Realize Protein Dynamic Docking Prediction! Shanghai Jiaotong University/Xingyao Technology/Sun Yat-sen University and Others Jointly Launched the Geometric Deep Generative Model DynamicBind
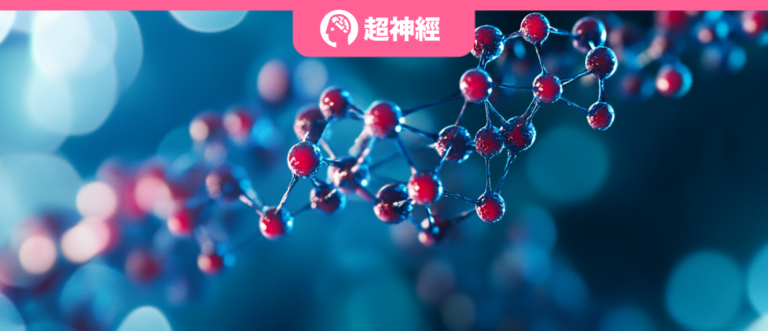
Protein is the material basis of life. Its function is closely related to the dynamics of protein structure and conformation, and is regulated by ligands. The study of protein-ligand interactions is of great significance for drug discovery and screening. Looking at its research progress, the launch of AlphaFold is a milestone breakthrough that can predict the spatial three-dimensional structure of a single protein, providing a structural basis for studying protein-ligand interactions.
However, AlphaFold can only predict the static structure of a protein at a moment in time and fails to predict the dynamic changes in protein structure.When the ligand-free protein structure predicted by AlphaFold is used as the input for docking, the resulting ligand position prediction often does not match the ligand-bound co-crystal structure. In addition, the structure predicted by AlphaFold is unlikely to show the most favorable side chain and main chain configuration for ligand binding, resulting in the relevant active sites not being in the correct position. Therefore, it is currently difficult to use AlphaFold structures for drug screening and design.
In view of this,The research group led by Zheng Shuangjia from Shanghai Jiao Tong University, in collaboration with Star Pharma Technology, Sun Yat-sen University School of Pharmacy, and Rice University, proposed a geometric deep generative model DynamicBind designed for dynamic docking of proteins.It can effectively adjust the protein conformation from the initial AlphaFold predicted state to a holo-like state, providing a new research paradigm based on deep learning and considering the dynamic changes of proteins for drug development in the post-AlphaFold era.This method was also verified by wet experiments in the international drug screening competition CACHE, and can screen competitive lead compounds for difficult-to-drug targets for the treatment of Parkinson's disease.
The study, titled “DynamicBind: predicting ligand-specific protein-ligand complex structure with a deep equivariant generative model,” was published in Nature Communications.
Research highlights:
* Using advanced deep diffusion models and equivariant geometry neural network technology, protein conformation generation and ligand pose prediction are unified into one framework, realizing dynamic docking prediction of proteins and ligands
* DynamicBind outperforms traditional docking methods and deep learning-based rigid docking methods in protein-ligand docking
* DynamicBind uses the protein conformation predicted by AlphaFold to dynamically adjust the protein conformation and find the optimal conformation that best suits the ligand
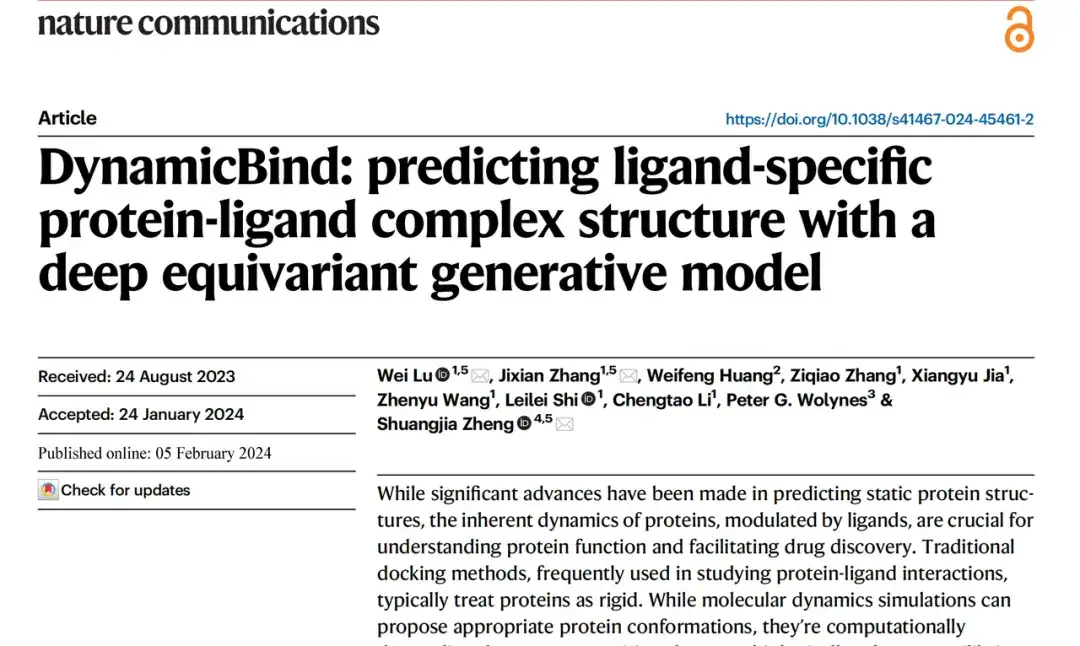
Paper address:
https://www.nature.com/articles/s41467-024-45461-2
The open source project "awesome-ai4s" brings together more than 100 AI4S paper interpretations and provides massive data sets and tools:
https://github.com/hyperai/awesome-ai4s
Dataset: Based on the PDBbind dataset, the MDT test set is used to expand the evaluation scope
The researchers first used the PDBbind dataset to train the model's training, validation, and test sets in chronological order, paired with experimentally measured binding affinities.Since the PDBbind test set contains approximately 300 structures from 2019, including many non-small molecule ligands (53 are peptides), the researchers expanded the scope of the evaluation with a curated major drug target (MDT) test set.
