Deep Learning Fights Against Marine Red Tide Crisis! Zhejiang University GIS Laboratory Proposes ChloroFormer Model to Provide Early Warning of Marine Algae Outbreaks
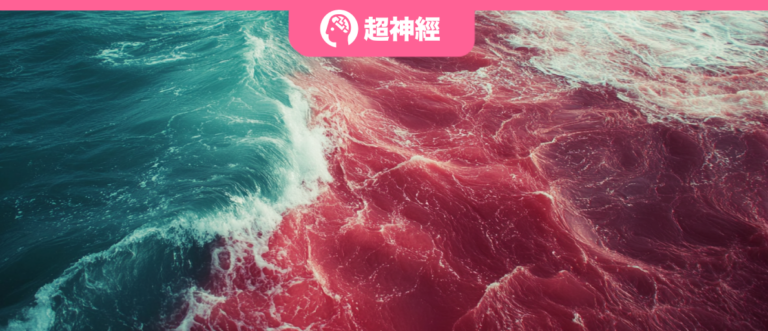
In August 2014, more than 500,000 residents of Toledo, Ohio, suddenly received an emergency notice from the city government - they were not allowed to drink tap water without permission!
Water is the basic supply for human survival. This notice is of great importance and caused a lot of panic after it was issued. The reason is that Lake Erie in the United States has experienced a large-scale outbreak of blue algae, with billions of single-celled algae covering the surface of the water. Two local water treatment companies have detected that the toxins accumulated in the lake have reached a dangerous level and the water quality is no longer suitable for residents to drink.
This ecological abnormality caused by the outbreak of marine algae under specific conditions is what people often call red tide, also known as harmful algal blooms (HABs).Experts say that harmful algal blooms pose a serious threat to the ecological balance of the ocean, the local economy and human health. Specifically, the large number of algae gathered on the surface of the sea can endanger the survival of deep-sea creatures by blocking sunlight and reducing water transparency. The mucus secreted by these organisms can also adhere to the gills of fish, causing them to have difficulty breathing or even suffocate to death. Furthermore, drinking water sources are polluted, and the toxins caused by red tide organisms can be transmitted to the human body through the food chain, and human health is also seriously affected.

Image source: Qianzhan.com
This shows that it is crucial to observe, warn and prevent harmful algal blooms.Generally speaking, the concentration of chlorophyll a (chl-a) can be used as a key indicator to evaluate whether HABs are rampant.Its accurate prediction will directly affect the subsequent prevention and control strategies. At present, machine learning has made significant progress in predicting chl-a concentrations, however, it still has shortcomings in effectively modeling complex dynamic time patterns and dealing with data noise and unreliability.
In response to this, researchers from the GIS Laboratory of Zhejiang University proposed a new deep learning prediction model, ChloroFormer, which combines Fourier analysis and Transformer neural network and adopts a time series decomposition architecture to effectively improve the accuracy of chl-a concentration prediction.In addition, the researchers conducted experiments in two different coastal research areas. The results showed that the proposed model not only outperformed the other six comparison models in multi-step prediction accuracy, but also maintained its relative advantages under extreme and frequent algal blooms.
The study, titled “Enhanced forecasting of chlorophyll-a concentration in coastal waters through integration of fourier analysis and transformer networks,” was published in Water Research.
Research highlights:
* The deep learning model ChloroFormer outperforms the baseline in both short-term and medium-term predictions of chlorophyll a.
* ChloroFormer performs well in predicting the chlorophyll a peak with high accuracy and a high goodness of fit to the observed data distribution.

Paper address:
https://doi.org/10.1016/j.watres.2024.122160
Follow the official account and reply "Harmful Algal Bloom" to get the full PDF
The open source project "awesome-ai4s" brings together more than 100 AI4S paper interpretations and provides massive data sets and tools:
https://github.com/hyperai/awesome-ai4s
Dataset: Taking Taizhou Sea Area and Ala Wai Port as an example
This study selected the Taizhou waters of Zhejiang Province and Ala Wai Port of Oahu Island, Hawaii as the research areas.The two places have different HAB conditions. In Taizhou, due to over-intensive use of the waters for economic development, a large amount of waste pollutants have poured in, and the deterioration of seawater quality has led to frequent red tides. Ala Wai Harbor is a popular place for boating and yachting, with various human activities such as surfing, boating, sailing, swimming and fishing, making HABs prone to occur.
Specifically, the Taizhou Sea Area data TZ02 includes chlorophyll-a (chl-a) concentrations from May 30, 2014 to July 29, 2021, monitored at hourly intervals. The Ala Wai Port data NSS002 covers chl-a concentrations from March 1, 2013 to July 26, 2018, collected by water quality monitoring sensors at 4-minute intervals and averaged over 15 time points. Furthermore, the datasets were standardized and log-transformed to reduce the data range and improve the prediction accuracy of the model.

Model architecture: coexistence of periodicity and trend, multi-step prediction of chl-a concentration
This study proposes a deep learning model ChloroFormer to predict chl-a concentration. The model framework is shown in the figure below:first,The original time series is decomposed into periodic lnit and trend lnit components by moving average method, and modeling is performed separately.

(a) ChloroFormer network architecture, the two paths are the periodic and trend sequence components
(b) Internal structure of the frequency attention module
Secondly,For the trend component, the researchers used the Transformer neural network and its self-attention mechanism to capture long-range dependencies. For the periodic component, the researchers designed an attention mechanism based on Fourier analysis on the basis of the Transformer network, including frequency filters and frequency attention mechanisms, which can more effectively capture periodic patterns in frequency.
at last,The researchers combined the two modeled sub-series and directly mapped them to multi-step prediction results through linear projection.
Experimental conclusion: Highly non-stationary temporal dynamics of chl-a concentration can be predicted
The researchers used the ChloroFormer model to predict the short-term and medium-term chl-a concentrations. The results are shown in the figure below.In short-term prediction, ChloroFormer has higher accuracy than other compared models in both study areas, and the error rate is significantly reduced.

(a) Comparison of MAE, RMSE and CORR metrics at the TZ02 monitoring station
(b) Comparison of three metrics at the NSS002 monitoring site
As shown in the figure below,In the mid-term forecast, ChloroFormer still outperforms other models, although the overall prediction accuracy decreases.

(a) Comparison of MAE, RMSE and CORR metrics at the TZ02 monitoring station
(b) Comparison of three metrics at the NSS002 monitoring site
In addition, the researchers compared the results of different models predicting peak chl-a concentrations under frequent algal bloom conditions. As shown in the figure below, ChloroFormer has a higher accuracy in short-term predictions, but as the prediction time increases, ChloroFormer's accuracy in long-term predictions decreases. Overall, compared with other models,ChloroFormer performs well in capturing time series trends and accurately matching extreme points, which can provide important information for algal bloom early warning.

Finally, this study also proved that the prediction results of ChloroFormer are highly consistent with the actual observed data distribution through the Kolmogorov-Smirnov test.This shows that the ChloroFormer model is robust and can accurately reflect the data characteristics.On the other hand, by analyzing the distribution of attention weights, the researchers also found that ChloroFormer can capture key temporal patterns in different datasets.
In summary, this study proposed a deep learning model, ChloroFormer, which integrated Fourier analysis technology and Transformer network to improve the prediction accuracy of chl-a concentration with highly non-stationary time dynamics, and is a reliable and cost-effective tool for harmful algal bloom prediction in environmental management.
Building a blue future with the power of science and technology
The ocean is the largest life support system on Earth. Protecting marine biodiversity is protecting our common future. However, with the continuous expansion of human activities, crises such as climate change, overfishing, habitat destruction and pollution continue to emerge, and the marine ecosystem is facing severe challenges. In response, researchers at home and abroad have responded and look forward to building a blue line of defense together.
In recent years, with the accumulation of data and the development of new technologies such as deep learning, innovative research in the field of marine protection has continued to emerge.For example, the Zhejiang University GIS Laboratory research team mentioned in this article has long been committed to exploration and innovation in the field of marine protection.They have combined machine learning with satellite products to conduct in-depth research on the highly dynamic changes in dissolved oxygen in the global ocean and explore the laws and causes of ocean hypoxia.
Specifically, the researchers developed a global surface dissolved oxygen comprehensive modeling framework DOsurface-Pred Framework, and generated a large-scale surface dissolved oxygen dataset SSDO based on the framework. The results show that even in the oxygen-rich sea surface area, the dissolved oxygen content has shown a downward trend, and this decline is mainly attributed to changes in sea surface temperature (SST).
In addition, the research team of the University of Montpellier in France has also combined the random forest model and artificial neural network.Extinction risk prediction for 4,992 marine fish species with insufficient or unassessed data. The results show that the extinction threat to marine fish is far greater than previously generally recognized. In the future, we need to pay more attention to species with insufficient data and key hotspots to ensure that subsequent conservation efforts can be targeted and hit the mark. Through this study, scientists not only filled the data gap in the field of marine fish conservation, but also provided a new perspective for adjusting global conservation priorities.
These cutting-edge research results are like stars, gathering into a brilliant galaxy to protect the ocean. In the future, we have reason to believe that with the participation of more scientific research teams, government agencies, non-governmental organizations and the public, this battle to protect the marine ecology will become more powerful, and we will definitely be able to build a solid line of defense for the marine ecology.
References:
https://dialogue.earth/zh/3/42441/
https://m.thepaper.cn/newsDetail_forward_4890474