Academic Sharing | No Fear of Data Shortage! Shanghai Jiaotong University Postdoctoral Fellow Zhou Ziyi Explains the Small Sample Learning Method FSFP of Protein Language Model
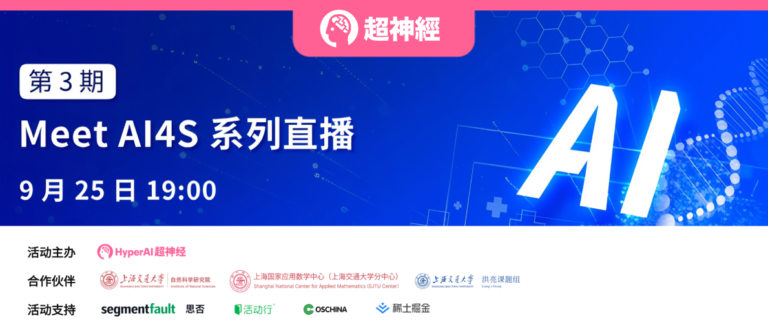
Pre-trained protein language models (PLMs) can learn the distribution features of amino acid sequences in millions of proteins in an unsupervised manner, showing great potential in revealing the implicit relationship between protein sequences and their functions.
In this context, the research group of Professor Hong Liang from the School of Natural Sciences/School of Physics and Astronomy/Zhangjiang Institute for Advanced Studies/School of Pharmacy of Shanghai Jiao Tong University, together with Tan Pan, a young researcher from the Shanghai Artificial Intelligence Laboratory,We developed a small sample learning method for protein language models, which can significantly improve the prediction performance of mutation effects of traditional protein language models using very little wet experimental data.It has shown great potential in practical applications.
In the third episode of the "Meet AI4S" live series, HyperAI was fortunate to invite the first author of the research paper, Zhou Ziyi, a postdoctoral fellow at the Institute of Natural Sciences of Shanghai Jiao Tong University and Shanghai National Center for Applied Mathematics. On September 25, Dr. Zhou Ziyi will further share with everyone the small sample learning method of protein language model in the form of online live broadcast, and explore new ideas for directed evolution assisted by AI.
Click to schedule a live broadcast:
Scan the QR code and remark "AI4S" to join the discussion group↓
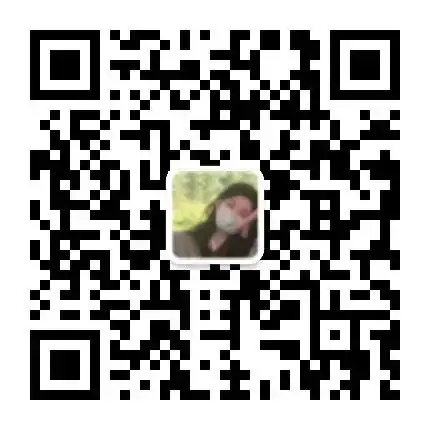
Event Details
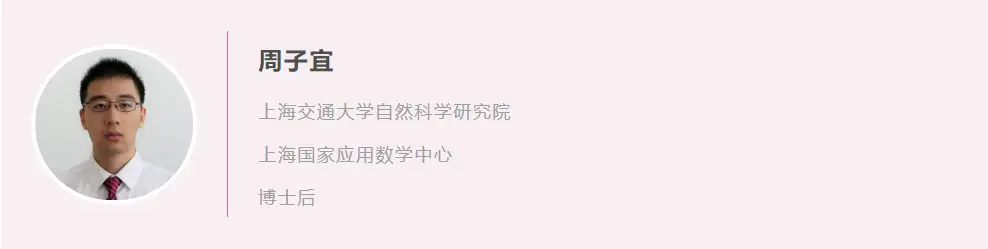
Share the topic
Few-shot learning method for protein language model
Introduction
Protein language model (PLM) has made breakthroughs in protein function prediction, but it often requires a large amount of experimental data fine-tuning to achieve high accuracy. This paper introduces a small sample learning method for PLM, which can significantly improve the mutation effect prediction performance of PLM using only dozens of training samples.