The MDT test set includes 599 structures archived in 2020 or later, including drug-like ligands and four major families of proteins, including kinases, GPCRs, nuclear receptors, and ion channels. These protein families represent the targets of approximately 70%'s FDA-approved small molecule drugs and are representative.
DynamicBind: A geometric deep learning-based model for predicting the structure of dynamic complexes
Different from traditional docking methods that treat proteins as mostly rigid entities, DynamicBind uses advanced deep diffusion models and equivariant geometry neural network technology to unify the two traditionally separate steps of protein conformation generation and ligand pose prediction into a single framework, achieving dynamic docking prediction of proteins and ligands.At the same time, as an end-to-end deep learning method, it is also several orders of magnitude faster than traditional MD simulations in sampling a wide range of protein conformational changes.
DynamicBind accepts PDB-formatted apo-like structures and several widely used small molecule ligand formats, such as SMILES or SDF.During inference, the model randomly places ligands whose seed conformations are generated around the protein using RDKit. During the training phase, the model aims to learn the process from apo-like conformation to holo conformation. During inference, the model updates the initial input structure 20 times.
As shown in Figure a below, pink represents the holographic state (holo) of the protein conformation, green represents the initial apolipoprotein and model predicted conformations, cyan represents the native ligand, and orange represents the predicted ligand.
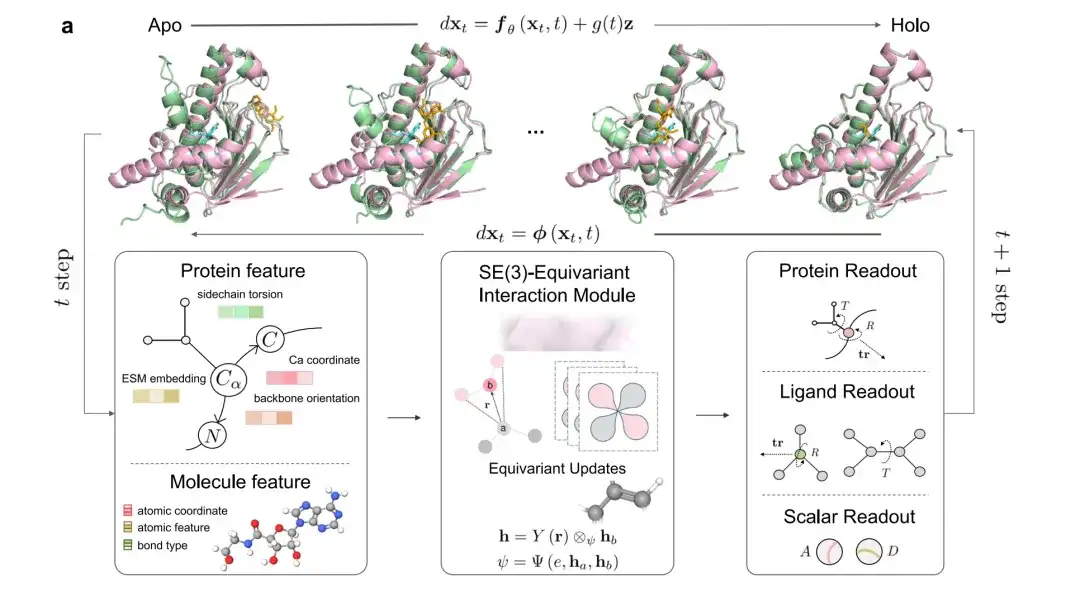
In each iteration, the features and coordinates of the protein and ligand (including sidechain torsion, Ca atomic coordinates, etc.) are input into a SE(3) Equivariant Interaction Module. The model outputs include the global translation and rotation of the ligand and each protein residue, the torsion angle of the ligand and the rotation of the chi angle of the protein residue, and two prediction modules (binding affinity A and confidence score D).
Figure b below shows the sampling efficiency of the DynamicBind model. In the transition from DFG-in to DFG-out of the kinase protein, the model can predict two different holographic conformations when the protein binds to two different ligands. And DynamicBind can predict the bound protein conformation within 20 steps, while the all-atom MD simulation to find the same binding state requires millions of steps.
