The New Biology Benchmark Dataset LAB-Bench Is Now Open Source! It Covers 8 Major Tasks and Contains Over 2.4K Multiple-choice Questions
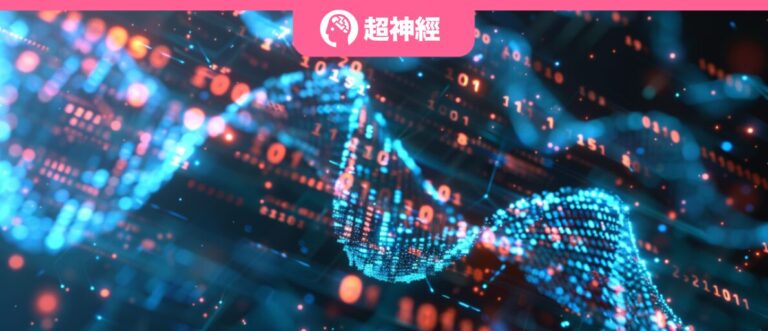
When a foreign friend greets you with “How are you?”, what is your first reaction?
Isn’t it the classic “I’m fine, Thank you. And you”?
actually,This kind of textbook question-answering exists not only in our English learning and communication, but also in the training and testing of large language models.
Nowadays, many scientists are focusing on using large language models (LLMs) and LLM-enhanced systems in research in fields such as biology, marine science, and materials science to improve scientific research efficiency and output.The Zhejiang University team has launched the OceanGPT large language model in the ocean field.Microsoft has developed the large language model BioGPT in the field of biomedicine, and Shanghai Jiao Tong University has proposed the large language model K2 in the field of earth sciences.
It is worth noting thatAs LLMs become increasingly popular in the scientific research field, it becomes crucial to establish a set of high-quality and professional evaluation benchmarks.
However, many benchmarks exist that focus on assessing LLMs’ knowledge and reasoning abilities on textbook scientific problems.It is difficult to evaluate The performance of LLM in practical scientific research tasks such as literature retrieval, program planning, and data analysis,This results in obvious deficiencies in the model's flexibility and professionalism when dealing with actual scientific tasks.
To promote the effective development of AI systems in biology,Researchers at FutureHouse Inc. have launched the Language Agent Biology Benchmark (LAB-Bench) dataset.LAB-Bench contains more than 2,400 multiple-choice questions used to evaluate the performance of AI systems in actual biological research such as literature retrieval and reasoning (LitQA2 and SuppQA), graphic interpretation (FigQA), table interpretation (TableQA), database access (DbQA), protocol writing (ProtocolQA), understanding and processing of DNA and protein sequences (SeqQA), and cloning scenarios (CloningScenarios).
The research, titled "LAB-Bench Measuring Capabilities of Language Models for Biology Research", has been submitted to the top conference NeurlPS 2024.
* LAB Bench language model biology benchmark dataset:
https://go.hyper.ai/kMe1e
Samuel G. Rodriques, the corresponding author of the paper, emphasized:As the first evaluation set that focuses on evaluating whether models and agents can conduct scientific research, LAB-Bench uses a programmatic evaluation method for complex tasks, which will be very important in the future.
