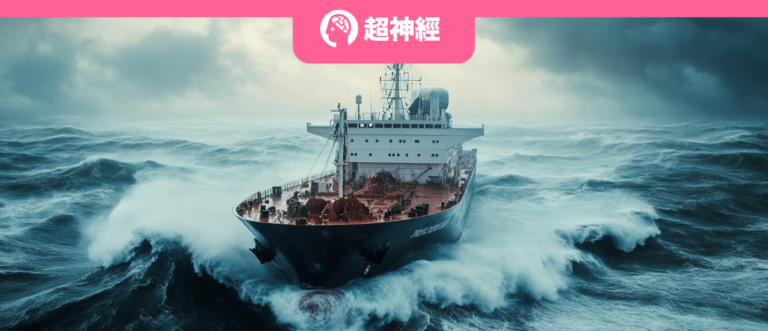
Recently, at the 20th CCF HPC China 2024 conference, the 6th Ocean Numerical Forecasting and High Performance Computing Forum was successfully held.Li Benxia, Director of the Wave Forecast Office of the National Marine Environmental Forecast Center, gave a speech on the theme of "Application of Artificial Intelligence in Wave Forecasting".HyperAI has compiled the core content of the speech without violating the original intention. The following is the transcript of the speech.
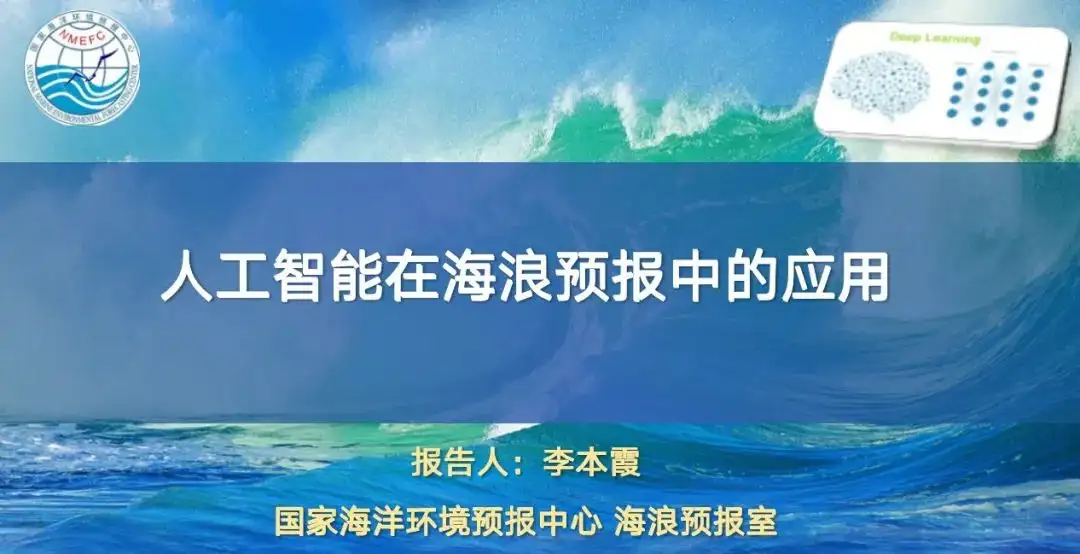
AI helps intelligent wave forecasting become one of the industry development trends
my country is one of the countries with the most serious ocean wave disasters in the world. Ocean wave forecast plays an indispensable role in maintaining the safety of maritime activities, promoting economic development and protecting the marine environment.For example, in terms of maritime safety, wave forecasts can help fishermen avoid severe sea conditions and ensure the safety of marine operations; for coastal tourism, wave forecasts can warn tourists in advance and avoid accidents in dangerous waters; in the field of marine energy development, it provides an important reference for the design and maintenance of offshore wind power and tidal energy facilities.
China's wave forecasting began in 1965, initially relying mainly on empirical statistical forecasting. Later, with the introduction of overseas numerical forecasting models and the development of our own models, we gradually established a numerical forecasting system covering China's coastal waters, the Western Pacific, and bathing areas. Furthermore, the human-computer interaction platform and the ocean intelligent grid forecasting technology are becoming increasingly mature.
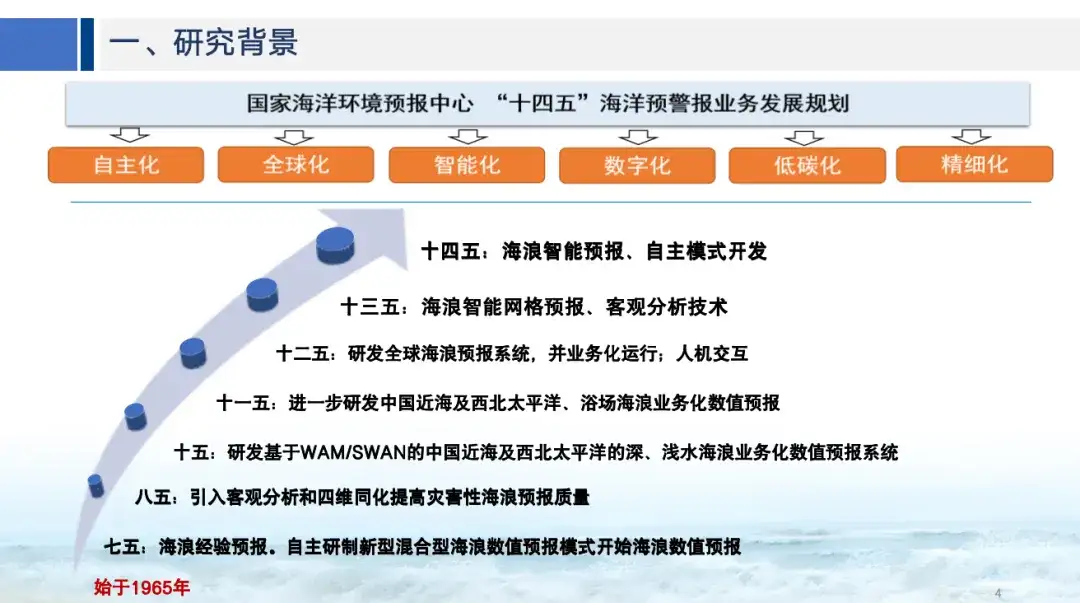
From the perspective of the development of forecasting technology, the current numerical forecast model of ocean waves is still the mainstream. However, when facing typhoon disasters in coastal areas, due to the uncertainty of the typhoon path, the typhoon path and intensity forecast need to be updated quickly, which requires the ocean wave forecast to respond quickly to these changes. However, the ocean wave numerical forecast model needs to go through processes such as manual experience correction, human-computer platform interaction, and product release, and cannot respond quickly to demand. In addition, its high computing cost is also a major constraint.
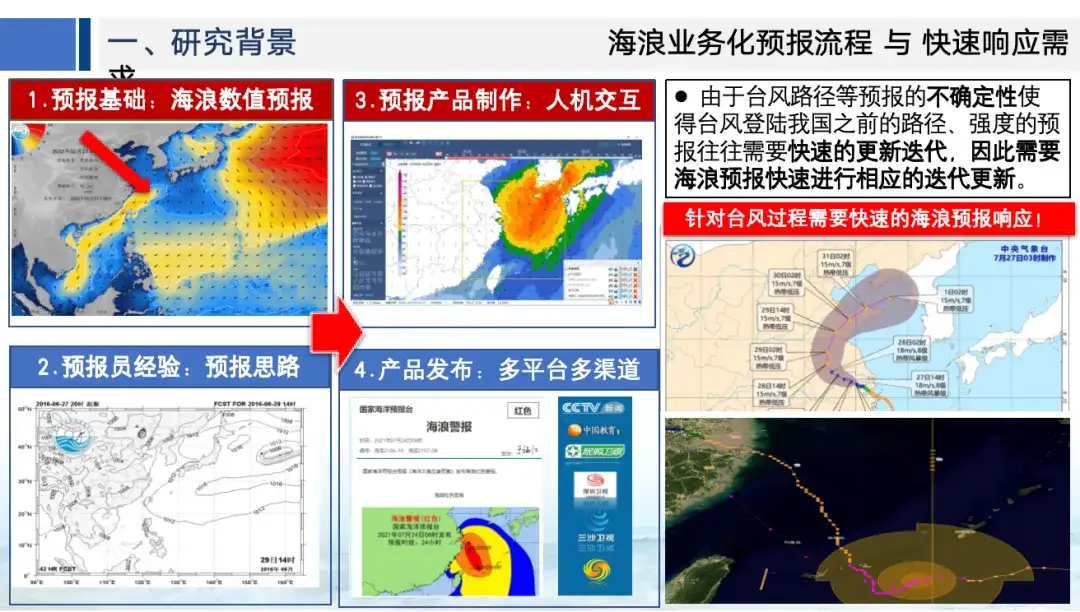
In recent years, with the continuous advancement of artificial intelligence and computing technology, intelligent forecasting has become increasingly mature. In addition, past numerical wave forecasts have accumulated a large amount of training data, which also provides important basic support for the development of intelligent wave forecasting.In this case, intelligent wave forecasting stands out with its characteristics of short time, simplicity, convenience and high prediction accuracy.
Intelligent wave forecasting practice: 72-96 hours of forecast can be completed in 1 minute
Intelligent forecasting technology mainly uses deep learning methods to simulate numerical and manual experience forecasting processes, and then establishes an intelligent forecasting model for wave elements, and combines cloud computing and edge computing technologies to build a lightweight and rapid forecasting system.
Intelligent wave forecasting can serialize and output the forecast results of waves at different times in parallel. Similar to the traditional physical model, the wind field sequence from T0-m to T0+n can be directly used to predict the wave field sequence from T1 to T0+n.
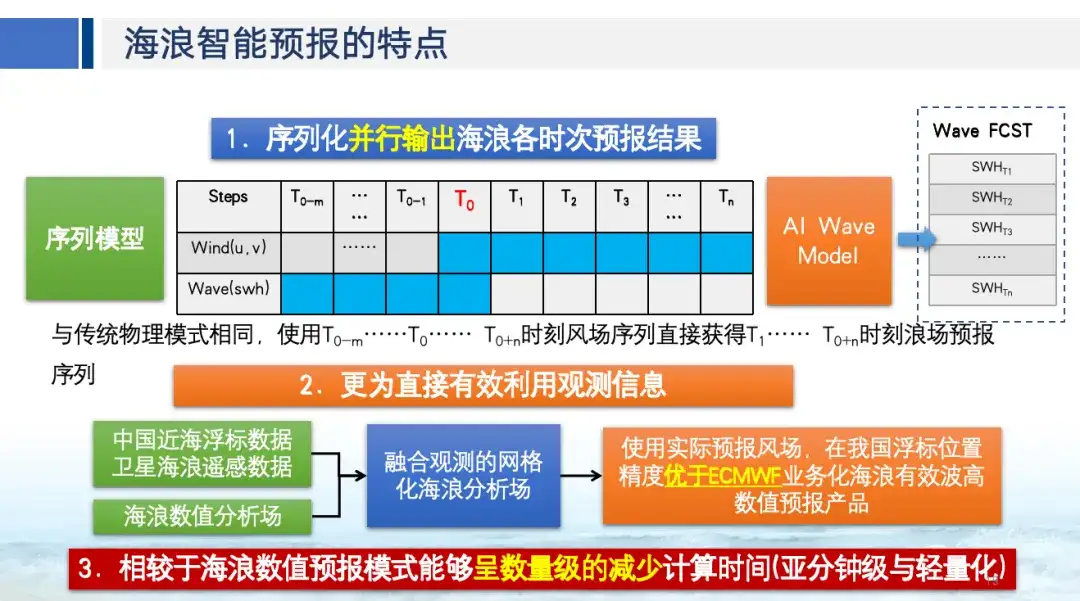
Secondly, intelligent wave forecasting can make more direct and effective use of observation information. For example, it can integrate observation data such as China's offshore buoy data and satellite wave remote sensing data into the wave numerical analysis field. This correction technology can improve the accuracy of the analysis field used for training, thereby improving the forecast results.
Finally, compared with the numerical wave forecasting model, intelligent wave forecasting can significantly reduce the calculation time, completing a 72-96 hour forecast in just 1 minute.
Correcting training data to improve wave forecast accuracy
In terms of building intelligent wave forecast products, Li Benxia mentioned that her team had developed a set of intelligent wave forecast products for China's coastal waters and the northwest Pacific. The training data used is shown in the figure below.The main data used include the fifth-generation atmospheric reanalysis data ERA5 released by the European Centre for Medium-Range Weather Forecasts (ECMWF), the global climate reanalysis data released by the National Centers for Environmental Prediction (NCEP) of the United States, the wave analysis field data of the European Centre for Medium-Range Weather Forecasts (ECMWF), and the wave analysis field data independently developed by the National Marine Environmental Forecast Center (NMEFC).
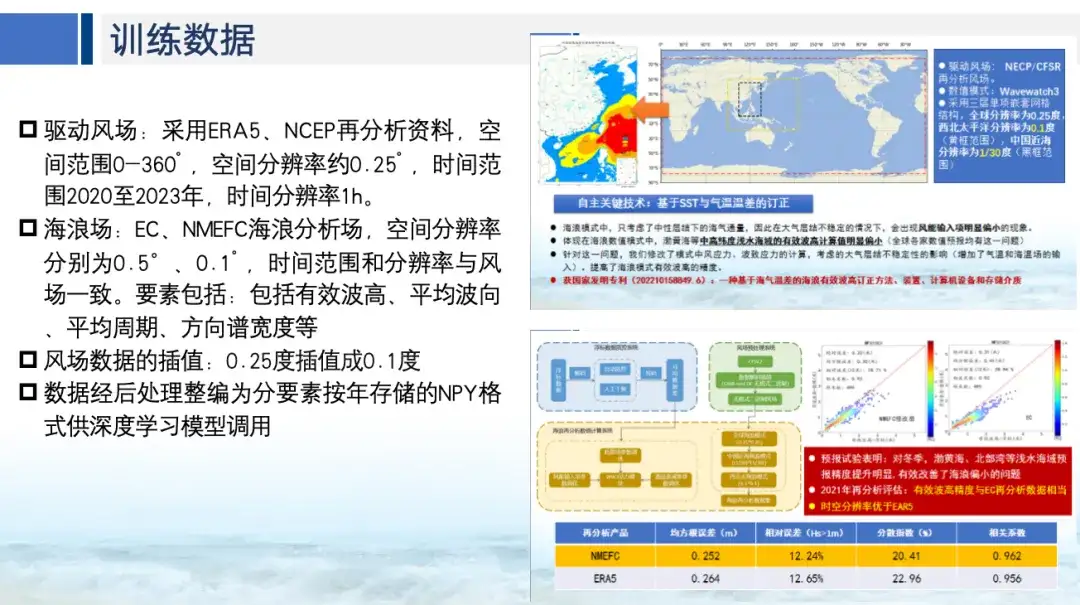
It is worth noting that during the development of the wave training data, the team paid special attention to the fact that when the atmospheric stratification is unstable, the wind energy input may be significantly smaller.We have independently developed a method for correcting effective wave height based on sea-temperature difference, which can significantly improve the accuracy of wave simulation and address the problem of small wave forecasts in shallow waters.
Specifically, the researchers selected dozens of offshore operational wave observation buoys from north to south along the coast of China, and integrated satellite data from the North Indian Ocean region for correction.The observation data are fused into the wave numerical analysis field through optimal interpolation.
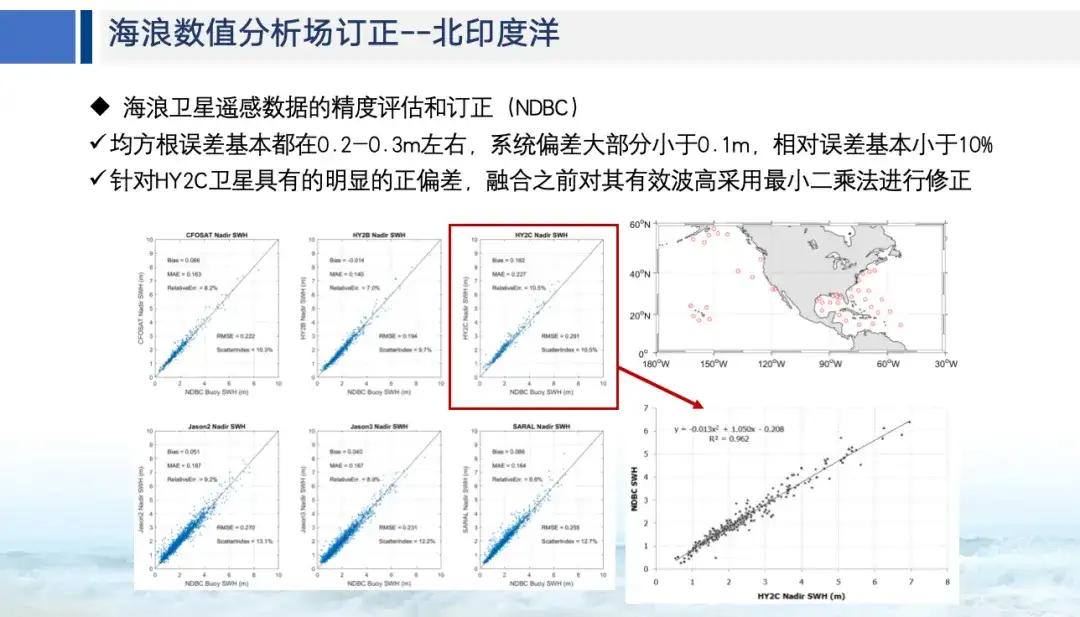
In specific applications, researchers also performed fusion field correction on data with obvious systematic deviations of Ocean II C magnitude. The results showed thatThe fusion field has obvious improvements in terms of system deviation, root mean square error, relative error and Scatter Index within the full wave height, has high real-time performance, and can improve the accuracy of the numerical model field.
