Complete Disassembly of AlphaFold 3, Zhong Bozitao of Shanghai Jiaotong University: Extreme Use of Data to Predict All Biomolecular Structures With Atomic Precision, but It Is Not Perfect
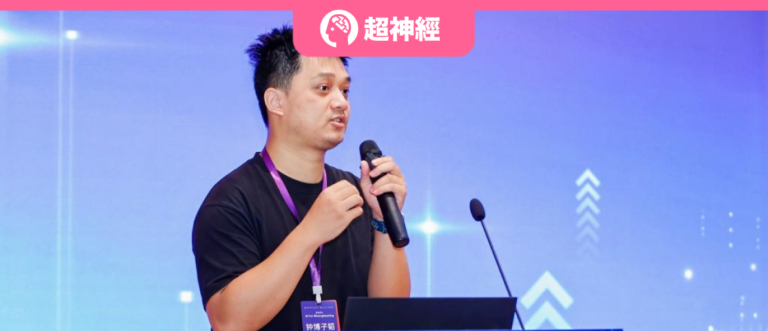
AlphaFold 3, which can predict all biomolecular structures and interactions with "atomic precision", has aroused widespread discussion in the industry since its release. On August 13, at the AI for Bioengineering Summer School event of Shanghai Jiao Tong University,Dr. Zhong Bozitao systematically sorted out his learning experience with the topic of "AlphaFold 3: Principles, Applications and Prospects", and extensively sorted out many relevant research results from the scientific research community, sharing his deep insights into AlphaFold 3 with everyone.HyperAI has organized the core content of the speech without violating the original intention. The following is the transcript of the speech.
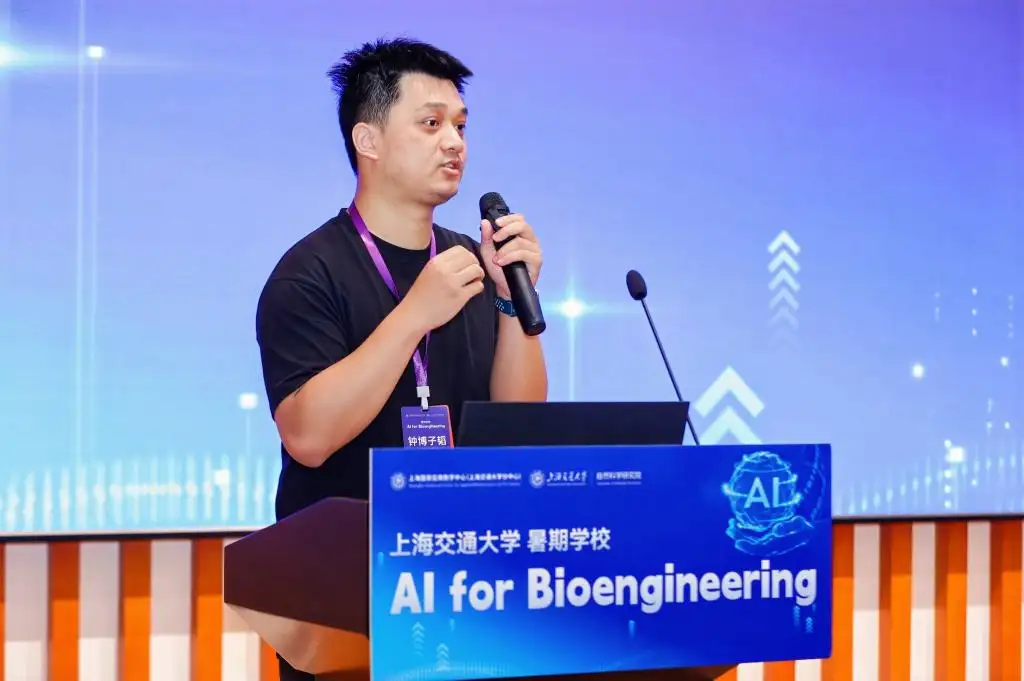
Focusing on protein structure prediction, today we will talk about AlphaFold 3, which is currently the top protein and even more extensive biological molecular structure prediction tool.The status of AlphaFold 3 is self-evident.
Protein synthesis begins with DNA transcription, which then transfers genetic information to RNA, which is then translated into protein, and further folded into secondary, tertiary, and quaternary structures. Most proteins fold into unique conformations, and the information required for the structure is encoded in the amino acid sequence, which is what we often say: sequence determines structure, and structure determines function.Protein structure prediction is crucial for understanding biological functions.
AlphaFold 3 breakthrough: Innovative model architecture and improved data utilization
Comparison of AlphaFold 3 and AlphaFold 2 model architectures
In the past, AlphaFold 2 directly "beat" other algorithms in protein structure prediction.Its core architecture can be summarized into three key parts, as shown in the figure below: The first part, the MSA & Template module in the blue box, is responsible for collecting and integrating multiple sequence alignment (MSA) and template structure information as input data for the model. The second part, the Evoformer module in the green box, is responsible for understanding the co-evolutionary information in multiple sequence organizations, refining and processing the collected information, and passing it to the Structure Module module in the purple box in the third part.
