The World's First! Feng Jianfeng's Team at Fudan University Developed a Digital Twin Brain Platform With 86 Billion Neurons
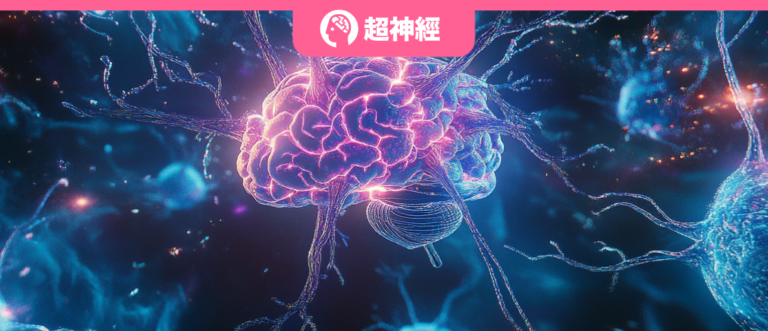
The human brain is one of the most complex and mysterious information processing systems in nature. It weighs only about 1.4 kilograms, accounting for only 2% of the body's weight, but miraculously consumes about 20% of oxygen and blood in the human body.In this sophisticated network, approximately 86 billion neurons are intertwined with each other, forming more than 100 trillion synaptic connections, constituting a complex information transmission and processing system.It is this system that gives humans the ability to think, feel, remember, learn, create and act, and creates different personalities, characters and behaviors.
In order to crack the mystery of the human brain, countries around the world are using computational neuroscience technology to conduct brain science research. In particular, with the advent of digital twin brains, a new perspective and method is provided to solve this problem. It uses and draws on digital twin technology, builds a digital copy of the biological brain through reverse engineering technology, and "decodes" the brain's information processing and neural coding principles, which can achieve major breakthroughs from structural brain simulation to functional brain simulation.
Recently, Professor Feng Jianfeng's team at the Institute of Brain-Inspired Intelligence Science and Technology at Fudan University released the Digital Twin Brain (DTB) platform, which is the world's first full-human brain-scale brain simulation platform developed based on data assimilation methods, with 86 billion neurons and one trillion synapses.The study found that the closer the digital twin brain is to the human brain in scale and structure, the more it will gradually exhibit critical phenomena and similar cognitive functions observed in the human brain.
The study was published in the internationally renowned journal National Science Review (NSR) under the title "Imitating and exploring human brain's resting and task-performing states via resembling brain computing: scaling and architecture".This article is also included as the cover article in NSR's special topic "Human Brain Computing and Brain-like Intelligence".
Research highlights:
* This study provides a quantitative framework for digital twin brains, which can be used to discover the relationship between brain structure and function and to digitally simulate and study different cognitive, medical and injury approaches.
* The study established a brain-wide spiking neuronal network containing up to 20 billion neurons and a data-constrained structure that is unique in terms of scale and multimodal structural constraints.
* The study used data assimilation methods to demonstrate their effectiveness in estimating “large” models by fitting BOLD signals in resting and moving states.

Paper address:
https://doi.org/10.1093/nsr/nwae080
The open source project "awesome-ai4s" brings together more than 100 AI4S paper interpretations and provides massive data sets and tools:
https://github.com/hyperai/awesome-ai4s
Biological data acquisition and preprocessing: Integrating multimodal neuroimaging data into the DTB model
The study used 3 Tesla MRI technology to conduct a comprehensive multimodal MRI scan on a single subject.
First, the study used a rapid gradient echo sequence to capture high-resolution T1-weighted images (T1w). Subsequently, the study collected multi-shell diffusion-weighted imaging (DWI) and functional magnetic resonance imaging (fMRI) data through a gradient echo planar imaging (EPI) sequence. These data were used to analyze the voxel-based morphology (VBM), structural connectivity, and blood oxygen level-dependent (BOLD) signals of gray matter, respectively.
In the data preprocessing stage, the study performed a detailed data cleaning process to efficiently integrate these multimodal neuroimaging data into the dynamic topological base model (DTB). Ultimately, the study constructed a cortico-subcortical model containing 16,043 voxels, providing a new perspective for in-depth understanding of brain structure and function.
The DTB model samples up to 20 billion neurons, covering 16,043 voxels and 374 regions.
The neural network model under the DTB process can flexibly adjust the number of neurons and synaptic connectivity.As shown in Figure A below, the number of voxel neurons is proportional to the VBM gray matter volume, the synaptic density is proportional to the PET SV2A data, and the number of excitatory synaptic connections is proportional to the DWI trajectory density. In particular, each cortical voxel simulates a layered structure from L2/3 to L6, and neurons between each layer are connected by synapses. As shown in Figure B below, the model can sample up to 20 billion neurons, covering 16,043 voxels and 374 regions.

As shown in Figure C above, the neurons in the model are represented by the LIF model, the background current is driven by the Ornstein-Uhlenbeck (OU) process, and the simulated BOLD signal is obtained using the Balloon-Windkessel model. In some areas, neurons can be injected with external currents of Gamma distribution, and then the experimental BOLD signals of the same subject at rest and during the task are collected by using the Vw-dHMDA method based on sampling currents (recording signals) from the estimated hyperparameters. Finally, the performance of this statistical inference can be evaluated by comparing the time course similarity of the simulated data (simulated output) with the BOLD signal and the functional connectivity between regions.
To investigate resting-state scaling, we first assimilated the resting state cortico-subcortical model by fitting the static BOLD signal of the thalamic region and measured the similarity of the model to the biological data.
As shown in Figure A below, the average Pearson correlation coefficient (PCC) between the simulated and experimental BOLD signals across all voxels in the thalamus is 0.977 (left) and 0.981 (right). The study then measured the similarity between the assimilated model and its biological counterpart by calculating the PCC between the static BOLD signals of the assimilated model and real brain voxels, and the similarity of the regional functional connectivity (FC) matrix calculated by measuring the Frobenius norm (F-norm) of the assimilated and biological static BOLD signals.

In this way, the cortico-subcortical model constructed by this study contains 20 billion neurons with an average synaptic connectivity of 100, which is significantly similar to the resting state fMRI data. As shown in Figure B below, the average PCC of the BOLD signal of all voxels is 0.624, and as shown in Figure C below, the PCC of the simulated and actual FC matrices is 0.551, and the F-norm distance is 0.271.
The more similar the digital twin brain is to the real brain in terms of scale and connectivity
This study analyzed the impact of the number of neurons and average synaptic connectivity on the similarity between the model and biological data.The results are shown in Figure D below. When the average synaptic connectivity is 100, as the number of neurons increases, the similarity between the integrated static BOLD signal and the biological data increases, the consistency between the FC matrices increases, and the F-norm distance decreases.

As shown in Figure F below, when the number of neurons is 10 billion, the PCCs of the simulated and biological static BOLD signals first increase and then stabilize as the average synaptic connectivity increases, the PCCs of the FC matrix increases, and the F-norm distance decreases.

As shown in Figure E below, the collapse criticality analysis shows that when the number of neurons increases to 5 billion, the simulated static BOLD signal is closer to the critical point, and the collapse duration and size follow a power-law distribution.

In addition to scales, another important feature of the computational model is its reliance on DWI-based neuroanatomy.To demonstrate the impact of this dependence, the study performed a rewiring process on a neural network, setting the number of neurons to 1 billion, the average synaptic connectivity to 100, and randomly selecting connections to local neighborhoods with probability P.
By changing the P value from 0 to 1, as shown in Figure A below, the artificial voxel-level architecture gradually changes from the architecture based on the original DWI data to the k-nearest neighbor algorithm. As shown in Figure B below, the correlation of the voxel-level BOLD time course between the model and the biological data and the correlation of the FC matrix decreases with increasing P, while the F-norm distance of the FC matrix between the model and the biological data increases with increasing P. Therefore, in the resting state, this reconnection destroys the similarity between the model and the real brain.

To further investigate the impact of the interoceptive circuit on the resting state, as shown in Figure C below, the study used the hippocampus, insula, ACC, vmPFC/sgACC, and thalamus as the interoceptive "input" regions to which the voxel-level BOLD signals were fitted. The results are shown in Figure D below. By using a two-sample t-test, the study found that the similarity between the assimilated static BOLD signal and the biological data was significantly improved when the interoceptive regions were used as input.

As shown in Figure E below, the study calculated the five average activities by inputting the time series of current assimilation hyperparameters, with the highest spectral peak at 0.02-0.025 Hz and several lower peaks at about 0.02-0.08 Hz. As shown in Figure F below, the study conducted conditional Granger causal analysis on the average current hyperparameter sequence and found that there was a relatively strong causal relationship from the thalamus to the ACC, from the thalamus to the insula, from the vmPFC/sgACC to the ACC, and from the thalamus to the hippocampus.

To analyze the impact of neuronal and synaptic scale on the similarity between the assimilation model and its biological counterpart, we first fitted the voxel BOLD signals of the primary auditory cortex (A1), the sensory "input" area, and thenAn assimilation model is established by estimating the hyperparameters of the Gamma distribution of the input current received by the neurons in this area.
As shown in Figures AC below, the model contains 20 billion neurons with an average connectivity of 100. The average PCC across all voxels between the assimilated and biological BOLD signals is 0.570, and the correlation between the predicted and experimentally evaluated numbers is significant.

When studying neural network models with different numbers of neurons and average synaptic connectivity, as shown in Figure DE below, the study found that the similarity of the simulated signal to the biological data increased with the increase in the number of neurons and average synaptic connectivity. When performing reconnection disruption similar to the static model, the study found that the correlation between the evaluation scores and the voxel-level BOLD time course between the model predictions and the biological counterparts increased with the increase of P.

The cortico-subcortical model also opens up new possibilities for certain “digital impairment” operations.As shown in Figure A below, the study removed synaptic connections from the primary visual area (V1) to the basic visual pathway in the dorsal and ventral visual pathways. The study showed that this operation did not affect the similarity of the model to the biological data in the cortico-subcortical area, as shown in Figure B below, confirming the importance of the visual pathway to the memory and learning functions of the hippocampus. As shown in Figure C below, when the connection from V1 to the dorsal or ventral pathways was removed, this operation significantly reduced the correlation between the hippocampal BOLD signal and the biological data. In contrast, removing the connection from V1 to the motor area had less effect on the hippocampus.

Feng Jianfeng has been studying brain science using mathematical methods for 40 years
As the first dean of the Institute of Brain-Inspired Intelligence Science and Technology at Fudan University, Feng Jianfeng is also the chief professor of the Shanghai Center for Mathematics and the dean of the School of Big Data at Fudan University.Although he has made great achievements in the field of brain science, it is surprising that Feng Jianfeng originally graduated from the Department of Mathematics, which laid the foundation for his later research path.
In 1981, Feng Jianfeng was admitted to the Department of Mathematics at Peking University. Although he initially had lofty ideals for mathematical research, Feng Jianfeng was soon attracted to the field of applied mathematics. From his sophomore year, Feng Jianfeng began to audit courses in the Department of Biology. From then on, Feng Jianfeng always insisted on using mathematical methods to study brain science. When he wrote his doctoral thesis, he had already begun to apply the theory of random processes to the study of neural networks.
From officially joining Fudan University in 2008 to being hired as the first dean of the Institute of Brain-Inspired Intelligence Science and Technology in 2015, Feng Jianfeng has been working tirelessly to unlock the mysteries of the brain in the past decade. He used the mathematical theory of systematic development of neural computing to solve a class of optimal stochastic control problems and became a well-known expert in the field of international bioinformatics.
In 2018, Feng Jianfeng's team built a digital brain composed of 70 million spiking neurons for the first time. Through mathematical algorithms, the team achieved a precise computational simulation of the functions of hundreds of billions of neurons in the human brain.Through computational simulation, the working mechanisms of brain perception, learning and memory, emotional decision-making and information processing are further analyzed, providing experimental and theoretical basis for original breakthroughs in artificial intelligence. By the end of 2021, the number of neurons in the digital brain will be comparable to that of a real human brain. Surprisingly, the prototype of this digital brain is Feng Jianfeng himself.
It is based on this spirit of daring exploration in scientific research and its outstanding achievements in computational psychiatry and digital twin brain.Jianfeng Feng was awarded the 2023 Humboldt Research Award.The award is specifically given to outstanding scholars who have made outstanding achievements in basic research, theoretical innovation, and disciplinary leadership, and are expected to continue to make cutting-edge achievements in the future. The number of winners each year does not exceed 100.
Under the leadership of Feng Jianfeng, the Institute of Brain-Inspired Intelligence Science and Technology at Fudan University now has a faculty of 120 people, including multiple research teams in cognitive neuroscience, computational systems biology, artificial intelligence algorithms, and whole-brain computing.He has published nearly 100 papers as the first or corresponding author in high-level journals such as Nature Medicine and Nature Human Behavior. His achievements have been selected for "China's Important Medical Advances in 2023". He has won many awards at home and abroad, including the Silver Award at the Geneva International Invention Exhibition and the SAIL Award at the World Artificial Intelligence Conference. He took the lead in establishing the "Young Scholars Alliance of Brain and Intelligence Science" and so on.
at present,Fudan University's Institute of Brain-Inspired Intelligence Science and Technology is relying on two cutting-edge experimental technology platforms, the Zhangjiang International Brain Imaging Center and the Zhangjiang International Brain Bank, to focus on interdisciplinary scientific research in key areas such as mathematics, brain science and artificial intelligence.In the future, the Institute will continue to stand at the forefront of brain science and brain-like research in the world, committed to promoting the development of the "first brain-like intelligence", and continuously contribute wisdom and strength to the progress of this field.