DeepMind's New Results Are Criticized As Advertisements? AlphaProteo Can Efficiently Design Target Protein Binders With 300 Times Higher Affinity
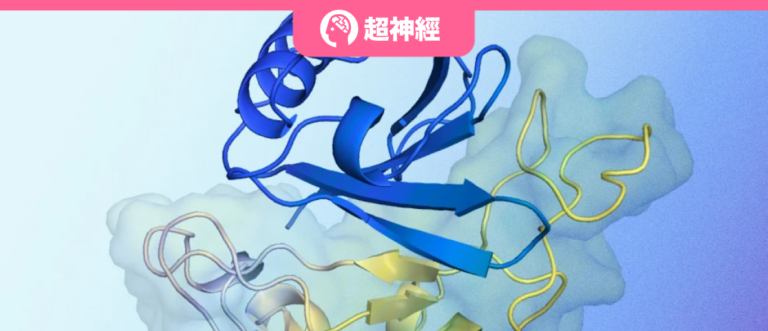
AlphaFold is far ahead in the field of protein prediction, and AlphaFold 3 has broken through the limitations and achieved the prediction of all life molecules. When it was released, it was said that AlphaFold 3's structured prediction and generation will accelerate the development of AIDD (Artificial Intelligence-driven Drug Design).
Now, DeepMind has taken another shot at AI drug design by releasing AlphaProteo for new protein design.
This new member of the Alpha family also demonstrated a revolutionary improvement in capabilities. In the seven target proteins tested in the experiment, AlphaProteo not only achieved a higher experimental success rate,The binding affinity is also 3 to 300 times higher than the best existing methods.At the same time, the researchers also applied it to the target protein VEGF-A related to cancer and diabetic complications.A breakthrough was achieved in the use of AI tools in the design of VEGF-A protein conjugates.
One-round medium-throughput screening generates “ready-to-use” protein binders
On September 5th local time, DeepMind researchers proposed AlphaProteo, a machine learning model for protein design, which can design target protein binders with high success rate, high affinity and no need for complex human intervention. AlphaProteo requires only one round of medium-throughput screening and does not require further optimization to generate “ready-to-use” protein binders for many research applications.
Model structure: Combining generative models with filters to efficiently generate target protein binders
AlphaProteo consists of 2 components, as shown in the figure below.
A generative model (Generator),Trained on the structure and sequence data of the Protein Data Bank (PDB) and the distillation set of AlphaFold predicted structures to learn how molecules bind to each other;A filter,Used to score the generated designs to predict whether the binder can successfully bind to the target protein in the experiment.
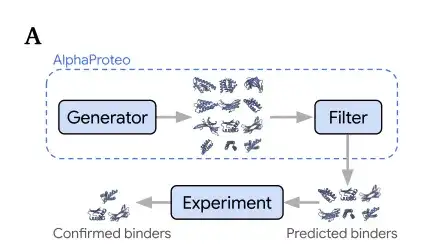
Specifically, in order to design target protein binders, the researchers first input the structure of the target protein and select the "hot spot" residues of the target protein epitope to infer the preferred binding position. The generative model will output the candidate binder structure and sequence for the target protein. The filter will screen these predicted binders into a smaller set before experimental testing. In computer simulation benchmark tests, the generative model performed better than the best existing methods.
Experimental conclusion: Surpassing the best existing solutions, with high success rate and strong affinity
The researchers used AlphaProteo to design binders for eight target proteins with different structures and tested them experimentally. Among them, there are two viral proteins related to infection, BHRF1 and SARS-CoV-2 spike protein receptor binding domain SC2RBD, as well as five proteins related to cancer, inflammation and autoimmune diseases, IL-7Rɑ, PD-L1, TrkA, IL-17A and VEGF-A, and one protein TNF associated with autoimmune diseases such as rheumatoid arthritis.
As shown in the figure below,The seven target protein binders generated by AlphaProteo were able to bind tightly to the target protein when tested experimentally.However, it was unable to design successful binders for the eighth target, TNF.
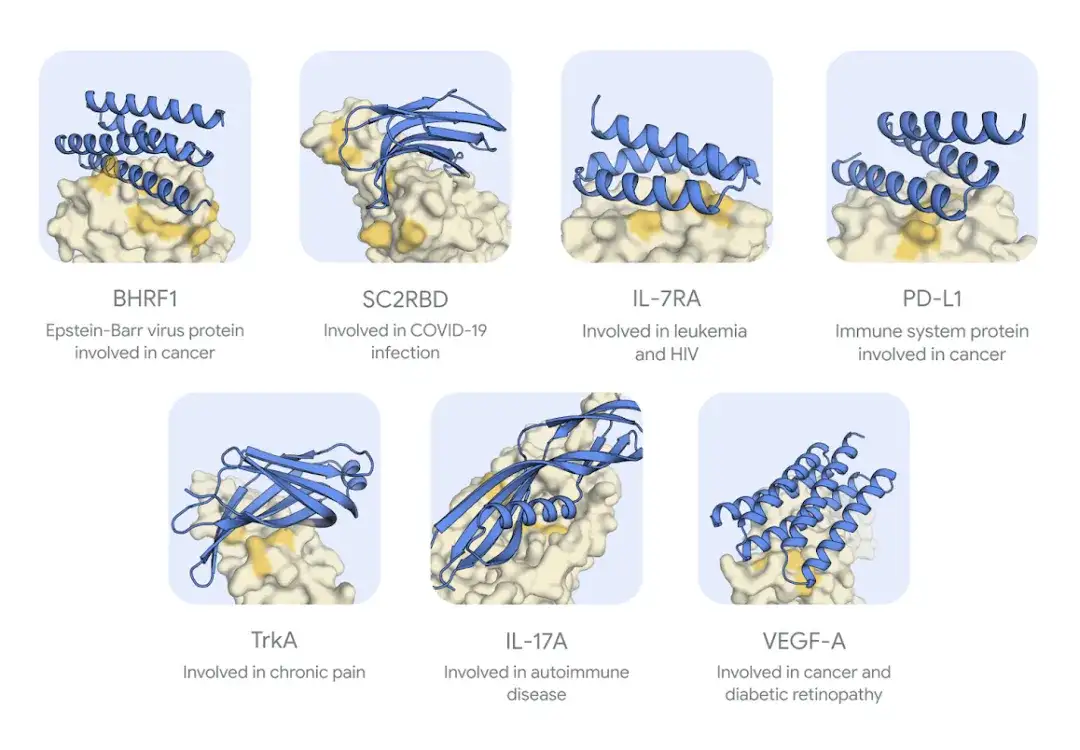
* Blue indicates binder, yellow indicates protein target, dark yellow is expected binding region
Furthermore, as shown in the figure below, the researchers compared AlphaProteo with other design methods to compare the in vitro success rate.In wet lab testing, the 9%-88% candidate binders were able to successfully bind to the target protein at 5-100 times higher levels than other methods.
Among them, for the viral protein BHRF1, the candidate molecules generated by AlphaProteo have a binding success rate of 88% with the target protein, and the binding strength is 10 times that of the existing best design method; for the protein TrkA, the new binder is stronger than the best designed binder that has been optimized for this protein after multiple rounds of experiments.
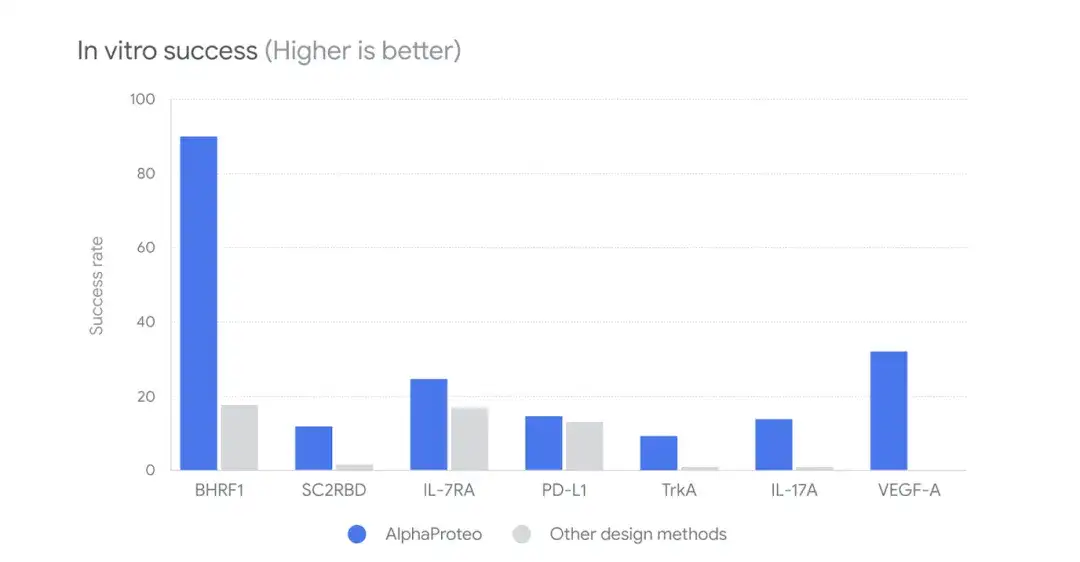
In addition, the researchers explored the best affinity of AlphaProteo-designed binders to target proteins compared with other design methods without experimental optimization.AlphaProteo-generated binders have higher binding affinities, approximately 3-300 times higher, than binders designed using the best available methods.
* The greater the affinity, the tighter the binding
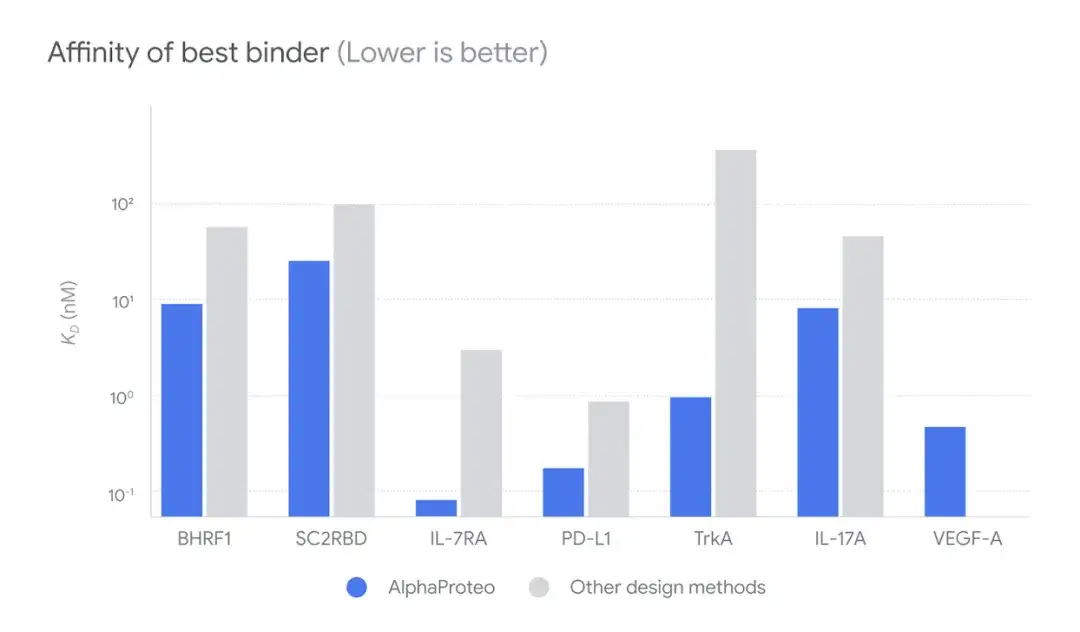
The researchers further explored the similarities between stronger binders of SC2RBD and VEGF-A protein and those designed by AlphaProteo and found thatThe binding interactions of these stronger binders were similar to those predicted by AlphaProteo, which indicates the reliability of AlphaProteo.
