Professor Hong Liang of Shanghai Jiao Tong University: If AI Really Wants to Break Through the Engineering Field, It Must Achieve Engineering Results That Existing Human Experts Cannot Achieve
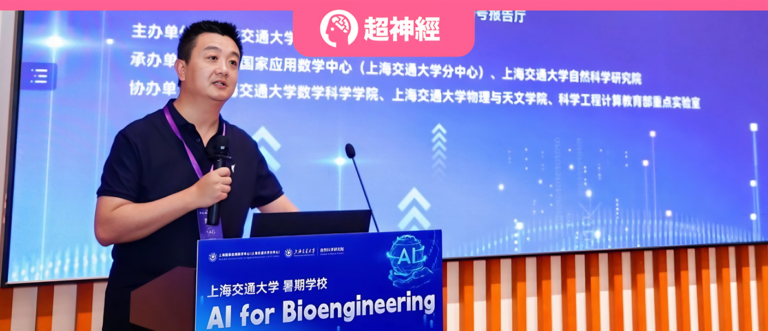
Recently, the AI for Bioengineering Summer School of Shanghai Jiao Tong University came to a successful conclusion. More than 100 industry experts, business representatives and outstanding young scholars from enterprises, research institutions and universities gathered together to engage in a fierce exchange of ideas around the application of AI in the field of bioengineering.
in,Hong Liang, distinguished professor of Natural Science Research, School of Physics and Astronomy, and School of Pharmacy at Shanghai Jiao Tong University, shared in a simple and easy-to-understand manner the application of AI in scientific research, especially in protein design, as well as his outlook on the future development of AI for Science.
Excerpts of key viewpoints:
* To truly implement AI for Science, one must first define the scientific problem and then come up with an artificial intelligence solution.
* AI can achieve the transformation of hundreds of amino acid sequences, and still maintain good activity and high positive rate. AI is already far better than human experts in this type of sequence generation task. * The field of protein engineering has the most negative data. AI can combine negative and positive sites, expanding the imagination space of protein engineering, which goes beyond the rational design scope of professional enzyme engineers. AI has basically replaced the old path of physical calculation. * If artificial intelligence wants to break through an engineering field, it is not simply to create an assistant for scientists and do basic work such as collecting literature, but to do what human experts cannot do. * In the next three years, in the fields of protein design, drug development, disease diagnosis, new target discovery, chemical synthesis path design, and material design, general artificial intelligence in professional fields will bring about a clear paradigm change, transforming the scientific discovery model that relied on sporadic trial and error of the human brain in the past into an AI large model automated standard design model.
HyperAI has compiled and summarized Professor Hong Liang’s wonderful sharing without violating the original intention. The following is a transcript of the highlights of the speech.
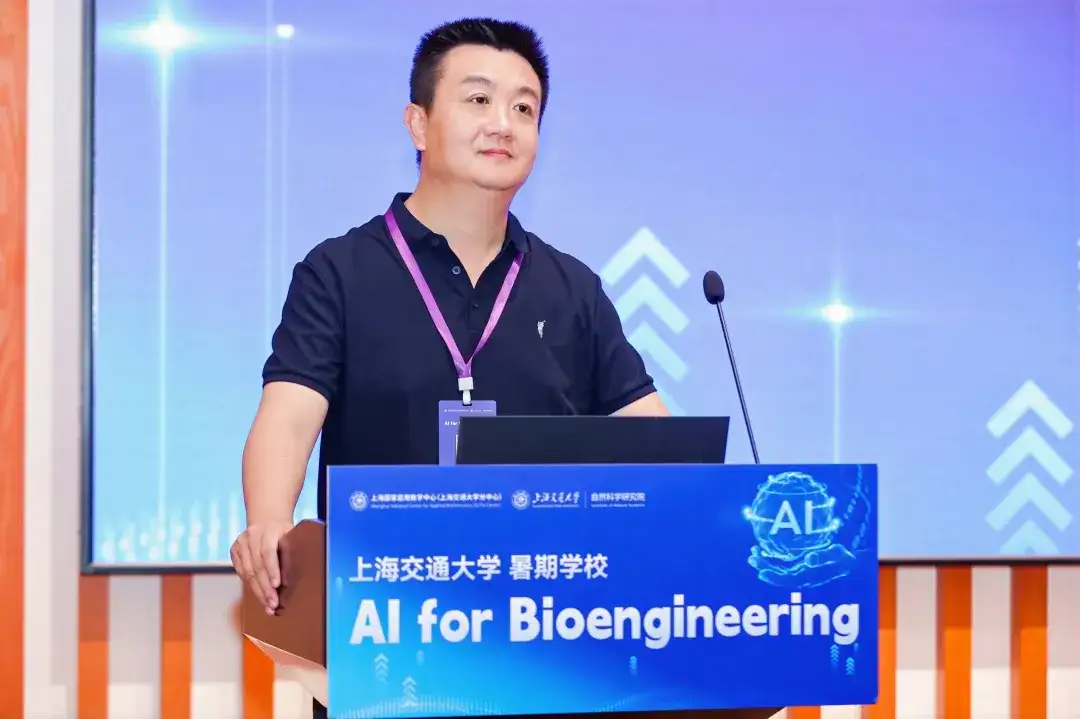
AI Arts Students vs. AI Science Students
Professor Hong Liang introduced the application of AI in life (AI for Life) and scientific research (AI for Science) from the perspectives of AI liberal arts students and AI science students respectively.
AI Liberal Arts Students: Personal Assistants in Life
Regarding AI for Life, Professor Hong Liang believes that current AI has become a personal assistant in people’s lives, helping people reduce the burden of repetitive, creative and unscientific work.Its characteristics are that the scale of data available for training is already very large, and the generated results do not require high accuracy. Therefore, it has strong cross-domain generalization capabilities and can build large general-domain models.
Then, he used specific cases such as AI text generation, AI image generation, AI video generation, etc., combined with the currently popular big models, to vividly describe the application of AI in life.
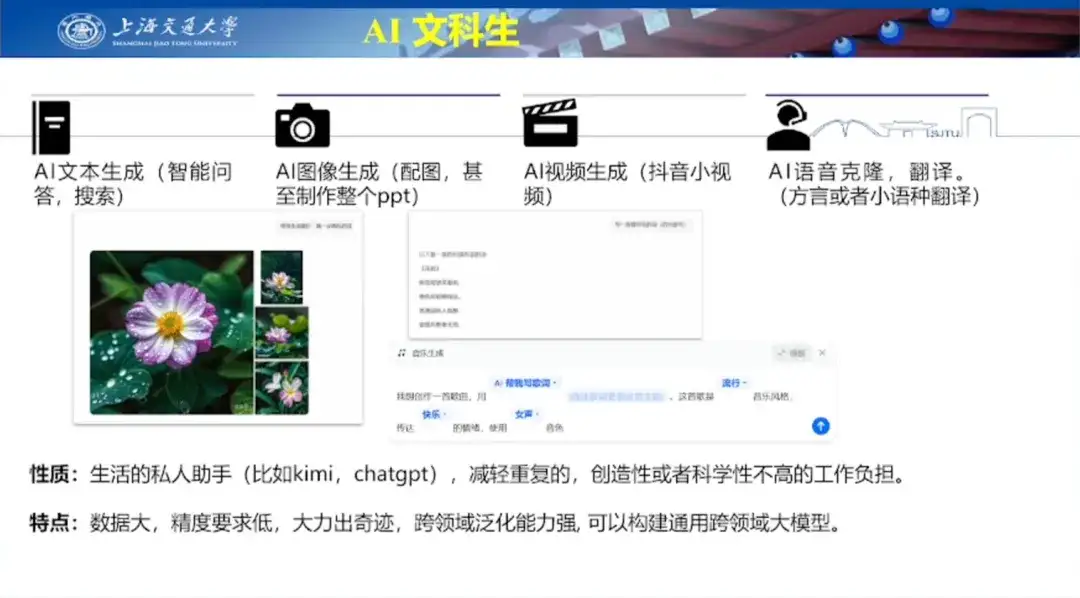
In terms of AI text generation, Professor Hong Liang demonstrated ChatGPT's poetry creation ability by taking the example of writing a poem for his wife on Valentine's Day. He also shared an example of using Wenxinyiyan to help his son in elementary school write a self-review, demonstrating Wenxinyiyan's text creation ability.
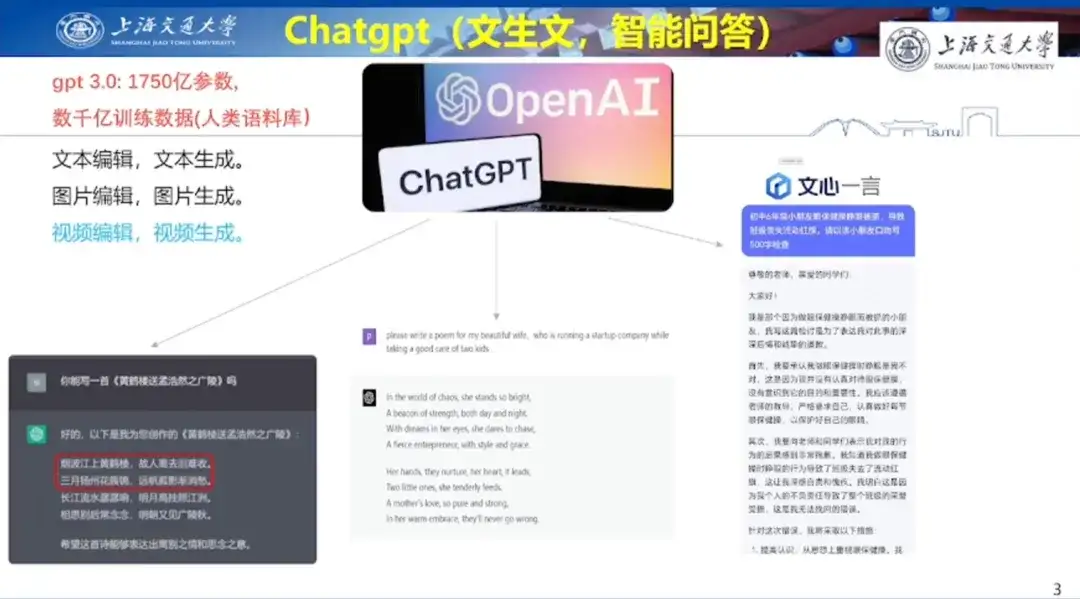
In terms of AI image generation, Professor Hong Liang demonstrated Baidu Wenxin Yiyan, Adobe firefly and Midjourney, respectively, and the different effects generated based on the same prompt words, as shown in the figure below.
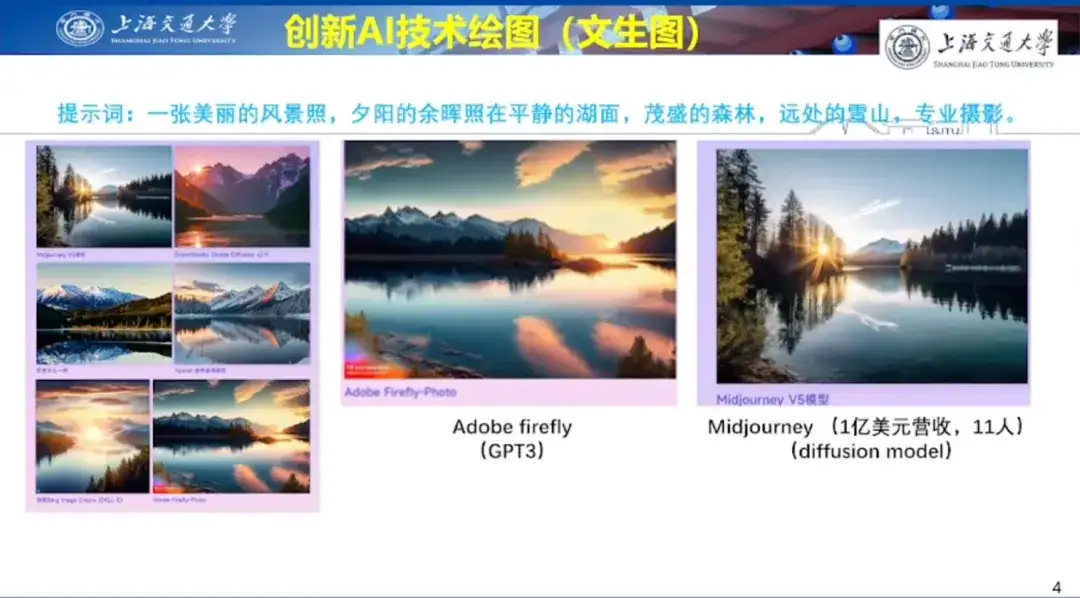
In terms of AI video generation, Professor Hong Liang demonstrated the powerful capabilities of the popular Sora in video generation. He took a video generated by Sora of a fashionable lady walking on the streets of Tokyo as an example, praising the one-shot technology and the detailed processing of the facial pores shown in the video.
At the same time, he also agreed with the evaluation made by industry insiders that "Sora is a data-driven physics engine", and believed that Vincent Video has been of great help to content creators on platforms such as TikTok.
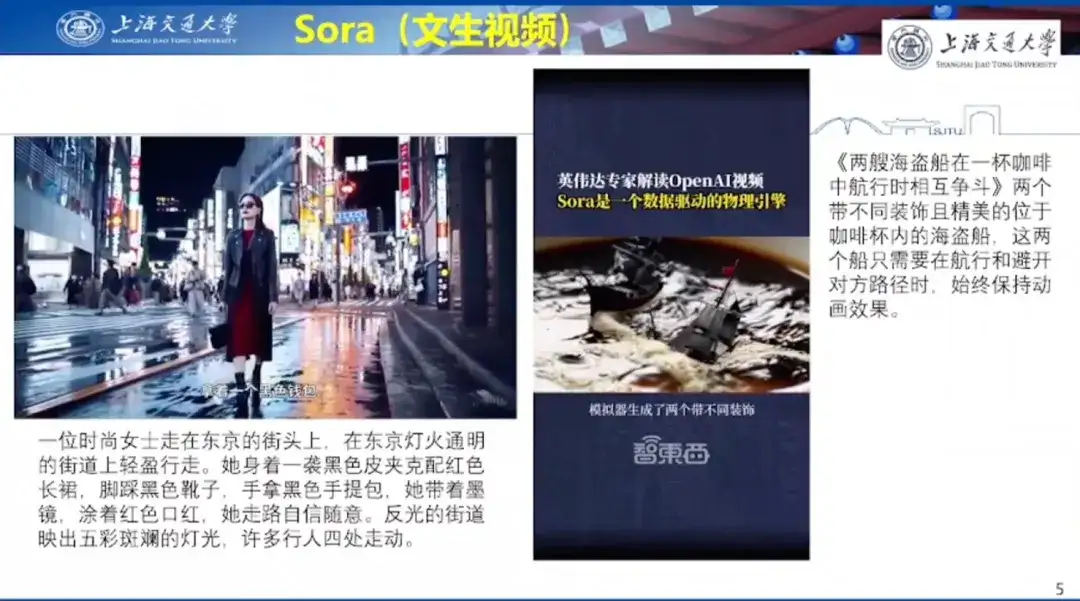
AI Science Students: Scientists who solve a class of scientific problems
For AI science students, that is, AI for Science or AI for Engineering, Professor Hong Liang believes "It is a scientist who solves a type of scientific problem. In essence, it is to create a scientist for different fields such as biomedicine, material chemistry, nuclear physics, etc."The core difficulty is that the accuracy requirements are very high, and there is relatively little functional data available for training, so only proprietary AI models can be built.
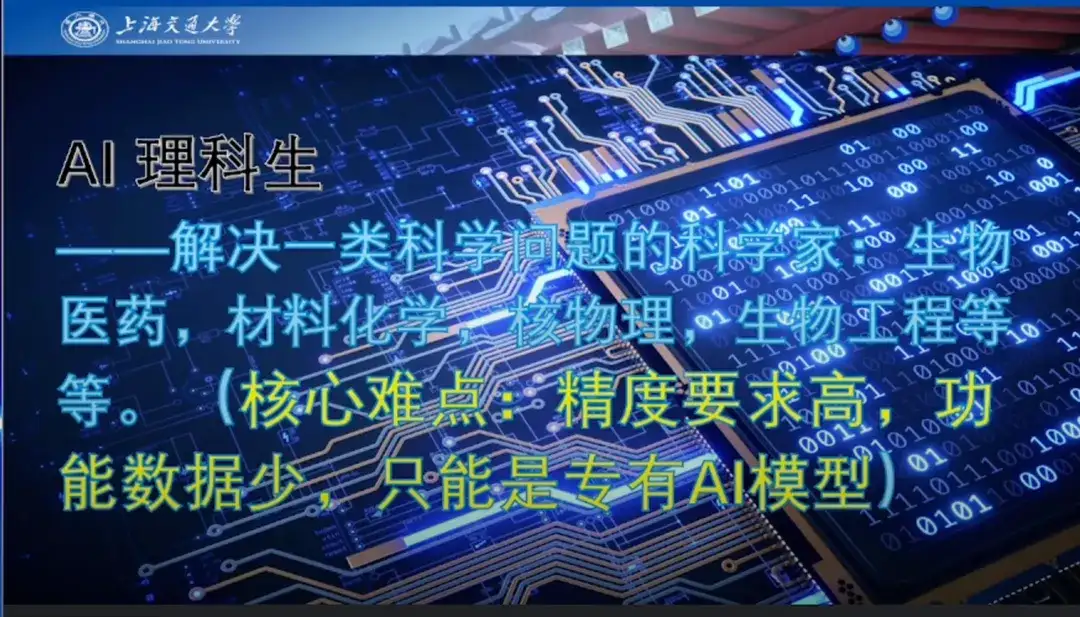
In order to help everyone better understand the application of AI for Science, Professor Hong Liang conducted an in-depth analysis based on specific cases such as AI for biology/medicine, AI for materials/chemistry, and AI for controlled nuclear fusion.
First is the case of AI for the biological field.Professor Hong Liang said, "Protein 3D structure prediction is the most important starting point for AI for Science." He said that protein structure prediction has troubled scientists for nearly 50 years. "Before DeepMind released the AlphaFold model, scientists generally believed that using AI to predict protein structure was just a game."
From AlphaFold 1 to AlphaFold 3, AI has demonstrated its prowess in predicting protein three-dimensional structures. In particular, the accuracy of AlphaFold 3 has been significantly improved compared to many previous specialized tools, such as protein-ligand interaction, protein-nucleic acid interaction, and antibody-antigen prediction.
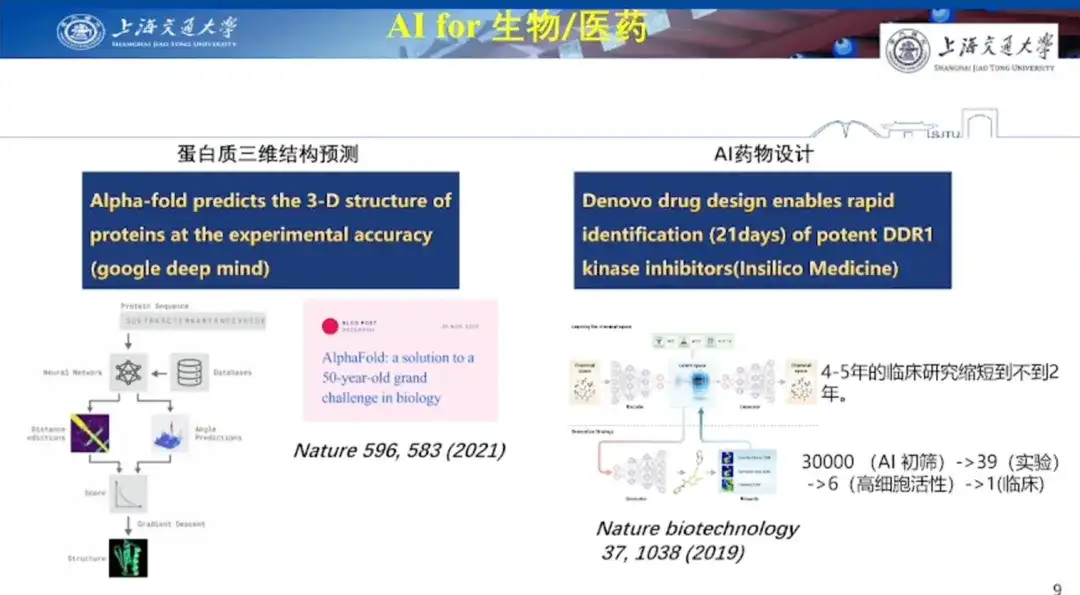
The second is the case of AI drug design.Professor Hong Liang said that AI drug design is relatively difficult because the application not only has to solve problems at the molecular level, but also faces the challenges of subsequent clinical trials. Traditional drug discovery methods such as high-throughput screening test thousands of small molecules and only obtain a small number of lead compounds, of which only one-tenth or even less can pass clinical trials.
Research results published in Nature Biotechnology in 2019 revealed the great potential of AI in drug design. Using reinforcement learning (GENTRL), researchers discovered an effective inhibitor of discoidin domain receptor 1 (DDR1), a kinase target associated with fibrotic diseases, within 21 days. The researchers used AI technology to initially screen out 30,000 molecules, and then conducted 39 cell experiments through various screening methods, found 6 with high cell activity, and finally promoted 1 to clinical trials.
In addition, Professor Hong Liang also cited cases of AI for materials/chemistry.He believes that"AI for materials, especially chemical materials, is a difficult thing to implement."However, unlike natural language, human language, and DNA sequences, materials do not have discrete tokens, because the essence of materials is a three-dimensional structure problem. When building a large model, it is necessary to combine DFT calculations, automated experiments, and AI recursion to promote the synthesis of specific inorganic compounds. For example, the DeepMind materials team launched the deep learning-based material exploration graph network (GNoME) in 2023. In the test task, the A-Lab laboratory successfully synthesized 41 of the 58 predicted materials within 17 days, which was only possible in the past 10 years or even longer.
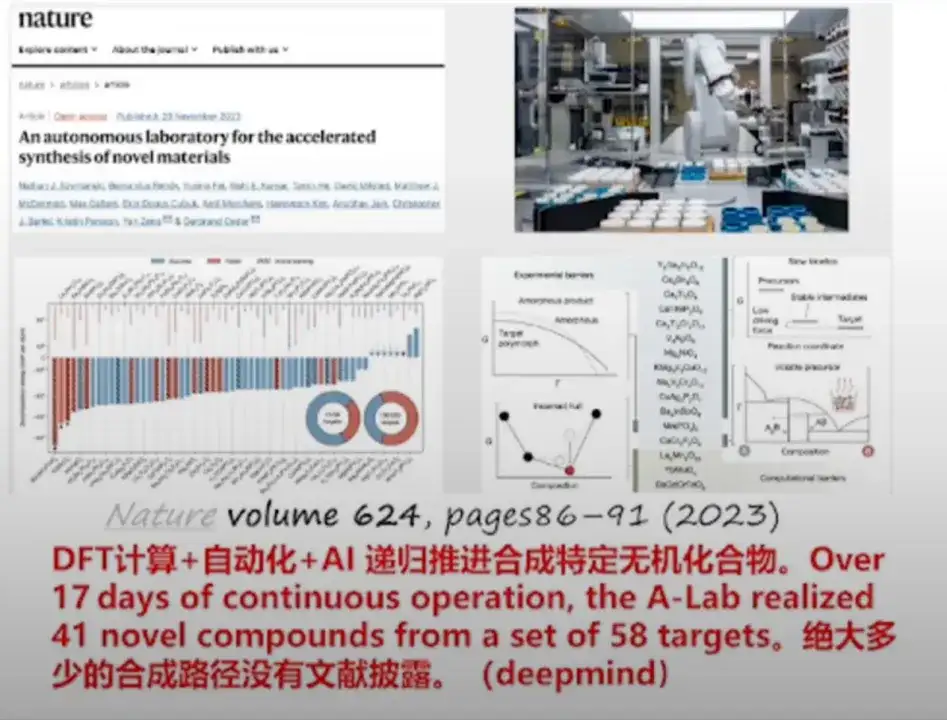
Finally, Professor Hong Liang cited cases such as AI for controlled nuclear fusion, and said that the progress in this direction is very gratifying.He pointed out that the main problem with nuclear fusion now is that plasma is very easy to "tear" and escape the strong magnetic field used to constrain it, thus causing the fusion reaction to be interrupted. The Princeton team has developed an AI controller that can predict the potential risk of plasma tearing 300 milliseconds in advance and intervene in time.
As shown in the figure below, researchers have integrated traditional physics-based methods with advanced AI technology to improve the control and understanding of plasma behavior. The following figures a, b, and c reveal the state of plasma in a fusion reactor.
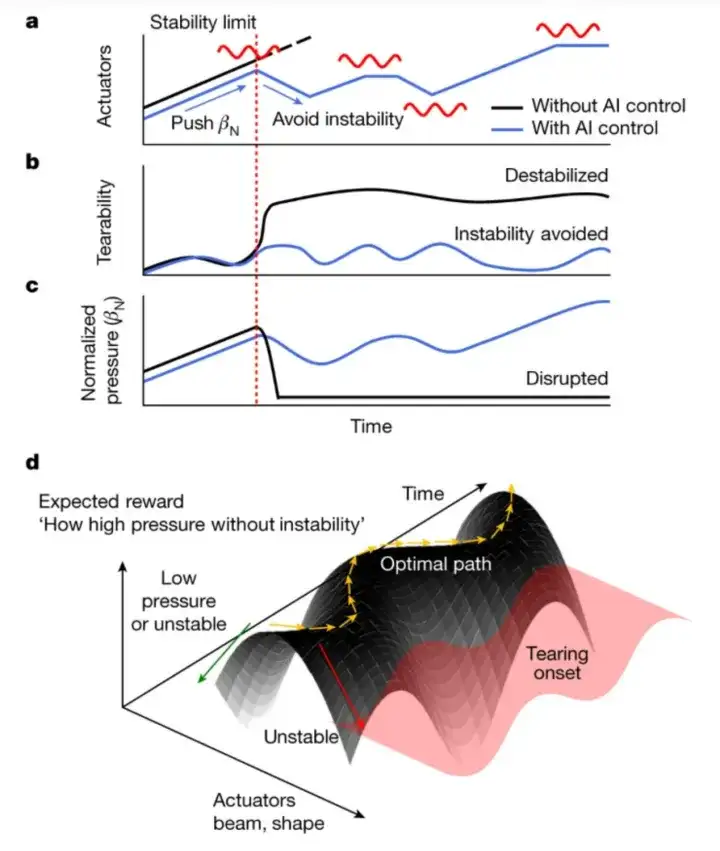
The black line in Figure a shows that when the plasma pressure is increased by increasing the external temperature (such as a neutral particle beam), a stability limit will eventually be reached. When this limit is exceeded, tearing instabilities will be stimulated. Once tearing instabilities are stimulated, the plasma will be rapidly destroyed, which will lead to serious consequences in actual operation, as shown in Figures b and c.
Based on deep neural networks and reinforcement learning, the researchers developed an intelligent control system that can respond to changes in plasma states in real time, predict the future state of plasma, and adjust control actions accordingly, so that the tokamak operation follows the ideal path and avoids tearing instabilities while maintaining high pressure.
Finally, Professor Hong Liang emphasized,"To truly implement AI for Science, one must first define the scientific problem and then come up with an AI solution."
AI for Bioengineering: Solving engineering problems and implementing multi-scenario products
Afterwards, Professor Hong Liang explained the definition and challenges of traditional protein engineering, the application of AI in the field of protein engineering, the team's R&D results and their implementation, and the team's core advantages, further revealing the value of AI for Bioengineering.
Protein engineering: mutating protein sequence sites to meet product application requirements
Professor Hong Liang pointed out that protein engineering refers to mutating 5-20 sites in a protein sequence to optimize its specific properties and realize its application in industry and medicine, thus turning it into a product.
He explained that protein is not only an important component of organisms, but also an indispensable product in people's daily lives. Enzymes, as protein molecules, are widely used in industrial scenarios and have catalytic effects. For example, antibody ADC site-coupled enzymes in the field of innovative drugs, enzymes in laundry detergents, enzyme additives in feed that help animal metabolism, and various enzymes in beauty, food, and bioengineering.
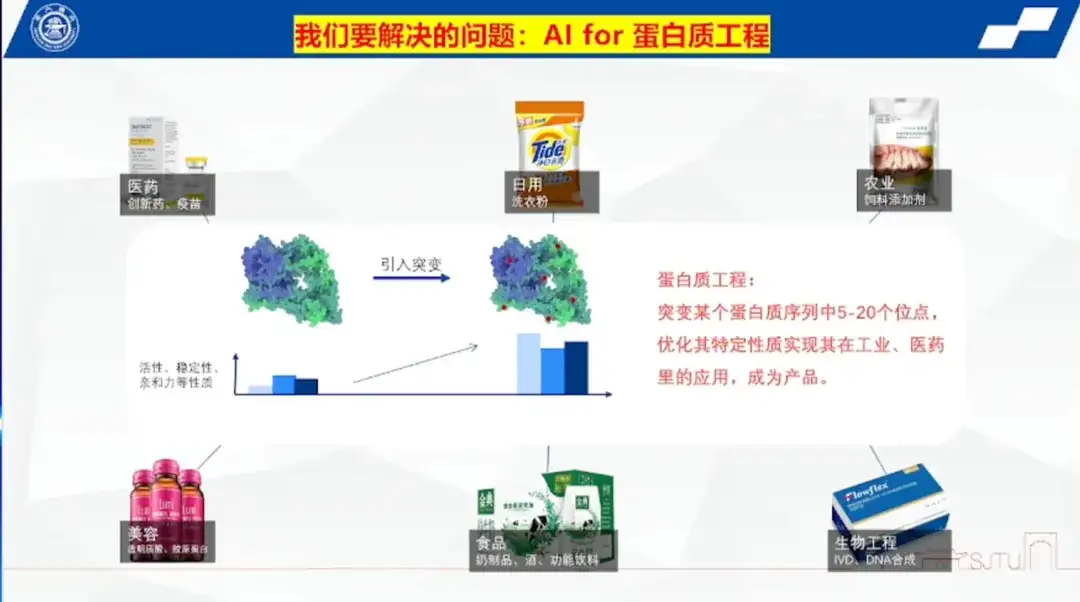
Afterwards, Professor Hong Liang introduced the two most mainstream practices in current protein engineering.
The first is rational design/semi-rational design.Generally, it is necessary to study the protein structure and catalytic mechanism clearly and then modify it according to the mechanism. However, the disadvantage of rational design is that it takes a long time, the sites that need to be modified are mainly concentrated around the active pocket, the scope of design is relatively limited, and the scope of thinking is also relatively restricted.
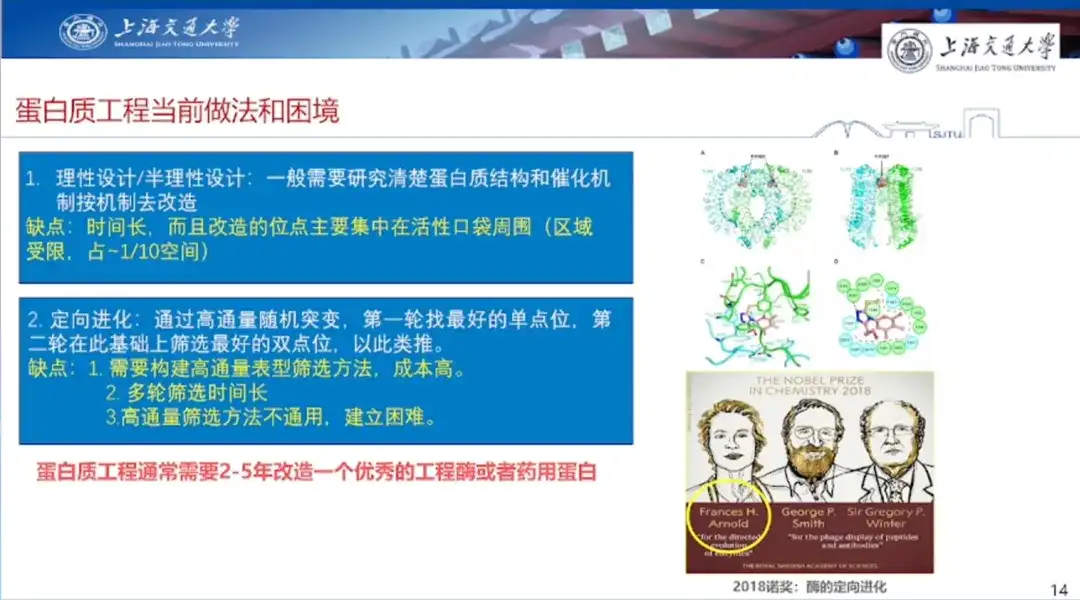
The second is directed evolution.That is, breaking the human thinking paradigm, through high-throughput screening, high-throughput single-site random mutation on the basis of wild, the first round to find the best single-site mutant, the second round based on this screening of the best double site, and so on. Its advantage is that it does not rely on past experience, "just money can be done"; the disadvantage is that it needs to build a high-throughput phenotypic screening method, which is costly, multi-round screening time is long, and high-throughput screening methods are not universal and difficult to establish.
Professor Hong Liang introduced the experiment on green fluorescent protein, taking the research paper published in Nature in 2016 as an example. He pointed out that in this experiment, although high-throughput screening can select positive sites, and when researchers mutate sites individually, the properties of the protein can be improved, but if multiple mutation sites are combined, the synthetic protein will lose its activity.
He said,"How to find excellent mutation sites in the vast phase space and combine them into excellent multi-site mutants to realize their application value is the challenge currently facing protein engineering."
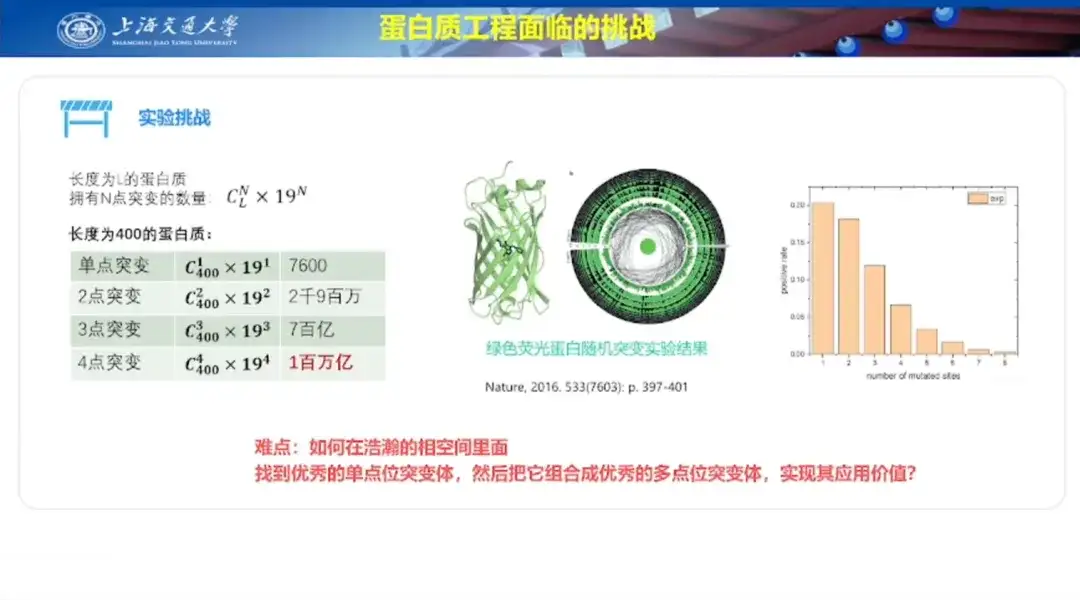
General artificial intelligence technology for protein engineering: end-to-end function-oriented sequence design
"If artificial intelligence wants to make a breakthrough in an engineering field, it is not simply to build an assistant to scientists and perform basic work such as collecting literature, but to do things that human experts cannot do."Based on this, Professor Hong Liang's team began exploring proprietary models in the field of protein engineering in 2021, designing functional sequences end-to-end.
The research team compiled a database of hundreds of millions of complete protein sequences based on all known proteins in nature, and built a general artificial intelligence for protein engineering to learn the arrangement and rules of amino acids based on this database.
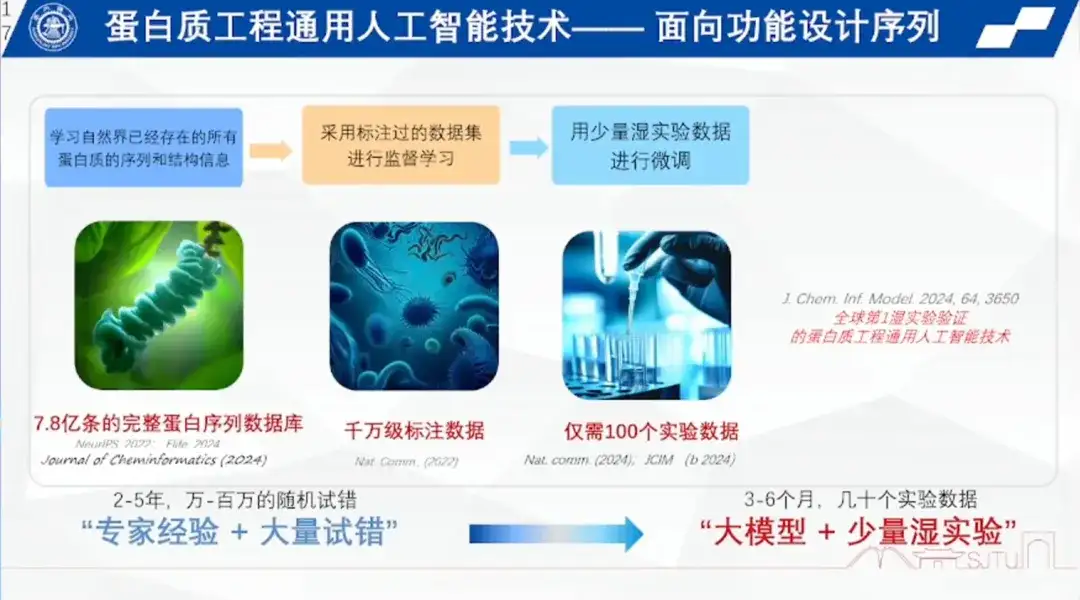
Professor Hong Liang gave a detailed explanation of the application scenarios of general artificial intelligence technology in protein engineering through five real-world practical application cases, including collaborating with Professor Liu Jia from ShanghaiTech University to improve the thermal stability of Crisper cas12a, collaborating with Jinsai Pharmaceutical to improve the alkaline resistance of single-domain antibodies, and collaborating with Hanhai New Enzyme to launch enzymatic innovations.
Case 1: Improving the thermal stability of Crisper cas12a
This project was completed by Professor Hong Liang's team and Professor Liu Jia from ShanghaiTech University. Crisper cas12a is composed of 1,300 amino acids. The wild type has good activity, but poor stability. As an in vitro diagnostic kit, it cannot be used at room temperature and has high refrigeration costs. In response to this, the research team conducted three rounds of experiments. In the end, the stability of the mutant reached a continuous rising state, and the ratio of protein activity not lower than the wild type reached 100%.
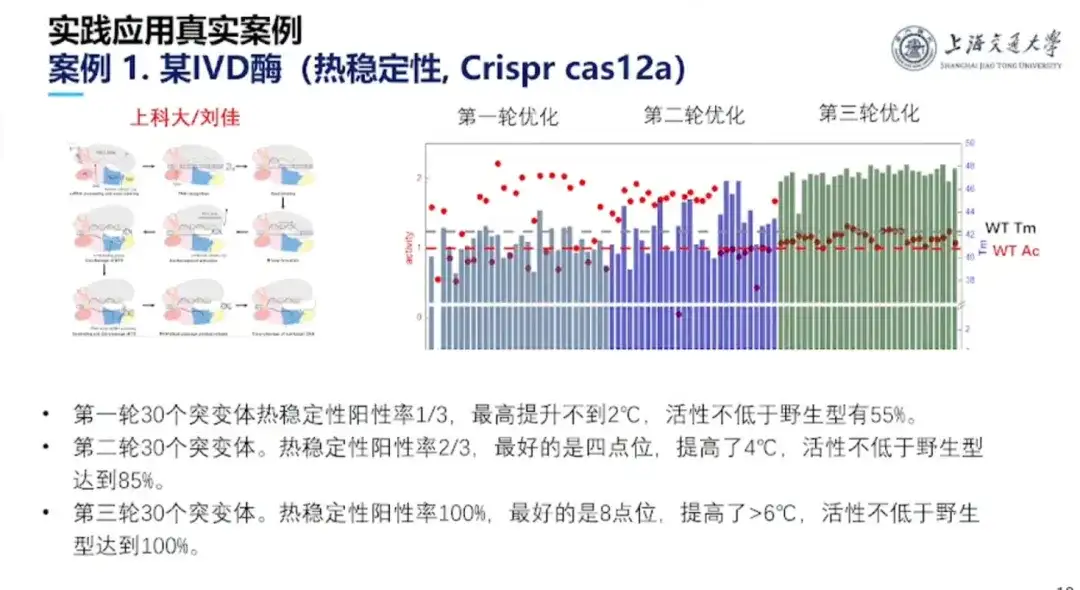
Professor Hong Liang introduced,"The field of protein engineering has the most negative data. AI can combine negative and positive sites, expanding the imagination space of protein engineering. This goes beyond the rational design scope of professional enzyme engineers. AI has basically replaced the old path of physical calculation."
He further introduced the underlying logic of how AI combines protein negative and positive mutation data, which is divided into three steps.
The first step is to build a protein language vocabulary.He compared the process of pre-training protein sequence information to a cloze test, that is, using a model to randomly cover any sequence in a database of hundreds of millions of complete protein sequences, either continuously or discretely, and the model can fill in the covered areas. Repeating this operation multiple times ensures that a model can pre-train hundreds of millions of protein sequences, thereby building a vocabulary of protein language.
The second step is labeling.Such as temperature, pressure, and pH, the research team has tagged tens of millions of them.
The third step is small sample learning.That is, fine-tuning is performed using a small amount of wet experimental data to complete reinforcement learning, thereby solving the small sample problem in bioengineering.
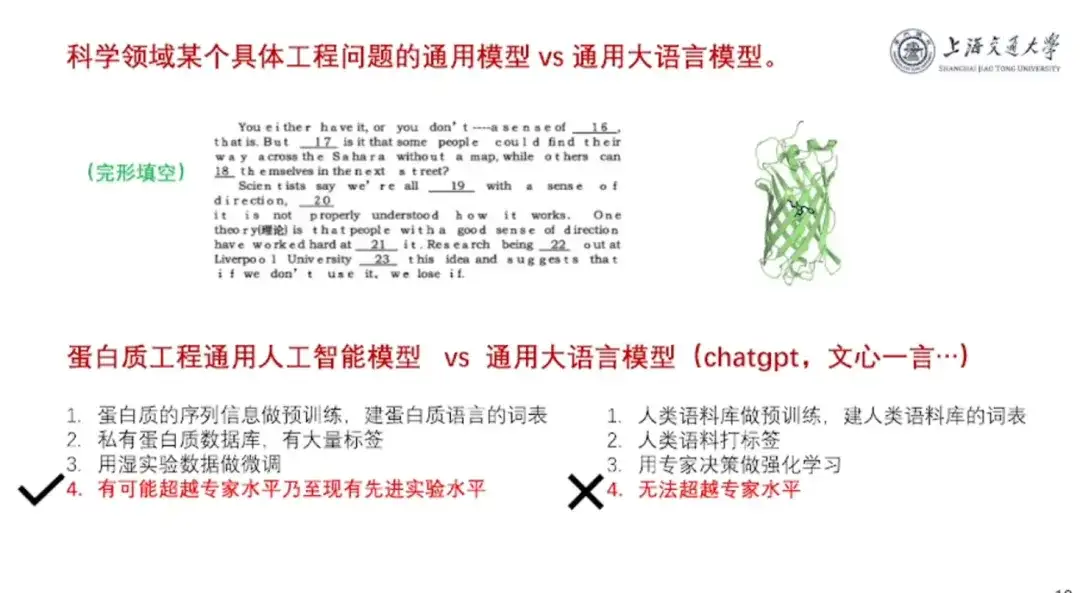
Case 2: Collaboration with Jinsai Pharmaceuticals on the development of extremely alkali-resistant single-domain antibodies
Professor Hong Liang pointed out that Jinsai Pharmaceuticals often purifies growth hormone by screening single-domain antibodies from the alpaca single-domain antibody library and placing them on hydrogen and columns. However, during the purification process, hydrogen and columns will inevitably be contaminated by some impurities and need to be cleaned with strong alkali before they can be used in the next purification experiment. However, organisms are not resistant to strong alkali and there is a risk of corrosion. Therefore, Jinsai Pharmaceuticals hopes to improve the alkali resistance of single-domain antibodies.
In this regard,The research team treated the single-domain antibodies designed after the Pro series large model with 0.5M NaOH for 24 hours and successfully improved the alkaline resistance of the single-domain antibodies.The alkali-resistant protein designed in this project has achieved 5,000 L mass production.It is the first protein product made using a large model that has been industrialized.
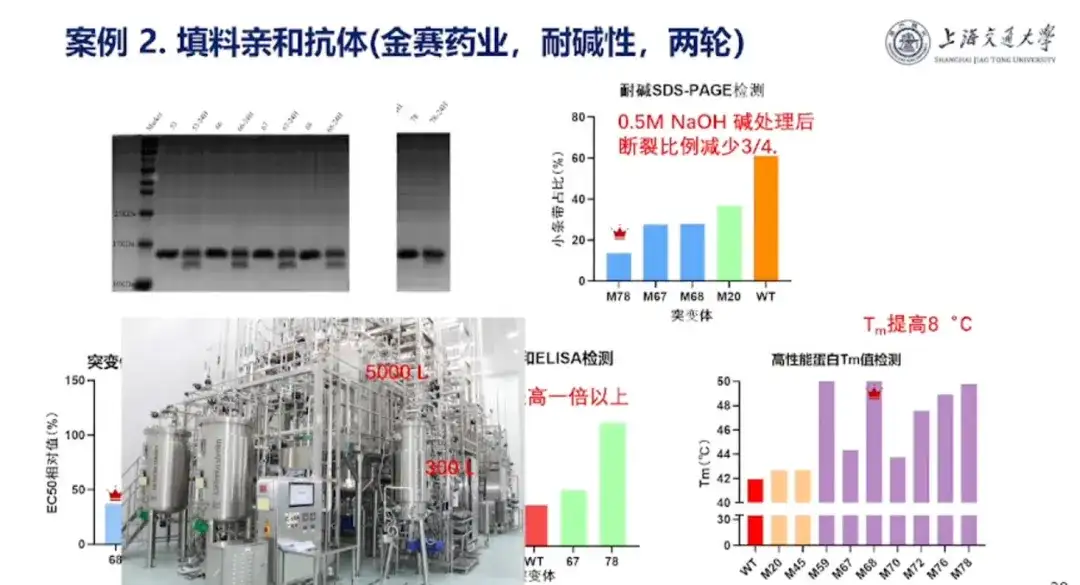
Case 3: Improving the selectivity, activity and yield of glycosyltransferases through enzyme innovation
The core material for screening acute pancreatitis and sialadenitis is maltoheptaglycoside, which has a very complex structure and a high cost for chemical production. It is sold in China for hundreds of thousands of yuan per kilogram. In response to this, Professor Hong Liang's team and Hanhai New Enzyme jointly launched an enzyme innovation, which uses a glycosyltransferase to produce maltoheptaglycoside. The research team needs to improve four indicators, namely, enhancing the transglycosylation reaction, enhancing the reaction specificity, reducing the hydrolysis activity and increasing the yield.
Through two rounds of transformation experiments, the researchers improved the BUG index of 80 mutants, increased the total transglycosylation activity by 8 times, increased the purity of the target product from 80 to 95, reduced the hydrolysis activity index to 10, and doubled the P3 yield.This product has already been put into production on a 1,000-kilogram production line in Yichang, Hubei, significantly reducing production costs.
Case 4: Antibody affinity test based on small sample learning in single-blind test
"AI for Science needs to solve the problem of small samples. Simply publishing articles is of little practical use." Professor Hong Liang elaborated on this through a demo completed in cooperation with an antibody pharmaceutical company.
Professor Hong Liang introduced that this is a ScFv antibody with a total length of 245 amino acids and 21 mutation sites, and its possible mutation sequences exceed 10 million. However, the partner only provided 33 known mutant affinity data and 14 new sequence affinity data for prediction of unknown. Based on small sample learning, the team achieved a correlation coefficient of 0.65 for the single-blind test.
"Whether it is biomedicine or synthetic biology, the final implementation still has to solve the cost issue, that is, the yield must be high."Professor Hong Liang introduced that "the team's AI protein design model learns from nature, and the yield of the mutants it recommends will not be too bad. Many of them are comparable to the wild type, and there are even mutants with higher expression than the wild type."
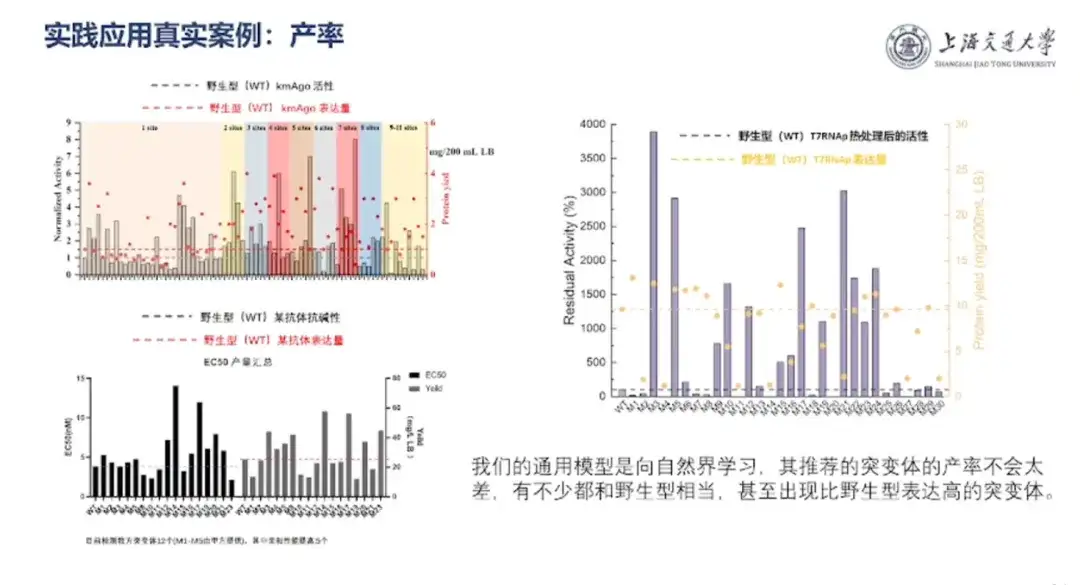
Talking about the difference between protein design in the human brain and the AI protein design model, Professor Hong Liang pointed out that the key difference is that humans like to summarize experience, but human experience is generally low-dimensional, such as protein extraction sequence and low-dimensional features in structure. Although these features are related to protein function, they are not complete factors that determine the latter, and are difficult to predict quantitatively and qualitatively.The AI protein design model can use high-dimensional features to describe the sequence and structure of proteins, and predict target functions more accurately, quantitatively and quickly.
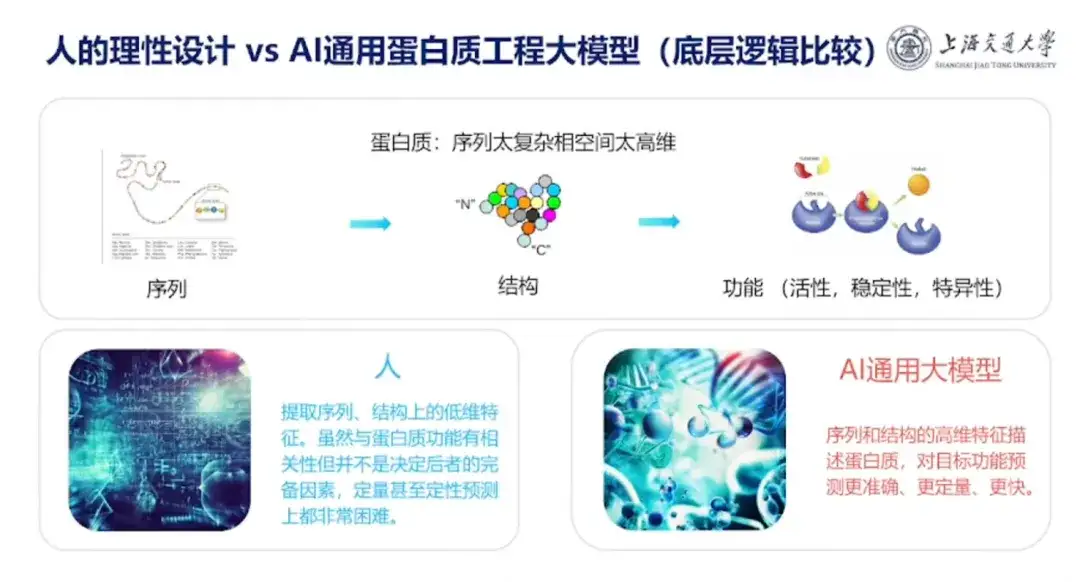
Case 5: De novo protein sequence design
To further illustrate this issue, Professor Hong Liang shared a result of his research group Cell Discovery. He said that this is the largest protein sequence reported to be obtained through de novo design, a gene editing enzyme with 6 domains and more than 700 amino acids.
There are only more than 600 known editing enzymes in nature, and the research team used this as a template to generate 27 new sequences. Compared with nature, the sequence similarity is all lower than 65%, and the lowest is 49%. In other words, the research team modified more than 300 of the more than 700 amino acid sequences, of which 23 are active, 2/3 are more active than wild types, and the highest wild type is 8.6 times.
Professor Hong Liang said, "The AI protein design model can achieve the modification of 300 amino acid sequences while maintaining good activity and a high positive rate. AI is already far superior to human experts in this type of sequence generation task."
In addition, Professor Hong Liang also shared his understanding of artificial intelligence:"Artificial intelligence is a mapping from y to x, where x is the input feature and y is the desired result, such as protein stability and activity. Artificial intelligence is now doing a high-dimensional fitting."
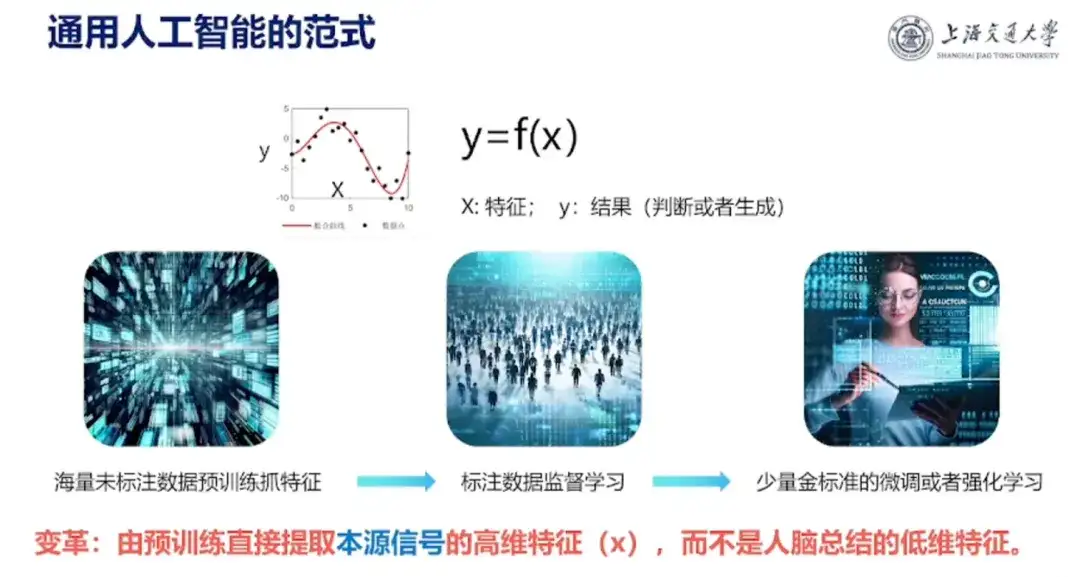
AI protein design big model achieves huge improvement in productivity
Professor Hong Liang demonstrated the AI protein design model built by the team and introduced that "researchers input a sequence into the internal software, and the platform will select 30 or 50 sequences that conform to the laws of nature for experiments, and then enter the small sample learning phase, which is to fine-tune the AI model to the indicators required by the researchers. Finally, the dominant mutants are produced."
It is worth mentioning that currently there are only two researchers in the team who focus on protein design, one in the biomedicine field and the other in the synthetic biology field, but the team is running more than 40 projects at the same time.This also confirms what Professor Hong said: "Once AI has the ability to break through the underlying engineering, it will unleash huge productivity."
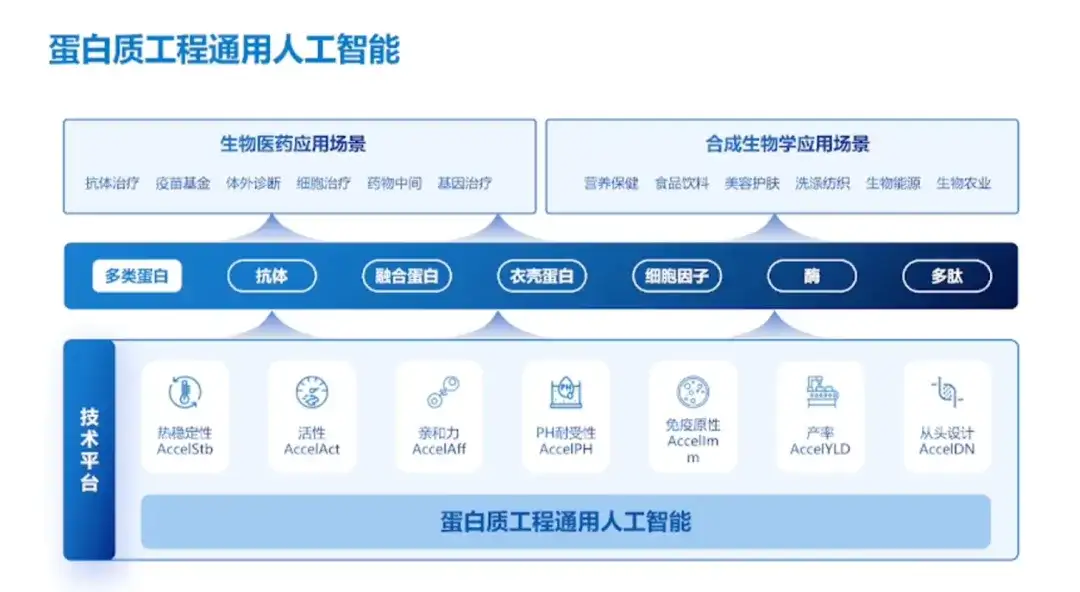
We have in-depth cooperation with many universities and enterprises, and have three core advantages
In addition, Professor Hong Liang also demonstrated the team’s achievements and core advantages.
In terms of achievements, the team has conducted in-depth cooperation with universities/research institutes such as Tsinghua University and the Institute of Immunochemistry of ShanghaiTech University, as well as companies such as Jinsai Pharmaceuticals, Hanhai New Enzyme, and Corning Jeol.In the past year, 20 proteins have been successfully transformed with fruitful results.
In terms of team advantages, Professor Hong Liang said,The team "has core advantages in three aspects: new data, independent models, and first-to-market products."First, the team has complete protein sequence data that is significantly larger than public data sets; second, the team has independent models, self-built protein vocabulary, small sample learning methods, and sequence + structure pre-training methods, and the experimental accuracy and research speed are at the forefront of the world; finally, on a global scale, the team has taken the lead in realizing the practical application of multiple protein products.
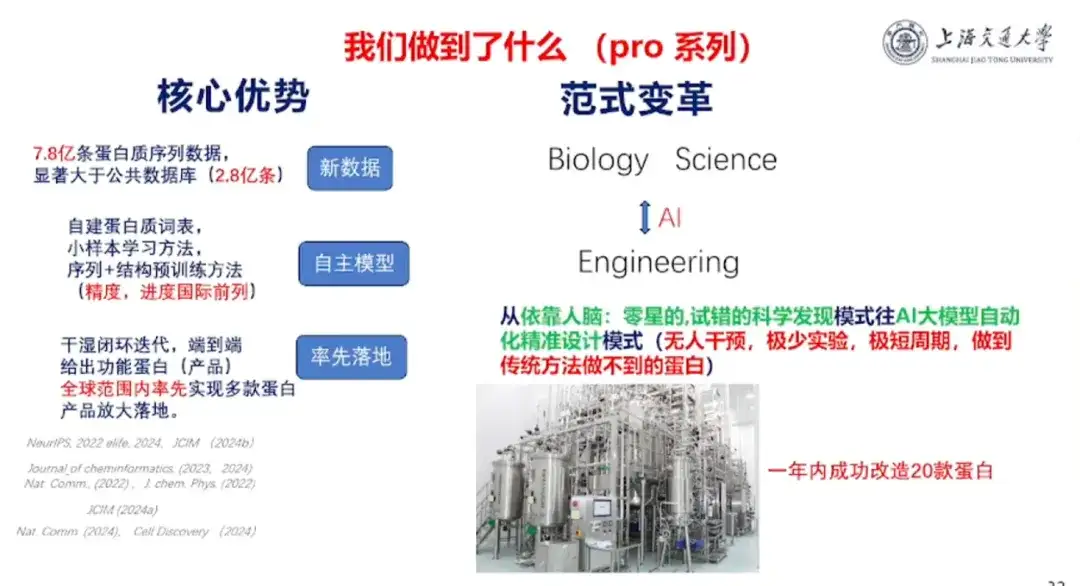
AI for Science Outlook: In the next three years, we will realize the standard design mode of AI large model automation
Professor Hong Liang believes that "in the next three years, in the fields of protein design, drug development, disease diagnosis, new target discovery, chemical synthesis pathway design, and material design, general artificial intelligence in professional fields will bring about a clear paradigm shift, transforming the scientific discovery model that used to rely on sporadic trial and error of the human brain into an AI large-model automated standard design model."
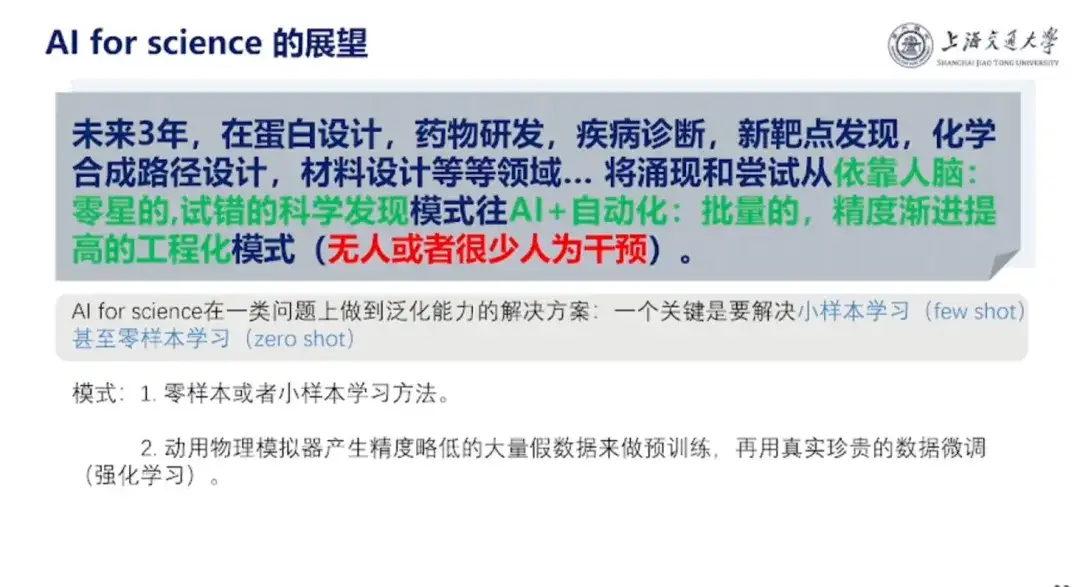
Specific changes include building zero-sample or small-sample learning methods, and building pre-training technology models.In the absence of data, a large amount of fake data with slightly lower accuracy is generated through a physical simulator for pre-training, and then fine-tuned with real and valuable data to complete reinforcement learning. Professor Hong emphasized, "Fake data refers to data that is not in the real world, but has a certain degree of reliability. It can be generated by AI or obtained through physical computing simulation for data enhancement. Finally, real wet experimental data is the most valuable and is used for the final fine-tuning of the model."
At the end of this sharing session, Professor Hong Liang once again summarized the AI liberal arts students vs. AI science students. He believes that AI liberal arts students are essentially personal assistants for human life and work, such as Kimi, ChatGPT, etc., can help people reduce repetitive creative or unscientific work. Its characteristics are large data, low precision requirements, the ability to work hard, strong cross-domain generalization ability, and can be used to build cross-domain general large models, but it should belong to large companies and is not suitable for universities and research institutes.
and AI science students need to solve a type of scientific or engineering problem.Replacing the R&D brains of scientists from enterprises and scientific institutes, doing highly creative things, greatly reducing costs and increasing efficiency, and even developing products that were impossible with previous scientific experience, teams from universities and research institutes can combine their unique professional barriers to explore AI solutions in related fields.
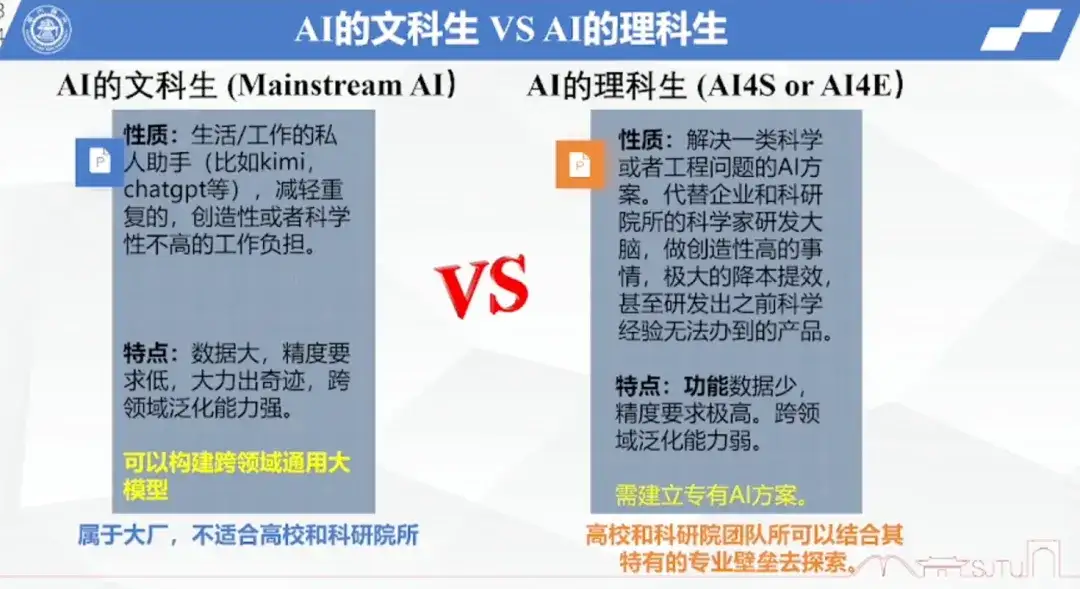
About Professor Hong Liang
Professor Hong Liang studied for his undergraduate degree in the Department of Physics at the University of Science and Technology of China, and for his graduate studies at the Chinese University of Hong Kong, where his research direction was the synthesis/characterization of nanomaterials. He received his doctorate from the University of Akron in the United States, where his main research directions were the physicochemical properties, dynamics, and phase transitions of polymers/proteins.
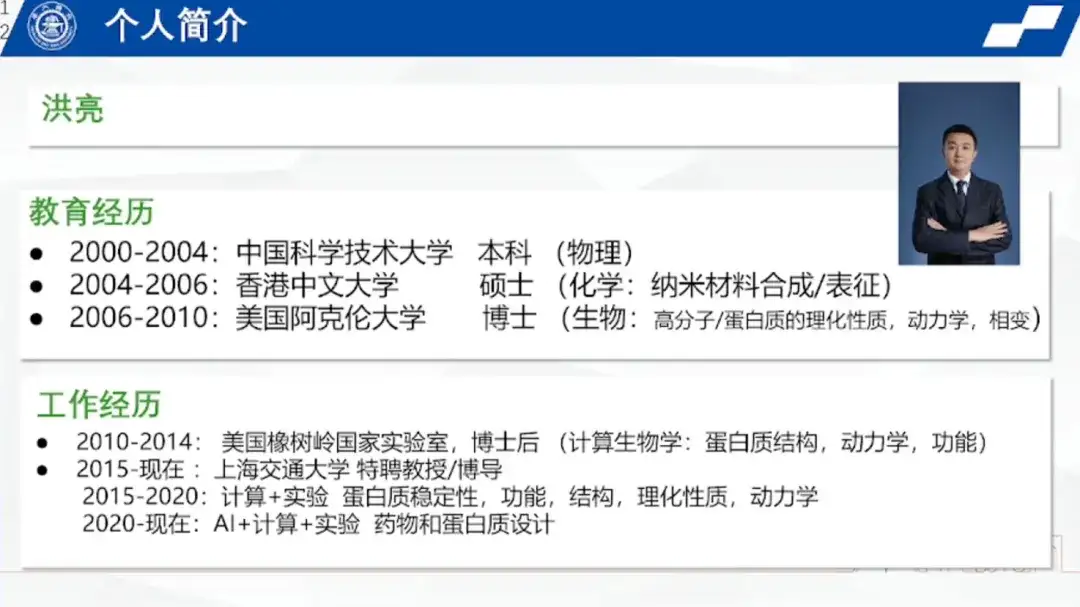
In 2010,Professor Hong Liang joined Oak Ridge National Laboratory in the United States as a postdoctoral student, focusing on protein structure, dynamics and function in the field of computational biology. In 2015,Professor Hong Liang joined Shanghai Jiao Tong University as an independent PI to conduct molecular biophysics research. In 2020,Professor Hong Liang combines AI, computing, and wet experiments to conduct protein design research. He went from physics to chemistry, from chemistry to biology, and finally from wet experiments to computing and artificial intelligence, which is a typical interdisciplinary research background.
After three years, Professor Hong Liang's team independently developed the "from sequence to function" AI protein general artificial intelligence Pro series: From the pre-training of large models, to the exploration of underlying vocabulary lists, and then to supervised learning methods, we have created a database of protein physical and chemical property labels, and on this basis, we have developed a small sample fine-tuning method, and finally opened up an artificial intelligence solution for functional design of protein sequences.
For related results, please refer to the homepage of its research group:
https://ins.sjtu.edu.cn/people/lhong/papers.html
So far, the research team led by Professor Hong Liang has carried out rich and in-depth exchanges and cooperation with academic and industrial partners.It involves many fields such as biomedicine, in vitro diagnostics, pharmaceutical intermediates, nutrition and health care, food and beverages, beauty and skin care, washing and textiles, bioenergy, bioagriculture and environmental engineering.At a time when scientific research results are being produced at a high rate or even at a crazy rate, they still adhere to the original intention of "conducting practical research", practice what they preach, and keep their feet on the ground, bringing one scientific research result after another from the laboratory to the production line.
For more information about Professor Hong Liang, please visit:
https://ins.sjtu.edu.cn/people/