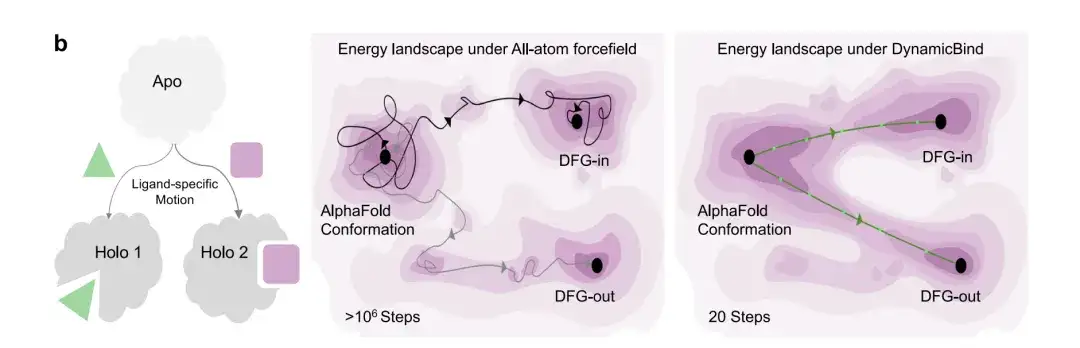
DynamicBind is an all-rounder in protein dynamic docking prediction. It performs well in five major tasks.
To evaluate the performance of DynamicBind’s model, researchers tested it on five tasks, including:
(1) Benchmark DynamicBind against current docking methods;
(2) the ability to sample conformational changes in a large number of proteins;
(3) the scope of addressing protein conformational changes;
(4) the ability to predict hidden pockets to achieve dynamic docking;
(5) Screening performance in antibiotic benchmark tests.
DynamicBind outperforms traditional docking methods and deep learning-based rigid docking methods
During testing, the researchers did not use holographic structures as input and assumed that holographic protein conformations were not available, using only protein conformations predicted by AlphaFold as input.Because the holo conformations exhibit strong shape and charge complementarity with the cocrystallized ligands, the process of ligand pose prediction is simplified.
As shown in Figures a and b below, the researchers compared DynamicBind with other baseline models on the PDBbind and MDT datasets. DynamicBind outperformed other methods at different RMSD thresholds. Specifically, the proportion of DynamicBind ligands with RMSD thresholds below 2Å (5Å) on the PDBbind test set was 33% (65%), and on the MDT test set it was 39% (68%).
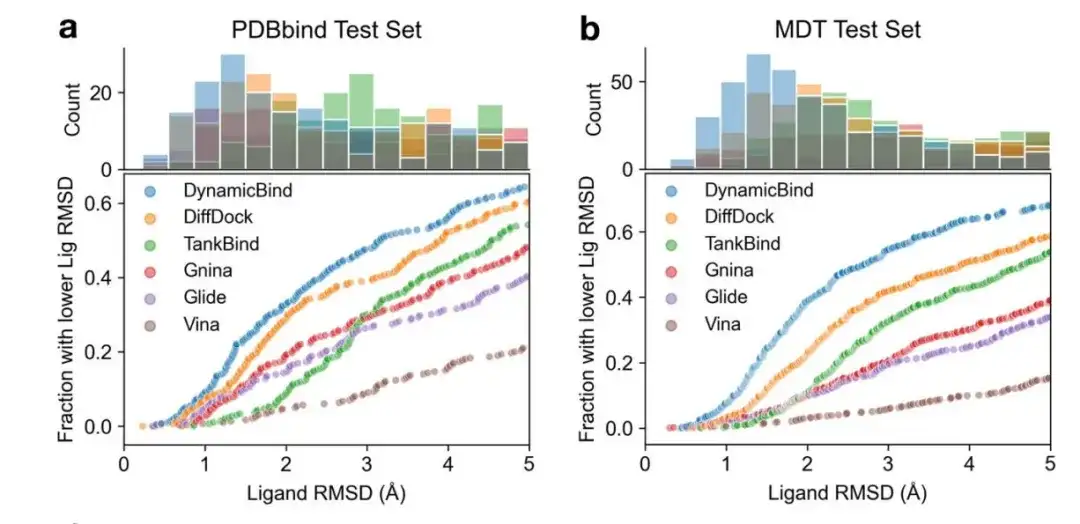
However, when evaluating the model, only the RMSD value of the ligand is used for evaluation, which is beneficial to deep learning-based models such as DiffDock, TankBind, and DynamicBind because they have a higher tolerance for conformational conflicts; but it is disadvantageous to the force field-based docking methods Gnina, Glide, and Vina that strictly implement Van der Waals forces, thus affecting the objectivity of the model evaluation. Therefore, the researchers used ligand RMSD and conflict scores to evaluate the success rate of ligand prediction.
Figure c shows the success rate of ligand prediction using strict criteria (ligand RMSD < 2 Å, conflict score < 0.35) and more relaxed criteria (ligand RMSD < 5 Å, conflict score < 0.5). Under the more stringent conditions, DynamicBind's success rate (0.33) is 1.7 times that of the best baseline DiffDock (0.19).
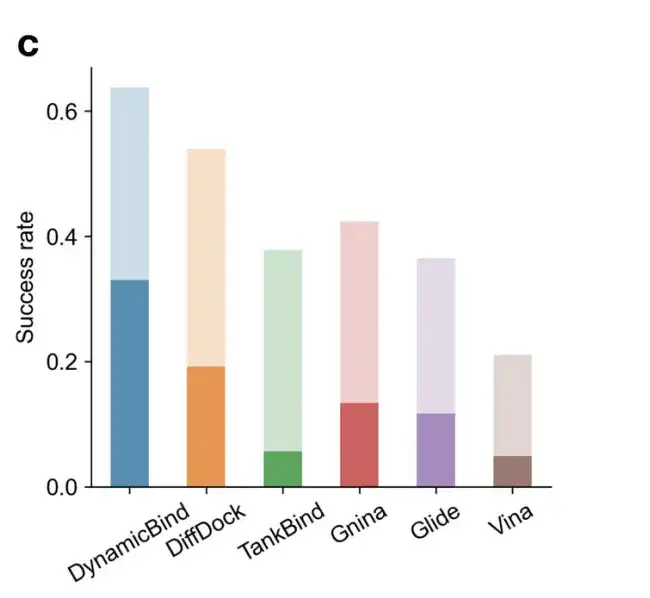
In addition, even when the RMSD between the initial pocket and the crystal structure is large, the pocket RMSD predicted by DynamicBind is significantly smaller than that predicted by AlphaFold, as shown in Figure d below.
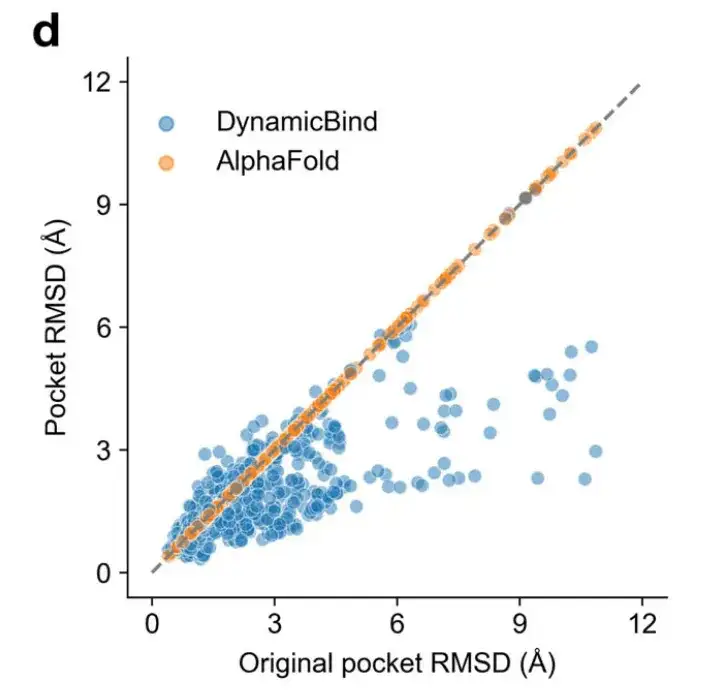
Given the ability of DynamicBind to generate diverse conformations and inspired by the LDDT scores of AlphaFold, the researchers developed a contact-LDDT (cLDDT) scoring module to select the most suitable complex structure from the predicted output.
As shown in Figure e below, the cLDDT predicted by DynamicBind has a good correlation with the actual ligand RMSD, indicating its effectiveness in selecting high-quality complex structures.
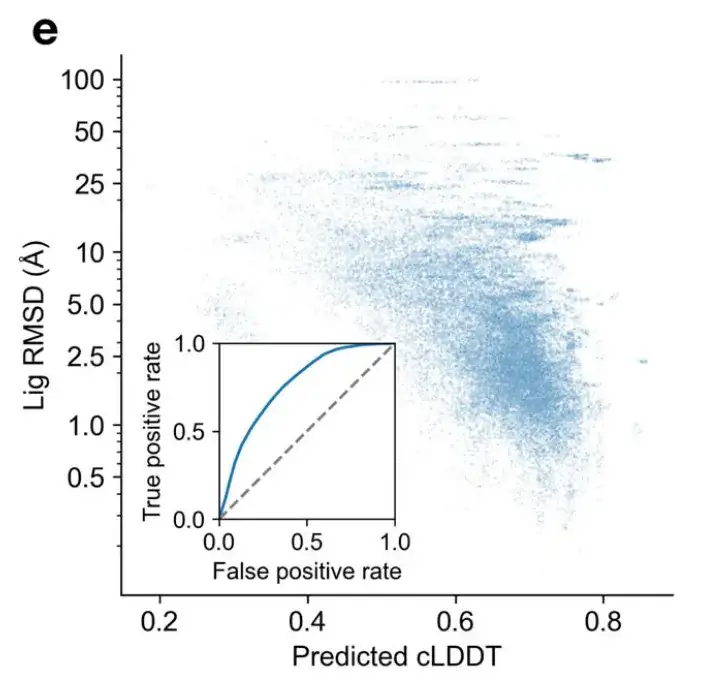
As shown in Figure f below, as the number of generated samples increases, the success rate of the DynamicBind model in predicting ligand poses also increases.
