New Results in the Authoritative Journal Cell Discovery! The Team of Hong Liang From Shanghai Jiaotong University Proposed the CPDiffusion Model, Which Can Design Functional Proteins at ultra-low Cost and Fully Automatically
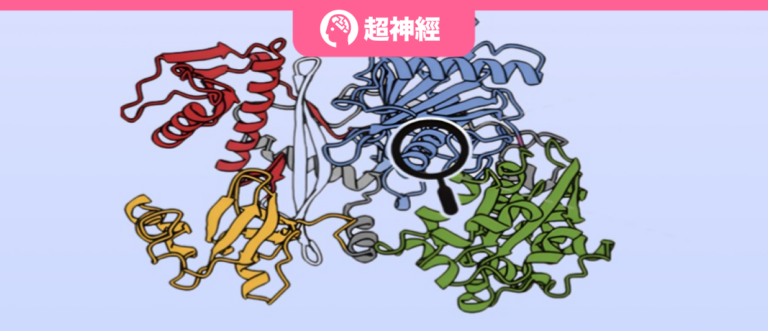
Proteins are the main executors of life activities, and the relationship between their structure and function has always been a core research topic in the field of life sciences. In recent years, with the rise of deep learning, with its powerful data processing capabilities, the model can learn the mapping relationship between protein sequence, structure and function, and design new proteins with higher stability, stronger binding affinity and higher enzyme activity, which can greatly improve the efficiency of protein design and effectively reduce its R&D costs.
However, existing methods usually require training a model with a large number of parameters on a large-scale data set, which is difficult to generalize to specific proteins with rare homologous sequences, and can often only generate proteins with relatively simple structures and functions. In addition, experimental verification shows that the designed proteins are generally less active, and those that can surpass wild-type proteins are even rarer.
In this regard, Zhou Bingxin, an assistant researcher in the research group of Hong Liang from the School of Natural Sciences/School of Physics and Astronomy/Zhangjiang Institute for Advanced Studies/School of Pharmacy of Shanghai Jiao Tong University, and others designed a diffusion probability model framework CPDiffusion.This framework combines multiple generation conditions such as protein backbone structure and active sites, and can learn the implicit mapping relationship between protein sequence, structure and function at very low training cost and data cost, and then generate diverse protein sequences. These generated sequences can pass the test with a very high success rate in wet experiment verification.
It is worth noting that the training and inference process of CPDiffusion requires almost no expert guidance.It can automatically identify highly conserved regions, and then introduce more changes in non-conserved regions based on the functions of the conserved regions to increase the diversity of the generated sequences. The study was published in Nature's Cell Discovery under the title "A conditional protein diffusion model generates artificial programmable endonuclease sequences with enhanced activity".
Research highlights:
* The study successfully designed and generated endonucleases KmAgo and PfAgo, whose DNA cleavage activity was increased by more than 10 times, significantly higher than the activity of the currently discovered medium-temperature wild-type proteins
* This study can change hundreds of amino acids at a time, providing more possibilities for protein engineering research
* The diverse generation of new protein sequences can also expand the database of protein families, providing scientists with richer research resources
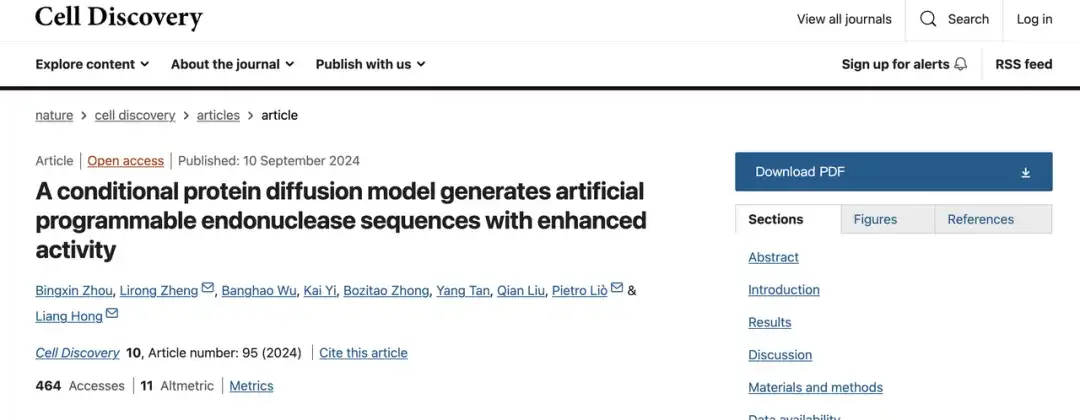
Paper link:
https://www.nature.com/articles/s41421-024-00728-2
The open source project "awesome-ai4s" brings together more than 100 AI4S paper interpretations and provides massive data sets and tools:
https://github.com/hyperai/awesome-ai4s
Dataset: Ensure sample diversity and avoid data bias
In order to learn the mapping relationship between protein sequence-structure-function,The CPDiffusion model was trained with 20,000 wild-type proteins from CATH 4.2. In addition, the researchers added 694 pAgos proteins to the training set to enhance the model's understanding of the features of the proteins to be generated.
These proteins come from the pAgo protein family compiled in previous studies, including short, long-A, and long-B pAgo proteins, ensuring the diversity of the selected samples to reduce possible data bias issues. In addition, most WT proteins in the dataset are mesophilic pAgos, and only a few long-A pAgo proteins are thermophilic.
Model architecture: 6-step automated pAgo protein design
In order to verify the effect of CPDiffusion on the generation of functional proteins, the researchers chose to focus on the pAgo protein. The pAgo protein is an endonuclease that plays an important role in the DNA interference process of prokaryotes. It can specifically recognize and cut specific single-stranded DNA or RNA sequences and has a wide range of application value in the field of diagnostics. In addition, pAgo proteins have a high affinity for substrates and can specifically recognize target sequences, which makes them an important tool for imaging and gene editing.
Researchers used the CPDiffusion framework to design new pAgo proteins.As shown in Figure a below,First, the sequence and information of the input protein (Original pAgo) are converted into a graph representation that displays the molecular biochemical and topological properties of the protein at the amino acid level.As shown in Figure b,The protein enters the forward diffusion phase, where each amino acid type in the original protein follows a certain substitution probability matrix and is destroyed in a series of steps (T steps) until the entire sequence becomes evenly distributed.
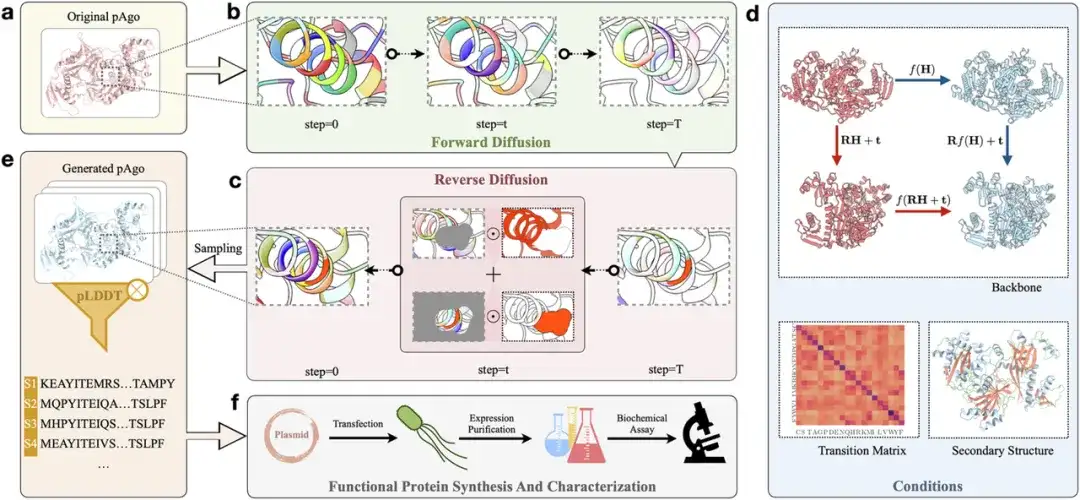
As shown in Figure c above,In the reverse diffusion stage, the researchers randomly sampled amino acids from 20 evenly distributed amino acid types and then gradually denoised the protein sequence.As shown in Figure d above,In the denoising process, the researchers guided the process based on some conditions (such as the wild-type backbone structure of the target protein, the secondary structure, and the amino acid replacement matrix Transition Matrix based on the wild-type protein). In order to ensure that the model can learn the equivariance implicit in the three-dimensional structure of the protein, the researchers used an equivariant graph convolution layer to fit the propagation function. The model then generates a joint probability distribution for each amino acid position on the protein backbone. By sampling the learned distribution (Sampling), the researchers can obtain the corresponding protein sequence (Generated pAgo).As shown in Figure e above.
Next, the researchers used AlphaFold2 to predict the structure of the generated sequences and screened out suitable sequences by evaluating indicators such as RMSD and pLDDT.As shown in Figure f below,These suitable sequences will undergo wet experiments in the laboratory (Synthesis, Characterization and Evaluation) to further confirm their actual properties, such as expression level, enzyme activity and thermal stability.
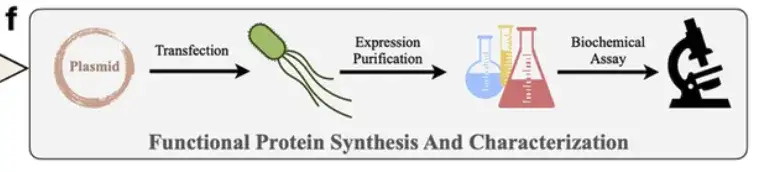
Experimental conclusion: The new protein has stronger activity and thermal stability
The researchers used mesophilic pAgo proteins (such as KmAgo) and thermophilic pAgo proteins (such as PfAgo) as candidate proteins and further generated two sets of new protein sequences. As shown in the figure below, using the generation and screening framework CPDiffusion, the researchers successfully generated 27 new artificial KmAgos (Km-APs) and 15 new artificial PfAgos (Pf-APs). These newly generated proteins have a sequence identity of 50%-70% compared to the original wild-type (WT) template, and a sequence identity of less than 40% compared to other non-template WT proteins (i.e. other WT proteins in the NCBI database).
* KmAgo is a mesophilic enzyme with relatively low DNA cleavage activity in the wild type, which limits its potential in practical applications
* PfAgo is an ultra-thermogenic enzyme. The wild type has higher DNA cleavage activity, but it usually only works at high temperatures. As the temperature drops, the activity also decreases.
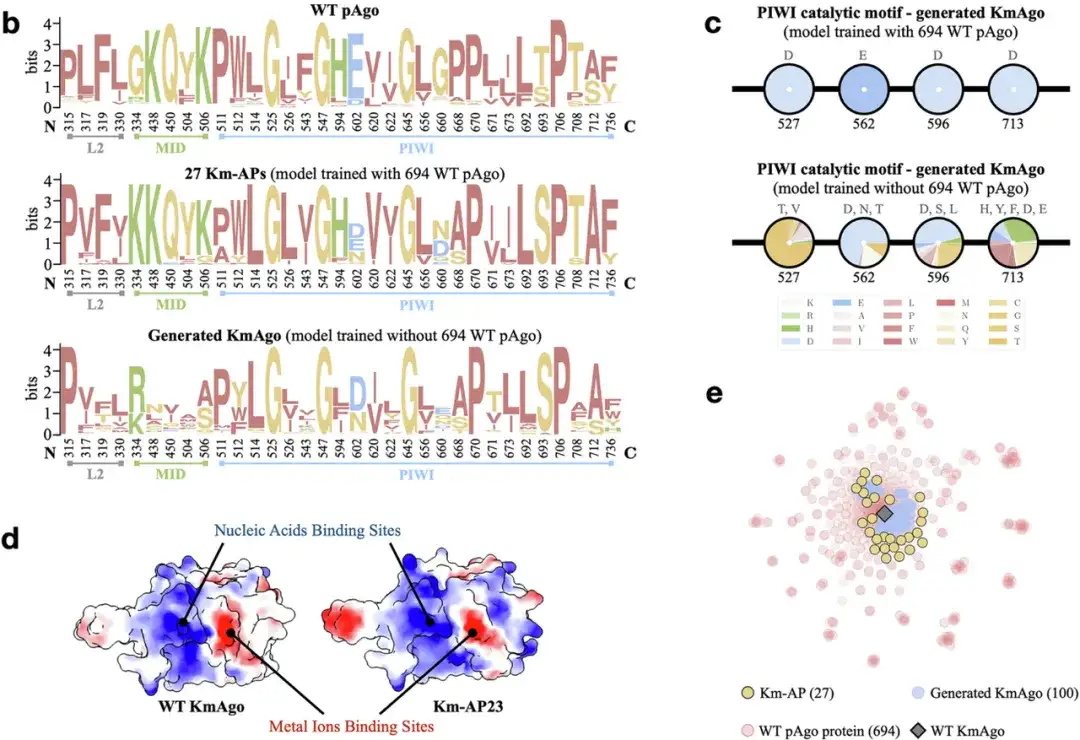
It is worth mentioning thatThe training and inference process of CPDiffusion requires almost no expert guidance.It can automatically identify highly conserved regions, thereby introducing more changes in non-conserved regions based on the determination of the functions of the conserved regions, thereby increasing the diversity of the generated sequences.
Through various experimental verifications, as shown in the figure below, the researchers found that in the new sequences generated for KmAgo,All sequences were expressed. Nearly 90% of the new sequences had DNA cleavage activity, and more than 70% sequences showed higher activity than the wild type. Among them, the best performing new KmAgo had an activity nearly 9 times higher than the wild-type KmAgo. In addition, compared with the wild-type KmAgo, the thermal stability of some Km-APs was also enhanced.
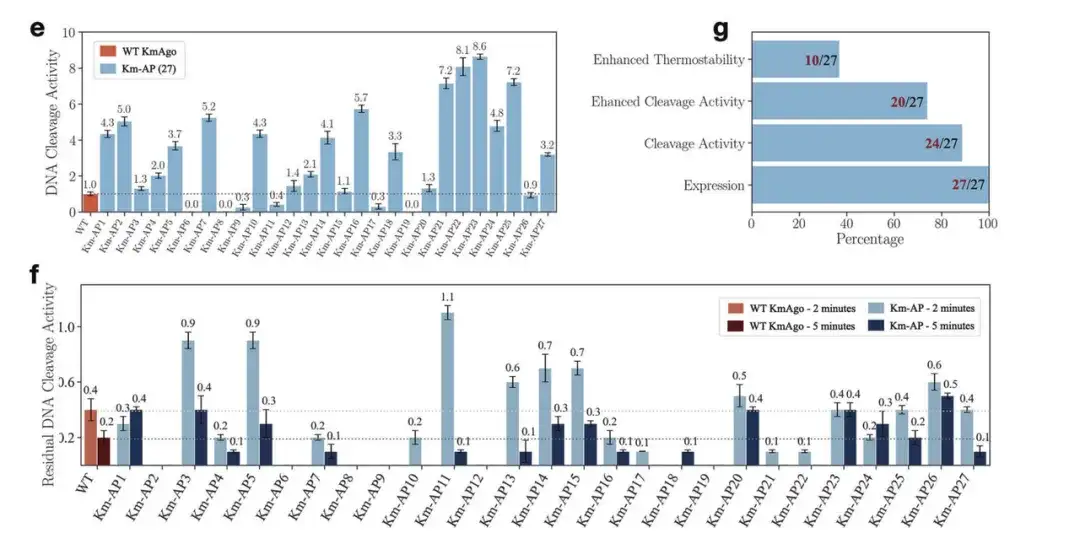
e: DNA cleavage activity of 27 Km-APs at 37 °C
g: The number of proteins playing different roles in 27 Km-APs
f: DNA cleavage activities of WT KmAgo and 27 Km-APs after incubation at 42°C for 2 and 5 min.
As shown in the figure below,Of the 15 new sequences generated for PfAgo, all were able to express and exhibit single-stranded DNA cleavage activity. The best performing new PfAgo not only lowered the melting temperature of wild-type PfAgo from about 100°C to about 50°C, but also had a single-stranded DNA cleavage activity at 45°C that was twice that of wild-type PfAgo at 95°C, and 11 times that of wild-type KmAgo at medium temperatures.
