SAM 2's Latest Application Has Landed! The Oxford University Team Released Medical SAM 2, Refreshing the SOTA List of Medical Image Segmentation
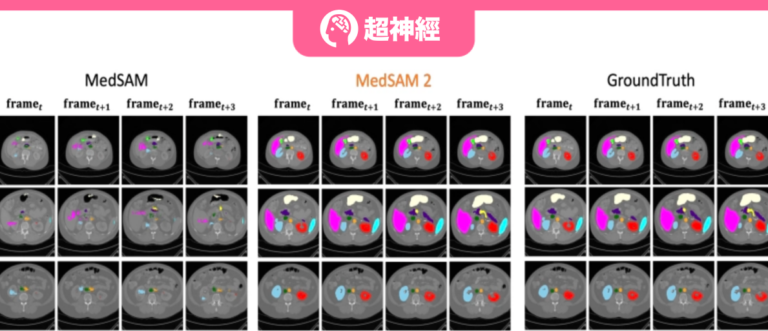
In April 2023, Meta released the Segment Anything Model (SAM), claiming to be able to "segment everything". It was like a bombshell that shook the entire computer vision field and was even regarded by many as a research that subverted traditional CV tasks.
After more than a year,Meta has released another milestone update - SAM 2, which can provide real-time, suggestible object segmentation for static images and dynamic video content, integrating image and video segmentation functions into the same system.As you can imagine, the strong strength has enabled the industry to accelerate the exploration of SAM's applications in different fields, especially in the field of medical image segmentation. Many laboratories and academic research teams have already regarded it as the only choice for medical image segmentation models.
The so-called medical image segmentation is to segment the parts with special meanings in medical images and extract relevant features, thereby providing a reliable basis for clinical diagnosis, pathological research, etc.
In recent years, with the continuous advancement of deep learning technology, segmentation based on neural network models has gradually become the mainstream method of medical image segmentation, and automated segmentation methods have greatly improved efficiency and accuracy.Given the particularity of the field of medical image segmentation, there are still some challenges that need to be addressed.
The first is model generalization.Models trained for specific targets (such as organs or tissues) are difficult to adapt to other targets, so it is often necessary to redevelop corresponding models for different segmentation targets;The second is data differences.Many standard deep learning frameworks developed for computer vision are designed for 2D images, but in medical imaging, data is usually in 3D format, such as CT, MRI, and ultrasound images. This difference undoubtedly causes great trouble for model training.
In order to solve the above problems,The Oxford University team developed a medical image segmentation model called Medical SAM 2 (MedSAM-2).The model is designed based on the SAM 2 framework and treats medical images as videos. It not only performs well in 3D medical image segmentation tasks, but also unlocks a new single-prompt segmentation capability. Users only need to provide a prompt for a new specific object, and the segmentation of similar objects in subsequent images can be automatically completed by the model without further input.
The relevant papers and results are currently titled "Medical SAM 2: Segment medical images as video via Segment Anything Model 2" and have been published on the preprint platform arXiv.
Research highlights:
* The team pioneered the medical image segmentation model MedSAM-2 based on SAM 2
* The team adopted a novel "medical-images-as-videos" concept, unlocking the "single prompt segmentation function"
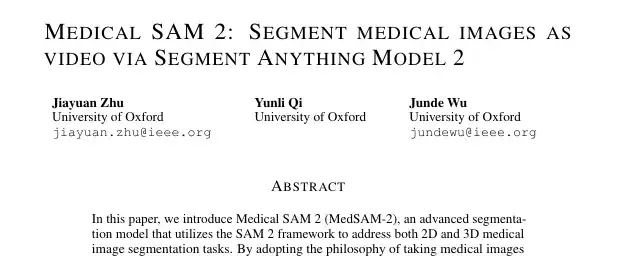
Paper address:
https://arxiv.org/pdf/2408.00874
SA-V video segmentation dataset direct download:
Medical SAM 2 sample medical segmentation dataset:
The open source project "awesome-ai4s" brings together more than 100 AI4S paper interpretations and provides massive data sets and tools:
https://github.com/hyperai/awesome-ai4s
Dataset: Classification Design, Comprehensive Evaluation
The team conducted experiments on 5 different medical image segmentation datasets using automatically generated mask cues, which were divided into two categories:
The first category aims to evaluate the general segmentation performance,The team chose the abdominal multi-organ segmentation task and selected the BTCV dataset, which contains 12 anatomical structures.
The second category aims to evaluate the generalization ability of the model across different imaging modalities.The researchers used the REFUGE2 dataset for optic disc and optic cup image segmentation; the BraTs 2021 dataset for brain tumor segmentation in MRI scans; the TNMIX benchmark was used to segment thyroid nodules in ultrasound images, which consists of 4,554 images from TNSCUI and 637 images from DDTI; and the ISIC 2019 dataset was used to segment skin lesions into melanoma or nevus.
In addition, the team set up 10 additional 2D image segmentation tasks and further evaluated the model's single-shot prompt segmentation capabilities by using different types of prompts. Specifically, the KiTS23, ATLAS23, TDSC, and WBC datasets use point prompts; the SegRap, CrossM23, and REFUGE datasets use BBox (bounding box) prompts; and the CadVidSet, STAR, and ToothFairy datasets use mask prompts.
Model architecture: Effective segmentation processing for medical images of different dimensions
The architecture of MedSAM-2 is basically similar to SAM 2, but the research team has also built a unique and efficient processing module and pipeline for it, combined with a confidence memory bank and a weighted pick-up strategy to technically guarantee the model's capabilities.
Specifically,The architecture of MedSAM-2 is shown in the figure below.Include:
* Image Encoder, abstracts the input into embedding
* Memory Attention, which uses memories stored in the memory bank to adjust the input embedding
* Memory Decoder, which abstracts the predicted frame embedding
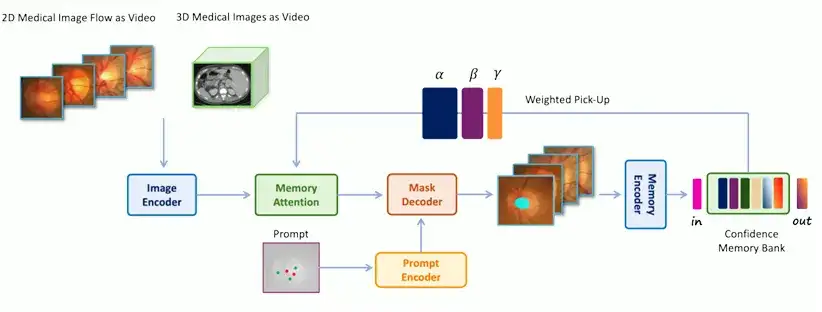
The encoder and decoder in the network are similar to those in SAM. The encoder consists of a layered visual transformer, and the decoder includes a lightweight bidirectional transformer that integrates prompt embedding and image embedding, where the prompt embedding is generated by the prompt encoder; the memory attention component consists of a series of stacked attention blocks, each of which contains a self-attention block and a cross-attention mechanism.
It is worth noting thatAn important innovation of MedSAM-2 is to treat medical image processing as video segmentation.This is the key to improving the performance of 3D medical image segmentation and unlocking the "single prompt segmentation function". To this end, the team has also developed two different operating processes for 2D and 3D medical images respectively to perform effective segmentation processing for medical images of different dimensions.
For 3D medical image processing,Because there is a strong temporal correlation between adjacent slices in 3D medical images, the processing method is similar to that of video data. The original storage system of SAM 2 is used to retrieve previous slices and their corresponding predictions for continuous slice segmentation. The input image embedding is then enhanced through the memory attention mechanism, and the segmentation results are added back to the storage area to assist in the segmentation of subsequent slices.
For 2D medical image processing,The processing method is different from the temporal first-in-first-out queue used in SAM 2. Instead, a group of medical images containing the same organ or tissue is grouped into a "medical image stream" and a "confidence-first" storage area is used to store the model's templates. The confidence is calculated based on the probability predicted by the model, and image diversity constraints are implemented. A weighted selection strategy is adopted when merging the input image embedding and storage area information. During the training phase, a calibration head is used to ensure more accurate model predictions. Ultimately, automatic segmentation of the target can be achieved with only one sample prompt without temporal association.
Experimental results: MedSAM-2 leads in performance and generalization ability
The research team used IoU (Intersection over Union) and Dice Score to evaluate the performance of the model in medical image segmentation, and introduced the Hausdorff Distance (HD95) metric to ensure the accuracy of performance evaluation.
*LoU, also known as the Jaccard index, is a metric used to evaluate the accuracy of an object detector on a specific dataset.
* Dice Score, also known as Dice Coefficient, is a statistical tool for comparing the similarities between two samples.
* The Hausdorff Distance (HD95) metric is a metric used to determine the degree of difference between two sets of points. It is often used to evaluate the accuracy of object boundaries in image segmentation tasks and is particularly effective in quantifying the worst case distance between the predicted segmentation and the actual boundary.
First, the team benchmarked MedSAM-2 against a range of SOTA medical image segmentation methods, including segmentation tasks for 2D and 3D medical images. For 3D medical images, the hint was randomly provided with a probability of 0.25; for 2D medical images, the hint was provided with a probability of 0.3.
In order to evaluate the general performance of the proposed model on 3D medical images,The team compared MedSAM-2 with advanced segmentation methods established on the BTCV multi-organ segmentation dataset, including the well-known nnUNET, TransUNet, UNetr, Swin-UNetr models, and Diffusion-based models (such as EnsDiff, SegDiff, and MedSegDiff). In addition, the team also compared and evaluated the original SAM, fully fine-tuned MedSAM, SAMed, SAM-Med2D, SAM-U, VMN, and interactive segmentation models such as FCFI. The performance was quantified using the Dice Score, and the results are shown in the figure below:
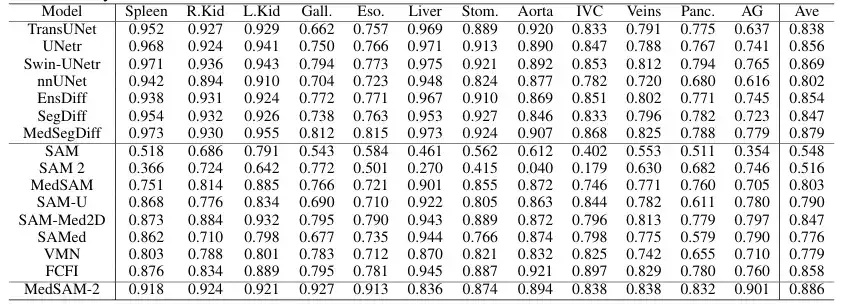
The results show that MedSAM-2 is a significant improvement over the previous SAM and MedSAM. On the BTCV dataset, MedSAM-2 achieves excellent performance on the multi-organ segmentation task, reaching a final Dice score of 88.57%. Among the interactive models, MedSAM-2 maintains its leading position, outperforming the previous leading interactive model Med-SA by 2.78%. All of these interactive models require prompts for each frame, while MedSAM-2 achieves better results with fewer prompts.
In the 2D medical image segmentation task,The team compared MedSAM-2 with methods tailored for specific tasks on different image modalities. Specifically, for optic cup segmentation, it was compared with ResUnet and BEAL; for brain tumor segmentation, it was compared with TransBTS and SwinBTS; for thyroid nodule segmentation, it was compared with MTSeg and UltraUNet; for skin lesion segmentation, it was compared with FAT-Net and BAT. In addition, the team also benchmarked the interactive model, and the results are shown in the figure below:
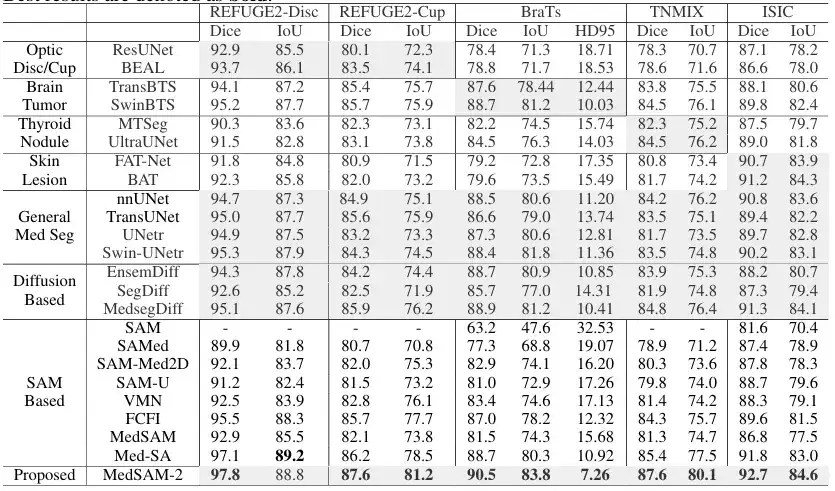
The results show that MedSAM-2 outperforms all other methods in 5 different tasks, demonstrating its excellent generalization ability in different medical image segmentation tasks. Specifically, MedSAM-2 achieved an improvement of 2.0% on the optic cup, 1.6% on the brain tumor, and 2.8% on the thyroid nodule. In the interactive model comparison, MedSAM-2 still maintains its leading performance.
at last,The team also evaluated the performance of MedSAM-2 when given only one cue.And there is no clear connection between sequential images, which further verifies the ability of MedSAM-2 to segment with a single prompt. Specifically, the team compared MedSAM-2 with PANet, ALPNeu, SENet, and UniverSeg, all of which were tested with only one prompt. In addition, the team also compared MedSAM-2 with single-shot models such as DAT, ProbONE, HyperSegNas, and One-prompt.
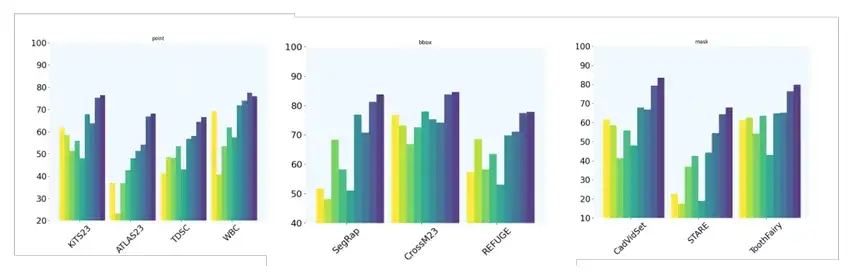
The results show that MedSAM-2 demonstrates robust generalization across a variety of tasks, and even compared to the intensively trained One-prompt, it still performs well, only falling behind in 1 of the 10 tasks. In addition, in scenarios where all methods provide masks, MedSAM-2 shows a more obvious advantage, usually surpassing the second place 3.1% on average, which is the largest gap among all prompt settings.
SAM helps medical image segmentation research in full swing
The publication of this paper can be regarded as another in-depth exploration of the potential of SAM and SAM 2 in the medical field. It provides a new idea and method for the field of medical image segmentation, especially showing great potential and value in clinical applications. It can greatly reduce the workload of medical image segmentation and improve the efficiency and accuracy of medical image segmentation.
What is more worth mentioning is that, as mentioned at the beginning of the article,Many laboratories and academic teams are exploring the potential of SAM.In the field of medical image segmentation, there is more than just the Oxford University team mentioned in this paper.
Coincidentally, shortly after the release of SAM, Professor Ni Dong's team from the School of Biomedical Engineering, School of Medicine, Shenzhen University, in collaboration with the University of Oxford, ETH Zurich, Zhejiang University, Shenzhen People's Hospital, and Duying Medical, launched a comprehensive, multi-angle experiment and evaluation on the application of SAM in medical imaging tasks. The relevant paper and results were published in the top international journal in the field of medical image analysis, "Medical Image Analysis", with the title "Segment Anything Model for Medical Images?"
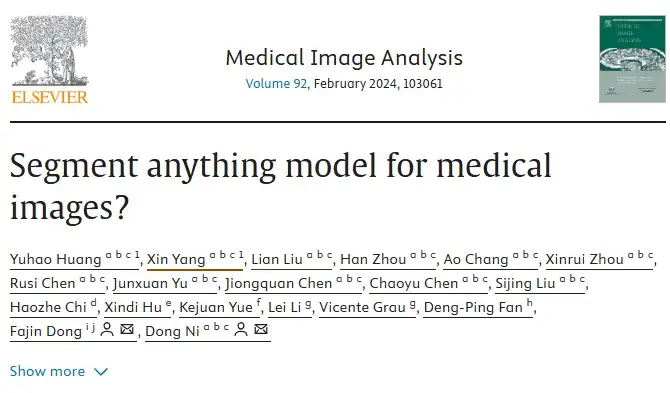
In the research of this paper, the relevant team finally constructed a large-scale medical image segmentation dataset COSMOS 1050K, which contains 18 imaging modalities, 84 biomedical segmentation targets, 1050K 2D images and 6033K segmentation masks. Based on this dataset, the researchers conducted a comprehensive evaluation of SAM and explored ways to improve SAM's ability in medical target perception.
COSMOS 1050K medical image segmentation dataset direct download:
In addition, teams from the School of Big Data at Fudan University and the School of Biomedical Engineering at Shanghai Jiao Tong University have also conducted a series of studies on SAM in the field of medical image segmentation. The relevant paper is titled "Segment anything model for medical image segmentation: Current applications and future directions" and is included in well-known academic websites and journals such as arXiv and Computer in Biology and Medicine.
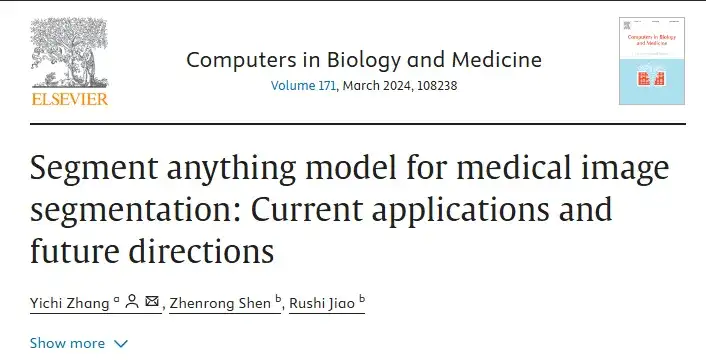
This paper focuses on the possible application of SAM, which has achieved remarkable achievements in natural image segmentation, in the field of medical image segmentation, and explores the fine-tuning of the SAM module and the retraining of similar architectures to adapt to medical image segmentation.
Paper address:
https://www.sciencedirect.com/science/article/abs/pii/S0010482524003226
In summary, as discussed in the above papers, scientists have made the processing and analysis of medical images simpler and more efficient by exploring the potential of SAM, which is a result worth looking forward to for the academic community, the medical community, and even patients. At the same time, the release of a general image segmentation model like SAM has also opened a magical door to various fields.I believe that not only the field of medical imaging, but also autonomous driving, new media, AR/VR... may benefit greatly in the future.
Book draw
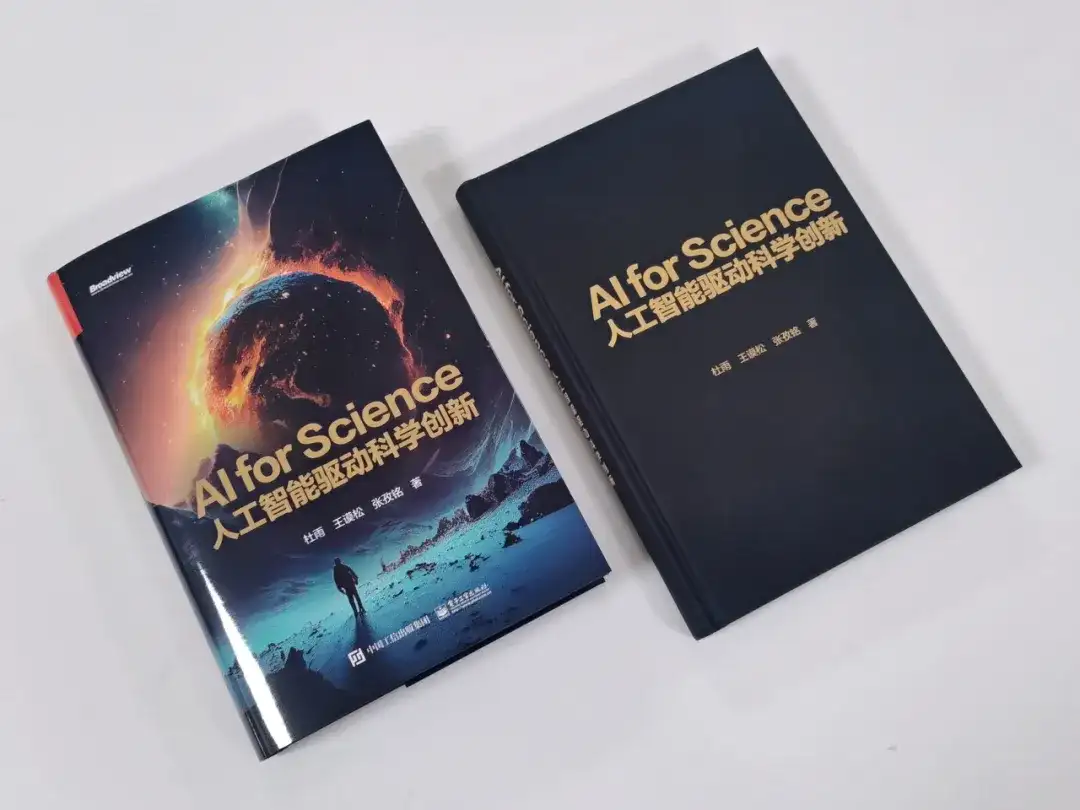
HyperAI and Electronics Industry Press have brought you a book giveaway! We have prepared 5 super-useful popular science books "AI for Science: Artificial Intelligence Drives Scientific Innovation". Come and participate in the lucky draw~
How to participate
Follow the HyperAI WeChat official account, reply "AI4S free book" in the background, and click on the lucky draw page to participate in the lucky draw. We have prepared 5 books for you, which will be delivered to you by express delivery. Come and participate!
Book Introduction
From predicting protein structure to inferring the pathogenicity of gene mutations, the new paradigm led by AI has allowed us to see new opportunities in various scientific fields, including life sciences.
The book "AI for Science: Artificial Intelligence Drives Scientific Innovation" focuses on the cross-integration of artificial intelligence with five major fields: materials science, life science, electronic science, energy science, and environmental science. It uses simple language to comprehensively introduce basic concepts, technical principles, and application scenarios, allowing readers to quickly master the basic knowledge of AI for Science. In addition, for each cross-field, the book provides a detailed introduction through cases, sorts out the industry map, and provides relevant policy inspiration.