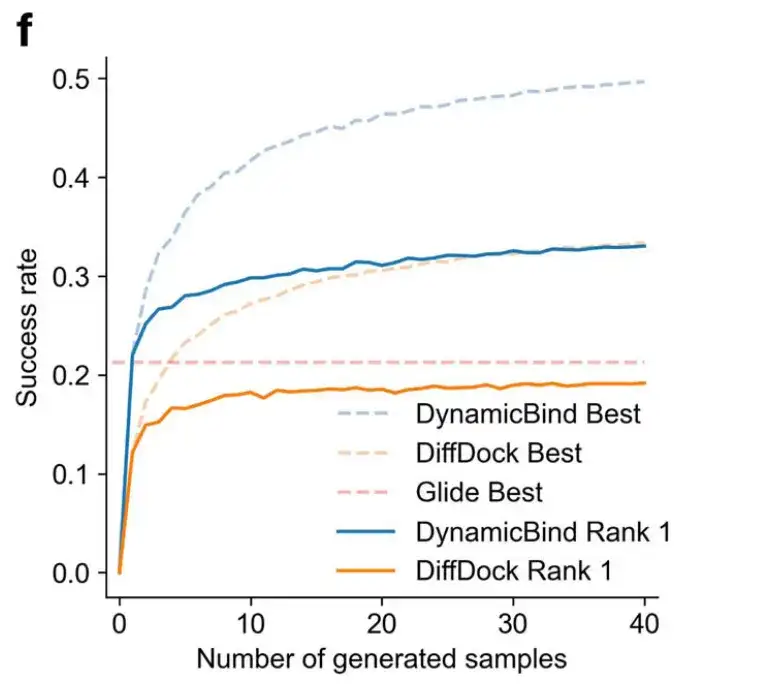
DynamicBind can capture ligand-specific protein conformational changes
Traditional docking protocols usually perform protein conformation sampling as a separate step from the docking process. However, in many cases, two different ligands may fit into mutually exclusive protein conformations. In previous docking models, the protein must be preset to the correct conformation before it is possible to identify the appropriate binding pose of the ligand.In contrast, DynamicBind uses the protein conformation predicted by AlphaFold to dynamically adjust the protein conformation and find the optimal conformation that best suits the ligand of interest, see Figure a below.
Figures b to e show the RMSD of the ligand and pocket predicted by DynamicBind and AlphaFold in PDB 6UBW and PDB 7V3S structures. For PDB 6UBW, the ligand RMSD predicted by DynamicBind is 0.49 Å and the pocket RMSD is 1.97 Å, while the pocket RMSD of the AlphaFold structure is 9.44 Å. For PDB 7V3S, the ligand RMSD predicted by DynamicBind is 0.51 Å and the pocket RMSD is 1.19 Å (AlphaFold 6.02 Å).
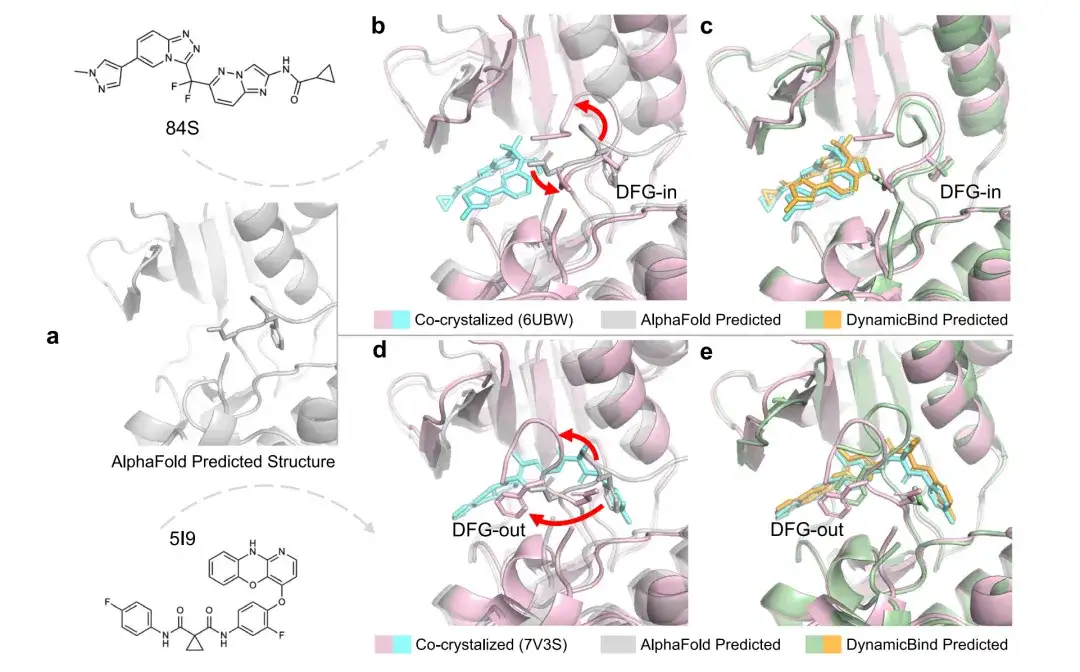
Figures f and g show how proteins labeled by UniProt ID, starting from the same initial structure, gradually move toward the DFG-in conformation after binding to type I inhibitors and tend to the DFG-out conformation when interacting with type II inhibitors.
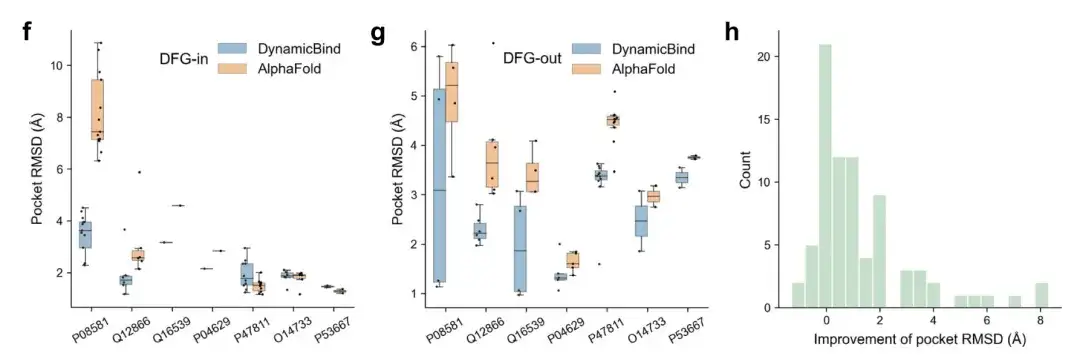
Figure h reveals that most of the protein structures predicted by DynamicBind show lower pocket RMSD compared to the initial AlphaFold structure.
The above results demonstrate that DynamicBind is able to capture ligand-specific conformational changes.That is, DynamicBind can identify compounds that bind well to other possible conformations of the protein, even if the particular conformation differs from the initially provided protein structure.
DynamicBind covers multiple scales of protein conformational changes
The researchers evaluated DynamicBind using six different types of cross-scale conformational changes, ranging from picoseconds to milliseconds.As shown in the figure below, pink represents the crystal structure, white represents the AlphaFold structure, green represents the structure predicted by DynamicBind, cyan represents the native ligand, and orange represents the ligand predicted by DynamicBind.
Based on comparison with the crystal structure,Δpocket RMSD measures the pocket RMSD difference between the model-predicted protein structure and the AlphaFold structure.A negative Δpocket RMSD indicates that the structure predicted by DynamicBind is closer to the structure predicted by AlphaFold than to the crystal structure.
Δclash measures the difference in clash scores between the predicted protein-ligand pair and the grafted ligand in the AlphaFold structure.A negative Δclash indicates fewer clashes in the predicted complex.
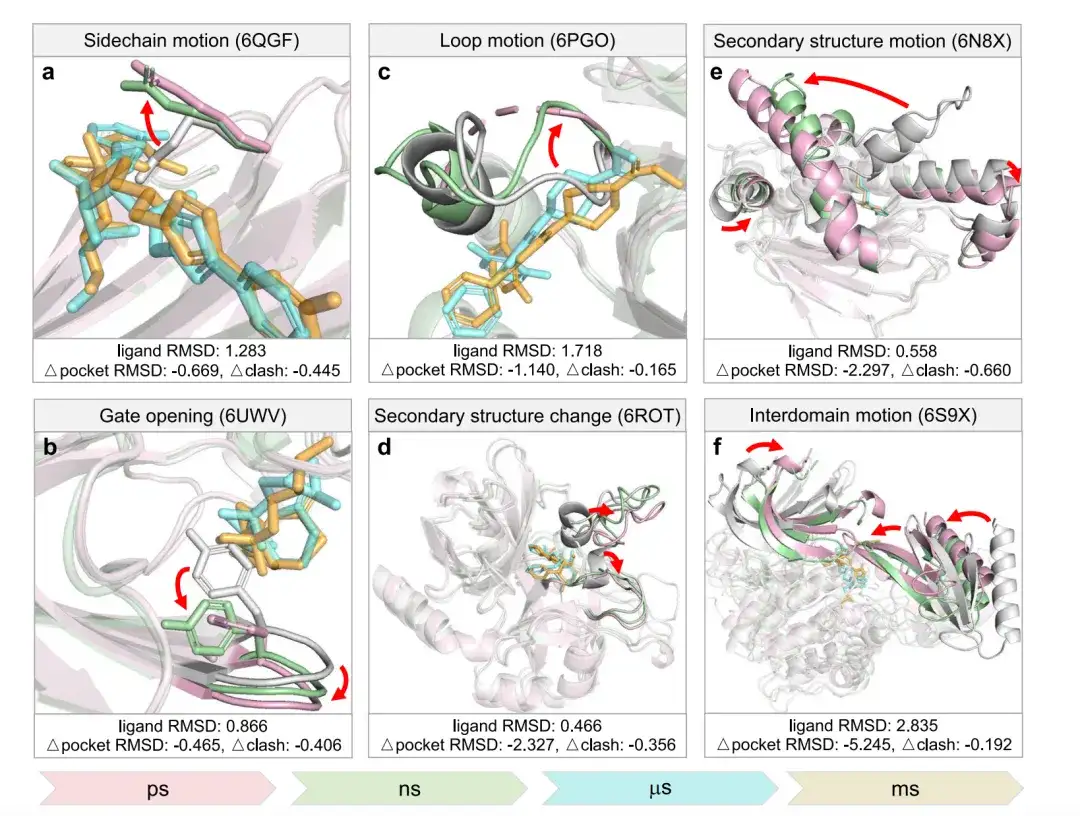
In Figure a, the native ligand clashes with a side chain of the superimposed AlphaFold structure; in the DynamicBind prediction, this side chain moves toward the native conformation, resolving the conflict. In Figure b, a tyrosine in the AlphaFold structure blocks part of the pocket; in the DynamicBind predicted structure and the native structure, this part of the pocket becomes accessible. In Figure c, a flexible loop intersects the ligand, and in the DynamicBind prediction it moves away, which is consistent with the native structure.
In panel d, the α-helix becomes a loop near the ligand binding site. In panel e, the heat shock protein Hsp90α undergoes a large movement of secondary structure as it transitions from a closed to an open state. In panel f, the two domains of the AKT1 kinase condense to form a pocket that did not exist before.
In summary, when the ligand binding pocket is not spacious enough or does not form the conformation predicted by AlphaFold, the DynamicBind model can predict various conformational changes associated with ligand binding.
DynamicBind identifies cryptic binding sites
Proteins often generate cryptic pockets during dynamic processes, which can reveal druggable sites that are not discovered in static structures, thereby making previously "undruggable" proteins potential drug targets.Using SET domain-containing protein 2 (SEtD2) as a case study, the researchers demonstrated the utility of DynamicBind in revealing these cryptic pockets.
SETD2, a histone methyltransferase that is a key drug for the treatment of multiple myeloma (MM) and diffuse large B-cell lymphoma (DLBCL), has a cryptic pocket and is the target of a highly selective compound, EZM0414, which is currently in phase I clinical trials.
As shown in Figures a and b below, all SETD2 homologs in the training set (defined by a protein Smith–Waterman similarity of more than 0.4) co-crystallized with S-adenosylmethionine (SAM) or Sinefungin analogs, represented by lines. The cyan bar represents the ligand EZM0414 from PDB 7TY2, and the pink bar represents the protein.
In Figure c, white represents the AlphaFold structure and its surface, where the cryptic sites are blocked, resulting in a large number of conflicts with the transplanted EZM0414.
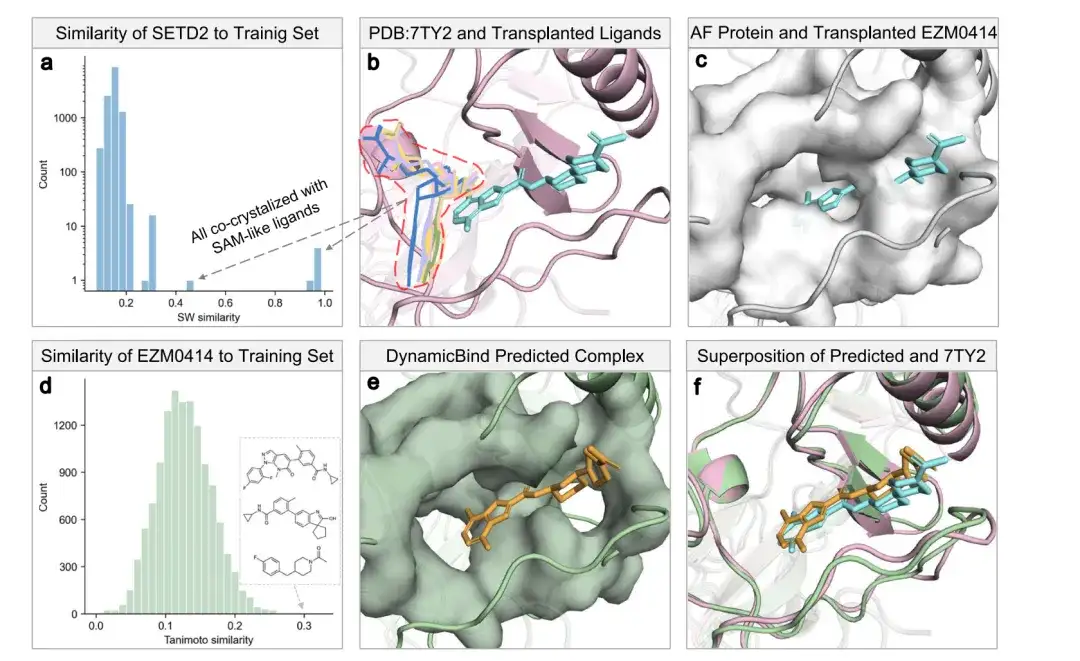
Figure d confirms that EZM0414 is an unseen ligand, and even the most similar Tanimoto ligand deviates significantly from EZM0414. Figure e shows the protein-ligand complex structure predicted by the DynamicBind model, which takes the AlphaFold predicted SETD2 structure and the SMILES representation of EZM0414 as input. Figure f shows the overlap of the DynamicBind predicted protein-ligand complex structure with the crystal structure of the SETD2-EZM0414 complex (PDB 7TY2).
Judging from the results, DynamicBind achieved dynamic docking of the hidden pocket, not only successfully placing the ligand, but also finding a more suitable pocket conformation (the RMSD of the obtained ligand was 1.4 Å, and the RMSD of the pocket was 2.16 Å).