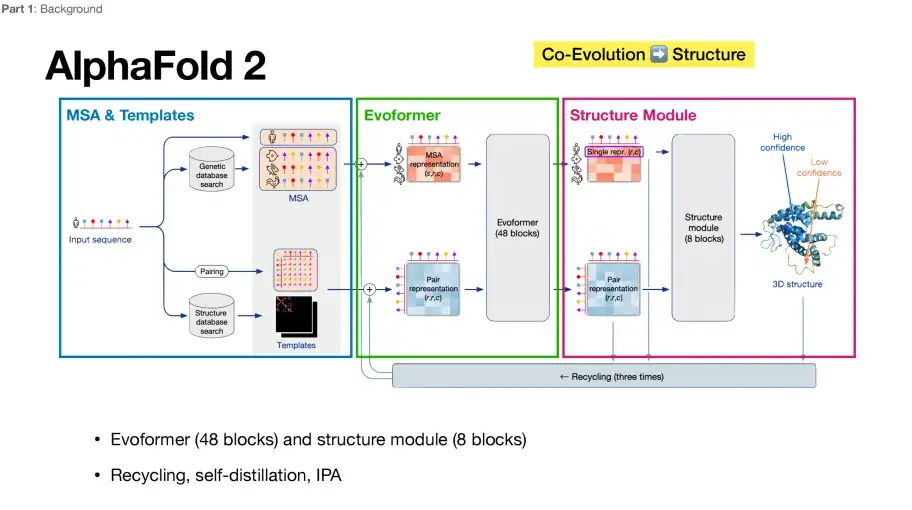
From a deep learning perspective, Evoformer plays the role of an encoder, while Structure Module is equivalent to a decoder.AlphaFold 2 has received much acclaim due in large part to its end-to-end optimization capabilities, mapping directly from sequence input to structural output.
It is generally believed that the changes in the model architecture of AlphaFold 3 are not as big as imagined. Its model framework is also composed of 3 key parts. The comparison between each part and AlphaFold 2 is as follows:
Part 1: Keeping it highly similar
As shown in the figure below, by comparing the architecture diagrams of AlphaFold 3 and AlphaFold 2, it can be seen that the first part of AlphaFold 3 (in the blue box) still includes MSA & Template, and additionally introduces the Conformer generation link.
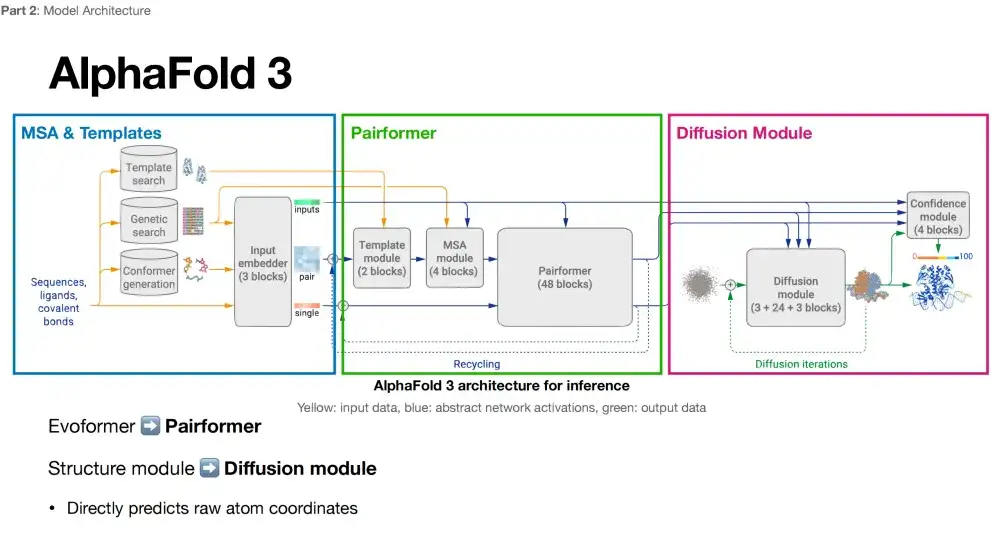
Part 2: Reducing reliance on MSA sequences
The second part of AlphaFold 3 (in the green box) is named Pairformer. Its structure is essentially very similar to Evoformer, but the number of MSA modules is reduced to 4. As shown in the figure below, the green arrows represent the same content of the two modules, and the yellow arrows represent the differences. You can see thatAlphaFold 3 places more emphasis on the target protein sequence and relies less on the MSA sequence.
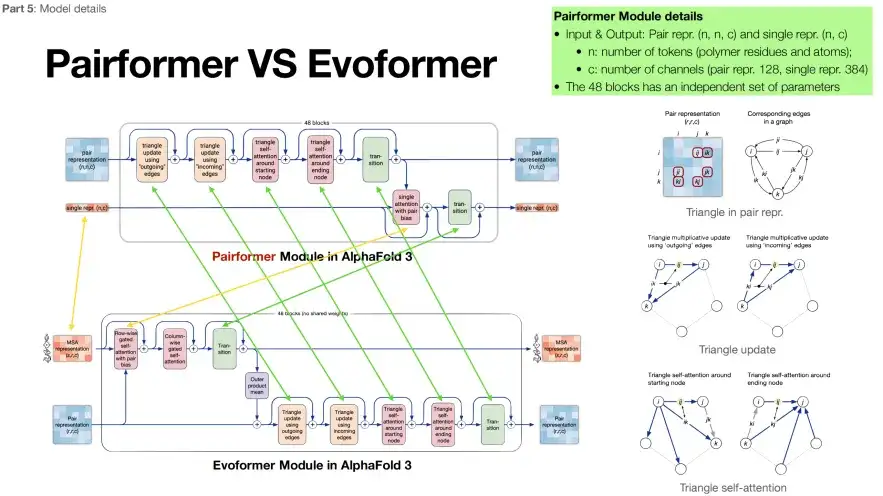
Furthermore, we believe that the reason why AlphaFold 3 can show strong performance in multiple tasks may be because it reduces its reliance on multiple sequence alignment (MSA).As shown in the figure below, the right side shows the impact of MSA on the performance of AlphaFold 2: As the number of MSAs increases, when it exceeds a certain threshold (pink line), the performance improvement of AlphaFold 2 tends to flatten out. According to the middle part of the figure below, compared with AlphaFold 2, the impact of MSA on AlphaFold 3 is weakened (the curve fluctuates very little).
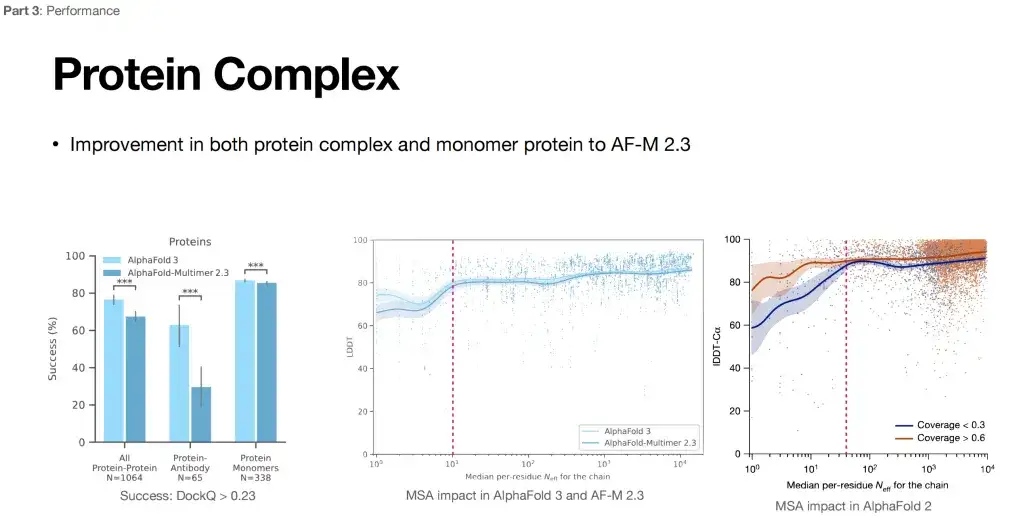
In addition, the maturation of antibodies often requires a hypermutation process in vivo. MSA information is of limited help in predicting their structure, and it is difficult to find paired MSA information for proteins and their complexes. From this point of view, the expansion of the application scope of AlphaFold 3 may be the reduction of its dependence on MSA.
Part III: All-atom structure generation + removal of stereo rotation invariance
The third part of AlphaFold 3 (in the purple box) adopts the Diffusion model, which also belongs to the category of structure module. The difference is that the Diffusion model replaces the repeated iterative optimization in the Structure Module with a new mechanism called the Diffusion model.
*Diffusion model: Add noise to the model (forward), denoise the model (reverse), learn the reverse process, and generate similar data distribution.
As shown in the figure below, in the third part, AlphaFold 3 achieves structure generation at the all-atom level. Atoms, as the basic building blocks of molecules, may contain richer physical information, which means that AlphaFold 3 may capture deeper physical laws when predicting protein structures. In addition, AlphaFold 3 abandons the stereo rotation invariance emphasized in AlphaFold 2. After deleting the additional architecture of this feature in AlphaFold 2, the researchers found that the design of the model (Diffusion Module) became freer.
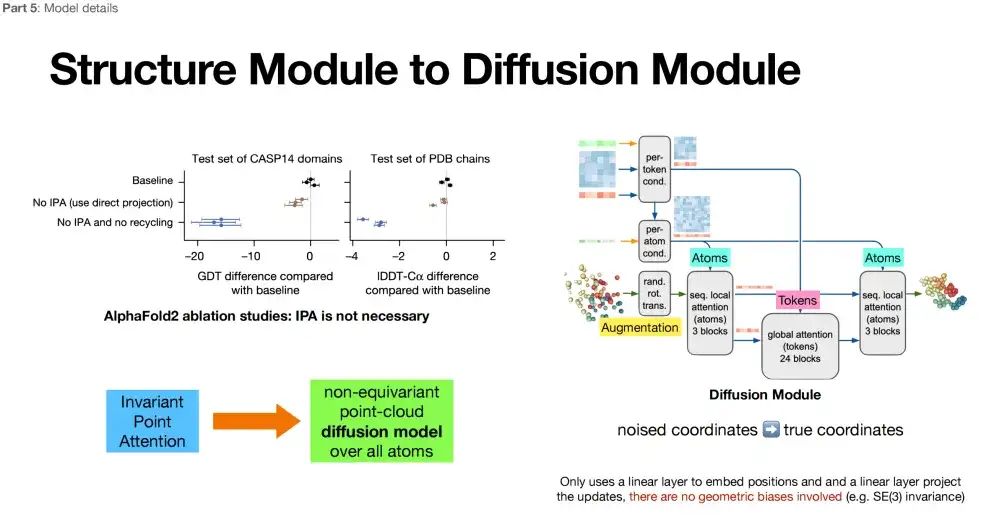
AlphaFold 3 improves data utilization
Protein data resources are limited, but AlphaFold 3 not only makes the data set larger, but also improves data utilization.Specifically, compared to the million-level data set of AlphaFold 2, AlphaFold 3 directly approaches the billion-level, and the training set is larger. In addition, in addition to the data in the PDB, its training set also incorporates a large amount of other data. For example, the structural data that AlphaFold 2 predicted more accurately is selected as the expansion of the training set. The specific training set is shown in the figure below:
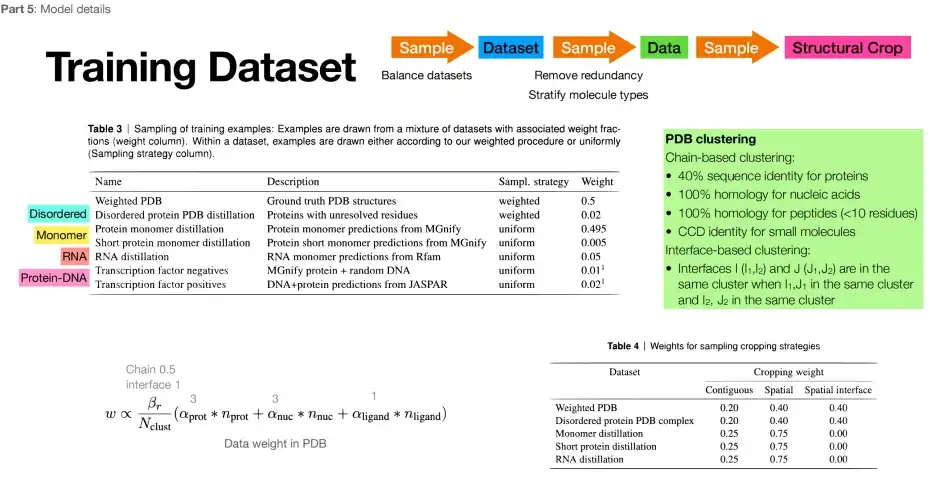
AlphaFold 3 achieves a huge leap in application scope
The biggest change of AlphaFold 3 is that it has achieved a qualitative leap in its scope of application.In the past, AlphaFold 2 was more focused on predicting amino acid structures, while AlphaFold 3 can directly predict atomic-level structures. Its functional expansion is specifically reflected in the following four aspects:
* Able to accurately predict ligands, that is, predict the binding sites of small molecules in proteins;
* Able to predict protein complex structure;
* Able to predict the post-translational modification structures of proteins and nucleic acids;
* Capable of predicting the structures of DNA and RNA, as well as the complex structures of DNA/RNA and proteins.
AlphaFold 3 changes the field of ligand docking
Among them, AlphaFold 3's greatest impact on the scientific field is the improvement of the Ligand Docking task.As shown in the figure below, the success rates of different deep learning algorithms in 4 different Ligand Docking tasks are evaluated under the PostBusters Benchmark. It can be found that AlphaFold 3 can achieve the highest success rate, i.e. 76.4%, under the premise of unknown pockets and structural prior knowledge.
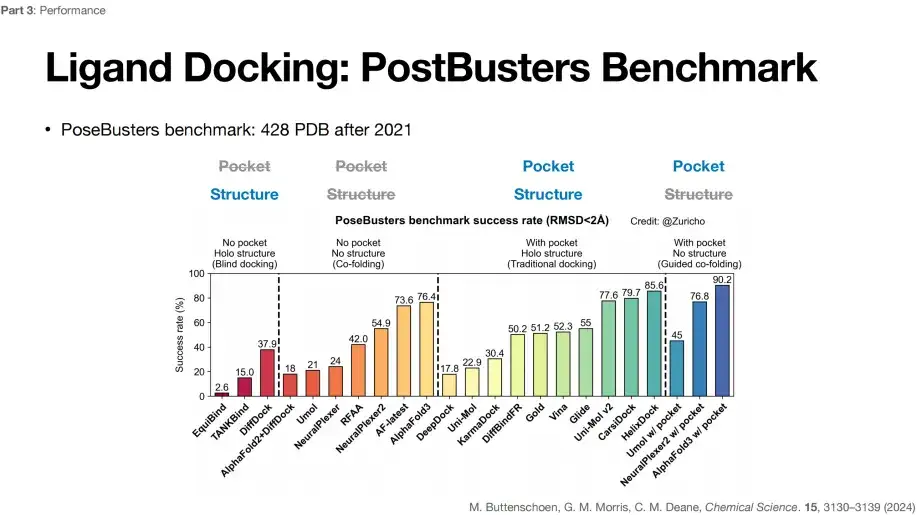
PostBusters Benchmark selects 428 PDB data from 2021 and later
The standard for task success is that the deviation between the predicted small molecule docking position and the actual docking position is less than 2 Å
As shown in the figure above, in the first type of blind docking task, with unknown pocket position and known protein structure (No pocket, Holo structure), DiffDock can achieve the highest success rate of 37.9%.
In the second type of co-folding task (small molecules and protein structures are folded), with unknown pocket positions and unknown protein structures (No pocket, No structure), the success rate of AlphaFold 2+DiffDock combined prediction dropped to 18%,In addition, AlphaFold 3 achieved the highest 76.4% success rate, which shows that AlphaFold 3 not only predicts accurately, but also does not rely on prior knowledge of pockets and structures.
In the third category of Traditional docking tasks, the pocket position of the small molecule and the protein structure are known (With pocket, Holo structure), that is, the pocket is exposed. Gold achieved a success rate of 51.2%, Vina showed a success rate of 52.3%, and Glide increased to 55%. Other deep learning algorithms can also achieve relatively good levels, indicating that the success rate is affected by the pocket.
In the fourth category of Guided co-folding tasks, the success rate of the model is significantly improved when the pocket position is known and the protein structure is unknown (With pocket, No structure), from 76.4% to 90.2% for AlphaFold 3, indicating that known pocket information can improve the success rate of the task. However, due to some controversy over the definition of pockets,Therefore, if you want to know the specific improvement of AlphaFold 3 on the Ligand docking task, you can only consider the success rate of the second type of task, which is relatively more stable.
As shown in the figure below, there are significant differences in the pocket definitions of different models. The Gold pocket is a 25Å sphere (the blue part in the upper left of the figure), while the Vina model uses a 25Å cube as the pocket representation, the DeepDock pocket size is 10Å, and the Uni-Mol pocket size is 8Å.
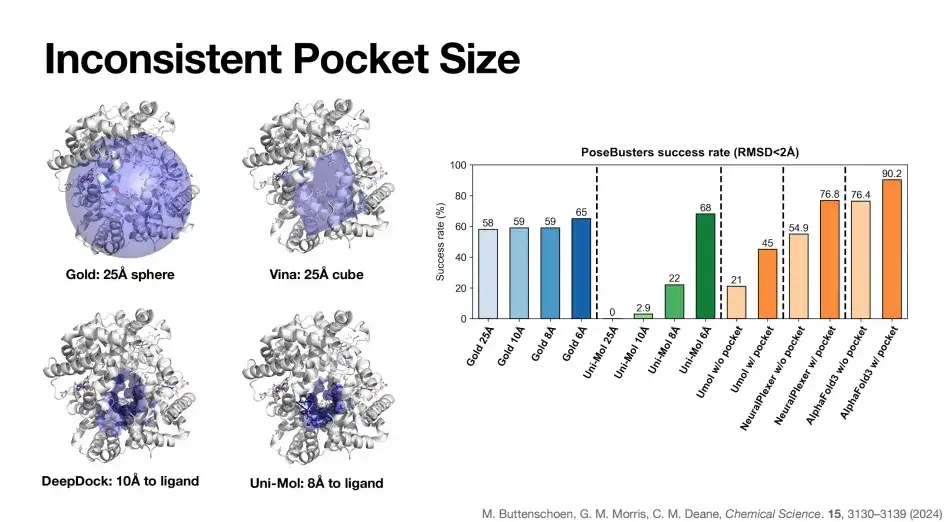
As shown on the right side of the figure above, when the pocket size of the Gold model is gradually reduced from 25Å to 6Å, its PoseBusters benchmark success rate is relatively stable, which is due to the characteristics of Gold based on physical algorithms. In contrast, the deep learning algorithm Uni-Mol gradually reduces the pocket to 6Å, and the success rate increases to 68%, and drops to zero at 25Å, reflecting the dependence of some deep learning docking algorithms on pockets.
Similarly, as mentioned earlier, after the introduction of pocket information, the docking success rate of AlphaFold 3 was significantly improved from 76.4% to 90.2%.In summary, pocket information plays a key role in improving the success rate of model prediction. But ideally, a model that can achieve high accuracy without pocket or structural information is our best choice, such as AlphaFold 3.
AlphaFold 3 enables antibody and antigen structure prediction
Another application of AlphaFold 3 is the structure prediction of antibodies and antigens. The left side of the figure below is the performance evaluation of AlphaFold 3 for antibody and antigen structure prediction. Under the lower evaluation standard (DockQ>0.23), only one run, the success rate of AlphaFold 3 is less than 40% (light blue line), but after 1,000 attempts, the prediction success rate can be increased to 60%.
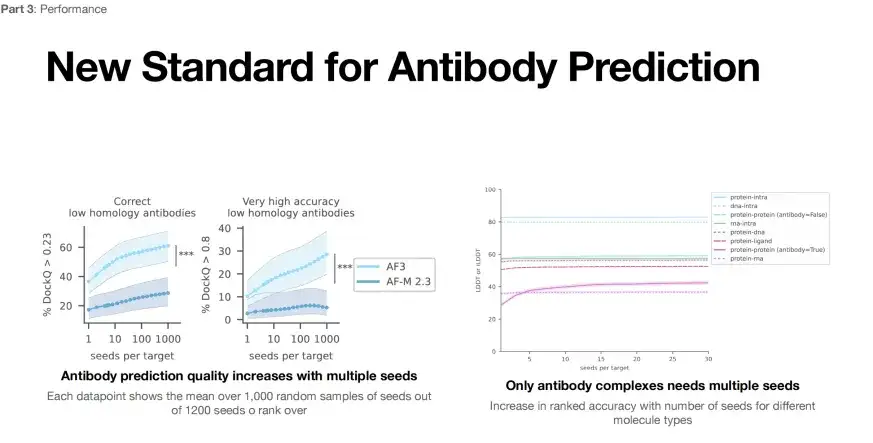
* Left: Antibody structure prediction, each data point represents the average score of 1,000 seeds randomly selected from 1,200 seeds
* Right: When the evaluation index DockQ is greater than 0.23, it can be considered that the structural accuracy is yet to be verified; when DockQ exceeds 0.8, the structural prediction is highly accurate
Furthermore, if measured by a more stringent standard (DockQ>0.8), the success rate of a single run may be as low as 10%, and by increasing the number of runs to 1,000, the success rate can be increased to 30%.This shows that we can improve the success rate of antibody antigen structure prediction by increasing the number of AlphaFold 3 runs (seeds per target).
However, as shown on the right side of the above figure, AlphaFold 3 can only improve the success rate by increasing the number of runs when predicting the structure of protein-protein complexes. This shows that the applicability of AlphaFold 3 for predicting other types of complex structures also needs to be further optimized.
AlphaFold 3 enables covalent modification prediction
As shown in the figure below, AlphaFold 3 also demonstrates excellent structural prediction capabilities in terms of modification prediction.The success rate can reach about 80%, 60%, and 40%. For researchers engaged in covalent modifications, AlphaFold 3 is undoubtedly a powerful tool.
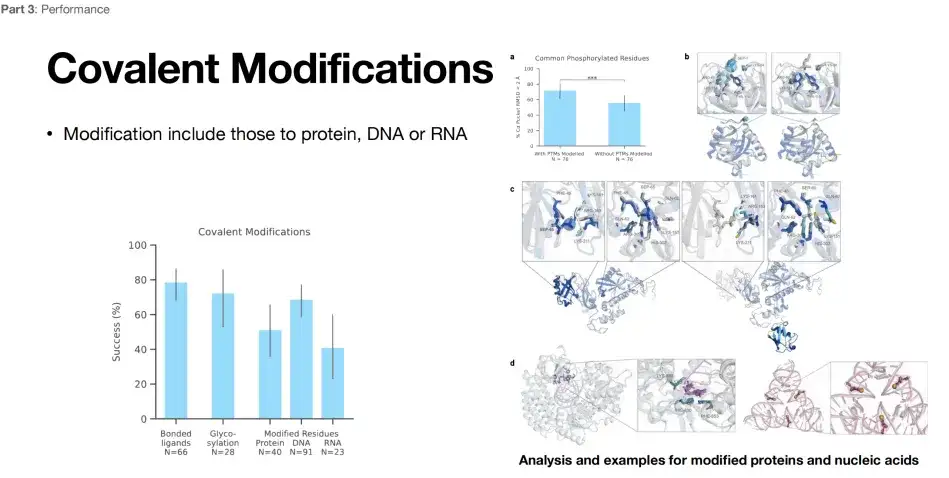
Limitations of AlphaFold 3 in RNA structure prediction
Currently, RNA structure prediction is still difficult.As shown in the figure below, compared with the RoseTTAFoId2NA model, the prediction performance of AlphaFold 3 has been greatly improved. However, when predicting the CASP15 RNA structure, the accuracy of AlphaFold 3 is lower than that of the Alchemy_RNA2 (has human input) model.
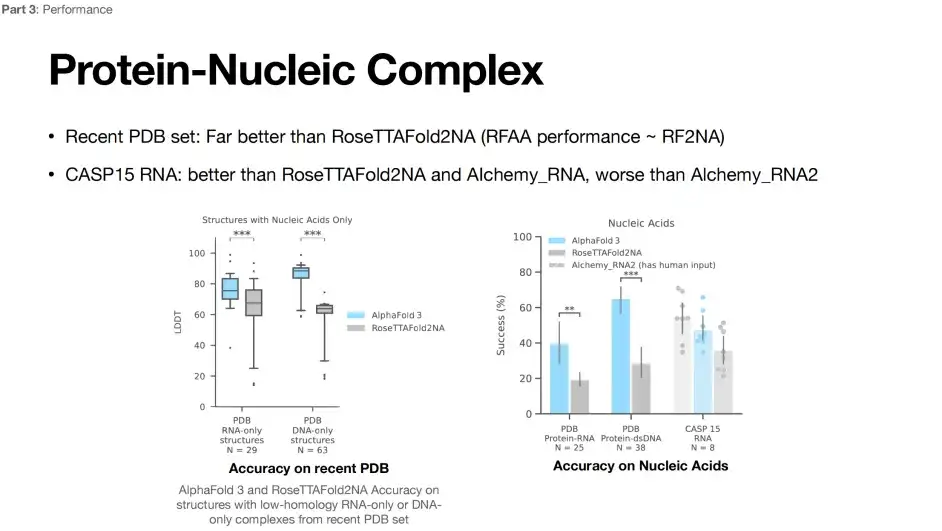
Comparing the pros and cons of AlphaFold 3 on different tasks
By analyzing the training curve of AlphaFold 3, we can clearly see the performance of the model on different tasks. The higher the LDDT index, the better.As shown in the figure below, the model performs best in predicting intra ligand structures; it also shows high accuracy in predicting intra protein structures; the model also performs well in intra DNA predictions, thanks to the stable double helix structure of DNA; in contrast, the model performs poorly on intra RNA structures.
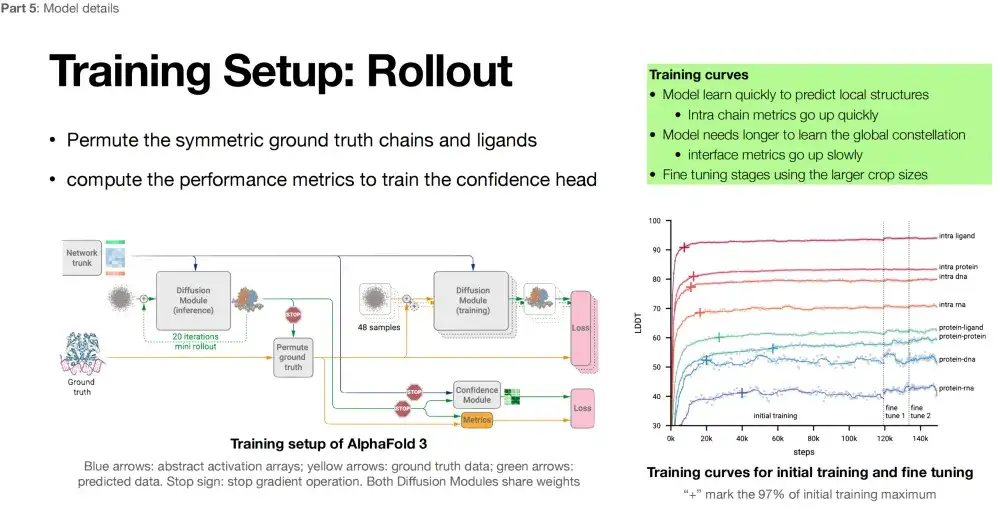
Turning to the field of complex prediction, the model performed best in protein-ligand complex structure prediction, followed by protein-protein complex prediction. In protein-DNA complex prediction, the model performance declined, and protein-RNA complex prediction performed the worst. This result also reflects the difficulty of RNA structure prediction. RNA structure data is scarce, and the structure is dynamic and flexible, which is one of the current challenges in the field of structural biology.
In addition, when researchers used AlphaFold 3 for structure prediction,The reliability of the prediction results can also be evaluated through the PAE table.
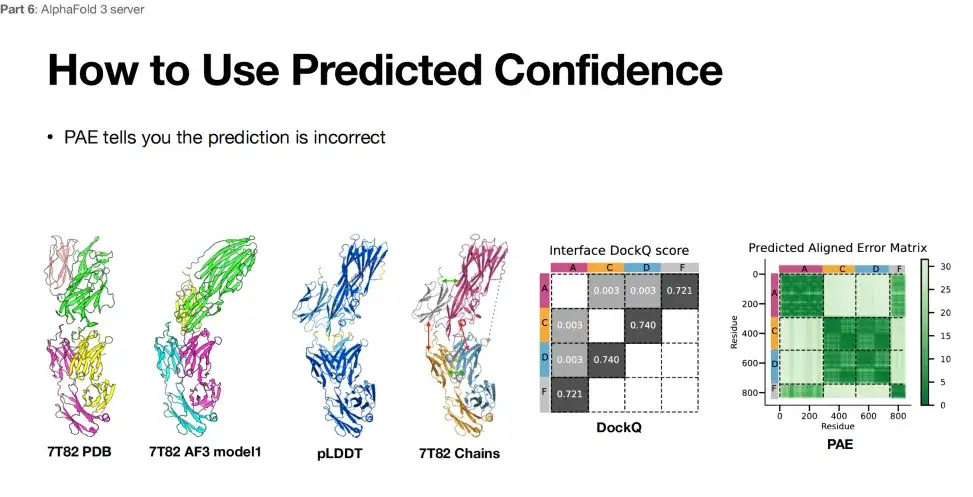
AlphaFold 3 isn’t perfect
AlphaFold 3 is not perfect. For example, it may find the wrong chirality.If an abnormal situation occurs during the operation, it is recommended to run it multiple times to verify the stability of the results. Secondly, AlphaFold 3 also has limitations in protein dynamic prediction, which may be due to the lack of structural data and the inability to grasp the multidimensional conformational information of proteins.
*If an object is different from its mirror image, it is called "chiral" and its mirror image cannot be superimposed on the original object, just like the left and right hands are mirror images of each other and cannot be superimposed.
In addition, AlphaFold 3 also has a common problem in generative models, namely hallucination.As shown in the protein structure prediction results below, only the gray area on the left side of the protein structure can be resolved, and the rest of the part may be in an unfolded state due to insufficient electron density. The middle figure is the result of AlphaFold 2 predicting the protein. The blue area is considered to be in a folded state, and the other "ribbon" parts are considered to be unfolded. The predicted structure is relatively reasonable. The right side is the prediction result of AlphaFold 3, which tends to fold all possible foldable areas. The structure seems reasonable, but in reality, most of the above areas are not actually folded.Therefore, AlphaFold 3’s illusion tends to predict proteins as folded rather than retaining their possible unfolded state.
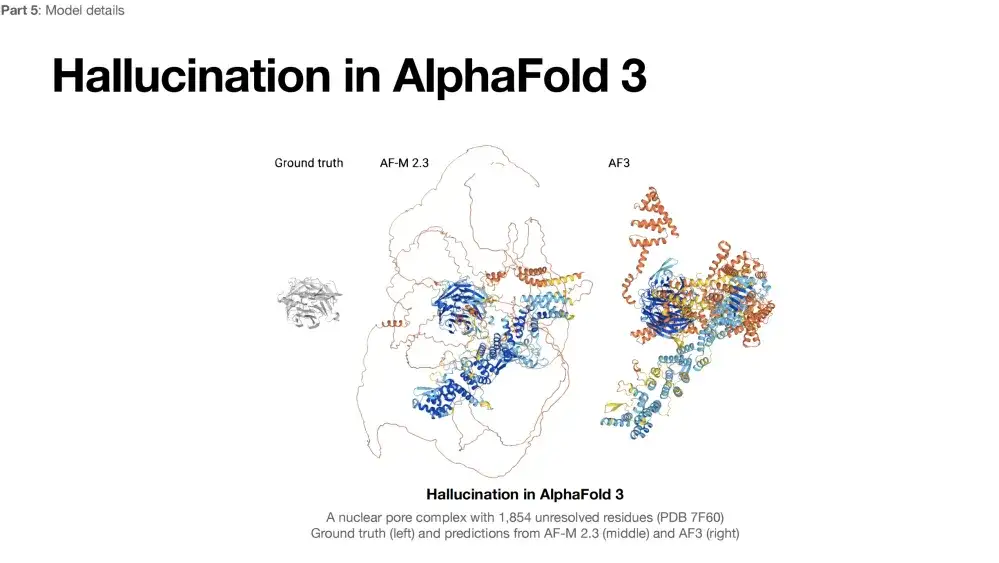
To address the hallucination problem of AlphaFold 3,The researchers chose a direct and effective method: since the results predicted by AlphaFold 2 are relatively reasonable, the results predicted by AlphaFold 2 are included in the training data set of AlphaFold 3 to enhance the training effect of the model. However, this method has a limitation: if there are errors in the prediction of AlphaFold 2 itself, it may affect the prediction quality of AlphaFold 3, unless other data sources can be introduced to further optimize the model.
In addition, when 256x OLA is submitted as input to AlphaFold 3, the predicted result shows a bilayer-like structure, as shown in the figure below.This structure is not expected or typical.
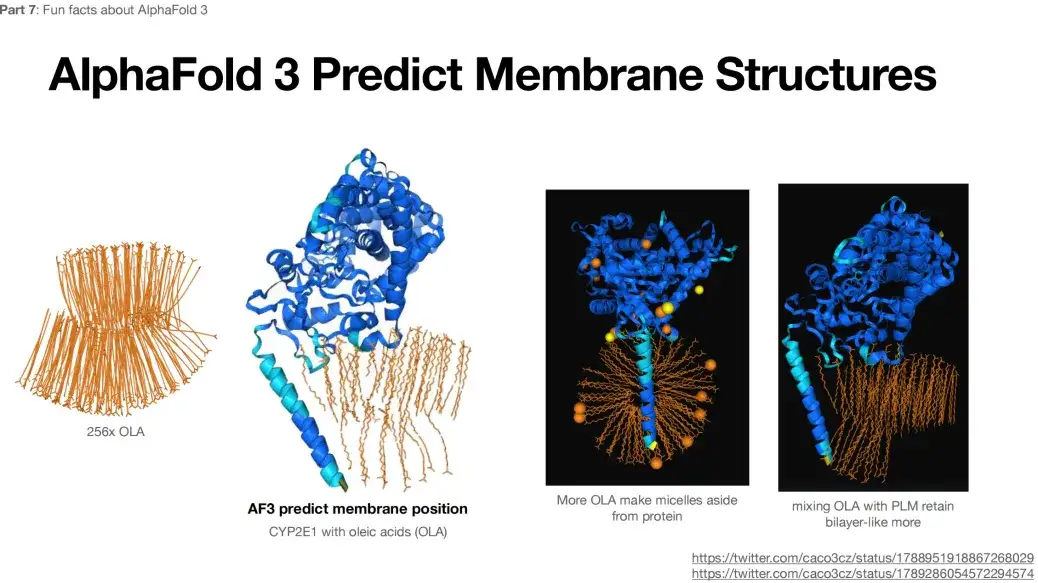
In addition, AlphaFold 3 is not accurate in predicting the structures of RNA and DNA.As shown in the figure below, even bizarre complementary pairings appear when predicting RNA structure, such as G:G, G:A, etc.
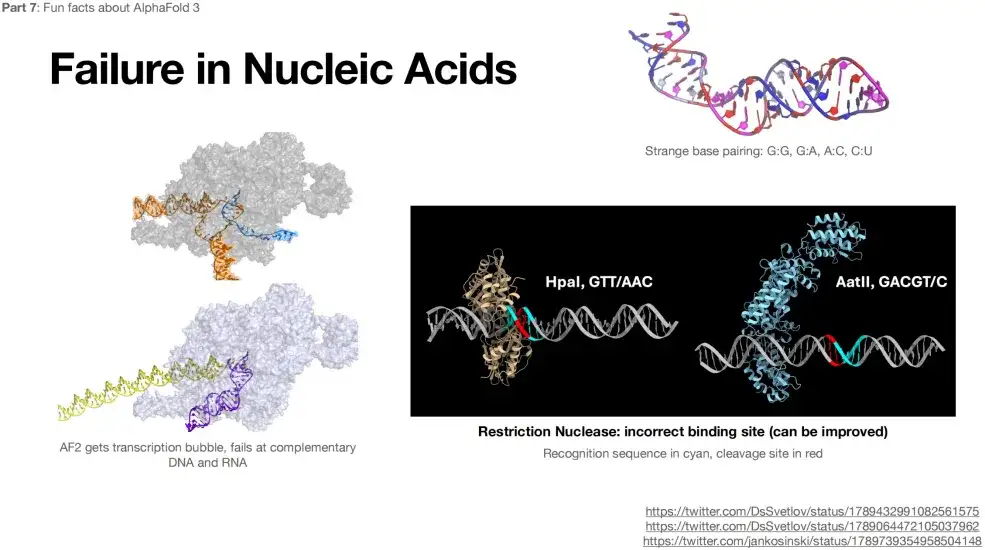
Limitations of use of AlphaFold 3
Under the premise of satisfying the non-high confidentiality of data, everyone can access AlphaFold 3 through the website provided by Google. However, the platform also has some usage restrictions. As shown in the figure below, in terms of protein modification, AlphaFold 3 currently only supports limited types of modifications at 3 specific positions, a total of 23 types, DNA modification only supports 9 types, RNA modification only supports 15 types, metal ions only support 10 different metals, and ligands are limited to 14 small molecules.
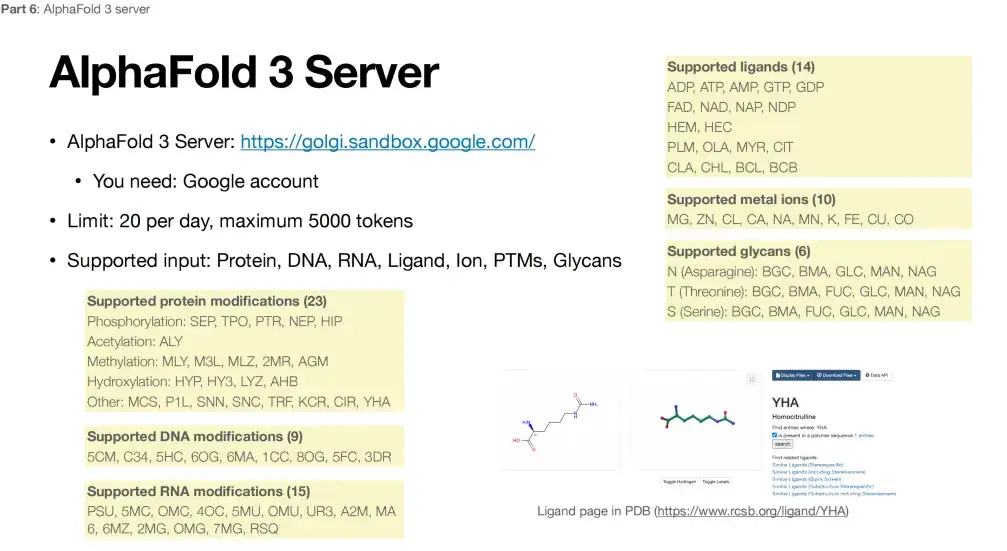
Therefore, with the specific limitations mentioned above, AlphaFold 3 may not be able to handle most research and reactions, and may have to wait until it is truly open source.
In summary, AlphaFold 3 has made significant achievements in expanding its prediction scope, surpassing existing AI models, but its performance on specific tasks still needs to be improved, especially in the prediction of fine structures.Therefore, although AlphaFold 3 has made significant progress, continued research and efforts are still needed to fully solve certain complex problems.
About Zhong Bo Zitao
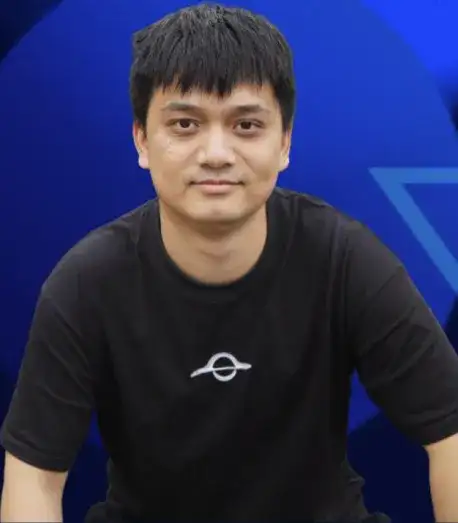
Zhong Bozitao is currently a doctoral student in artificial intelligence at Shanghai Jiao Tong University. His main research areas include high-throughput protein structure and function prediction, protein conformation generation, etc. He has published more than 20 papers since 2019, and published the results of high-throughput AlphaFold structure prediction and analysis of the association between deep-sea proteome and metabolic pathways in Nature Communications. He has won the gold medal of the International Genetically Engineered Machine Competition (iGEM) three times and served as a judge for the competition many times.
Google Scholar:
https://scholar.google.com/cita