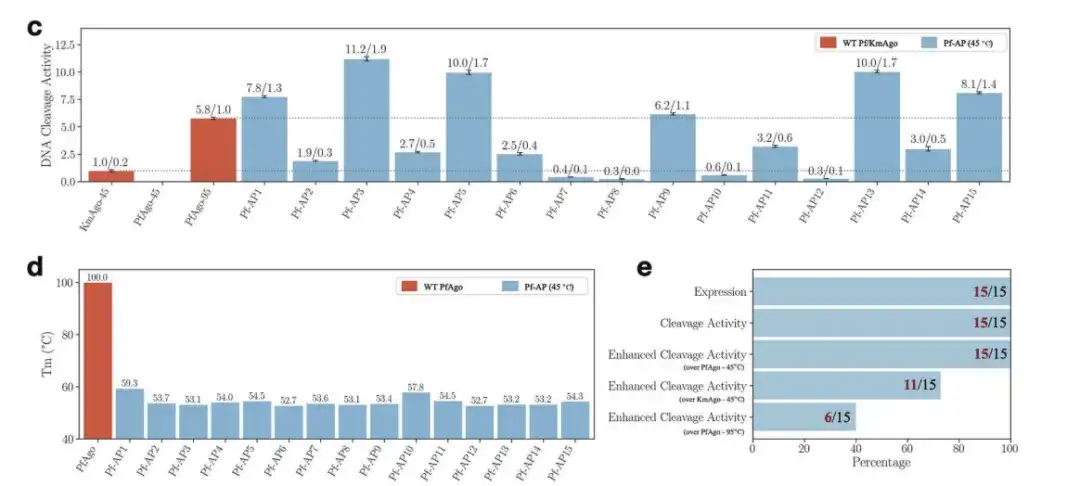
c: DNA cleavage activity of 15 Pf-APs at 45 °C
d: Melting temperature of WT PfAgo and Pf-AP
e: The number of sequences that play different roles among the 15 Pf-APs
In summary, CPDiffusion can be used as a powerful new protein sequence design tool that can automatically learn from wild-type functional proteins and design more powerful complex protein sequences, enriching the existing protein database and bringing more possibilities to protein engineering design.
AI reshapes the future of protein engineering
Using AI to decode the secrets of proteins is of key significance to the digitalization of life science research. In this race to explore the essence of life, Chinese research teams are making continuous progress and making contributions. As one of the outstanding representatives in this field,Professor Hong Liang, the corresponding author of this study, and his research team have long been focusing on AI protein-directed modification and assisted drug design.The specific research contents include but are not limited to protein structure prediction and optimization, protein-directed modification and design, auxiliary drug design and optimization, etc. The team has achieved fruitful results. So far, a total of 77 papers have been published, many of which have topped the Nature journal.
Home page of Professor Hong Liang's research group:
https://ins.sjtu.edu.cn/people/lhong/index.html
Since 2021, Professor Hong Liang's team has tried to apply AI to the protein field, for example,Build proprietary models in the field of protein engineering to design sequences for function from end to end.They have collaborated with researcher Tan Pan from the Shanghai Artificial Intelligence Laboratory to propose a fine-tuning training method FSFP based on the protein pre-training model. This method can efficiently train the protein pre-training model using only 20 random wet experimental data, greatly improving the model's single-point mutation prediction positivity rate. It can be used for small-sample learning of protein adaptability and has shown great potential in practical applications.
Professor Hong Liang's team also developed a microenvironment-aware graph neural network called ProtLGN.It can learn and predict beneficial amino acid mutation sites from protein three-dimensional structures, and guide the design of single-site mutations and multi-site mutations with different functions. Experimental results show that more than 40% ProtLGN designed single-site mutant proteins are superior to their wild-type counterparts.
More details: Without experimental data to guide directed protein evolution, the research group of Hong Liang from Shanghai Jiaotong University published the microenvironment-aware graph neural network ProtLGN
In addition, they introduced a simple, efficient and scalable adapter SES-Adapter,Combining protein language model embeddings with structure sequence embeddings to create structure-aware representations can significantly enhance the performance of protein language models.
The above research demonstrates the powerful potential of deep learning in protein design. There is no doubt that with the further application of deep learning technology in the protein field, protein engineering research will usher in a broader space for development.
References:
https://mp.weixin.qq.com/s/a4gsV4yjzKnW4u6Vtl8LiQ
https://ins.sjtu.edu.cn/article