DynamicBind achieves better drug screening performance on antibiotic benchmarks
In the target-based drug discovery process, both screening of potential drug candidates and counter-screening (identifying protein targets for specific compounds) are crucial.To evaluate the screening performance of the DynamicBind model in practice, the researchers added an affinity prediction module to the model, trained it using experimentally measured binding affinity data obtained from the PDBbind dataset, and evaluated it on drug screening test data from the antibiotic proteome released in 2023 (including 12 protein targets and nearly 3,000 measured activity data).
As shown in Figure a below, DynamicBind surpasses common docking methods such as VINA and DOCK6.9, as well as the best rescoring method based on machine learning, with an average area under the receiver operating characteristic curve (auROC) of 0.68. This performance improvement is due to the dynamic docking capability of DynamicBind, which can refine the AlphaFold structure to a state closer to the native state, thereby achieving more accurate binding affinity estimation.
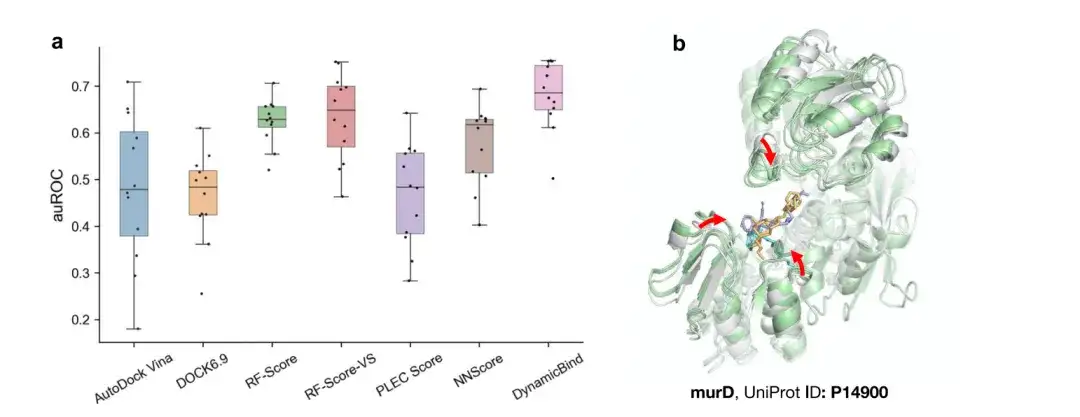
Figure b above shows that the protein murD structure predicted by DynamicBind surrounds the ligand more tightly, forming more interactions that were impossible to form in the initial AlphaFold structure.
These results indicate that DynamicBind consistently outperforms traditional docking methods and deep learning-based rigid docking methods, and the model shows great potential in virtual screening applications at the proteome level due to its binding affinity prediction capabilities.
Decoding the complex structure and function of proteins to contribute to smart drug discovery
Based on the static structure prediction of AlphaFold, the DynamicBind model innovatively introduces generative artificial intelligence technology and successfully solves the challenge of dynamic complex structure prediction. Predicting the dynamic changes of protein structure is of great significance for understanding life processes and developing new drugs. Especially in AI drug development, it can greatly improve the accuracy and clinical utility of AI drug screening.
As one of the main participants in this research result, Zheng Shuangjia's research group has long been deeply engaged in the cross-cutting frontier research of generative artificial intelligence and drug development, and has achieved fruitful results.
On June 21, 2024, Zheng Shuangjia's research group proposed a cross-modal learning method that uses perturbed high-content cell microscopy images at the phenotypic level to assist in molecular representation learning.This method can effectively build a bridge between molecules and representations, which is of great significance for drug development. The related research was published in Advanced Science under the title "Cross-Modal Graph Contrastive Learning with Cellular Images".
Paper address:
https://onlinelibrary.wiley.com/doi/10.1002/advs.202404845
On May 25, 2024, Zheng Shuangjia's research group proposed the multi-scale learning framework MUSE, which effectively integrates multi-scale information between atomic structure and molecular network scales.The study shows the potential of extending computational drug discovery to other scales. The related research was published in Nature Communications under the title "A variational expectation-maximization framework for balanced multi-scale learning of protein and drug interactions".
Paper address:
https://www.nature.com/articles/s41467-024-48801-4
On September 15, 2022, Zheng Shuangjia's research group developed a generative intelligent drug design algorithm for difficult-to-drug targets and designed PROTAC lead compounds in a short period of time.The results have been verified by animal experiments, demonstrating the great potential of the integration of information technology and biotechnology. This series of results has been positively cited and evaluated by top research groups in the field, such as the Google DeepMind AlphaFold team and the David Baker team of the University of Washington. The related research was published in Nature Machine Intelligent under the title "Accelerated rational PROTAC design via deep learning and molecular simulations".
Paper address:
https://www.nature.com/articles/s42256-022-00527-y
On February 14, 2020, Zheng Shuangjia's research group proposed a quasi-visual question-answering system based on an end-to-end deep learning framework.The study, titled "Predicting drug–protein interaction using quasi-visual question answering system," was published in Nature Machine Intelligence.
Paper address:
https://www.nature.com/articles/s42256-020-0152-y
Based on their understanding of the cross-cutting frontier research on generative artificial intelligence and drug development, Zheng Shuangjia's research group focuses on the intelligent design of drugs for diseases related to metabolism and aging, creates a new drug development model that integrates IT and BT, and is committed to contributing more to end-to-end intelligent drug discovery.
References: