31 Cases of Missed Diagnosis Were Identified Among 20,000 Cases. Alibaba Damo Academy Took the Lead in Launching "plain Scan CT + Large Model" to Screen Pancreatic Cancer
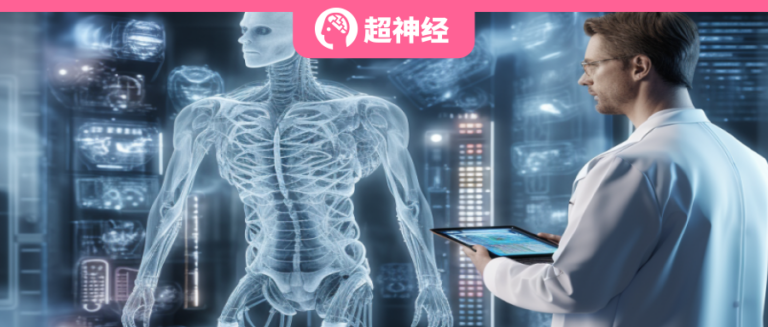
Despite the rapid development of medicine, people still can't help but talk about "cancer". Among them, pancreatic cancer is particularly difficult to treat due to its hidden onset and short survival time. Wu Zunyou, chief epidemiologist of the Chinese Center for Disease Control and Prevention, and Steve Jobs, the father of Apple, have not escaped the "clutches" of pancreatic cancer. Among them, pancreatic ductal adenocarcinoma (PDAC), which accounts for 95% of pancreatic cancer cases, is one of the most lethal types of cancer among all solid tumors and is known as the "king of cancer." According to 2020 data, PDAC causes about 466,000 deaths each year.
The World Health Organization has proposed that one-third of cancers can be cured through early detection. However, pancreatic cancer has few obvious signs in the early stages, and the pancreas is located "deep" in the body and hidden. Basic imaging examinations such as abdominal ultrasound and plain scan CT are difficult to identify early lesions due to low image contrast. Image diagnosis such as enhanced CT is not suitable for large-scale early screening of pancreatic cancer because it requires injection of contrast agents, has a long examination cycle, and is expensive.
Regarding the early screening and early treatment of pancreatic cancer,Alibaba DAMO Academy has developed Pancreatic Cancer Detection with Artificial Intelligence (PANDA) based on deep learning in collaboration with domestic and foreign medical institutions such as the Shanghai Institute of Pancreatic Diseases, the First Affiliated Hospital of Zhejiang University School of Medicine, and the Shengjing Hospital of China Medical University., through "plain scan CT + AI" to conduct large-scale early screening of pancreatic cancer. The research results have been published in "Nature Medicine".

Paper address
https://www.nature.com/articles/s41591-023-02640-w
Dataset: Contains 5 patient cohorts
The dataset for this study contains 5 patient cohorts:
* Internal training dataset queues for building AI models;
*Internal test queue for evaluating model performance;
*External multicenter testing cohort to evaluate model generalizability;
* Chest non-contrast CT test cohort, used to assess generalization to chest CT scans;
*Actual clinical evaluation cohort, used to evaluate clinical translation issues.
Among them, in the internal training cohort, PANDA was trained on a training set of abdominal plain CT scans from 3,208 patients admitted to the Shanghai Institute of Pancreatic Diseases (SIPD) between January 2015 and October 2020, with two years of follow-up confirmation by the researchers.
Model architecture: Transformer to identify lesion types
PANDA (Pancreatic Cancer Detection with Artificial Intelligence) consists of three cascaded network stages, with increasing model complexity and task difficulty. First, a segmentation network (nnU-Net) is built to locate the pancreas, then a convolutional neural network (CNN) is used to detect abnormal lesions, and finally a dual-channel Transformer is used to classify and identify the type of pancreatic lesions.

In the first stage (Figure a), since pancreatic lesions only appear as a small area in CT scans, pancreatic localization can accelerate the process of lesion discovery and eliminate information that is not related to professional training in the pancreatic area.The researchers used nnU-Net, a medical image segmentation framework, to segment the entire pancreas from an input non-contrast CT scan.
The second stage (Figure b) is mainly for lesion detection.The researchers extracted multi-level features from the segmentation network and constructed a convolutional neural network and classification head to distinguish subtle texture changes in pancreatic lesions in plain CT.At the same time, the researchers adjusted the second-stage model so that its specificity for lesion detection reached 99% in cross-validation of the training set, reducing false positive predictions.
The third stage (Figure c) is mainly about differential diagnosis of pancreatic lesions.If any abnormality is detected in the second stage, an auxiliary memory Transformer is integrated to automatically encode the characteristic prototypes of pancreatic lesions, such as local texture, location, and pancreatic shape, for more accurate fine-grained classification.
Experimental verification: The model performs slightly better than professional film readers
Internal testing
In the internal test evaluation, the research team selected 291 patients from the Shanghai Pancreatic Disease Research Institute for lesion detection, including 108 patients with pancreatic ductal adenocarcinoma, 67 patients with non-pancreatic ductal adenocarcinoma, and 116 normal controls.
The area under the receiver operating characteristic curve (ROC curve) of PANDA (AUC) was 0.996 (95% confidence interval 0.991-1.00), with a sensitivity of 94.9% and a specificity of 100%. For common subtypes of pancreatic ductal adenocarcinoma, PANDA had a sensitivity of 97.2% and a specificity of 97.3%. For smaller PDAC (diameter <2 cm), the detection sensitivity reached 85.7%.

External Testing
In the external multicenter test evaluation, the researchers selected 5,337 patients from 9 centers in mainland China, Taiwan, and the Czech Republic, including 2,737 patients with pancreatic ductal adenocarcinoma, 932 patients with non-pancreatic ductal adenocarcinoma, and 1,668 normal controls.
The results showed that for pancreatic lesions, the AUC value of PANDA was 0.984, the sensitivity was 93.3%, and the specificity was 98.8%; for the PDAC subgroup, the overall detection rate was 96.5%. For PDAC with smaller lesions (diameter <2 cm, T1), the sensitivity was 92.2%. Overall, the sensitivity of PDAC diagnosis reached 90.1%, and the specificity reached 95.7%.
Chest CT scan test
In addition, the researchers also verified the feasibility of using PANDA to detect pancreatic lesions on chest CT. The researchers collected non-contrast chest CT scan images of 492 patients from the Shanghai Institute of Pancreatic Diseases, including 63 patients with pancreatic ductal adenocarcinoma, 51 patients with non-pancreatic ductal adenocarcinoma, and 378 normal control patients as a test cohort independent of the training data.

Without adjusting any chest CT scans, PANDA had a sensitivity of 86.0% and a specificity of 98.9% for lesion detection. It is worth mentioning that according to the detailed chest CT protocol, some pancreatic lesions could not be completely scanned. The researchers analyzed the completeness of lesion scanning in chest CT by referring to the lesion location in contrast-enhanced abdominal CT scans and found that 67% of patients with pancreatic ductal adenocarcinoma and 43% of patients with non-pancreatic ductal adenocarcinoma were not completely scanned. Among the patients whose pancreatic lesions were not captured by the CT scan field of view, 75% of pancreatic ductal adenocarcinoma cases were successfully detected by PANDA.
Clinical application test
In addition, the research team conducted two rounds of clinical application evaluations to further verify the usability of PANDA in actual scenarios.
A total of 16,420 patients were selected in the first round to evaluate the effects of PANDA in terms of actual clinical performance, changes in nursing diagnosis scenarios, and patient benefits.
The results showed that in lesion detection, PANDA had a total sensitivity of 84.6% and a specificity of 99.5%; in the identification of pancreatic ductal adenocarcinoma, PANDA had a total sensitivity of 95.5% and a specificity of 99.9%. In addition, in the four scenarios of physical examination, emergency, outpatient and hospitalization, PANDA had the highest sensitivity for lesion detection in hospitalized patients, which was 88.6%, and PANDA had the highest specificity for lesion detection in physical examination patients, which was 99.8%.
Before the second round of testing and evaluation, the researchers optimized the model to reduce false positives and expand the types of diseases that had not been observed before. Through hard example mining and incremental learning, the researchers upgraded PANDA to PANDA-plus and conducted a second round of clinical application research.
A total of 4,110 patients were included in this round of evaluation.PANDA-plus reduced the false positive rate by more than 80.% compared with PANDA, and the specificity of pancreatic lesion detection and pancreatic ductal adenocarcinoma reached 99.9%. At the same time, the sensitivity of PANDA-plus for the detection of acute pancreatitis also reached 90.0%.

More importantly,In a real-world clinical study involving 20,530 patients, PANDA detected five cancers and 26 clinically missed cases, and provided curative treatment for one patient with a pancreatic neuroendocrine tumor.
In addition, the research team also collaborated with pancreatic imaging experts, general radiologists, and radiology residents to conduct actual film reading comparisons. Fifteen experts in pancreatic imaging interpreted multiphase enhanced CT scans of the same 291 patients, and PANDA performed slightly better on non-enhanced CT scans than the average performance of the expert readers using enhanced CT scans.
DAMO Academy’s AI Medical Landscape
In an interview with the media, Lü Le, head of the DAMO Academy's medical AI team and IEEE Fellow, said: "Our greatest innovation is that we have proven for the first time the feasibility of using AI for pancreatic cancer screening on plain scan CT scans, and have achieved high performance that was previously thought to be unattainable."
In addition, Dr. Cao Kai, co-first author of the paper and from the Shanghai Institute of Pancreatic Diseases, believes that "PANDA will broaden the industry's understanding of pancreatic cancer screening and promote the development of clinical treatment." Dr. Tang Wei, another co-first author and from the Department of Radiological Diagnosis, Affiliated Cancer Hospital of Fudan University, said, "PANDA proposes a potential large-scale pancreatic cancer screening method that improves the detection rate without causing additional radiation and financial burden to patients."
According to data provided by the official website of the DAMO Academy, PANDA has been called more than 500,000 times in hospitals, physical examinations and other scenarios, with only one false positive every 1,000 times.
There is no doubt that this is a heavy blow to pancreatic cancer under the combination of AI and medical data, making early screening and early treatment possible. This is exactly the original intention of medical AI. Readers who know about DAMO Academy should know that its medical AI team has long been committed to the integration of AI and medical imaging. It is reported that the medical AI team of DAMO Academy focuses on three major directions: precise cancer diagnosis and treatment, precise chronic disease diagnosis and treatment, and pre-screening of neurodegenerative diseases.
In addition to PANDA,In August this year, DAMO Academy, together with the Cancer Center of Sun Yat-sen University, Sichuan Cancer Hospital, the First Affiliated Hospital of Zhejiang University, Shengjing Hospital, Guangdong Provincial People's Hospital and other institutions, proposed a unified multi-cancer imaging analysis model (cancerUniT).Based on Mask Transformer semantic segmentation, it solves the problem that it was previously difficult to uniformly detect, segment and diagnose multiple tumor images. It is applicable to 8 mainstream high-incidence and high-lethal cancers (lung, colorectal, liver, stomach, breast, esophagus, pancreas, and kidney) as well as tumor subtypes in related organs.
Previously, among the 100 AI patents that DAMO Academy has made available for free, three are specifically for precision cancer treatment, applied to "image-guided radiotherapy", "longitudinal lesion quantification", and "atlas-based segmentation and multimodal fusion computer-aided diagnosis".
also,In October 2022, DAMO Academy also jointly proposed the hierarchical segmentation model of risk organs (SOARS) with the First Affiliated Hospital of Zhejiang University School of Medicine.This automated and efficient algorithm system, SOARS, builds a two-dimensional hierarchical deep learning framework to achieve accurate segmentation of 42 head and neck OARs.
It is reported that the DAMO Academy's medical AI team is working with a number of top medical institutions around the world to use AI technology to explore new low-cost and efficient methods for multi-cancer screening, and also hopes that it can bring more AI "black technology" into the medical field.
References:1.https://mp.weixin.qq.com/s/WhWnkkAFJjAkqGlMTDEx9w2.https://mp.weixin.qq.com/s/wkNutLLWNHkZByY0QV90pg3.https://mp.weixin.qq.com/s/_qhIW3OB3qnjs83izKvWBg