Paper Review
HyperAI has previously interpreted and shared the research paper "Enhancing efficiency of protein language models with minimal wet-lab data through few-shot learning" with Dr. Ziyi Zhou as the first author.
The FSFP method consists of 3 stages:
Build auxiliary tasks for meta-training, train PLMs on the auxiliary tasks, and transfer PLMs to the target task via LTR.
Among them, FSFP uses ListMLE loss to learn to rank mutation fitness. In each training iteration, the predicted ranking of training samples by PLM is corrected to their true ranking. This ranking learning method is applied simultaneously to the internal optimization and transfer learning stages of the meta-training phase.
Dataset acquisition
This study selected the ProteinGym dataset as the benchmark dataset, which contains approximately 1.5 million missense variants from 87 DMS sequencing experiments.
ProteinGym protein mutation dataset download address:
https://go.hyper.ai/6GvFD
FSFP Method Evaluation
* In terms of average performance, PLMs trained by FSFP consistently outperform other baselines on all training data sizes.
* In terms of extrapolation performance evaluation, the Spearman correlation evaluation of FSFP-trained PLMs is superior.
* FSFP was successfully applied to the engineering modification of Phi29 DNA polymerase, significantly improving the positive rate.
Audience benefits:
1. Understand the basic principles of PLM and its application in protein engineering
2. Understand the basic principles of PLM and its application in protein engineering
3. Explore new ideas for directed evolution assisted by AI
Hong Liang's research group at Shanghai Jiao Tong University

The research group of Hong Liang of Shanghai Jiao Tong University is affiliated to the Institute of Natural Sciences of Shanghai Jiao Tong University. The research direction of the research group is mainly AI protein and drug design, molecular biophysics, including:
* Protein-directed modification, enzyme engineering directed evolution, and assisted drug design based on artificial intelligence technology;
* Neutron scattering, synchrotron radiation national large scientific facilities, single molecule fluorescence, molecular dynamics simulation and artificial intelligence algorithms, etc., to study the dynamics of biological macromolecules, biological macromolecule cryopreservation technology and principles.
The research group has achieved fruitful results. To date, they have published 77 research papers, many of which have been published in Nature journals.
Meet AI4S Live Series
HyperAI (hyper.ai) is China's largest search engine in the field of data science. It focuses on the latest scientific research results of AI for Science and tracks academic papers in top journals such as Nature and Science in real time. So far, it has completed the interpretation of more than 100 AI for Science papers.
In addition, we also operate the only AI for Science open source project in China, awesome-ai4s.
Project address:
https://github.com/hyperai/awesome-ai4s
In order to further promote the popularization of AI4S, further reduce the dissemination barriers of scientific research results of academic institutions, and share them with a wider range of industry scholars, technology enthusiasts and industrial units, HyperAI has planned the "Meet AI4S" video column, inviting researchers or related units who are deeply engaged in the field of AI for Science to share their research results and methods in the form of videos, and jointly discuss the opportunities and challenges faced by AI for Science in the process of scientific research progress and promotion and implementation, so as to promote the popularization and dissemination of AI for Science.
We welcome efficient research groups and research institutions to participate in our live broadcast activities! Scan the QR code to add "Neural Star" WeChat for details↓
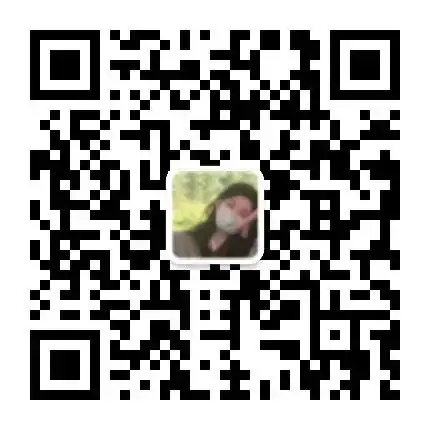