AI Is Adding to the Game, and Super-optics Is Entering a Booming Era
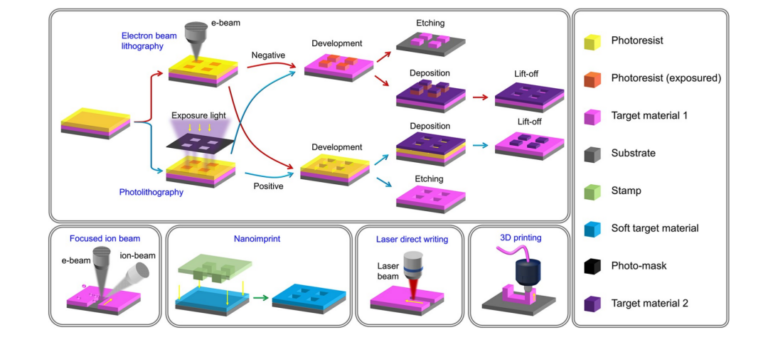
In the world we live in, light plays a core role. Because of the importance and uniqueness of light, scientific giants such as Galileo, Newton, Maxwell, and Einstein have all devoted themselves to the study of light. It can be said that optical research has a long history. However, with the development of technology and the continuous improvement of human needs,Some limitations in optical research have gradually become apparent.
Traditional optical imaging is approaching the physical limit in terms of hardware functions and imaging performance, and can no longer meet application requirements in many fields. In order to meet this challenge, in recent years,A new multidisciplinary field, "computational optical imaging", has emerged.At the beginning of the year, it was selected as one of the top ten technology trends in 2023 by Alibaba Damo Academy.
According to experts, compared with traditional optical imaging, computational optical imaging deeply integrates digitization and information into optical design, integrates software and hardware, and injects new "life" into optical imaging through calculation. Its research covers a wide range, including FlatCAM, super-optical technology, etc. In this regard, Bloomberg published an Opinion article at the end of last year, saying,Computational Optical ImagingMeta Optics is a technology that promises to gain widespread attention this year and be transformative over the next decade.
Then, one of the branches of computational optical imaging isWhat exactly is hyperoptics and why is it developing so fast?If we look into the reasons, we will find that a key factor is naturally indispensable in the process of the so-called integration of digitalization and informatization mentioned above - artificial intelligence (hereinafter referred to as AI).
Next, this article will focus on the paper "Artificial Intelligence in Meta-optics".Starting from the combination of AI and super-optics, the latest research results in related fields are introduced in detail.In the hope of providing some inspiration to scientific researchers.
Overview of Key Concepts in Meta-Optics
In ideal classical optics, the propagation of light in two media is related to the speed of light in the media and the optical properties of the two media, such as refraction and reflection of light.The emergence of meta-materials has changed this optical behavior.
Specifically,The metasurface consists of an array of nanostructures.Also known as meta-atoms, each of them is considered a secondary point light source. When incident light encounters this interface, the nanostructure changes the optical properties of the incident light and re-radiates new electromagnetic waves. By effectively controlling the phase distribution of the metasurface, the wavefront of the incident light can be reconstructed with unique properties and new functions.
Processing hyperoptics is a direct way to connect theoretical design and practical application.At present, processing technology has also been well developed for different purposes, such as subwavelength scale, structural engraving, large area, high aspect ratio, high output, etc.
In this regard,The researchers introducedOptical meta-device processing technology,Among them, the most commonly used processing methods are photolithography, electron beam lithography (EBL), focused ion beam (FIB) lithography, nanoimprinting, laser direct writing and 3D printing. Through these advanced processing methods, super devices can be further applied.

To meet the optical needs, some new and special optical functions of metadevices have been developed. The great advantages of metadevices are their new characteristics, compact size, lighter weight, high efficiency, better performance, broadband operation, lower energy consumption, data reduction and CMOS compatibility, which can be used for large-scale production.Optical metadevices have been well developed in technologies such as beam shaping, abnormal deflection and reflection, polarization control and analysis.
Leveraging AI to make great strides

The horizontal axis represents the year and the vertical axis represents the number of publications per year.
As can be seen from the above figure, the development trends of AI and super-optics are roughly the same, both entering a period of rapid growth around 2012.The researchers specifically analyzed The application of AI in direct and inverse problems in meta-optics, data analysis based on metasurface systems, and intelligent programmable meta-devices.
Surrogate Modeling
Optical property modeling
AI, especially deep learning, provides a direct and efficient breakthrough shortcut for optical simulation. In recent years, AI has achieved remarkable results in proxy modeling. In proxy models, ANNs are often used as approximate predictors of the optical response of meta-atoms. In addition,In certain design tasks, the ANN used for the surrogate model is the optimal solution.
In 2019,Sensong An and Clayton Fowler, postdoctoral fellows in the Department of Materials Science and Engineering at MIT, and others proposedA deep neural network called Predicting NN,Modeling the amplitude and phase responses of all-dielectric meta-atoms in the range 30-60 THz.
As shown in Figure a below, the input of the Predicting NN is the geometric parameters, and the output is the real or virtual projection coefficient. Sensong An and Clayton Fowler et al. developed two DNNs to predict the real and virtual parts respectively. The required amplitude and phase responses are further calculated using the projection coefficients.This indirect operation is due toThe typical metaatom amplitude and phase responses change abruptly near the resonant frequency.

(a) Amplitude and phase prediction of cylindrical metaatom
(b) Amplitude and phase prediction of free-form fully insulating metaatom
(c) Prediction of scattering cross sections of alternate-material-shell nanoparticles
(d) Prediction of the diffraction efficiency of a 16-sided polygonal superatom
(e) Prediction of absorption spectra of free-form surface superatoms using DNN
(f) Absorption spectrum prediction of free-form surface super-atom using CNN and RNN
The prediction performance of ANNs is greatly reduced at resonance due to the hard regression of sharp nonlinearities, so the authors innovatively use different continuous real and virtual parts of the scattering coefficient as prediction targets.The prediction accuracy of cylindrical and "H"-shaped superatoms reached more than 99%, which is 600 times faster than traditional simulations.
In 2020, Sensong An, Clayton Fowler and others proposed a new method to use CNN to characterize the amplitude and phase of meta-atoms in the same working band. The difference is thatThe modeling object is a freeform structure with different material properties, not a simple structure.As shown in Figure b above.
The designed free-form surface includes the 2D pattern image, the lattice size, the thickness of the structure, and the refractive index of the material. The head of the CNN is divided into two input branches. One processes the 2D pattern image and the other processes the index of different attributes. Through downsampling and upsampling procedures, the two branches are recombined into feature maps of matching dimensions. The output is still in the format of the real and imaginary parts of the scattering coefficient.
Compared with previous work, this method uses more training data and provides more power for free-form surface design.Under the same hardware conditions, the prediction speed is 9000 times faster than traditional simulation.This also significantly exceeds previous work.
Performance Evaluation
To evaluate the effectiveness of a proxy model, its accuracy is often compared to traditional simulation tools that solve Maxwell's equations. In general, most proxy models show high fidelity in various optical properties. In addition to acceptable accuracy,Proxy models are orders of magnitude faster than traditional simulations.

(a) Absorption spectrum of free-form surface structure
(b) Amplitude and phase responses of the “H”-shaped metaatom
(c) Forward and backward scattering of the nanorod in TE and TM modes, and internal electric field distribution (top)
(d) Reflectance spectrum and corresponding CD spectrum
(e) Verify the transmission spectrum using measurements of actual process designs
(f) Comparison of computational time between digital simulation and deep learning-based proxy model
To summarize the surrogate modeling with ANNs, the following table lists the interesting information for direct comparison and understanding. From protons to dielectric superatoms, the materials listed in the table cover common metals and dielectrics. The selected references in the table have different modeling responses,Prove that the currentThe proxy model can learn almost all common optical properties from the structural geometry of superatoms.

However, as an approximate solver of Maxwell's equations,The proxy model also has three disadvantages:
* The performance of the proxy model is limited by the construction of the training data. Each model can only operate under specific conditions (such as transmittance, reflectivity, polarization, etc.) and specific working wavelengths.
* The performance of some proxy models will degrade at resonant frequencies.
* The process of generating training data is a labor-intensive and tedious task.
Nevertheless, the proxy model based on ANNs is also many orders of magnitude faster than traditional simulation tools. In addition to its speed, the proxy model has another advantage. In the inverse design of super-optics, a real-time simulation response is required. Compared with current commercial software,ANNs-based surrogate models can be easily integrated into inverse design schemes and have more design freedom.
Reverse Design
Gradient-based Neural Networks
Depending on the type of model used,Deep learning-assisted inverse design can be divided into two parts:
1. Based on the discriminant model
2. Based on the generative model
The inverse design methods based on discriminant models can be further divided into two categories. The first category is to put the design parameters at the input position, and the target response as the output will affect the design parameters through back propagation. This type of design solution is simple, but it is time-consuming as an iterative optimization method.The second type is more direct and therefore the mainstream method.That is, given an expected value, NN outputs a predicted value.

(a) Target optical properties and absorptivity of S parameters
(b) Proposed design workflow
(c) 3D graphics of the model under study, which can be represented by a matrix
NN-based inverse design requires less optical knowledge. ANNs only provide an approximate solution to the system, which is not exactly the same as the target requirement. Most methods show an accuracy of 70%+ when designing on demand, and the speed is quite fast. Traditional trial-and-error inverse design is time-consuming and cannot guarantee the accuracy of the solution. Despite the differences,But the proposed solutionIt's better than no solution.
Gradient-free evolutionary computation
Evolutionary computation is an important branch of AI and a family of meta-heuristic algorithms, including genetic algorithms, evolutionary algorithms, ant colony algorithms, and particle swarm algorithms. It imitates the process of biological evolution and simulates the process of racial reproduction through the iterative process of computer programs. Each generation introduces mutations as small random changes, and unqualified solutions are discarded through selection. Ultimately, the optimal solution is obtained through this evolution.Evolutionary computation is often considered a collection of global optimization algorithms.
Genetic Algorithm (GA) is one of the most commonly used evolutionary computing strategies.In recent years GA greatly facilitates the inverse design of metasurfaces.Such as superlens, terahertz quarter-wave plate, programmable metamaterials, and subwavelength lattice optics.
Data analysis
AI also demonstrated its powerful data analysis capabilities in super-optics, similar applications include computer vision tasks on images captured from metalenses. AI is more often used to process unreadable data from metasurfaces, such as image analysis, microwave signals, and infrared spectral information.

(ac) Data analysis for chemical composition classification tasks
(a) Schematic diagram of the metasurface chemical classifier
(b) Transmission spectrum of each chemical
(c) Visualization of the classification results of the first two principal components (top) and the first three principal components through PCA
(dg) Data analysis of acoustic imaging
(d) Schematic diagram of experimental configuration
(e) Propagation of waves with high-amplitude wave-vector components containing subwavelength signature information without (left) and with (right) a metalens.
(f) Data flow from the radiation source to the back-end reconstruction and identification
(g) Reconstruction and recognition results of far-field information without superlens (top) and with superlens (bottom)
Intelligent programmable super devices
With the help of AI, a system based on programmable metasurfaces is like a computer with a CPU installed. When a programmable or reconfigurable metasurface is combined with AI, the data flow between them forms a loop. AI is responsible for acquiring and processing optical datadata and regulate the reconstruction of programmable metasurfaces.
This allows the metasurface to evolve from a regular optical diffraction element to an intelligent element that understands input data and gives real-time responses on its own.

(ac) Intelligent Imager
(b) 16 radiation patterns and the corresponding patterns generated by machine learning
(c) Machine learning-driven imaging results for the two cases at different measurement times (100, 200, 400, and 600).
(d) Intelligent imagers and recognizers
(e) A smart cloak
In addition to the smart super devices discussed above,A programmable metasurface powered by AIIt can also achieve real-time complex beamforming and form three-dimensional perception.
The era of super devices may be coming
A report on emerging optical and photonic technologies by Lux Research, a US consulting firm, shows that meta-optical materials have been deployed commercially.andIt will account for a multi-billion dollar market by 2030.
byTaking Metalenz and NIL Technology, two international leading metasurface companies, as examples, their commercialization progress includes:Metalenz combines meta-optical technology with semiconductor manufacturing processes, achieves mass production in STMicroelectronics' 12-inch wafer foundry, and applies metalenses to STMicroelectronics' FlightSense series ToF ranging sensor VL53L8; NIL Technology has built a complete metalens industry chain, including design, prototyping, testing and characterization, and manufacturing capabilities, and has achieved shipments of metalenses.
It is worth noting that this year,Metalen announces the acquisition ofA new round of $10 million in venture capital,"We can replace up to six traditional optical devices in current modules with a single meta-optical device while improving system-level performance," said Robert Devlin, co-founder and CEO of the company.
It can be seen that super devices represented by super lenses are moving from laboratories to the industry, gradually becoming a hot spot in optical cutting-edge technology, and are expected to bring a revolution to the optical industry. Among them, AI plays a vital role in the development of super optics. Applying AI to super optics can solve complex optical designs, quickly obtain the best solutions to problems, and meet the needs of new functions. Therefore, it is certain that AI will be applied to super optics.The combination of the two will certainly further contribute to the research and development of advanced optical chips and promote the realization of next-generation optical devices and systems as soon as possible.
Reference Links:
[1]https://baijiahao.baidu.com/s?id=1755507538405155365&wfr=spider&for=pc
[2]https://zhuanlan.zhihu.com/p/540485936
Paper address: