Hawaii and Many Other Places Around the World Are Caught in the "doomsday Fire". Can AI Monitoring Outperform Wildfires at Critical Moments?
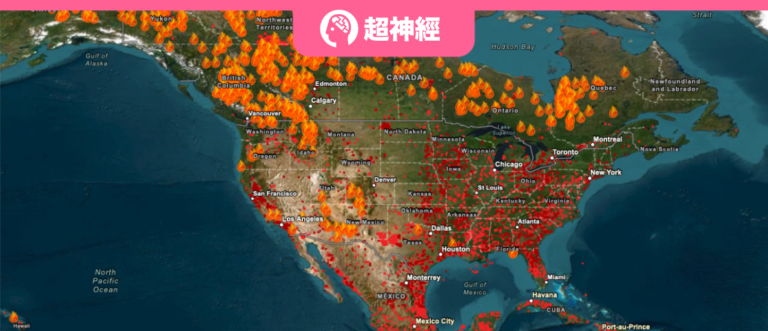
The Earth is experiencing irreversible global warming. According to the European Union's Copernicus Climate Change Service (C3S),July 2023 was the warmest month globally since 1940, with temperatures rising by about 1.5°C above the pre-industrial average.,Exceeding the critical value set by the Paris Agreement.
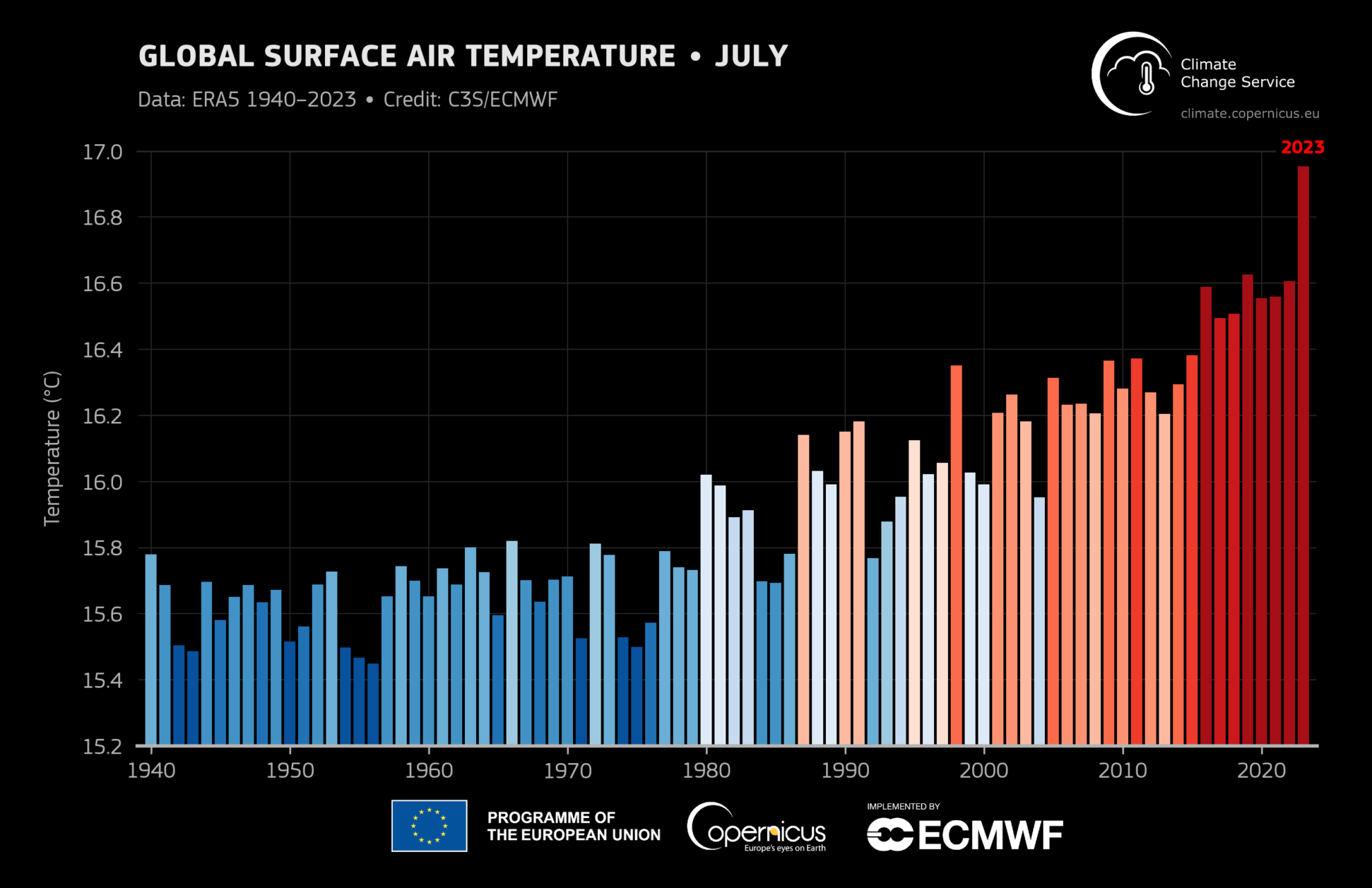
The most direct feeling brought about by global warming is high temperature.In areas with dense forests, hot and dry climates are very likely to cause wildfires. On August 8, a wildfire broke out on Maui Island in Hawaii, USA. Riding on Typhoon Dora, the wildfire quickly swept through the forest and spread to the local cultural center, Lahaina, leaving tens of thousands of people homeless.
At the same time, wildfires are also raging in the dry western part of North America. The fire map of the Fire Information for Resouce Management System US/Canada (FIRMS) shows thatIn the past week, wildfires with a burning area of more than 1,000 acres have spread across western Canada, and there are also a large number of fire spots in the eastern United States.

Wildfires are fast and relentless, and it is difficult for people to respond in time to sudden wildfires. But now,We can use AI to monitor and predict wildfires in real time and minimize the losses caused by wildfires.
Predicting Wildfire Trident
Geographic data: Australian university develops XAI
In May 2023, Abolfazl Abdollahi of the Australian National University and Biswajeet Pradhan of the University of Technology Sydney,Using Explainable AI (XAI), we conducted a comprehensive analysis of different geographical factors in Gippsland, Australia, and obtained a local wildfire probability distribution map., providing a new method for predicting the occurrence of wildfires.

The geographical features that have a greater impact on the probability of wildfires include environmental factors, geological factors, vegetation factors and meteorological factors.In this study, the researchers mainly used the following 11 characteristics, including precipitation, wind speed, temperature, humidity, vegetation distribution, vegetation area, fuel nitrogen in plants, moisture, and the altitude, slope and aspect of the area.
The study selected 521 wildfire sites in Gippsland City and trained them using Moderate Resolution Imaging Spectroradiometer (MODIS) data, thermal anomaly data, and fire history data from 2019 to 2020.The training set is processed by cross-validation and retention of data sets.Ensure the accuracy of the training process.
First, the cross-validation technique randomly divides the training set into 5 subsets, 4 of which are used for model training and 1 subset is used as a validation set.

The blue data is used for training and the orange data is used for validation.
After the training is completed, the environmental, geological, vegetation and meteorological characteristics of Gippsland are input into the model, and a complete wildfire probability map for the region can be obtained, as shown in the figure.The model prediction results are basically consistent with the historical wildfire areas in the area, indicating that XAI can effectively predict the occurrence of wildfires based on geographical characteristics.

a:Map of wildfire probability predicted by the XAI model;
b:Map of historical wildfire areas in Gippsland.
Paper address:
https://www.sciencedirect.com/science/article/pii/S0048969723016224
Video data: Identifying fire points based on smoke
Wildfire predictions based on geographic data can only raise people's awareness, but cannot observe the occurrence of wildfires in real time. To this end, the California Department of Forestry and Fire Protection, in collaboration with the University of California, San Diego (UCSD), developed a wildfire prevention project called ALERTCalifornia based on AI.This project uses more than 1,000 cameras distributed across California to monitor the area and use AI to identify abnormal conditions.Send an alert to the emergency command center, alerting on-duty personnel to confirm whether a wildfire may have occurred.

The project was officially put into operation in July 2023 and soon came in handy. At 3 a.m., a camera detected a fire in the Cleveland National Forest, 80 kilometers east of San Diego. Because the incident happened late at night, the smoke was difficult to detect, and it was difficult to identify the fire point with the naked eye, which could easily cause the fire to spread. But AI promptly alerted the fire chief and helped the fire department put out the wildfire within 45 minutes.
However, this technology also faces many challenges during its development. One of them is thatHow to enable AI to accurately distinguish wildfires from other interference factors and make accurate judgments.There are many factors in the forest that may cause false alarms, including clouds of different shapes, dust in the air, and even exhaust from passing trucks. With the joint efforts of more than 100 experts, AI has significantly improved its accuracy after several weeks of training and iteration.
ALERTCalifornia Address:
https://cameras.alertcalifornia.org
Satellite data: Near real-time wildfire monitoring with secondary screening
In certain areas, cameras can effectively monitor wildfires, but this method is difficult to promote on a large scale, especially in areas with vast areas and complex terrain, where the cost of deploying and maintaining cameras will increase significantly.Real-time satellite data can also be used as a weapon for AI to monitor wildfires in real time.
He Binbin's research group at University of Electronic Science and Technology of China,A machine learning model was built by combining the Random Forest model and the Spatial Contextual Algorithm.Near real-time wildfire monitoring is enabled by satellite data.
Traditional AI wildfire identification systems often use a single algorithm, which results in missing data or false positives.The data is first strictly screened by the random forest model to avoid omissions. Then it is screened again using a spatial context algorithm with a relatively low threshold to eliminate false alarms.This will improve the accuracy of the monitoring model.

The researchers used wildfire data recorded by the Japan Aerospace Exploration Agency (JAXA) and NASA as a training set to train the model. Subsequently, satellite data of wildfire locations from 2020 to 2022 were used as validation data for the model to make judgments.

As can be seen in the figure,For most fires, this model can accurately locate the source of the wildfire and provide timely warnings. The AI wildfire identification model uses real-time satellite data as its data source, combined with a random forest model and spatial context algorithm, to reduce the model's missed alarm rate and false alarm rate, and achieve near real-time wildfire warning.
Paper address:
Wildfire Fighting: A Race Against Time
The main cause of wildfires is human activities.Such as unattended campfires, carelessly discarded cigarette butts or improper use of equipment. The possible cause of the Hawaii wildfires was damaged power facilities. The flames grew rapidly under the combined influence of the hot and dry environment brought about by global warming and Typhoon Dora, causing huge losses to local residents.

Fighting wildfires is a race against time.It is estimated that wildfires can spread about 23 kilometers per hour, and the burned area will expand four times within four hours. The Thomas wildfire in California, USA in 2017 spread an area of a football field per second and eventually burned for more than three months.
Therefore, wildfire monitoring and firefighting often require the use of multiple technologies to detect the fire point in the shortest time possible, extinguish the wildfire as quickly as possible, and prevent it from spreading.Currently, my country has six lines of defense for monitoring wildfires, namely satellite monitoring, aircraft monitoring and patrol, forest lookout towers, video surveillance systems, ground patrols and online public opinion awareness.
With the support of various technologies, the number of forest fires in my country has dropped from 7,723 in 2010 to 709 in 2022. However, the forest fires in Muli County, Sichuan Province in 2019 and Xichang City, Sichuan Province in 2020 both caused significant casualties, and the monitoring and prevention of forest fires still face challenges.
At present, geographic data, video data and satellite data can all be used as raw data for AI to make early judgments on wildfires and nip the fire in the bud. Although my country has a vast territory and the climate and terrain vary greatly from place to place, it is difficult to completely prevent wildfires.butI believe that with the help of AI, we can eventually catch up and minimize the danger of wildfires.
Reference Links:
[1]https://climate.copernicus.eu/july-2023-warmest-month-earths-recent-history
[2]https://firms2.modaps.eosdis.nasa.gov/
[3]https://www.washingtonpost.com/climate-environment/2023/08/10/hawaii-wildfire-maps/
[4]https://www.bjnews.com.cn/detail/168429261314778.html