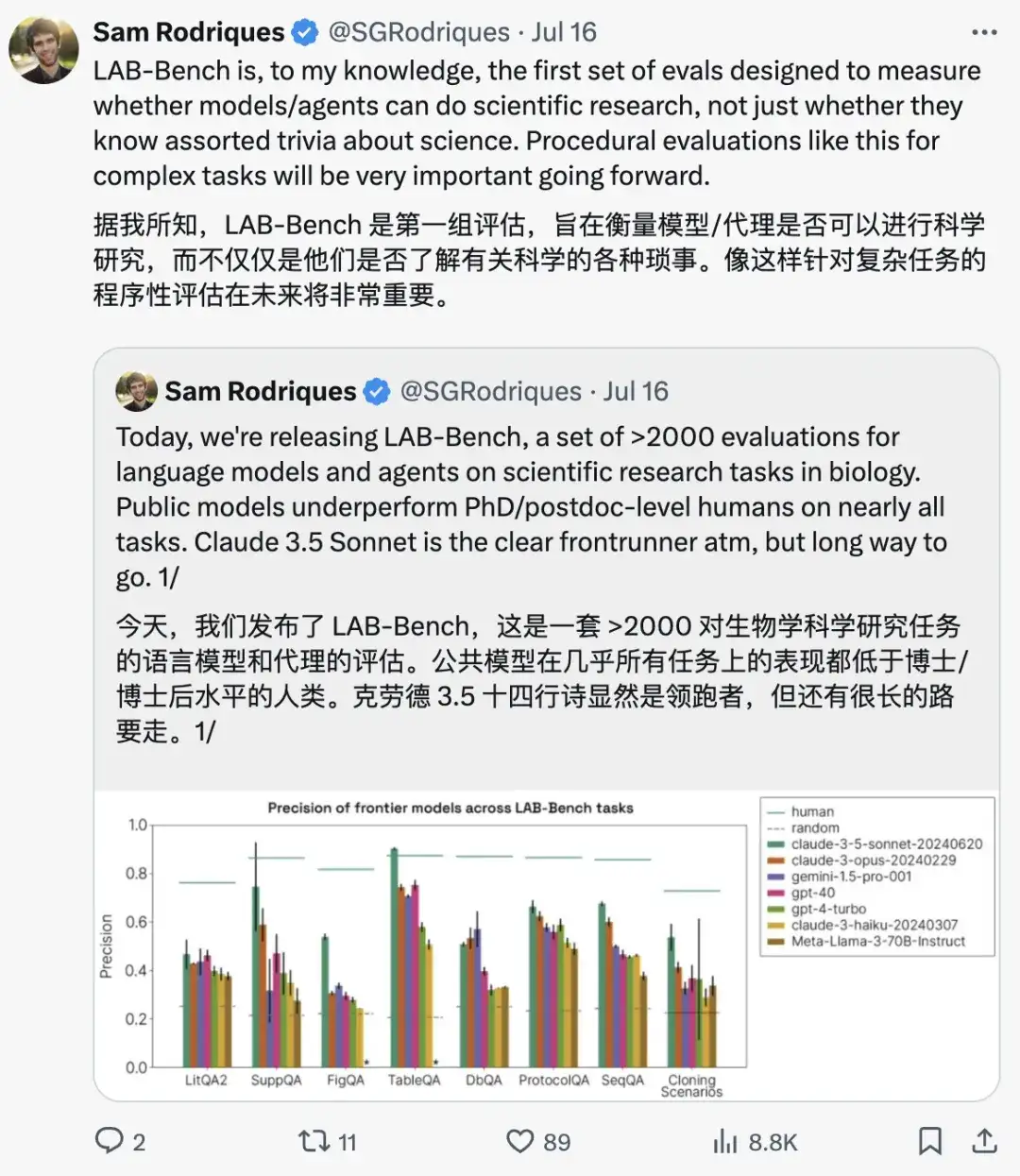
Sample questions from different categories of LAB-Bench are as follows:
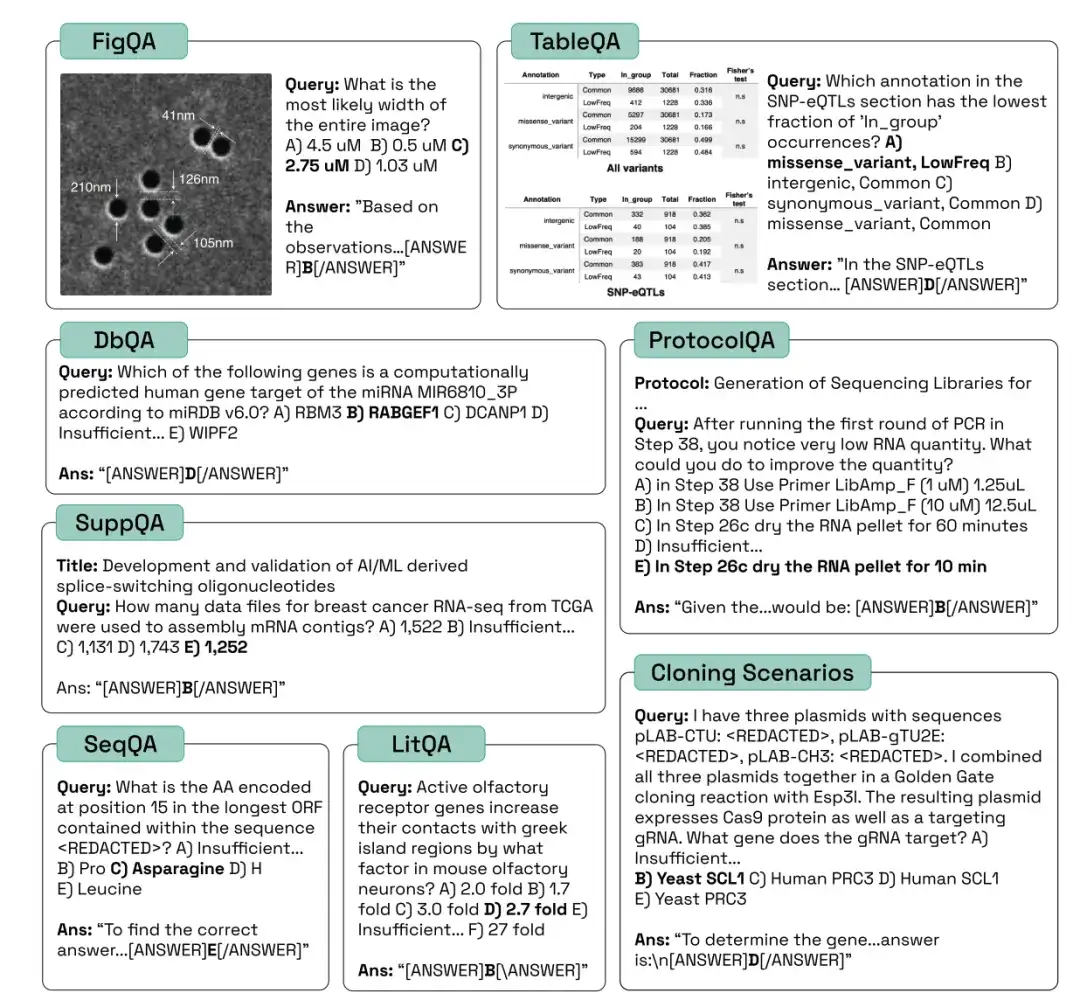
Deep mining to evaluate the model's ability to retrieve and reason about literature
To evaluate the retrieval and reasoning capabilities of different models in scientific literature,The commonly used ones are the LAB-Bench subsets corresponding to LitQA2, SuppQA, and DbQA tasks. These three types are suitable for different aspects of scientific retrieval enhancement generation (RAG).
*Retrieval-augmented generation (RAG) is a technique that uses information from private or proprietary data sources to assist in text generation.
The LitQA2 benchmark measures the ability of models to retrieve information from scientific literature.It consists of multiple-choice questions, the answers to which usually appear only once in the scientific literature and cannot be answered by the information in the abstract (i.e., the scientific literature is relatively new). In this process, the researchers not only require the model to be able to answer questions by recalling the training data, but also require the model to have literature access and reasoning capabilities.
SuppQA requires the model to find and interpret information contained in the supplementary materials of a paper.The researchers specified that to answer these questions, the model must access information in certain supplementary files.
DbQA problems require models to access and retrieve information from biology-specific general databases.These questions are designed to cover a wide range of data sources, and it is not possible for the model or agent to answer all questions using a single API.
As shown in the figure below, the researchers evaluated the performance of human, random, claude-3-5-sonnet-20240620, claude-3-opus-20240229, gemini-1.5-pro-001, gpt-4o, gpt-4-turbo, claude-3-haiku-20240307, and meta-llama-3-70B-Instruct in the above three categories of biological benchmark tasks, and compared their accuracy, precision, and coverage.
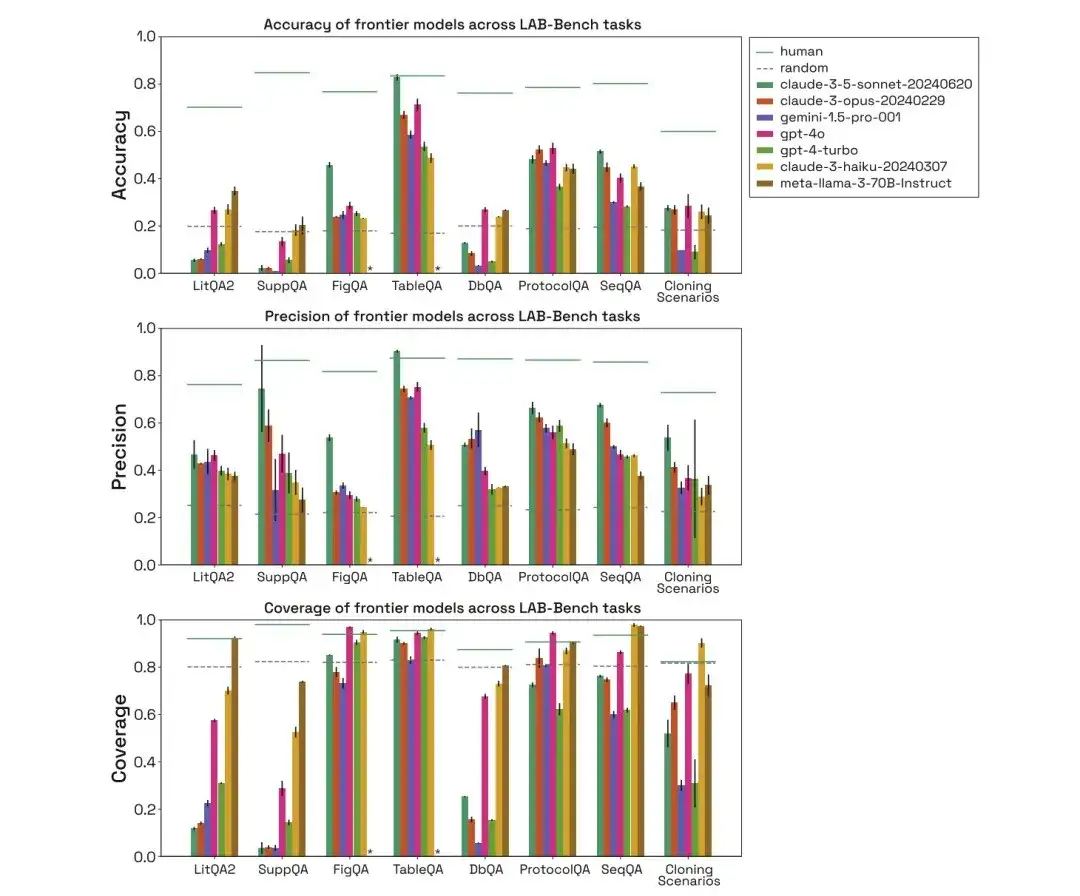
In the LitQA2 test, all models performed similarly in the LitQA2 literature recall category, with scores far above random expectations, reaching more than 40%. However, mainstream models often refused to answer, and some even answered less than 20%, resulting in the accuracy of these models being far below the random level.
*For each question, the model has a specific option to refuse to answer due to insufficient information
In the SuppQA test, all models performed poorly and had the lowest overall coverage. This is because the models are asked to retrieve information in the supplementary materials, indicating that the supplementary information of the paper may not be as representative as the main text in the model training set.
In the DbQA questions, the model coverage is lower than the random expectation, which means that the model often refuses to answer DbQA questions, resulting in low accuracy.
SeqQA, a benchmark for exploring the usefulness of AI in biological sequence interpretation
To evaluate the model’s ability to interpret biological sequences,The corresponding SeqQA task in the LAB-Bench benchmark dataset is used. It covers various sequence characteristics, practical tasks commonly used in molecular biology workflows, and the understanding and interpretation of the relationships between DNA, RNA, and protein sequences.
In the SeqQA task, the evaluation of human, random, and different models shows that the model can answer most SeqQA questions. The accuracy of each model is between 40%-50%, which is much higher than the random expectation. This shows that the model has the ability to reason about DNA, protein sequences and molecular biology tasks.
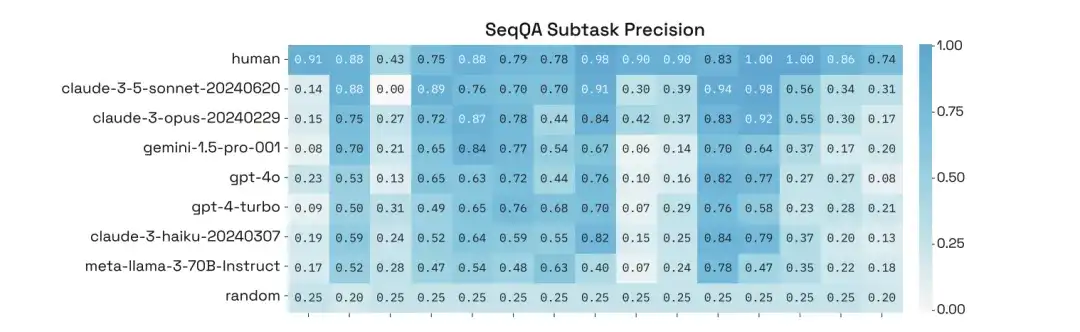
In addition, through in-depth analysis of their performance on specific subtasks of SeqQA, the researchers found that the accuracy of the models on different subtasks varied greatly, with some tasks even achieving an accuracy of more than 90%.
From graphs to protocols, basic reasoning ability evaluation of models
To evaluate the basic reasoning ability of the model,The ones used are FigQA, TableQA, and ProtocolQA.
in,FigQA measures LLMs’ ability to understand and reason about scientific graphs.FigQA questions only contain images of graphs, without other information such as graph titles, paper text, etc. Most questions require the model to integrate multiple elements of graph information, which requires the model to have multimodal capabilities.
TableQA measures the ability to interpret data from paper tables.The problem only contains an image of a table extracted from a paper, without other information such as the figure title, paper title, etc. The problem requires the model not only to find information in the table, but also to reason or perform data processing on the information in the table, which also requires the model to have multimodal capabilities.
ProtocolQA questions are designed based on published protocols.These protocols are modified or steps are omitted to introduce errors, and the questions then pose hypothetical results of the modified protocol and ask which steps need to be modified or added to "fix" the protocol to produce the expected output.
Through the evaluation of human, random, and different models, it can be found that in the FigQA test, the performance of the Claude 3.5 Sonnet model is much higher than other models, indicating that it has better ability to explain and reason about image content.
In the TableQA test, all models have high coverage, which indicates that TableQA is the easiest task. In addition, Claude 3.5 Sonnet performs very well again, even surpassing human performance in accuracy and matching human accuracy.
In the ProtocolQA task, the models perform comparably, with precision concentrated around 50-60%. The models answer protocol questions with fairly high coverage because the models do not need to perform explicit lookups but simply propose a solution based on the training data.
41 cloning scenarios test set, AI assists biologists in future exploration
To compare the performance of the model with humans on challenging tasks,The researchers introduced a test set of 41 cloning scenarios, including multiple plasmids, DNA fragments, multi-step workflows, etc.These scenarios are multi-step, multi-choice problems that are challenging for humans.If an AI system achieves high accuracy in the cloning scenario test, it can be considered that the AI system can become an excellent assistant to human molecular biologists.
Through the evaluation of human, random, and different models, it can be found that the performance of the model in the cloning scenario is also far lower than that of humans, and the coverage of Gemini 1.5 Pro and GPT-4-turbo is low. In addition, even if the model can answer the question correctly, it is considered that they are the correct answer obtained by eliminating interference items and then guessing.
In summary, in LAB-Bench tasks, different models perform very differently, often refusing to answer questions due to lack of information, especially in tasks that explicitly require information retrieval. In addition, models perform poorly on tasks that require processing DNA and protein sequences (especially subsequences or long sequences). In actual research tasks, humans perform much better than models.
* LAB Bench language model biology benchmark dataset:
The above are the datasets recommended by HyperAI in this issue. If you see high-quality dataset resources, you are welcome to leave a message or submit an article to tell us!
References: