Kew Gardens, UK, Uses Machine Learning to Predict Plant Resistance to Malaria, Increasing Accuracy From 0.46 to 0.67
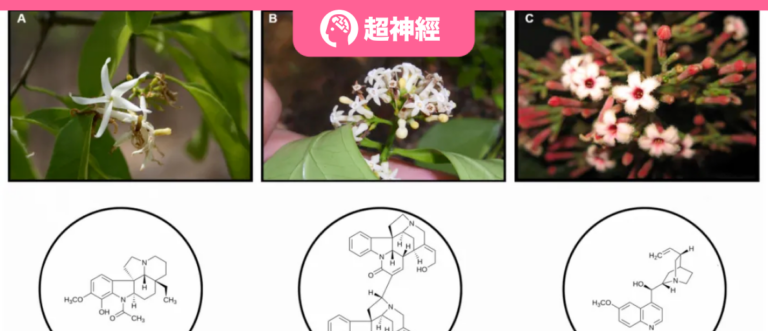
Malaria is a parasitic disease that ravages the world. It is transmitted by mosquitoes, and its morbidity and mortality rates remain high among vector-borne diseases. According to the latest World Malaria Report, the global malaria epidemic further intensified in 2021.There were 247 million new cases and 619,000 estimated deaths throughout the year.
At present, drug treatment is still the main means of malaria prevention and treatment in the world, and the anti-malarial active natural molecules of many drugs are derived from plants.Therefore, researchers have been working to find new plant-derived antimalarial compounds.However, to achieve this goal, large numbers of plants need to be screened and tested, a process that is time-consuming and expensive.
Recently, researchers from the Royal Botanic Gardens, Kew and the University of St Andrews demonstrated that machine learning algorithms can effectively predict plant antimalarial properties with an accuracy of 0.67, a significant improvement over the 0.46 of traditional experimental methods.Currently, the research results have been published in the journal Frontiers in Plant Science, titled "Machine learning enhances prediction of plants as potential sources of antimalarials".

The research results have been published in Frontiers in Plant Science.
Dataset and sampling bias correction
One of the important goals of this experiment is to evaluate whether plant feature data can be used to train machine learning models to predict plant antimalarial activity.first,The researchers provided a dataset of 21,100 plant species from three floral plant families in the Gentianales order: Apocynaceae, Loganaceae, and Rubiaceae.These plants have been found to contain many alkaloids, such as quinine, an antimalarial alkaloid, and its isomer quinidine.

Figure 1: Examples of antimalarial alkaloids found in oleander, Strychnos nux vomica and Rubiaceae
A: Aspidocarpine is an alkaloid found in plants of the Apocynaceae family.
B: Strychnogucine, an alkaloid found in plants of the Strychnaceae family.
C: Quinine, an alkaloid found in Rubiaceae plants and now widely used in antimalarial drugs.
The data set specifically includes information on plant morphological characteristics, biochemical characteristics, growth environment conditions, and geographic location.The following figure shows the relationship between binary features (features with only two possible values, such as toxic/non-toxic) in this dataset.

Figure 2: Relationships between binary features in the dataset
X-axis: binary features.
Y-axis: the average value of each feature, where each feature represents a different plant attribute, such as whether it is poisonous, whether it is used as a traditional medicine, etc.
As shown in the figure, 101 TP3T of all plant species are used as traditional medicines, while 771 TP3T of poisonous plant species are used as traditional medicines.The researchers call this difference sampling bias and propose that it is caused by the ethnobotanical approach.
Ethnobotany is the search for medicinal plants by finding and studying plants that local people use to treat illnesses.However, due to differences between regions and cultures,It is possible that one or several antimalarial plants appear frequently in the data set, while other plants that may have antimalarial properties are ignored. This is called sampling bias.
In order to better train the model, the researchers corrected for sampling bias.The specific method is to re-weight each plant species, that is,Inverse Probability Weighting is used ,In this way, each species sample can be treated equally in model training, thereby improving the representativeness of the dataset and the performance of the model.
Experimental results display
Model training and validation
In this experiment,The researchers trainedSupport Vector Machine (SVC), Logistic Regression (Logit), XGBoot (XGB), and Bayesian Neural Network (BNN) 4 machine learning models,These models were combined with 2 ethnobotanical methods:Searching for traditional antimalarial plants and traditional medicinal uses(not specific to malaria) plants for comparison.
For the three models based on Logit, SVC and XGB,The researchers' training method is to adjust the model's hyperparameters through the GridSearchCV algorithm and use the F0.5 indicator to evaluate the model performance.Among them, the researchers adjusted the regularization parameter C and class_weight parameter for the two models based on Logit and SVC; for the model based on XGB, they adjusted the max_depth parameter.
For the BNN-based model, the researchers used two layers of neural networks with 10 and 5 layers respectively and the Tahn activation function.The model was trained with 100,000 Markov chain Monte Carlo iterations.
During the verification phase,The researchers used 10-fold stratified cross-validation with 10 iterations in two cases (without sampling bias correction and with sampling bias correction). The model performance was evaluated using the 10 iterations of 10-fold stratified cross validation method.
Experimental Results
First, without sampling bias correction,The researchers' experimental results on screening plant-derived antimalarial compounds are as follows:

Figure 3: Without bias correction
Comparison of machine learning models with two ethnobotanical methods
As shown in the figure, overall,The average score of the machine learning model was higher than that of the two ethnobotanical methods.,And it can predict antimalarial activity from data features (BNN: 0.66, XGB: 0.66, Logit: 0.62, SVC: 0.65, Ethno (M): 0.57, Ethno (G): 0.50).
When bias correction is performed,The researchers' experimental results on screening plant-derived antimalarial compounds are as follows:

Figure 4: When bias correction is performed
Comparison of machine learning models with two ethnobotanical methods
As shown in the figure, although the variance of the model performance is higher due to the added weights for the training and test sets,butThe machine learning model still performed better than the ethnobotanical approach.The researchers estimated the accuracy of the traditional plant selection method to be 0.47, while the prediction accuracy of the machine model was generally higher than this number (BNN: 0.59, XGB: 0.63, Logit: 0.66, SVC: 0.67).
However, although this experimental result shows that machine learning models can relatively accurately screen plants with antimalarial activity, the researchers said,There are still some areas that need improvement in this experiment:
* Increase training data:Currently the training dataset is relatively small, and more plant species data need to be added to further improve the performance of the model.
* Solve the problem of sampling bias:Although this experiment has attempted to address the sampling bias problem, more bias correction methods still need to be explored.
* Optimize feature selection:More plant trait selection and optimization is needed.
* Further testing of plant species with too few species or uneven sample distribution:For species that are underrepresented in existing data, more testing is needed to obtain more accurate results.
Kew Gardens, Kew: Discover the power of plants
Regarding this research result, the director of the Royal Botanic Gardens, Kew, said:“Our results showPlants have great potential for producing new medicines.An estimated 34,300 vascular plant species are known, but many have not been extensively studied scientifically.We hope that machine learning methods can be applied in this regard to find new medicinal compounds.And these results also highlight the importance of protecting biodiversity and sustainable development of natural resources."
The world-famous Royal Botanic Gardens, Kew is often referred to as Kew Gardens. Kew Gardens is an internationally renowned botanical research and education institution funded by the Department for Environment, Food and Rural Affairs (UK) of the UK government. It is a non-governmental public organization. Kew Gardens' goals are:“Protect biodiversity and develop nature-based solutions to address the global challenges facing humanity.”
About a few months ago,There are news reports that Greensphere Capital, a fund dedicated to sustainable development, plans to invest £100 million in Kew Gardens.The investment will go toward sustainable agriculture and recruiting new researchers to work on projects such as plant and fungal science, habitat conservation, agriculture and forestry.