Tsinghua Team Proposes AI-based Model ROAM to Achieve Accurate Diagnosis of Glioma
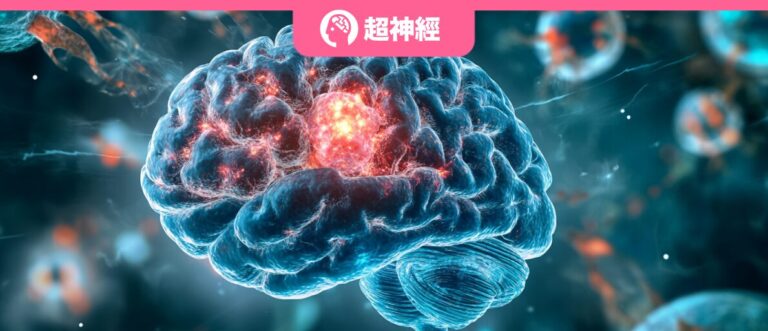
Glioma is a tumor that originates from glial cells in the brain. It accounts for 40% to 60% of all primary central nervous system tumors and is known as the most common primary intracranial tumor in adults. The histopathological classification of gliomas is very complex and is usually divided into three subtypes: astrocytomas, oligodendrogliomas, and ependymomas, each of which can be further divided into several grades. Therefore,Accurate classification and grading are crucial for the prognosis and treatment of gliomas.
The diagnosis of glioma is usually completed by experienced pathologists through observation of tissue sections. However, this method has challenges such as scarcity of pathologists, subjective diagnosis and time-consuming diagnosis process.It is difficult to meet the current diagnostic needs of gliomas.
Recent advances in digital pathology and machine learning have enabled the digitization of histological sections, which are converted into gigapixel whole-slide images (WSIs) containing rich contextual data, with great potential in diagnosis, prognosis, and molecular characterization. However, these methods only analyze regions of interest (ROIs) in pathological images selected by pathologists.Automated analysis of the entire slide is not possible.
In this context,Associate Researcher Lü Hairong, Professor Jiang Rui, Professor Zhang Xuegong from the Life Basic Model Laboratory of the Department of Automation at Tsinghua University, and Professor Hu Zhongliang from the Xiangya Hospital of Central South University,A precise pathological diagnosis AI basic model ROAM based on large regions of interest and pyramid transformer is proposed for clinical-level diagnosis and molecular marker discovery of glioma, and can be extended to pathological diagnosis of other types of tumors.
The related research results were published in Nature Machine Intelligence under the title "A transformer-based weakly supervised computational pathology method for clinical-grade diagnosis and molecular marker discovery of gliomas".
ROAM can effectively extract rich multi-scale information from pathological images and achieve accurate diagnosis for various classification tasks such as glioma tumor detection, subtype classification/grading, and molecular feature prediction.On in-house data, ROAM demonstrated excellent diagnostic performance, automatically capturing key morphological features consistent with pathologists’ experience, and providing accurate, reliable, and adaptable clinical-grade diagnosis for gliomas.
In addition, ROAM can be extended to independent external data and has excellent generalization capabilities. By visualizing and explaining the diagnosis, ROAM can help pathologists verify the reliability of the model's diagnostic basis, extract valuable information, and promote auxiliary diagnosis and improve medical standards. Most importantly, ROAM helps discover molecular and morphological biomarkers, providing new insights into the diagnosis and treatment of gliomas.
Research highlights:
* ROAM achieves efficient extraction of visual representations of whole-slice tissue pathology images through large-size image patches and multi-scale feature learning modules
* ROAM can be extended to independent external data and has excellent generalization ability
* ROAM facilitates the discovery of molecular and morphological biomarkers, providing new insights into the diagnosis and treatment of gliomas
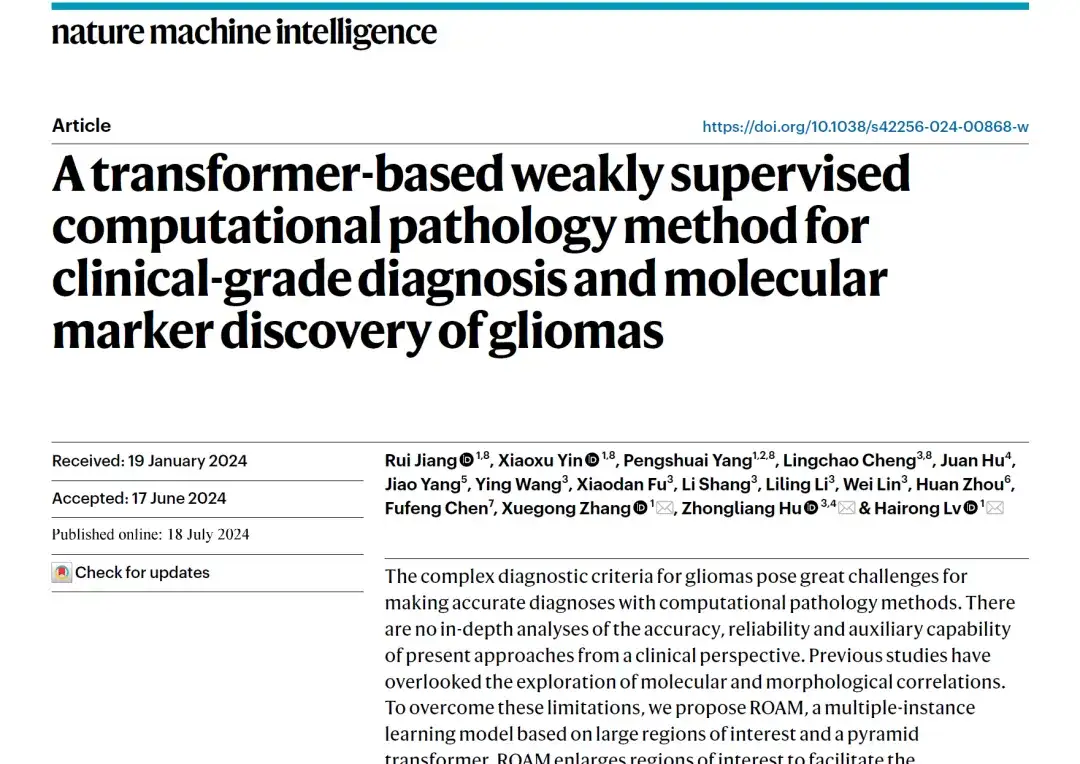
Paper address:
https://www.nature.com/articles/s42256-024-00868-w
Dataset download:
The open source project "awesome-ai4s" brings together more than 100 AI4S paper interpretations and provides massive data sets and tools:
https://github.com/hyperai/awesome-ai4s
Dataset: Two major digital tissue pathology slide datasets
In this study, two large-scale digital histopathology slide (WSI) datasets were collected for glioma research.
1. Xiangya Glioma WSI Dataset
This dataset comes from glioma sections from Xiangya Hospital of Central South University.As shown in the figure below, it contains 1,109 WSIs processed at ×40 magnification in the same number of different cases, covering diagnostic tasks such as glioma detection, subtype classification, grading, and molecular feature prediction, and the same number of slices were collected for each case.
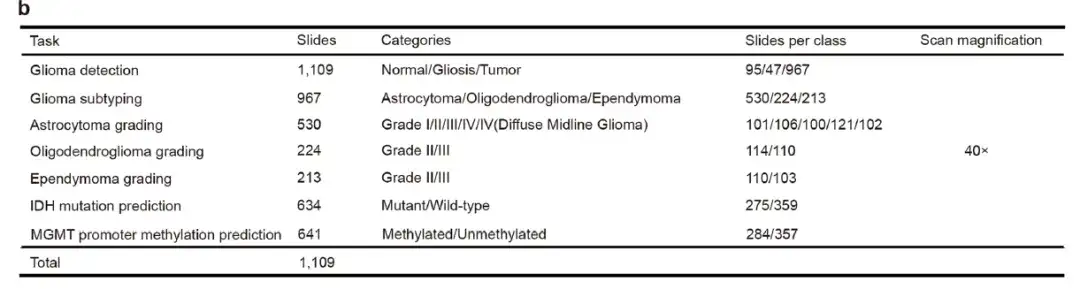
The dataset contains only slice-level annotations, which indicate the subtype and grade of 530 astrogliomas, 224 oligodendrogliomas, and 213 ependymomas. In addition, molecular testing was performed for 634 IDH mutation cases and 641 MGMT promoter methylation cases.
The dataset is randomly divided into two parts:One is an internal training dataset containing 736 WSIs, which is used for model training; the other is an internal testing dataset containing 373 WSIs, which is used for model evaluation and doctor-related experiments. The class ratios in both datasets are the same as the entire dataset.
2. TCGA Glioma WSI Dataset
Another glioma histopathology WSI dataset comes from the Brain Low-Grade Gliomas and Glioblastoma Project.There are 860 glioblastoma sections (from 389 cases) and 844 low-grade glioma sections (from 491 cases). The diagnostic criteria are different from those used in the Xiangya dataset, so only the slice-level annotations in the data are retained. Two pathologists who participated in the annotation of the Xiangya dataset were invited to review and revise the diagnostic results of these sections according to the 2016 version of the diagnostic guidelines.
Finally, the reviewed dataset includes 618 WSIs at magnifications of ×40 and ×20, covering 4 tasks consistent with the Xiangya dataset. This dataset serves as an external test dataset for glioma subtype classification, grading, and molecular feature prediction.
Model architecture: Based on large region interest and pyramid Transformer
ROAM is a weakly supervised computational pathology methodThis method uses multi-instance learning as the basic framework, large-size tissue image blocks as the basic research unit, and uses pyramid Transformer to systematically learn the intra-scale and inter-scale correlation features of each tissue block, thereby achieving effective extraction of visual representations of whole tissue slice images.
first,ROAM performs tissue segmentation on each whole slice image and extracts large-sized tissue image patches (2048×2048) from them as the basic unit for subsequent analysis, namely ROI, as shown in the following figure WSI patching:
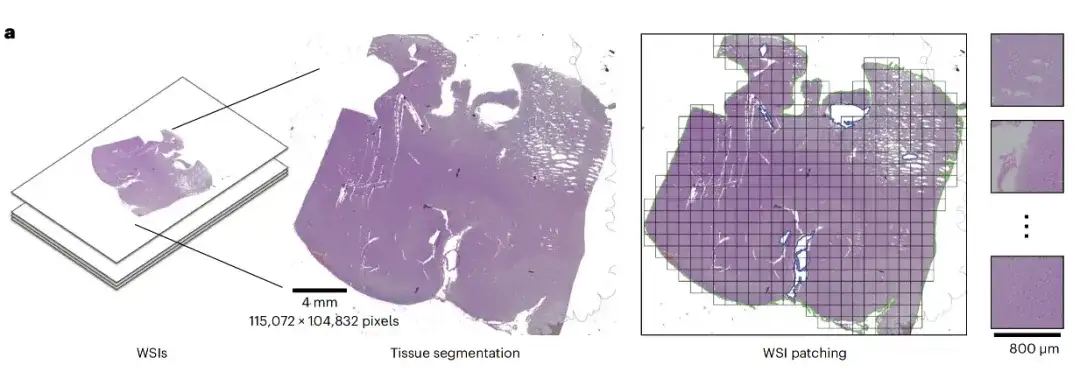
Secondly,Each ROI is downsampled twice continuously to generate three images with different magnifications. Each image is then divided into small image blocks, which are encoded by a pre-trained convolutional neural network to extract their visual representations, which are used as the input of the MIL model, as shown in the instance feature extraction on the left side of Figure b below; the multi-scale self-attention (SA) module and the attention network are used to generate instance-level representations, and this information is aggregated into slice-level representations, as shown in the multi-scale feature extraction on the right side of Figure b below.
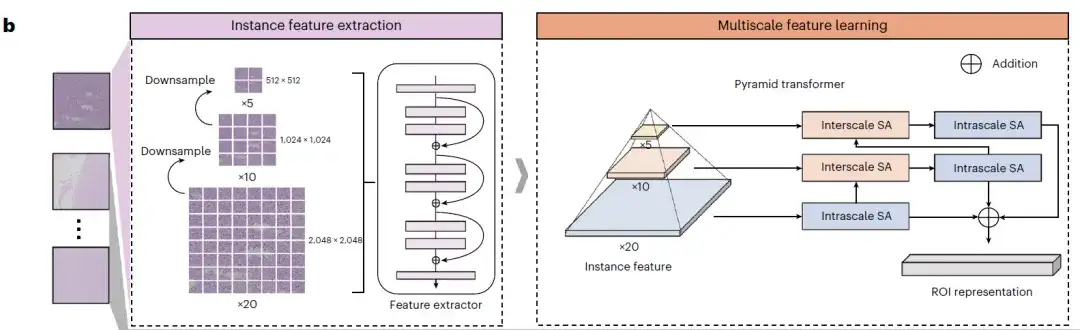
at last,As shown in the example feature aggregation in Figure c below, two different types of SA modules use the pyramid transformer architecture to gradually fuse multi-scale features from high magnification to low magnification to obtain multi-scale visual representations of tissue blocks. The intra-scale SA module and the inter-scale SA module learn the intra-scale and inter-scale related features of the ROI respectively. Both modules contain several multi-head SA layers and feed-forward layers.
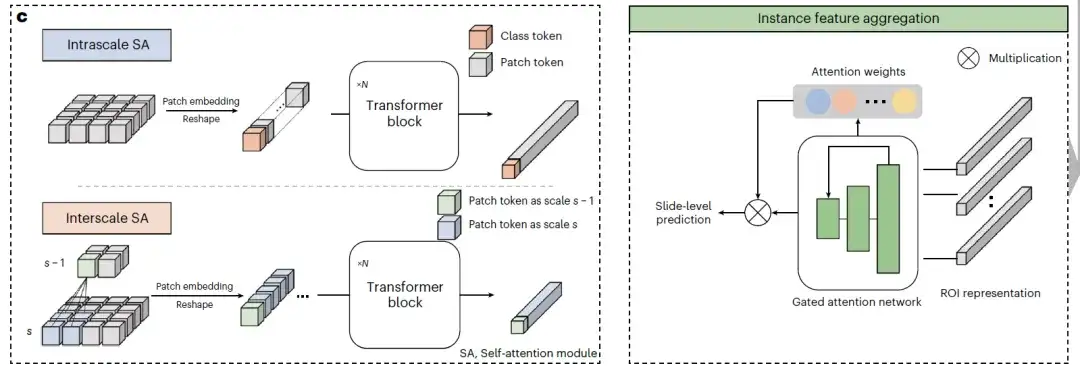
Research results: ROAM achieves accurate diagnosis of glioma
ROAM achieves accurate diagnosis of glioma
The researchers evaluated the classification performance of ROAM on internal datasets and TCGA external datasets.
As shown in Figure a below, ROAM outperforms five methods including CLAM, TransMIL, GTP, TEA-graph, and H(2)MIL, and outperforms other baseline methods in tasks related to glioma diagnosis in internal datasets. In the detection of three types of gliomas, normal, gliosis, and tumor, the macro-average one-to-one ROC curve area (AUC) of ROAM is 0.990±0.002.
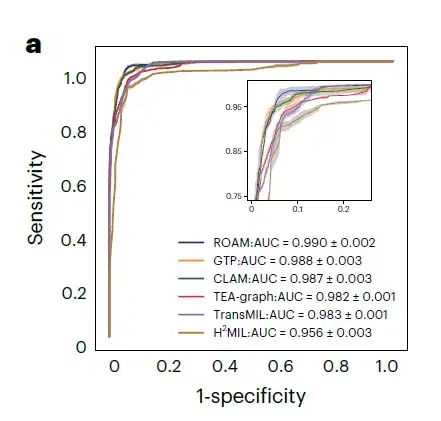
For the classification of three glioma subtypes, namely astrocytoma, oligodendroglioma, and ependymoma, the AUC of ROAM was 0.950±0.003, as shown in Figure b below.
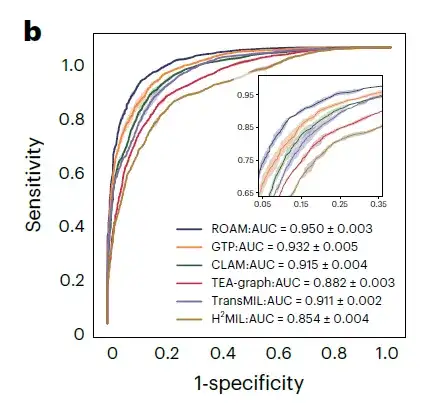
In these glioma diagnosis tasks, ROAM achieved the highest AUC among all baseline methods.The effectiveness and high performance of the ROAM model in glioma diagnosis were demonstrated.
at the same time,ROAM also has good generalization properties.Using only internal datasets for training and tested on external TCGA datasets, ROAM still outperforms other baseline methods overall.The visualization results of ROAM predictions also show that the diagnostic basis summarized by this method is very consistent with the clinical diagnostic criteria.
ROAM significantly advances clinical auxiliary diagnosis of glioma
The researchers conducted a comprehensive clinical-level evaluation of ROAM.The performance of ROAM in the auxiliary diagnosis of glioma was studied: 3 groups of 5 pathologists were invited to participate in the study, one group was junior pathologists with less than 5 years of clinical experience, two groups were intermediate pathologists with 5 to 15 years of clinical experience, and the other two groups were senior pathologists with more than 15 years of clinical experience.
As shown in the figure below, the proposed system performed well on the five tasks of glioma cascade diagnosis, outperforming four of the five pathologists and comparable to the best performing senior pathologist (senior 1). Specifically, in terms of glioma detection,The new system significantly outperformed all pathologists.Including the best performing pathologist, exceeding 21.30%, as shown in Figure f below.
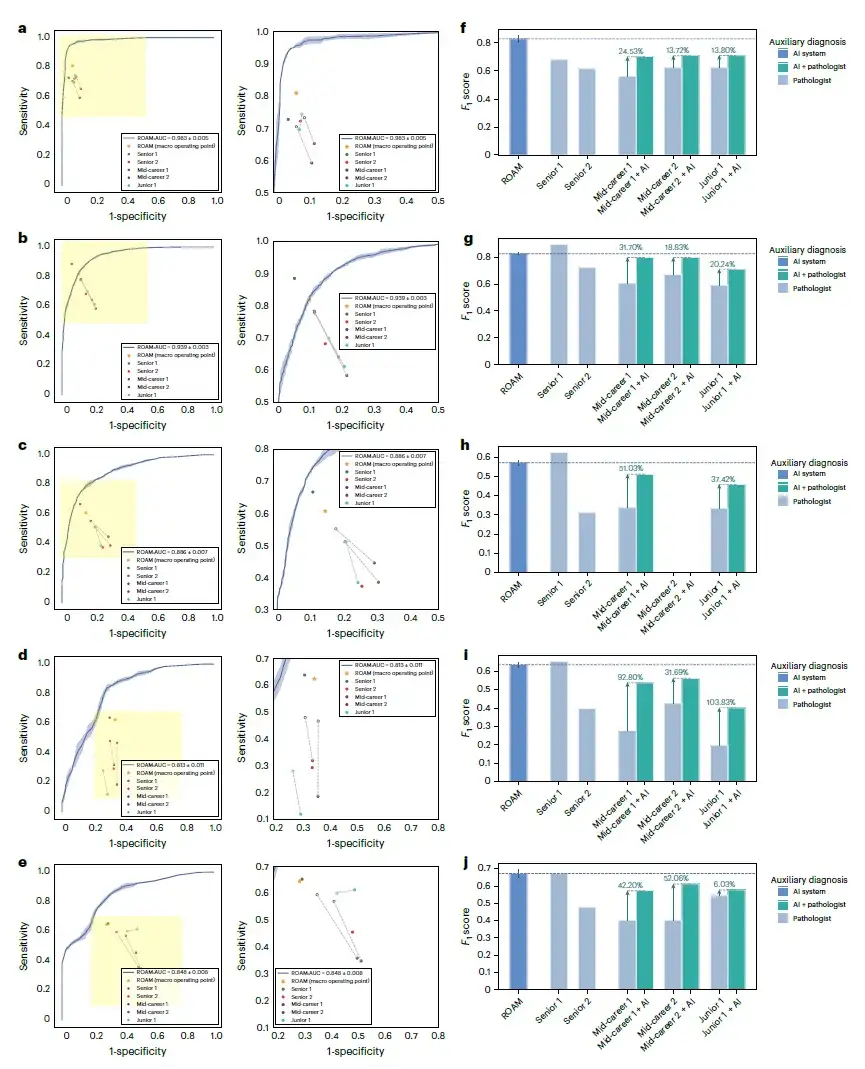
Subsequently, the researchers asked three junior and mid-level pathologists to perform diagnoses with the assistance of ROAM to investigate whether their diagnostic performance was improved. The results showed that with the help of ROAM, the diagnostic accuracy of the three pathologists improved by an average of 7.27% (junior 1), 12.87% (mid-level 1), and 9.96% (mid-level 2) in all tasks.This reflects the great clinical application value of ROAM.
ROAM promotes the discovery of molecular morphological markers of glioma
With the help of ROAM, the researchers explored the morphological manifestations of key molecular features related to glioma diagnosis, and noticed that ROAM performed well in the molecular feature task of predicting isocitrate dehydrogenase (IDH) mutations. They conducted a complete visual analysis of ROAM's prediction results in this task, and analyzed and summarized the tissue morphological features of the high-attention key areas that ROAM focused on. They found that eosinophilia, uniform cytoplasm, and dark staining of the nucleus were common in pathological images of IDH mutations.
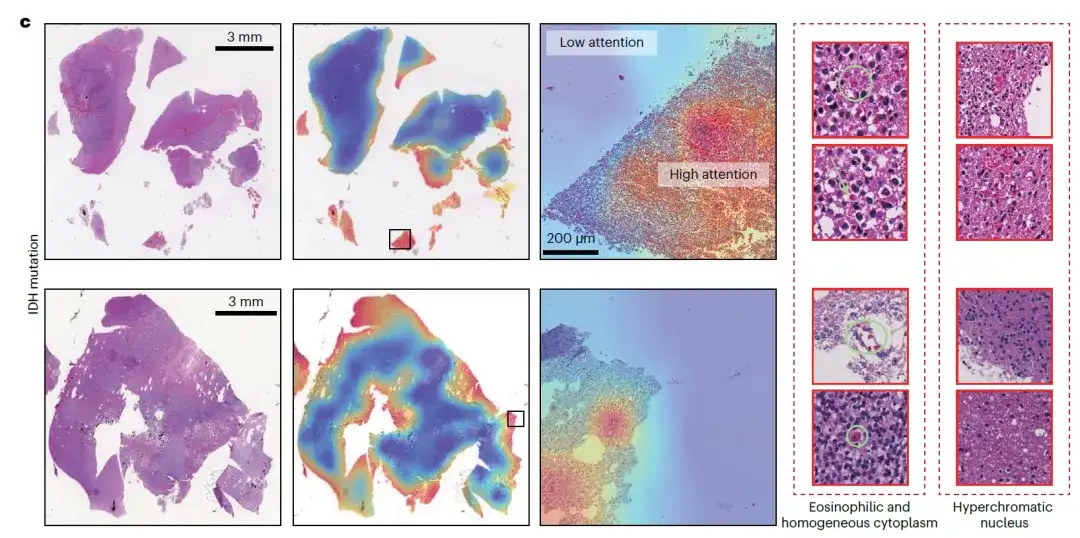
This important discovery helps doctors make preliminary predictions of IDH status without the aid of molecular testing, and will significantly promote the optimization and improvement of clinical diagnostic standards for gliomas.
Tsinghua University Department of Automation Life Basic Model Laboratory continues to promote AI to empower life science research
In this paper,Associate Researcher Lü Hairong, Professor Zhang Xuegong, and Professor Hu Zhongliang from Xiangya Hospital of Central South University are the corresponding authors.Professor Jiang Rui and master student Yin Xiaoxu from Tsinghua University, Yang Pengshuai from China Mobile Research Institute, and Cheng Lingchao from Xiangya Hospital are the co-first authors of the paper. Hu Jun, Yang Jiao, Wang Ying, Fu Xiaodan, Shang Li, Li Liling, Lin Wei, and Zhou Huan participated in data collection and annotation for this study. Chen Fuxun and the Fuzhou Data Technology Research Institute provided R&D support for the online software platform.
As one of the main contributors to this study,The Life Basic Model Laboratory of the Department of Automation at Tsinghua University is constantly committed to exploring how to empower life science research with advanced artificial intelligence technologies.
In June this year, Professor Zhang Xuegong, Director of the Life Basic Model Laboratory of the Department of Automation, Tsinghua University, Professor Ma Jianzhu of the Department of Electronics/AIR, and Dr. Song Le of Bio-Technology,A large cell model called scFoundation was established.The model is trained based on gene expression data from 50 million cells, has 100 million parameters, and can process about 20,000 genes simultaneously. As a basic model, it has shown excellent performance improvements in a variety of biomedical downstream tasks such as "virtual drug trials".Provides a new paradigm for artificial intelligence in single-cell research.
The research results were published in Nature Methods under the title "Large-scale foundation model on single-cell transcriptomics".Click to view the full report: "A cell model with 100 million parameters is here! Published in Nature sub-journal, Tsinghua University team releases scFoundation: Simultaneous modeling of 20,000 genes"
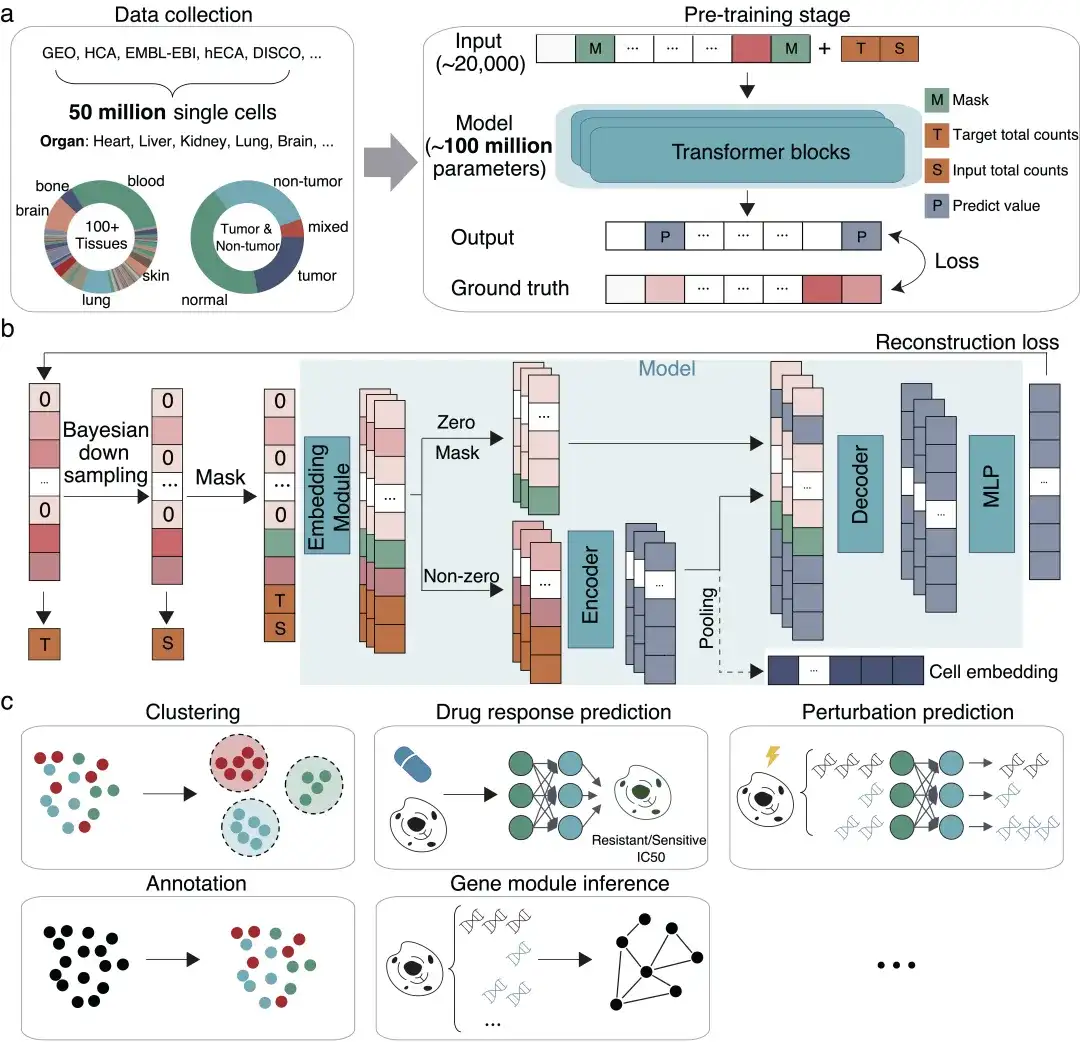
The scFoundation model provides innovative methods and tools for basic research in life sciences, prediction of cell perturbation responses, drug target discovery and other fields. It also provides new ideas and methods for large-scale cell model research in terms of model architecture, training framework and downstream demonstration application system. It has successfully expanded the boundaries of basic models in the single-cell field and laid the foundation for future research such as virtual drug experiments in digital space.
Looking to the future, the Life Basic Model Laboratory of the Department of Automation at Tsinghua University will continue to conduct research in the intersection of artificial intelligence and life sciences. With the continuous development and improvement of AI technology, the application of artificial intelligence in the field of life sciences will greatly promote the advancement of medical technology, improve the accuracy of diagnosis and treatment, reduce medical costs, and ultimately improve human health and quality of life.
References:
1.https://www.nature.com/articles/s42256-024-00868-w
2.https://mp.weixin.qq.com/s/oB3kTgcgObawPKU-75KsHQ
3.https://mp.weixin.qq.com/s/nflI4PVTJB3xVPXuA5zbZQ
Call to action
HyperAI (hyper.ai) is China's largest search engine in the field of data science. It has long focused on the latest research results of AI for Science and has interpreted more than 100 academic papers in top journals.
Research groups and teams that are conducting research and exploration around AI for Science are welcome to contact us to share their latest research results, contribute in-depth interpretation articles, and participate in the Meet AI4S live broadcast column. More ways to promote AI4S are waiting for us to explore together!
Add WeChat: PH (WeChat ID: G18539589505)