The Catalytic Capacity Is Increased by 3.5 Times! The Chinese Academy of Sciences Team Developed a P450 Enzyme De Novo Design Method Based on the Diffusion Model P450Diffusion
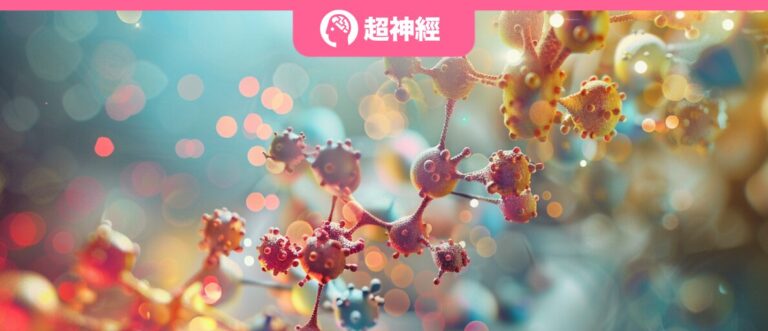
Cytochrome P450 enzymes are ubiquitous in almost all organisms and play an important role in various metabolic processes of life growth and development. As the most versatile biocatalyst in nature, P450 enzymes can not only catalyze more than 95% reported redox reactions, but also selectively oxidize inert carbon-hydrogen bonds under mild conditions.It is known as the "universal catalyst" in industrial applications.
Nowadays, directed evolution has been widely used to design new P450 enzymes with better performance. However, traditional methods usually require multiple rounds of random mutagenesis and high-throughput screening, making it difficult to explore the potential protein space in detail, either by performing actual experiments or by computer simulation calculations.
Although deep learning has made remarkable achievements in protein structure prediction, ideal functional design remains a huge challenge. When designing protein functions, it is difficult to collect enough high-quality functional data and train a complex model to create sequences with desired functions.Combining knowledge-driven techniques with powerful deep learning models to expand the natural protein sequence space,It may be an appropriate method for designing new P450 enzymes.
Recently, researchers Jiang Huifeng, Cheng Jian and others from the Tianjin Institute of Industrial Biotechnology, Chinese Academy of Sciences, analyzed the pocket design principles of the P450 enzyme flavonoid 6-hydroxylase (F6H).Developed a P450 enzyme de novo design method based on the diffusion model and pocket design principles, P450Diffusion.The related research was published in Research under the title "Cytochrome P450 Enzyme Design by Constraining the Catalytic Pocket in a Diffusion Model".
Based on P450 Diffusion, this study generated a new enzyme with better activity and higher stability than natural P450 enzymes. Compared with natural flavonoid 6-hydroxylase, the catalytic capacity of the new enzyme was increased by 1.3 to 3.5 times.
Research highlights:
* This study analyzed the origin mechanism of new functions in the evolution of P450 enzymes and proposed the "three-point fixation principle" of P450 enzyme substrate binding
* The catalytic capacity of the new enzyme generated by P450Diffusion is increased by 1.3 to 3.5 times
* This study provides new ideas for the design of new functional P450 enzymes under the framework of deep learning diffusion model. In the future, this method is expected to play a role in fields such as bioengineering and industrial catalysis, and promote the development and application of new enzymes.
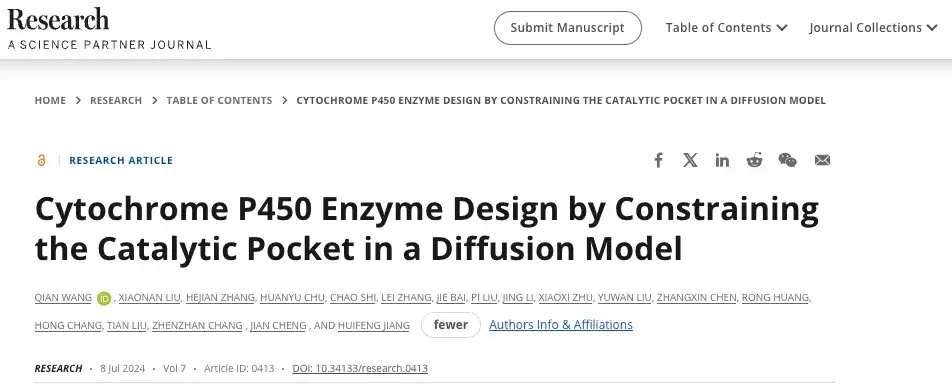
Paper address:
https://spj.science.org/doi/10.34133/research.0413
The open source project "awesome-ai4s" brings together more than 100 AI4S paper interpretations and provides massive data sets and tools:
https://github.com/hyperai/awesome-ai4s
Dataset: Collect and encode the dataset
To construct P450Diffusion, the researchers screened and analyzed all potential P450 enzymes from published P450 enzyme databases and public databases, filtered out sequences with a length greater than 560, and obtained 226,509 sequences as training data sets.
Subsequently, the researchers encoded the training dataset, with each amino acid in the protein sequence encoded as an 8-dimensional vector, and each batch of protein sequences encoded as a 64×1×560×8 vector - where 64 is the batch size, equal to the number of samples in the training data; 1 represents the channel size; 560 represents the maximum length of the protein sequence; and 8 represents the VHSE8 encoding vector for each amino acid in the protein sequence.
If the protein sequence is shorter than 560, the researchers added gaps until the length reached 560. In this case, they assigned a vector of eight zeros as the encoding for the gap.
Model architecture: P450 Diffusion, a de novo design method for P450 enzymes
The researchers took a flavonoid 6-hydroxylase (CYP706X1) from Erigeron breviscapus as an example. This enzyme belongs to the CYP706X subfamily and converts apigenin to breviscapine in the breviscapine biosynthesis pathway.
first,Through ancestral sequence reconstruction, back mutation experiments, progressive forward accumulation, and crystallographic analysis, the researchers identified the founding residues that make up the catalytic pocket, which are responsible for the innovation of the P450 enzyme gene function;Secondly,Through in-depth structural analysis, the researchers elucidated the design principles of the functionally innovative catalytic pocket;at last,It combines the catalytic pocket design principle with the denoising diffusion probability model that performs well in image generation, and designs an artificial P450 enzyme generation model P450Diffusion.
Step one: identify the founding residues that constitute the catalytic pocket and are responsible for the innovation of P450 enzyme gene function.
Among the identified CYP706 family P450 enzymes, only CYP706X subfamily P450 enzymes are able to catalyze flavonoid substrates, suggesting that the function of the P450 enzyme flavone 6-hydroxylase (F6H) may have been innovated de novo in the ancestor of the CYP706X subfamily.
To elucidate the molecular mechanism of the formation of the catalytic pocket with F6H function, the researchers proposed to analyze the changes in residue composition between the catalytic pockets of non-functional ancXY (the common ancestor of the CYP706X and CYP706Y subfamilies) and functional ancX (the common ancestor of the CYP706X subfamily). Within 8 Å from the active center, 16 of the 48 residues were different.
As shown in Figure A below, the residues of ancX and ancXY are colored cyan and magenta, respectively; and when all these 16 residues are replaced with the corresponding residues in ancX, the mutant (called ancXY-16) acquires the F6H function, as shown in Figure B below.
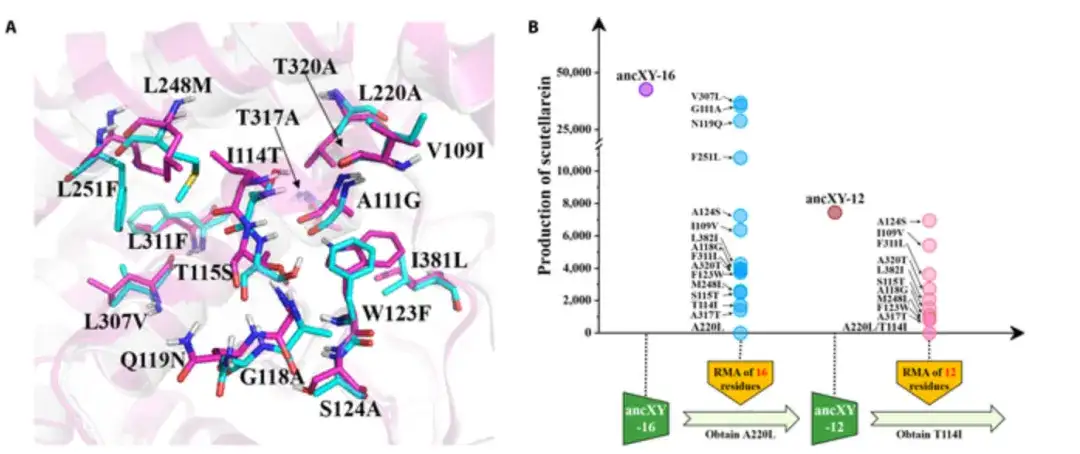
Given that the positions of residues in the catalytic pocket are different in three-dimensional space, not all residues contribute significantly to substrate recognition and binding. The researchers tried to find the founding residues in the catalytic pocket through a reverse mutation assay (RMA: assessing the mutation effect of each residue in the ancXY-16 catalytic pocket by returning it to its ancestral type). In order to identify the founding residues more quickly, the researchers also adopted a progressive forward accumulation (PFA) strategy to gradually add important mutations to ancXY until the mutant acquires F6H function.
Finally, the experiment found that the mutation of 5 amino acids (L220A/I114T/T317A/W123F/L248M) played a founder role (founding residue) in the functional innovation process of F6H from ancXY to ancX.
Step 2: Elucidate the design principles of the catalytic pocket with functional innovation.
By deeply analyzing the apigenin binding model in ancXY-5, the researchers further interpreted the potential mechanism of the five founding residues involved in functional innovation. Based on the mutations of the five founding residues, the catalytic pocket seems to follow the "three-point fixation" principle.
"Three-point fixation" refers to the key interactions with the three hubs in the apigenin molecule.These include: the 4'-OH (first hub) in the apigenin molecule is fixed by hydrogen bonds provided by T114, the "B" ring of apigenin (second hub) is fixed by π-stacking interactions between F123 and M248, and the 7-OH of apigenin (third hub) is fixed by hydrogen bonds with the iron-oxyl group of CpdI.
The model holds the substrate apigenin in a near-reaction conformation (NAC), maintaining the relative orientation between the apigenin reaction site and the CpdI iron-oxyl group at a favorable distance and angle (3.6 Å and 155°), thereby initiating the 6-hydroxylation reaction of apigenin during the catalytic process.
The researchers proposed that "three-point fixation" can be used as a catalytic pocket design principle for natural functional innovation of F6H, which also provides new ideas for designing P450 enzymes with desired functions.
Step 3: Combine the catalytic pocket design principle with the denoising diffusion probability model that performs well in image generation to design an artificial P450 enzyme generation model P450Diffusion.
The researchers combined the diffusion model with the design principle of the F6H catalytic pocket to design a P450 enzyme with the desired function from scratch, as shown in the figure below. The design process of the new P450 enzyme includes P450Diffusion model construction, sequence generation, screening and experimental verification.
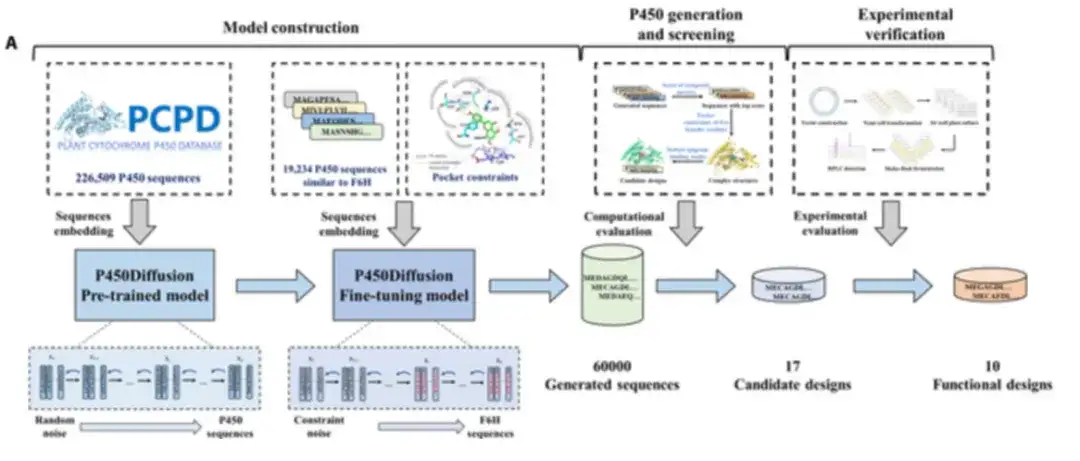
P450Diffusion mainly consists of two models, namely the pre-trained model and the fine-tuning model.
first,226,509 natural P450 enzyme sequences were collected to train the pre-trained P450 sequence diffusion model.
The pre-trained model consists of two subprocesses: a forward diffusion subprocess that gradually adds Gaussian noise to the representation of the P450 enzyme sequence until it becomes random noise; and a reverse generation subprocess that starts with random noise and gradually denoises the representation of the P450 enzyme sequence to generate new P450 sequences. After 150,547 rounds of training, the pre-trained diffusion model can generate a wide variety of sequences with similarities ranging from 20% to 50% to natural sequences.
Secondly,The pre-trained diffusion model was fine-tuned using 19,202 P450 enzyme sequences with significant similarity to the CYP706X subfamily to ensure that the generated sequences had a similar structural backbone to F6H.
In addition, constraints were imposed on the five founding residues, T114, F123, A220, M248, and A317, to ensure that the “three-point fixed” design principle could be reproduced in the de novo generated sequences. The model that combines training set fine-tuning with constrained generation is called the fine-tuning diffusion model.
Then,To improve the success rate of experimental validation, the researchers conducted a virtual screening of 60 000 generated sequences using three criteria: the calculated score of a comprehensive indicator for evaluating the quality of the generated sequences, the three-dimensional pocket constraints of the five founding residues, and the robustness of the apigenin binding mode.
After virtual screening,The researchers carefully selected 17 promising non-natural P450 enzymes for further exploration.
Research results: Catalytic capacity increased by 1.3 to 3.5 times
The researchers experimentally tested whether the sequences generated by P450Diffusion were authentic P450 enzymes and performed F6H functions.
After virtual screening, the researchers synthesized the selected 17 designs and expressed them in a yeast expression system.These designs showed sequence identity from 70% to 87%,This highlights their potential as new catalysts.
By feeding apigenin as a substrate and culturing the recombinant yeast for 4 days and performing HPLC analysis, the researchers found 10 designs with significant F6H activity, as shown in the figure below.
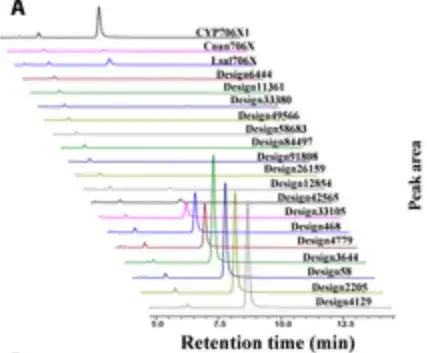
Surprisingly,Six designs showed 1.3- to 3.5-fold increases in catalytic capacity for oleandrin production compared with CYP706X1.As shown in the 6 bars on the right side of the figure below, the remaining 4 active designs also showed activities comparable to those of other natural F6H enzymes (i.e., Cnan706X and Lsal706X).
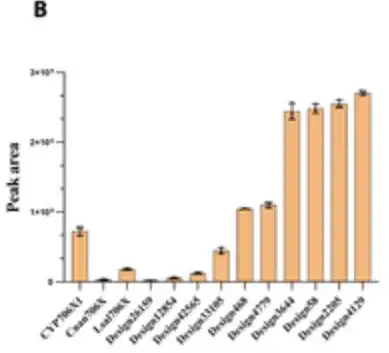
The results showed that P450Diffusion can not only capture the basic design principles of the F6H catalytic pocket and effectively generate P450 enzyme sequences with F6H activity,It is also possible to screen out P450 enzymes that are better than natural sequences from the P450 enzyme sequence space.
Data-driven, machine learning accelerates enzyme evolution
Enzymes existing in nature have a variety of functions and have been applied in industrial production and academic research. However, the properties and functions of many of these enzymes cannot fully meet the needs of application. Improving certain properties of these enzymes through modification is an important task of enzyme engineering.
in,Directed evolution can increase the rate of enzyme evolution by simulating the evolutionary process in nature.It has become a key technology for enzyme molecular modification. Directed evolution plays an important role in biocatalysis and drug design, but the large number of mutants generated by the randomness of mutations makes the ability of experimental screening face huge challenges. In recent years, emerging technologies such as artificial intelligence and big data processing have developed into important research methods in the field of biocatalysis. Among them, machine learning obtains the mapping of sequence/structure to enzyme function in a data-driven way, which helps to improve the efficiency of enzyme engineering.
Although the genes encoding the enzymes can be easily identified, in the vast majority of cases (more than 99%), the exact function of the synthetase is unknown because experimental characterization of enzyme function—that is, which starter molecules are converted into which specific end molecules by a particular enzyme—is extremely time-consuming.
To address this challenge, researchers at the University of Düsseldorf (HHU) developed a general machine learning model ESP for predicting enzyme-substrate pairs.Outperforms 91% in accuracy on independent and diverse test data. ESP can be successfully applied to the widely diverse enzymes and broad range of metabolites included in the training data, outperforming models designed for individual, well-studied enzyme families.
The study was published in Nature Communications in May 2023 with the title "A general model to predict small molecule substrates of enzymes based on machine and deep learning".
Paper link:
https://www.nature.com/articles/s41467-023-38347-2
Although de novo enzyme design is exciting, it is also challenged by the complexity of enzyme catalysis. Researchers at Enzymit, a cell-free enzyme engineering company, introduced CoSaNN (Conformational Sampling using Neural Networks), a new strategy for enzyme design.Harnessing advances in deep learning for structure prediction and sequence optimization.By controlling the conformation of the enzyme, researchers can expand chemical space beyond the scope of simple mutagenesis.
In addition, the team further developed SolvIT,This is a graph neural network trained to predict protein solubility in E. coli.As an additional optimization layer for producing highly expressed enzymes. With this approach, the researchers designed novel enzymes with superior expression levels, with 54% designed for expression in E. coli and improved thermostability, with more than 30% designed having a higher Tm than the template enzyme.
The study, titled "Context-Dependent Design of Induced-fit Enzymes using Deep Learning Generates Well Expressed, Thermally Stable and Active Enzymes", was published on the bioRxiv preprint platform in August 2023.
Paper link:
https://www.biorxiv.org/content/10.1101/2023.07.27.550799v3
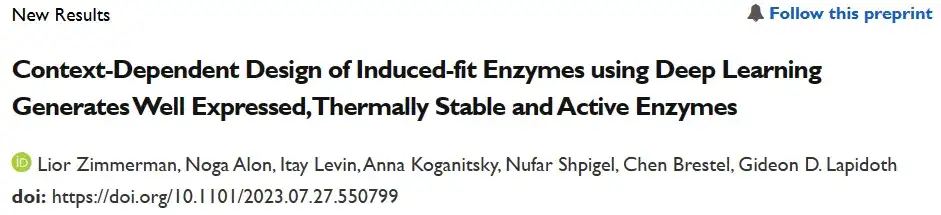
Data shows that the global market size of industrial enzymes alone will reach $7.4 billion in 2023. In the future, by leveraging the power of artificial intelligence and learning characteristic information about protein composition and evolution, researchers will be able to solve many types of enzyme engineering problems, such as predicting mutations with beneficial effects, optimizing protein stability, improving catalytic activity, etc. This will further reduce the cost of biomanufacturing and bring higher commercial value.
References:
1.https://spj.science.org/doi/10.34133/research.0413
2.https://www.cas.cn/syky/202407/t20240718_5026250.shtml
3.https://biotech.aiijournal.com/CN/10.13560/j.cnki.biotech.bull.1985.2022-0724
4.https://www.jiqizhixin.com/articles/2023-06-25-12
5.https://www.jiqizhixin.com/articles