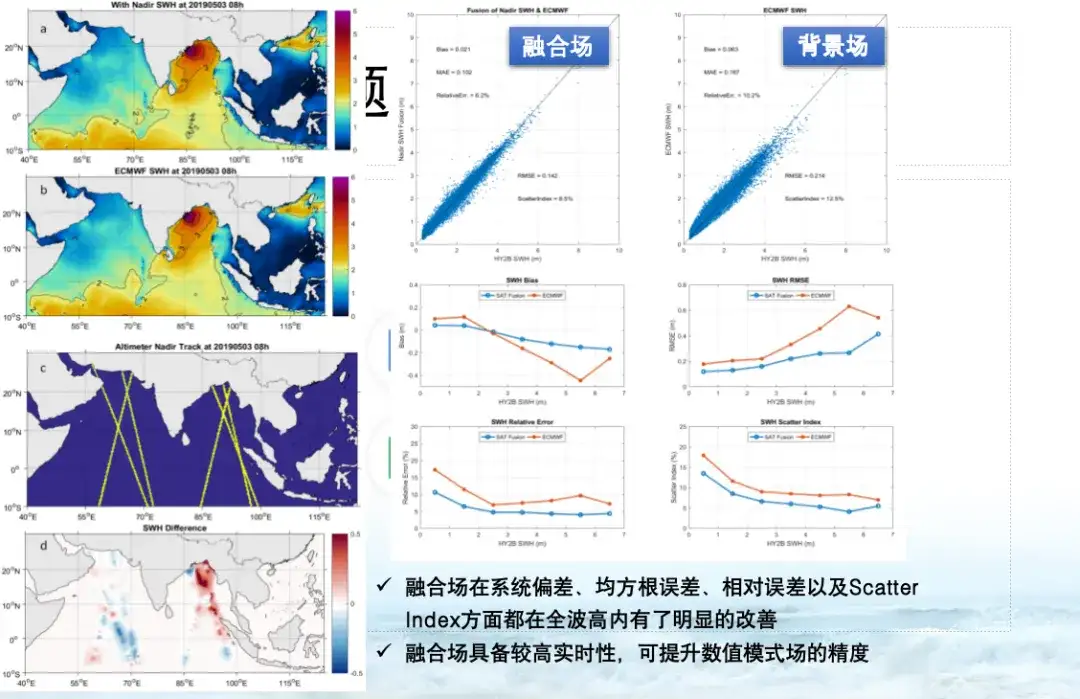
The first commercially available intelligent wave forecasting system has been launched and has passed expert review
Based on the above data, Li Benxia's team used deep learning methods such as Vision Transformer to develop an intelligent wave forecasting system for China's coastal waters and the northwest Pacific.This is my country's first intelligent wave forecasting system put into commercial operation, and it passed expert review in June 2022.
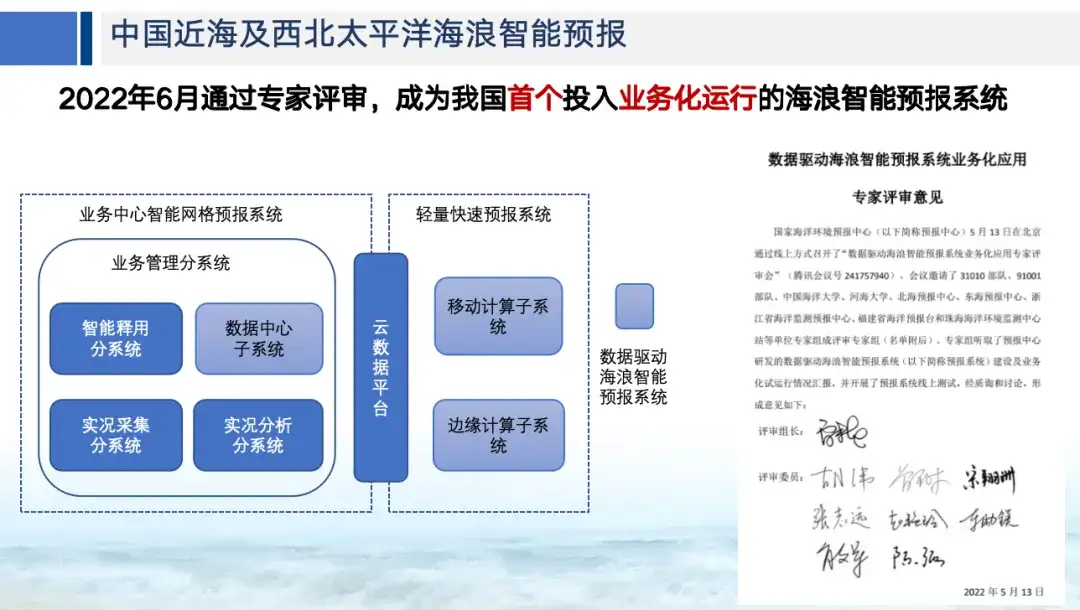
It is worth mentioning that Vision Transformer is a neural network architecture that specializes in processing sequence data. As one of the current leading deep learning architectures, it can capture long-distance dependencies and solve the problem of traditional RNN in processing long sequences. It can also process all elements in the sequence at the same time, thus supporting highly parallelized computing.Model training speed is significantly accelerated.
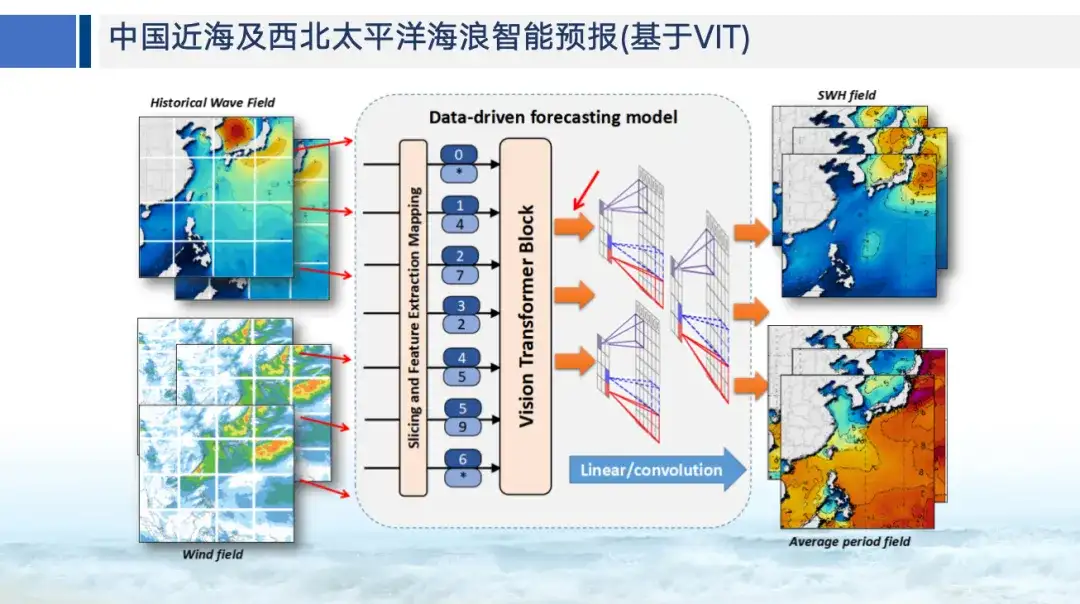
As shown in the figure below, in the forecast of the average period of ocean waves, from the perspective of spatial distribution characteristics,This set of AI intelligent wave forecasts is very consistent with the European ECMWF's operational wave numerical forecast results.
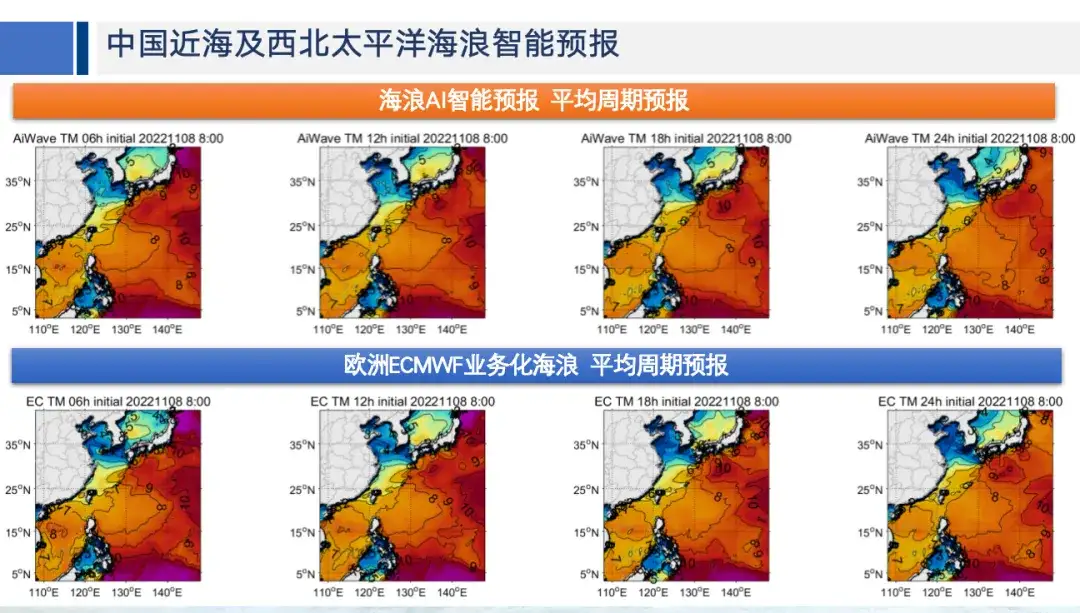
In terms of the error variation with forecast time, the AI intelligent forecast of ocean waves is comparable to the ECMWF operational ocean wave numerical forecast accuracy. As shown in the figure below, the observation data is fused to correct the training data, and the effect of ocean wave prediction is better (yellow line).This shows that it is necessary to fuse and correct the observed data in wave prediction.
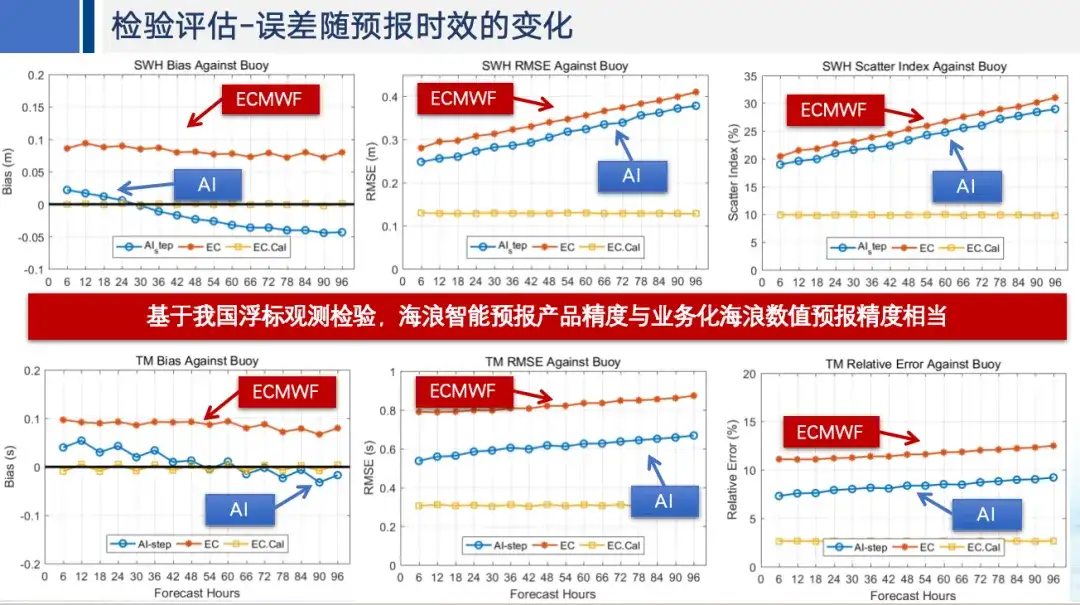
In addition, in terms of error variation with wave height,The forecast results of AI intelligent wave forecast are obviously smaller when the effective wave height is above 4m.
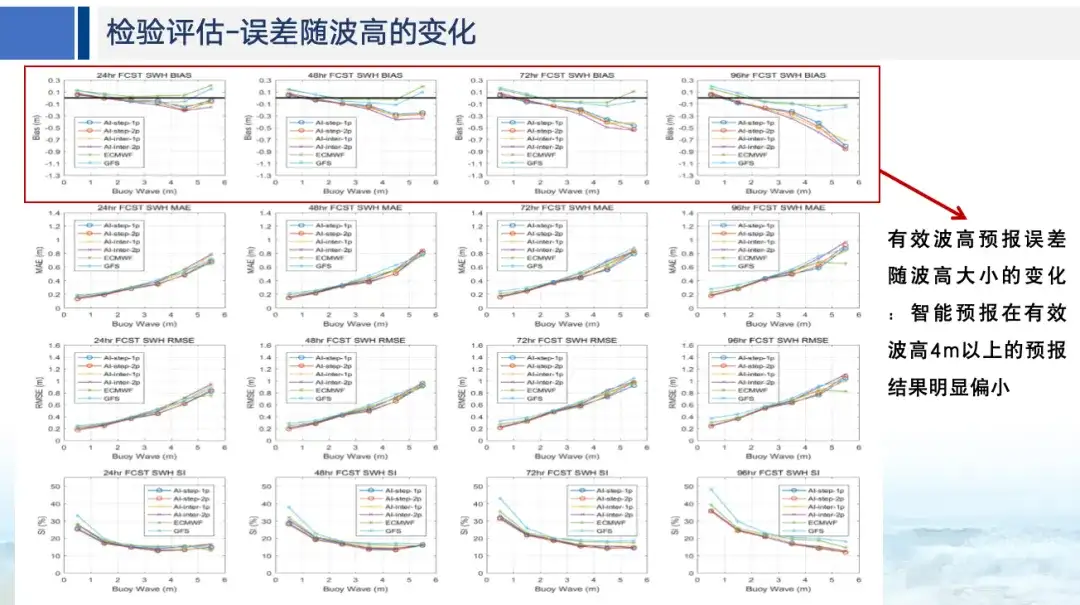
In order to improve the problem of underestimation of strong sea conditions caused by typhoons, the research team carefully selected wind and wave fields of typhoon processes in China's coastal waters from 2021 to 2023 to construct learning samples. The results show thatIncreasing the learning samples of typhoon processes can improve the forecast accuracy of the intelligent model for large wave processes. The larger the typhoon waves, the greater the improvement in accuracy, and the forecast of the large wave area near the typhoon center will be smoother.
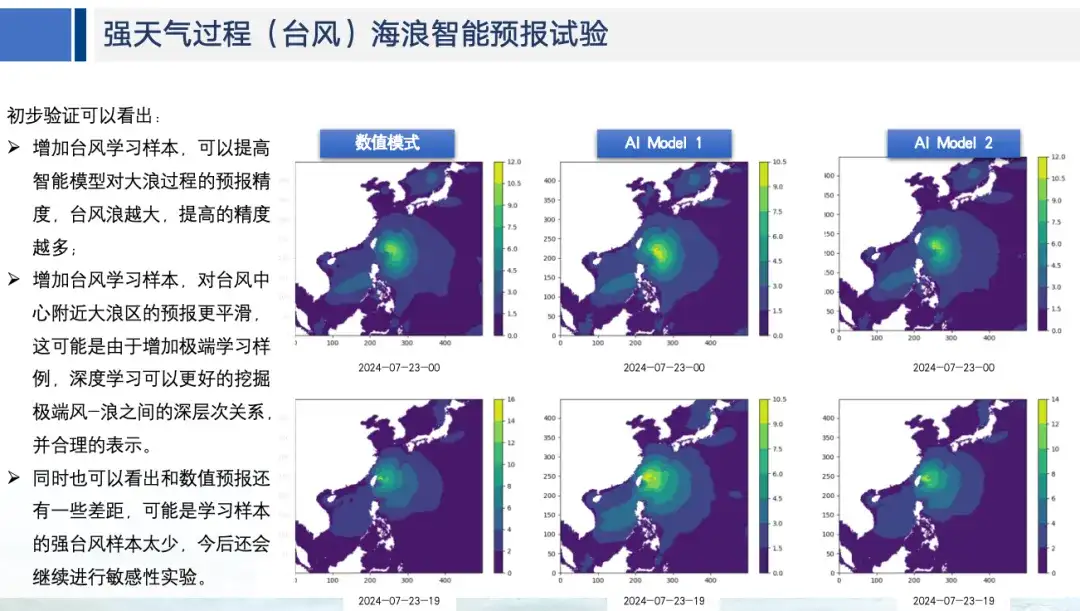
Fruitful achievements: Intelligent wave forecasting ushers in a new era
In addition, the research team took into account the characteristics of the southern and northern Indian Ocean wave fields being dominated by wind waves and swell waves respectively.An intelligent wave forecasting model based on State Force Coupled Network (SFCN) was developed.
The model adopts a dual-branch structure, processes wind and wave fields through different branches, and realizes loose coupling of wind and wave fields through high-level semantics of the network, avoiding false wind-wave coupling, and solving the problem that the traditional convolutional long short-term memory network has poor forecasting ability in areas with weak wind-wave relationship. The effective wave height along the track of the HY-2B satellite was used for testing, and the results showed that the calculation efficiency of SFCN was greatly improved, and the forecast results were highly close to the numerical forecast.
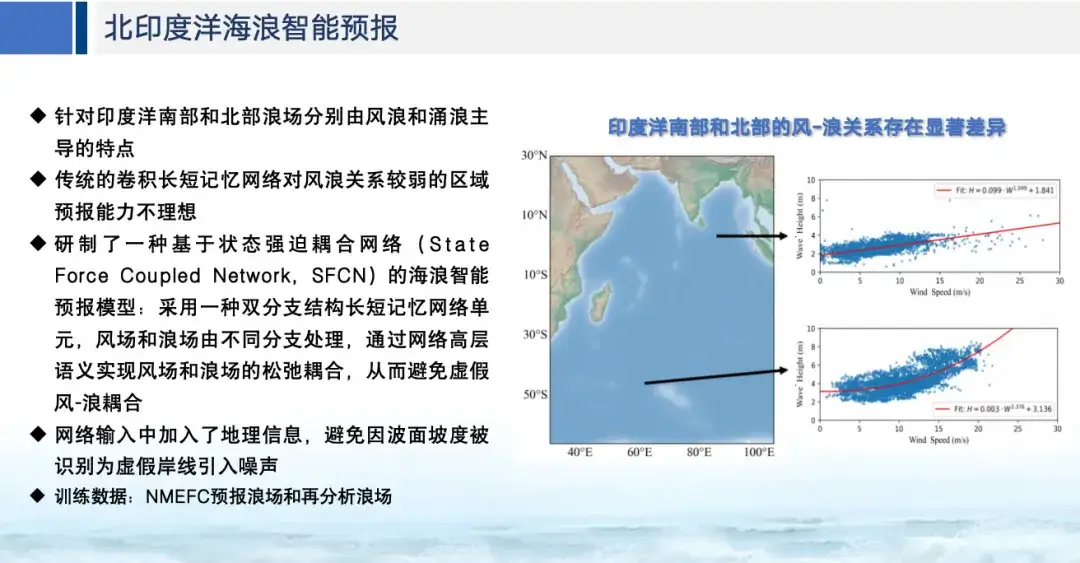
It is worth noting that the shipborne lightweight forecasting system established based on this model has been used in the 2023 Jiaolong Northwest Pacific scientific expedition and the onboard forecasting support for China's 13th Arctic scientific expedition, achieving commercial promotion and application.
Currently, the research team is exploring a global wave intelligent forecasting system based on super-resolution models.The amount of training data and computing power grow exponentially with the increase in accuracy, but due to the limitations of graphics card memory and training time, it is difficult to directly train a global high-resolution model. Therefore, the research team plans to proceed in two steps: first, use Swin Transformer to build a coarse-resolution global model and perform coarse-resolution feature mining; second, build a super-resolution model to supplement detailed information and realize a high-resolution global wave forecast model. The forecast test results are shown in the figure below:
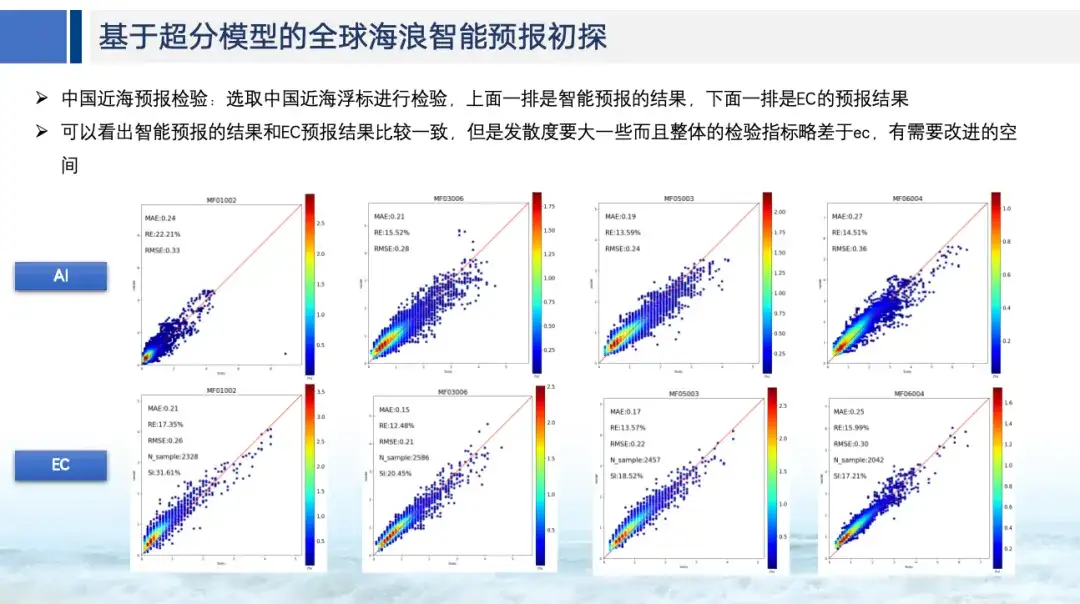
Finally, Li Benxia focused on introducing the rolling correction technology for numerical wave forecasting based on machine learning developed by the team.This technology introduces a true value observation sequence, which enables the neural network to obtain the difference information between the forecast values that have already occurred and the actual wave observations. As time goes on, the neural network can adaptively adjust the values of future forecasts and use the changing observation sequence to complete the rolling wave forecast correction. It is particularly suitable for high-precision wave forecasts at on-site real-time observation engineering points (such as offshore wind farms).
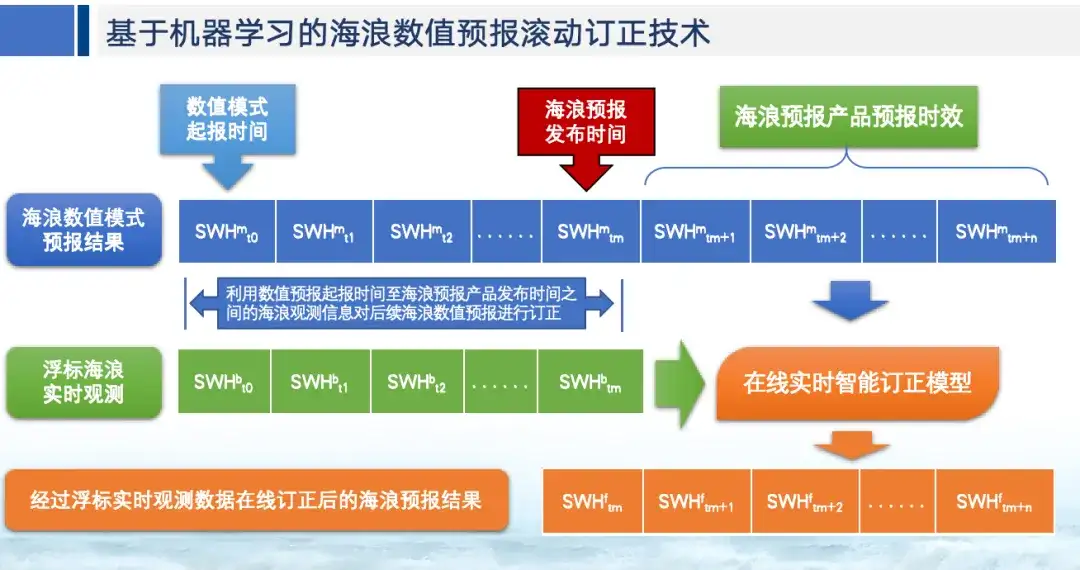
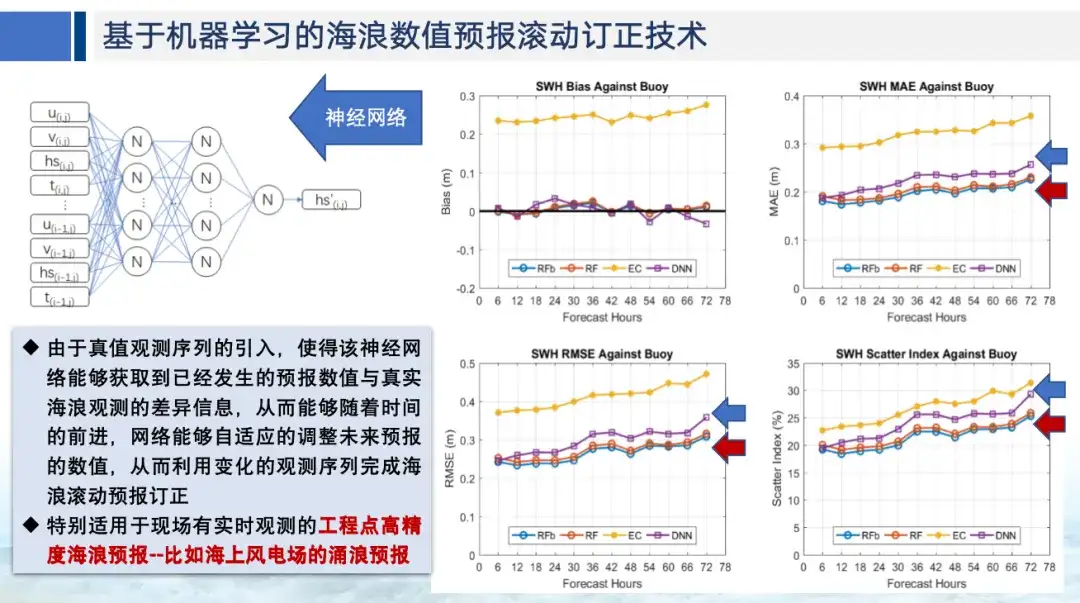
As an emerging interdisciplinary subject, AI oceanography combines knowledge and technologies from the fields of ocean science, artificial intelligence, atmospheric science, and computer science, and has broad development prospects. Although the current data-driven AI model is still inseparable from the traditional numerical model, the training and prediction of the model need to be based on the numerical model and the accuracy of the numerical model results.But in the future, the organic combination of AI forecasting and traditional numerical forecasting will be an effective way to achieve breakthroughs in forecasting technology.
About Li Benxia
Dr. Li Benxia is currently the director of the Wave Forecast Office of the National Marine Environmental Forecast Center. She serves on the editorial board of the Chinese core journals "Ocean Forecast" and "Frontiers of Marine Science". She is also a part-time master's tutor at the Ocean University of China, a review expert at the National Natural Science Foundation of China, a degree thesis review expert at the Ministry of Education, and a national marine disaster risk survey expert.
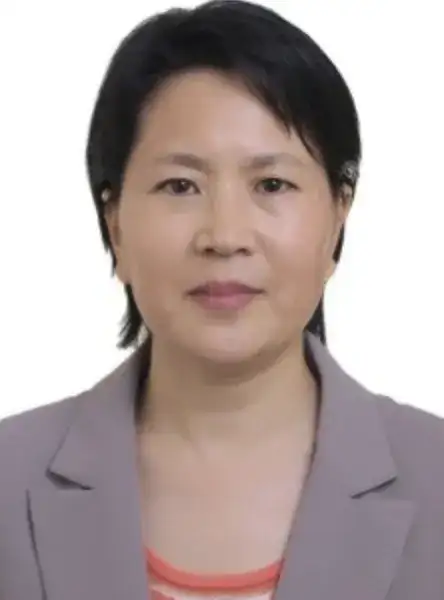
Her research interests include intelligent ocean wave forecasting, numerical wave simulation and early warning technology, marine disaster risk assessment and zoning, and climate change response.
It is worth noting that Li Benxia was the deputy director of the Forecast and Warning Professional Working Committee of the Public Safety Science and Technology Society, and presided over more than 10 national and provincial scientific research projects, including national key R&D projects, marine public welfare industry scientific research projects, and global change and sea-air interaction projects. As the main person in charge, he completed the forecast and guarantee work of many tasks such as the "Marine Environment Forecast and Guarantee for the Construction of the Hong Kong-Zhuhai-Macao Bridge Island Tunnel Project", "Shenzhen-Zhongshan Channel Forecast and Guarantee", and "Marine Environment Forecast and Guarantee for the Trial Mining of Combustible Ice in the South China Sea". He edited and published 3 national standards, 3 industry standards, 3 invention patents, 1 translated book, and published more than 20 academic papers in core journals at home and abroad.