In addition, the researchers also confirmed that these binders have actual biological functions. For example, some SC2RBD binders were shown to prevent SARS-CoV-2 and its variants from infecting cells. More importantly, AlphaProteo has a wide range of applicability and does not require high-throughput screening or affinity optimization experiments, which can greatly reduce the initial experimental time for designing protein binders.
Collaborate with Europe's largest biomedical research laboratory
Among the first experiments to test the new protein design system AlphaProteo are three teams from the Francis Crick Institute.
The Francis Crick Institute is located in London, UK. It is currently the largest biomedical research laboratory in Europe and a research center in the UK. It was jointly established by the National Institute for Medical Research (NIMR) and Cancer Research UK at a cost of approximately £700 million.
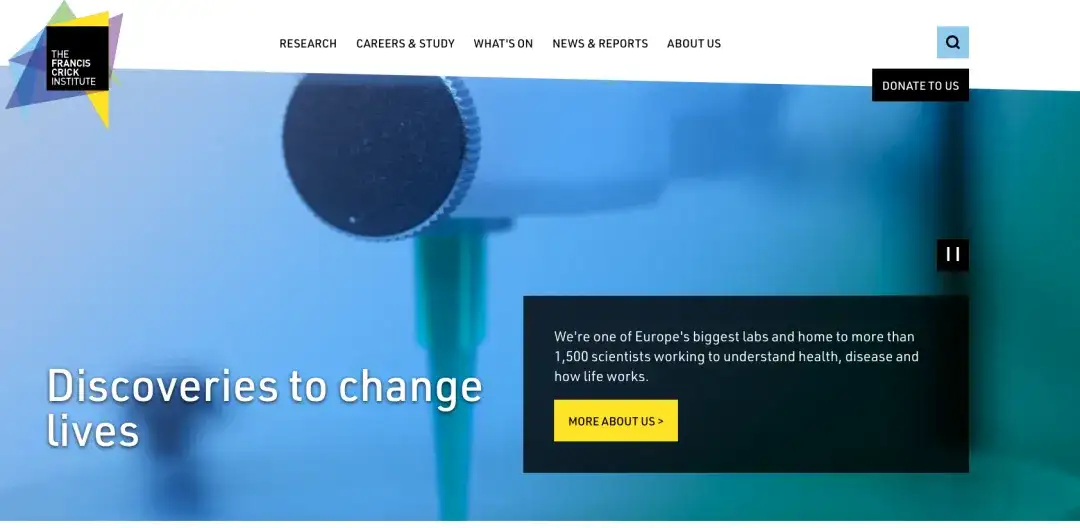
The Crick Institute currently has more than 2,000 researchers and more than 100 research groups. In addition, the institute is a unique partnership established by six of the world's leading biomedical research institutions, including the Medical Research Council, Cancer Research UK, the Wellcome Trust, University College London, Imperial College London and King's College London.
Francis Crick Institute official website address:
* https://www.crick.ac.uk/
Deepmind's latest achievements have received mixed reviews
Research significance vs. open source
Although AlphaProteo's high value in actual research is obvious to all, many netizens are more concerned about whether this tool is open source. One netizen said, "Is it open source? If not, it would be too boring."
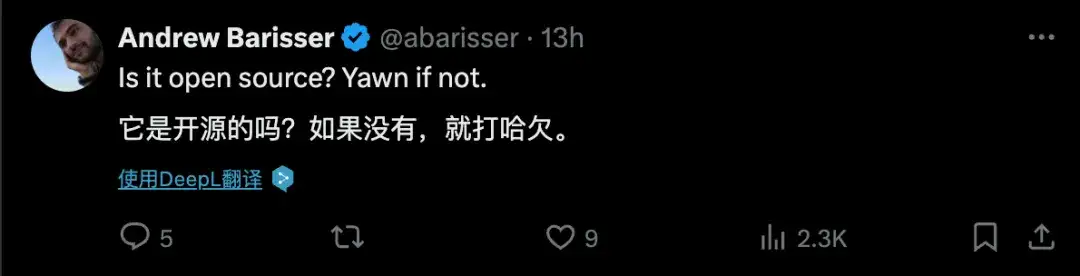
Some other netizens said,AlphaProteo is more important for saving lives than whether it is open source.And expressed optimism about its development potential.
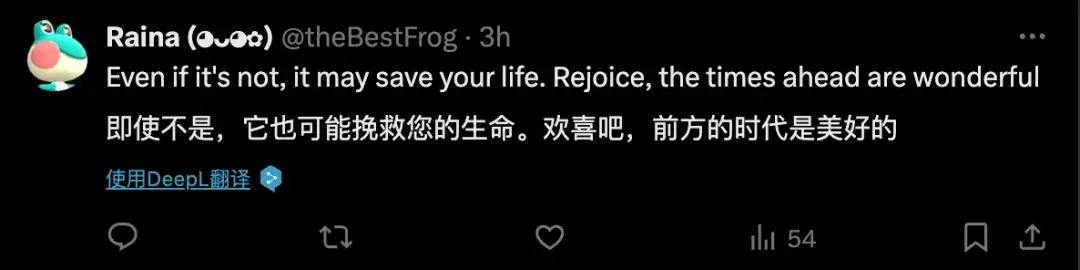
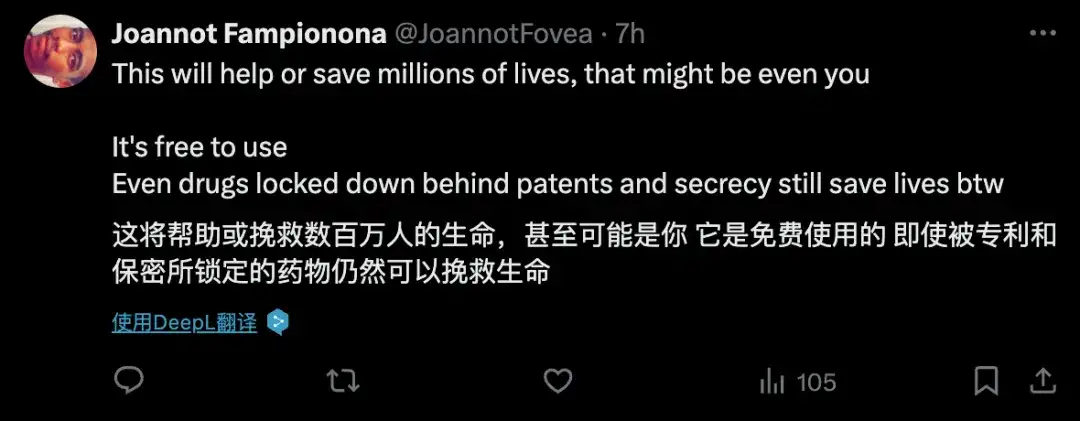
The methodological details are too few, it looks more like an advertisement than a paper
The DeepMind team wrote a paper for the model, but it contained very few details about the model's methods, or even almost no details. A scientist from a leading global pharmaceutical and healthcare company posted three messages in a row saying that "the method details in this white paper are too few", "it feels more like an advertisement than a proper publication", and questioned the same legend indicators in the paper.
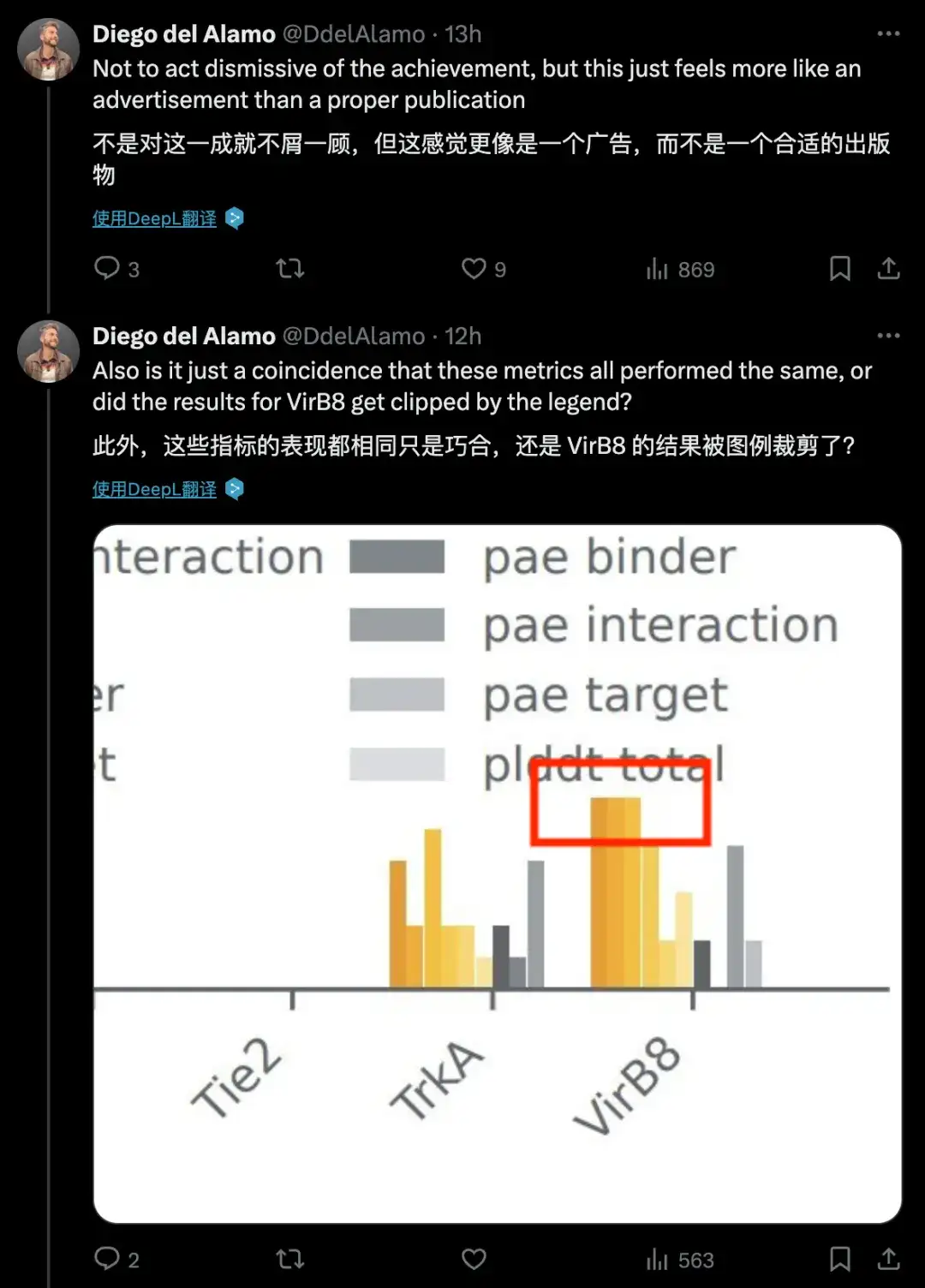
Another biology PhD from MIT not only reiterated the significance of open source science, but also criticized DeepMind's move, "DeepMind has gone too far, and I really want to know how it works."
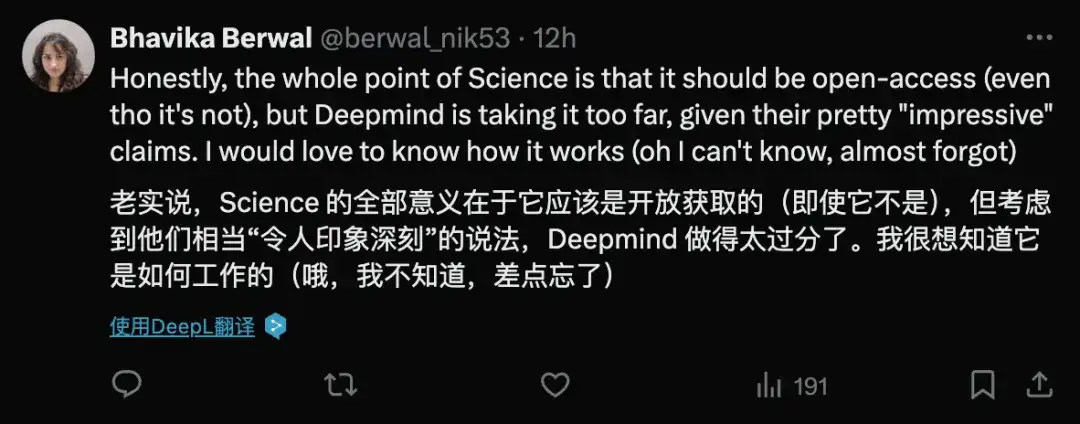
DeepMind opens up a new battlefield and complements AlphaFold
DeepMind's "upgrade and fight monsters" for the biopharmaceutical industry has opened up a new battlefield. Can it become a shot in the arm to accelerate the implementation of AI drug design?
As we all know, AlphaFold 3 is already able to predict the structures and interactions of all life molecules (proteins, DNA, RNA, ligands, etc.) with ultra-high precision. Regarding its future iteration direction, industry insiders predict that there may be improvements in the prediction of kinetic information.The AlphaProteo released this time can, to a certain extent, complement and promote each other with AlphaFold.
on the one hand,Protein design needs to start from function and design new or improved protein structures to achieve specific biological or chemical functions, so it is highly dependent on the understanding of the relationship between protein structure and function.on the other hand,Protein prediction can provide key structural information to guide design. At the same time, the designed proteins can also help improve the prediction model and enhance the prediction accuracy of protein folding, dynamics and other aspects.
DeepMind mentioned in the introduction of AlphaProteo that this innovation may accelerate people's understanding of biological processes and help discover new drugs, the development of biosensors, etc. In the future, the company will work with the scientific community to use AlphaProteo to solve far-reaching biological problems and understand its limitations. At the same time, DeepMind has also been exploring its drug design applications with Isomorphic Labs.
Isomorphic Labs was born out of DeepMind and focuses on the field of AI medicine. Its mission is to use artificial intelligence and machine learning methods to accelerate and improve the drug discovery process in order to find treatments for some of humanity's most devastating diseases.
To some extent, Isomorphic Labs is also DeepMind's tentacles in the field of AI medicine, which can explore the application of its latest achievements in drug design in a targeted manner at the first time. The team also appeared in the research of AlphaFold 3. It is expected that with the support of DeepMind, it can bring good news for the implementation of AI drug design.
Finally, I recommend an academic sharing activity!
The third live broadcast of Meet AI4S invited Zhou Ziyi, a postdoctoral fellow at the Institute of Natural Sciences of Shanghai Jiao Tong University and the Shanghai National Center for Applied Mathematics. On September 25, Dr. Zhou Ziyi will share with you the small sample learning method of protein language model in the form of online live broadcast, and explore new ideas for directed evolution assisted by AI. Click to make an appointment to watch the live broadcast!