Not Replacement, but Symbiosis! The Future of Meteorological Science Requires the Organic Combination of AI and Numerical Forecasting
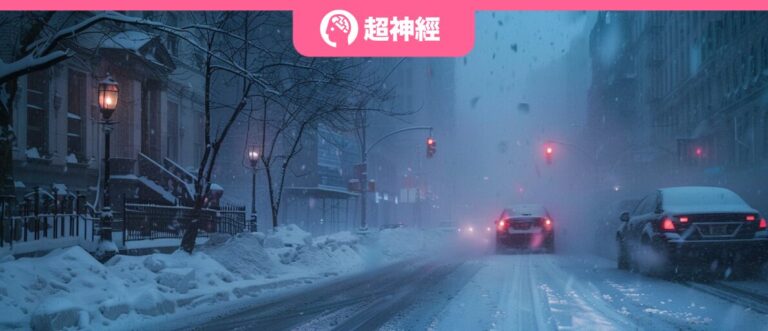
2022 is often regarded by industry insiders as the first year for AI to empower weather forecasting.
Since this year, the application of AI in the field of meteorology has shown explosive growth. A series of innovative methods and models such as NVIDIA DLWP and Google MetNet-2 have sprung up like mushrooms after rain. "First", "first" and "groundbreaking" results have frequently appeared at home and abroad. Especially in improving forecasting skills, AI has shown amazing results. In just a few months, it has achieved the goals that numerical forecasting took decades to achieve, or even better.
It should be noted that numerical forecasting has a history of 100 years. It has promoted the rapid development of atmospheric science for nearly half a century and is the mainstream model of modern weather forecasting. In some scenarios, the accuracy of numerical forecasting is surpassed by AI, which has also caused people in the industry to think and question:Is this classic method, which has been proven for a century, about to be caught up with, surpassed or even completely replaced by AI?
Huang Wei, deputy director of the Shanghai Typhoon Research Institute of the China Meteorological Administration, said: "In the foreseeable future,The organic combination of AI weather forecasting and traditional numerical forecasting is the most effective way to achieve breakthroughs in forecasting technology."
Indeed, there is a natural coupling relationship between numerical forecasting and AI. Numerical forecasting can provide explainable forecast results through physical and mathematical theories, and AI can use knowledge and experience to improve the speed and accuracy of forecast calculations. The complementarity between the two may be the key path for the future development of weather forecasting.
We attempt to help readers understand the complex relationship and potential possibilities between numerical forecasting from detail to surface by sorting out and analyzing the past and present of numerical forecasting and representative cutting-edge artificial intelligence methods.
From theory to practice, a century of exploration of numerical forecasting
In fact, before the advent of numerical forecasting,Extrapolation forecast based on weather maps was the mainstream means of weather forecasting at that time.
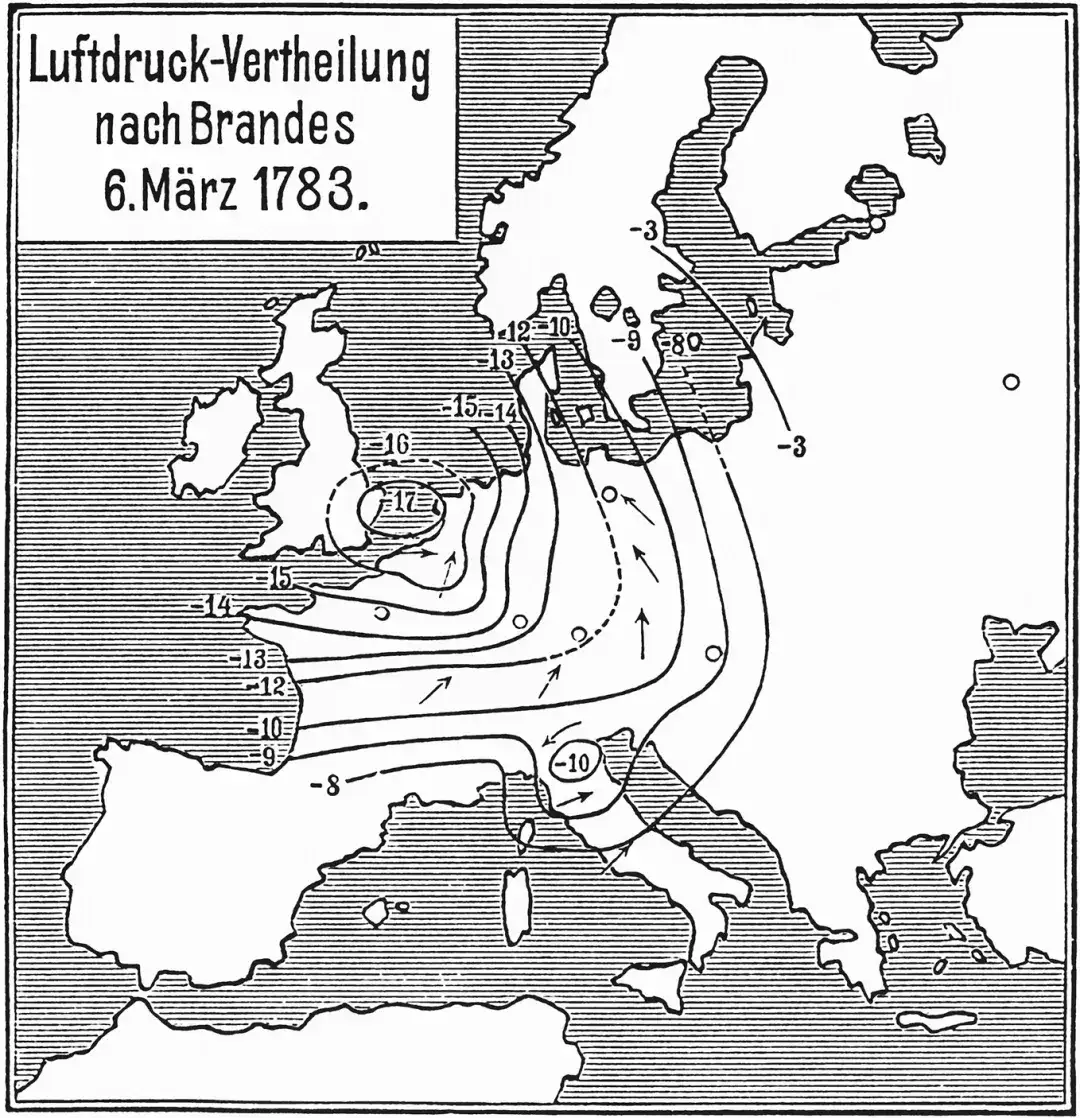
Fill in the locations of cities, observation stations, and major rivers, lakes, mountains and other geographical landmarks on the base map, and plot the meteorological elements of the same time around the world on this map to form a weather map. By analyzing the weather map and based on the principle that meteorological evolution is continuous in a short period of time, the current weather trend can be extended for a period of time to obtain the forecast result.
The extrapolated forecast based on weather maps cannot reflect the movement and change patterns within the atmosphere, and its forecast level is greatly limited.In order to obtain more accurate weather forecasts, meteorologists try to introduce mathematical physics methods to study the physical laws of weather evolution.A forecasting technique based on quantitative description was developed, which later became numerical weather forecasting.
With the help of computers, numerical forecasting has risen strongly
The movement trajectory of the atmosphere follows the basic theory of fluid mechanics. Norwegian meteorologist Pieknes believes that "the future state of the atmosphere is, in principle, determined by the initial state of the atmosphere, the atmospheric motion equation, the mass conservation equation, the state equation, the thermodynamic equation, etc." Therefore, people try to combine these influencing factors into a set of equations to reflect the state of atmospheric motion, and use the real-time meteorological elements of the atmosphere provided by the meteorological observation network as the input of the equation set, solve the variable values of the equation over time, and then obtain the future state of the atmosphere -This is the basic idea of numerical weather forecasting.
From 1916 to 1919, Dr. Richardson of the United Kingdom conducted the first actual calculation of numerical forecasting, but the experiment was a complete failure. He later concluded,This kind of prediction requires a lot of calculations.Even with the help of a manual calculator, it is difficult to achieve a forecast before the weather arrives. "Although the result of this attempt was not good, he showed the outside world the basic calculation process, steps, and problems faced in numerical weather forecasting, providing a good learning experience for future generations.
In 1946, the world's first electronic computer ENIAC was launched, with a computing speed of 5,000 addition operations per second. Since then, von Neumann, known as the "Father of Computers", has always been surrounded by outstanding meteorologists from all over the world. Their goal is toUse the newly invented electronic computers to make accurate numerical weather forecasts.
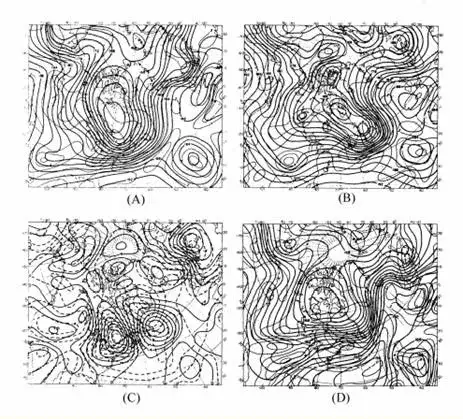
In 1950, American scientists Charney, Feyerfuss, and von Neumann, based on previous experience, successfully calculated a 24-hour forecast of 500 hPa weather conditions on ENIAC for the first time.This was the first successful numerical weather forecast.It is also a landmark event. Since then, research on numerical weather forecasting has entered a period of vigorous development, with numerous scientists and institutions at home and abroad devoting themselves to the development of this field.
China Meteorological Administration: Adhere to independent research and development and master four-dimensional variational data assimilation technology
In 1975,The European Centre for Medium-Range Weather Forecasts (ECMWF) was established.In the fourth year of its establishment, it released medium-term numerical weather forecast products, gradually establishing its leading position in the field of numerical weather forecasting in the world. Today, ECMWF has the most advanced numerical weather forecast system in the world, and works with the national meteorological and hydrological agencies of 34 member states and cooperating member states to design new service products, evaluate and diagnose forecast quality. It is understood that the meteorological agencies of member states have the privilege of receiving the numerical weather forecast products and services made by the center in real time every day. This service is also provided to customers in more than 30 countries through commercial channels.
However, China is not a member of ECMWF.
In the early stages of the development of my country's numerical forecasting model, it faced a choice: should we introduce the most advanced numerical forecasting from ECMWF, or develop our own numerical forecasting? Direct introduction saves time and effort, but the core forecasting technology of ECMWF, the four-dimensional variational data assimilation technology, has never been made public to other countries. "In order not to be strangled by European and American countries,China needs to master core technologies". Sun Jian, a member of the Global Numerical Weather Prediction System Engineering and Technology Team of the Earth System Numerical Prediction Center of the China Meteorological Administration, said.
* Three-dimensional variational data assimilation: Atmospheric state data at multiple times, such as temperature, humidity, air pressure, wind speed, etc., are assumed to be at the same time and calculated through numerical forecasting models to obtain the weather conditions at a certain time in the future.
* Four-dimensional variational data assimilation: Based on three-dimensional variational data assimilation, the time dimension information is added to link the atmospheric state data at multiple times, find the change relationship between the data, and then estimate the future weather through model calculations.
The National Climate Center was established in 1995. After ten years of research and development, the National Climate Center successfully launched the NCC climate prediction model system in 2005. As my country's first generation of short-term climate prediction dynamic climate model business system, it was quickly put into operation and played an immeasurable role in the country's disaster prevention and mitigation and meteorological support services.
Through continuous independent innovation and breakthroughs, my country's second-generation and third-generation short-term climate model forecasting business systems have been launched one after another, becoming an indispensable part of the modern climate business system. To further promote the development of meteorological forecasting technology, the China Meteorological Administration has established a numerical forecast innovation base and a numerical forecast center, and has achieved major breakthroughs in four-dimensional variational assimilation, model dynamic framework, and satellite data assimilation technology.
In 2021, my country's numerical weather forecast system won the National Science and Technology Progress Award again, becoming another milestone in the development of the industry. In January 2024, the China Meteorological Administration reported that its independently developed Global Assimilation Forecast System (CMA-GFS) V4.0 was officially put into operation. After this upgrade, the number of available forecast days in the Northern Hemisphere exceeded 8 days, and the global forecast spatial resolution was increased from 25 kilometers to 12.5 kilometers. China's assimilation technology has achieved international leading achievements.my country has become one of the few countries in the world to independently develop a global four-dimensional variational assimilation system.
Although numerical forecasting has made significant progress in the past few decades, even today's supercomputers still need several hours of simulation to complete a 3-day weather forecast in the face of massive amounts of observation data from satellite remote sensing, radar observations, aircraft observations, ship observations, and offshore buoys.Computing resources face great challenges in processing huge amounts of information.
The wave of science and technology has never stopped. With the rise of AI, this technology, which is "designed for processing big data", can bring faster computing speed, higher prediction accuracy and lower cost to weather forecasting, and has attracted widespread attention.
Solving three major problems, AI weather forecasting achieves double improvement in accuracy and speed
Weather forecasting based on machine learning can train prediction models based on historical meteorological data (including observational and analytical data). This model can identify and learn factors in the data set that are not easily expressed by explicit equations, thereby improving prediction accuracy.
However, there are still many challenges for machine learning models to make the leap to more accurate predictions.There are three major problems: iterative error accumulation, sparse high-resolution data, and smoothing of extreme weather forecasts.In recent years, both academia and industry have launched efforts to tackle these three major challenges, promoting the dual improvement of AI meteorological forecasting in both accuracy and speed of weather forecasts.
Pangu-Weather solves the problem of iterative error accumulation
In terms of solving the iterative error accumulation, in July 2023,Huawei Cloud researchers launched Pangu-Weather, a global AI weather forecast model.This model is the first AI method whose prediction accuracy exceeds that of traditional numerical forecasting models. The prediction speed can be increased by more than 10,000 times. The model has a horizontal spatial resolution of 0.25°×0.25° and a temporal resolution of 1 hour, covering 13 vertical layers. It can accurately predict fine-grained meteorological characteristics and predict meteorological results such as potential, humidity, wind speed, temperature, and sea level pressure in seconds.
Pangu-Weather solves the long-standing problem of insufficient accuracy in AI weather forecast models: since atmospheric phenomena are essentially 3D, AI weather forecast models based on 2D neural networks have certain limitations when processing uneven 3D weather data; in addition, data-driven AI methods lack mathematical and physical mechanism constraints, and will continue to accumulate iterative errors during long forecast iterations.
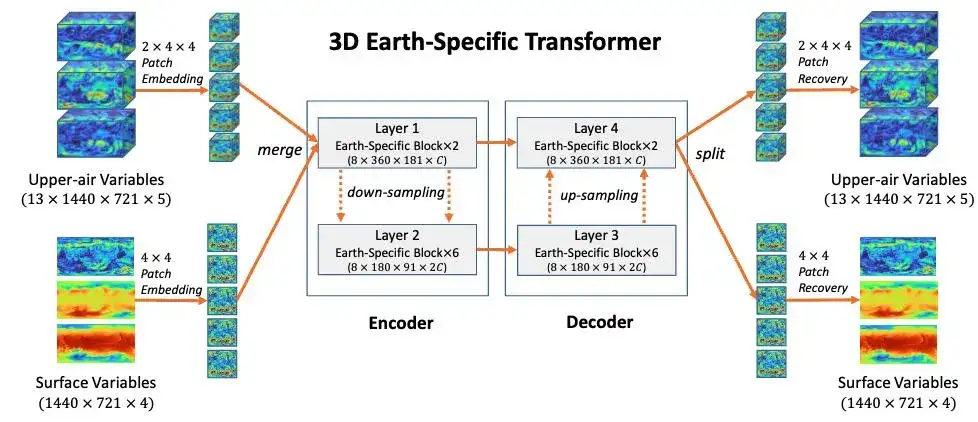
In response to this, the Pangu team creatively proposed a 3D Earth-Specific Transformer that adapts to the Earth coordinate system and used a hierarchical time-domain aggregation strategy to reduce the number of forecast iterations.This reduces the iteration error.The research was published in Nature under the title “Accurate medium-range global weather forecasting with 3D neural networks”.
Paper address:https://www.nature.com/articles/s41586-023-06185-3
Although Pangu-Weather has achieved 0.25° high-resolution forecasts, many meteorologists still hope to obtain higher-resolution weather forecasts to bring more detailed forecast results.
FengWu-GHR solves the problem of scarcity of high-resolution data
In solving the problem of high-resolution data sparsity, in March 2024,The Shanghai Artificial Intelligence Laboratory, in collaboration with the National Meteorological Center, the National Meteorological Information Center, Nanjing University of Information Science and Technology, the Hong Kong University of Science and Technology and other institutions, released the global high-resolution AI weather forecast model FengWu-GHR.This model is the first AI meteorological model to increase the forecast resolution to 0.09° (9km*9km), which can model and forecast medium-term weather at the 10-kilometer level. Compared with the previous 0.25°, the range accuracy is more than 7 times, and the effective forecast time is increased from 10.75 days to 11.25 days.
The research was published on arXiv under the title “FengWu-GHR: Learning the Kilometer-scale Medium-range Global Weather Forecasting”.
Paper address:https://arxiv.org/abs/2402.00059
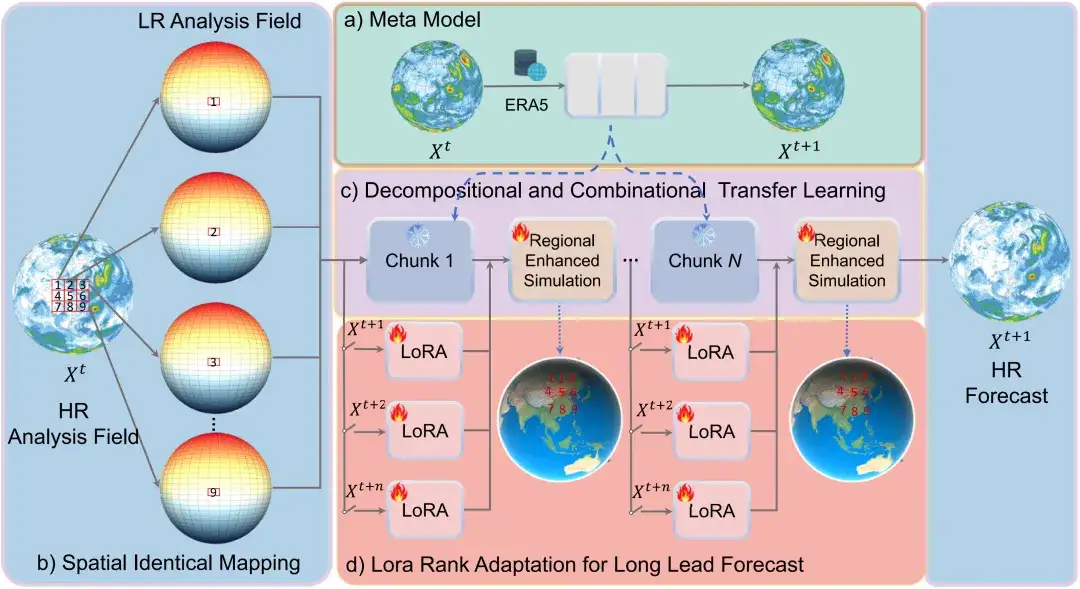
Limited by past observation technology, high-resolution data is scarce. The researchers innovatively proposed spatial consistency mapping and decoupled combined transfer learning techniques, which increase the secondary modeling of regional atmospheric activities by learning the prior knowledge of the pre-trained model on low-resolution reanalysis data and combining it with a small amount of high-resolution real-time operation analysis data.Solve the problem of scarcity of high-resolution data.
"Pangu-Weather used to use a separate training model at each prediction time point to solve the error accumulation problem. This method is effective, but its training cost is very high. We added a LoRA module to each step of the prediction process and trained each step with a small number of parameters. This is equivalent to having a new model for each step of the prediction, but only requiring a small number of parameters, which significantly reduces the computational cost." said Su Rui, a young researcher at the Shanghai Artificial Intelligence Laboratory.
“In addition, there is also very little data on extreme weather events. Data-driven AI models tend to smooth out results when predicting extreme weather. How to improve the accuracy of extreme weather predictions is also a difficult problem that needs to be solved in the future.”
Aurora Predicting extreme weather 5,000 times faster
In solving the problem of smoothing extreme weather forecasts, in May 2024,Microsoft has released Aurora, its first large-scale atmospheric base model.The model was trained on more than one million hours of diverse weather and climate simulations. By running at a high spatial resolution of 0.1° (about 11 kilometers at the equator), it can capture the complex details of atmospheric movement and provide accurate high-resolution weather forecasts for 10 days in less than 1 minute. Its computing speed is 5,000 times faster than the most advanced numerical forecast system IFS.
Even more surprising is that the model can improve the ability to predict extreme weather, such as the rapid deterioration of severe weather events such as Storm Ciaran. The study was published on arXiv under the title "Aurora: A Foundation Model of the Atmosphere".
Paper address:https://http://arxiv.org/abs/2405.13063
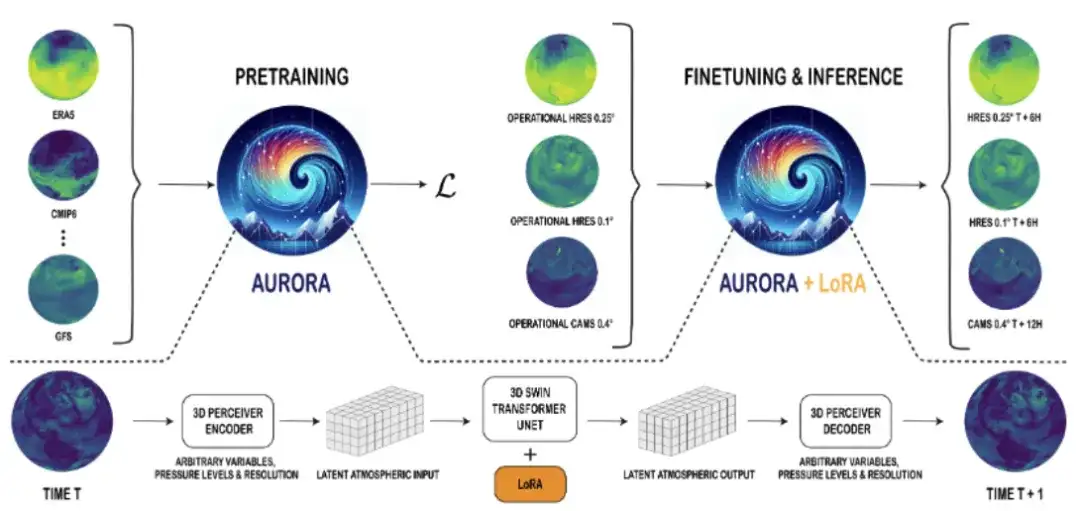
Aurora is designed to handle heterogeneous gold standard inputs and generate forecasts at different resolutions and fidelity. The model consists of a flexible 3D Swin Transformer and Perceiver-based encoders and decoders that can process and predict atmospheric variables across different spatial and pressure levels.
By pre-training on a massive and diverse dataset and then fine-tuning it for a specific task, Aurora can capture the complex patterns and structure of the atmosphere.Even when fine-tuned for specific tasks with limited training data, such as extreme weather, it still has excellent predictive capabilities.
In addition to the three achievements mentioned above, the emergence of many AI meteorological models, such as NVIDIA's FourCastNet, Google DeepMind's GraphCast, Alibaba's SwinVRNN, NowcastNet released by Tsinghua University and the China Meteorological Administration, and Fuxi from Fudan University, are constantly expanding the boundaries of AI meteorological forecasting.
It is worth mentioning that in order to further accelerate the application of artificial intelligence in the field of meteorological business, in May 2024, my country Meteorological Administration released the "Artificial Intelligence Meteorological Large Model Training Special Data Catalog", which disclosed 6 big data resources/products, including the 1.5 version of the China Meteorological Administration's global atmospheric reanalysis product, the 4.0 version of the China Meteorological Administration's Global Assimilation Forecast System (CMA-GFS) global weather model product, the China Meteorological Administration's global marine meteorological real-time analysis product, the global tropical cyclone data set, the global ground compilation data set, and the global upper air compilation data set. This move aims to promote convenient access to data resources by all sectors of society to support and accelerate their research and development and innovation in the application of artificial intelligence technology, especially in the field of meteorology.
It can be said that AI weather forecasting is in a golden period of rapid development both in terms of technology and policy, and has received high attention from "government, industry, academia and research".
AI combined with traditional numerical forecasting can go further
Artificial intelligence, as the current technology leader and darling of all fields, is regarded as a new force capable of subverting the field of atmospheric science.However, the explainability and credibility of AI are still lacking."Black box" AI models obtain information directly from massive amounts of data and do not need to comply with the laws of physics, which makes it difficult for meteorologists to understand the driving force behind AI forecasts.
Focusing on the traditional numerical forecasting method, it is based on meteorological dynamics theory, expressed mathematically and integrated with partial differential equations, and implemented with fluid dynamics programming techniques. It can provide highly reliable and explainable forecast results, which is a very necessary supplement to AI methods.
In fact, Chinese meteorologists are actively engaged in cutting-edge research on the integration of AI and traditional numerical forecasting. In May 2024, Xu Hongxiong's team, a researcher at the State Key Laboratory of Disaster Weather at the Chinese Academy of Meteorological Sciences, published a groundbreaking research result. They successfully combined the Pangu-weather weather AI forecasting model with the regional numerical forecasting model WRF-ARW. This is the first application of an AI weather model in driving regional numerical forecasting. It not only captures the key physical processes of extreme weather events, but also extends the effective forecast period of 400 mm precipitation to 8.5 days, significantly improving forecasting skills.
The study, titled “Improvement of disastrous extreme precipitation forecasting in North China by Pangu-weather AI-driven regional WRF model,” was published in Environmental Research Letters.
Paper link:
https://iopscience.iop.org/article/10.1088/1748-9326/ad41f0
With the further development of weather forecasting, it is becoming a consensus among most experts to combine AI with traditional numerical forecasting. AI may not be able to completely become the new leader in understanding the weather, rain, snow, temperature and temperature changes. The classic numerical forecasting method that has been verified for a hundred years also needs to keep pace with the times and continue to iterate in the context of frequent extreme weather and the difficulty of accurate prediction. Only when the old and new methods complement each other can we hope to incubate and derive a more reliable and efficient composite method in the face of unknown challenges. This may be the best way to forecast weather in the future.
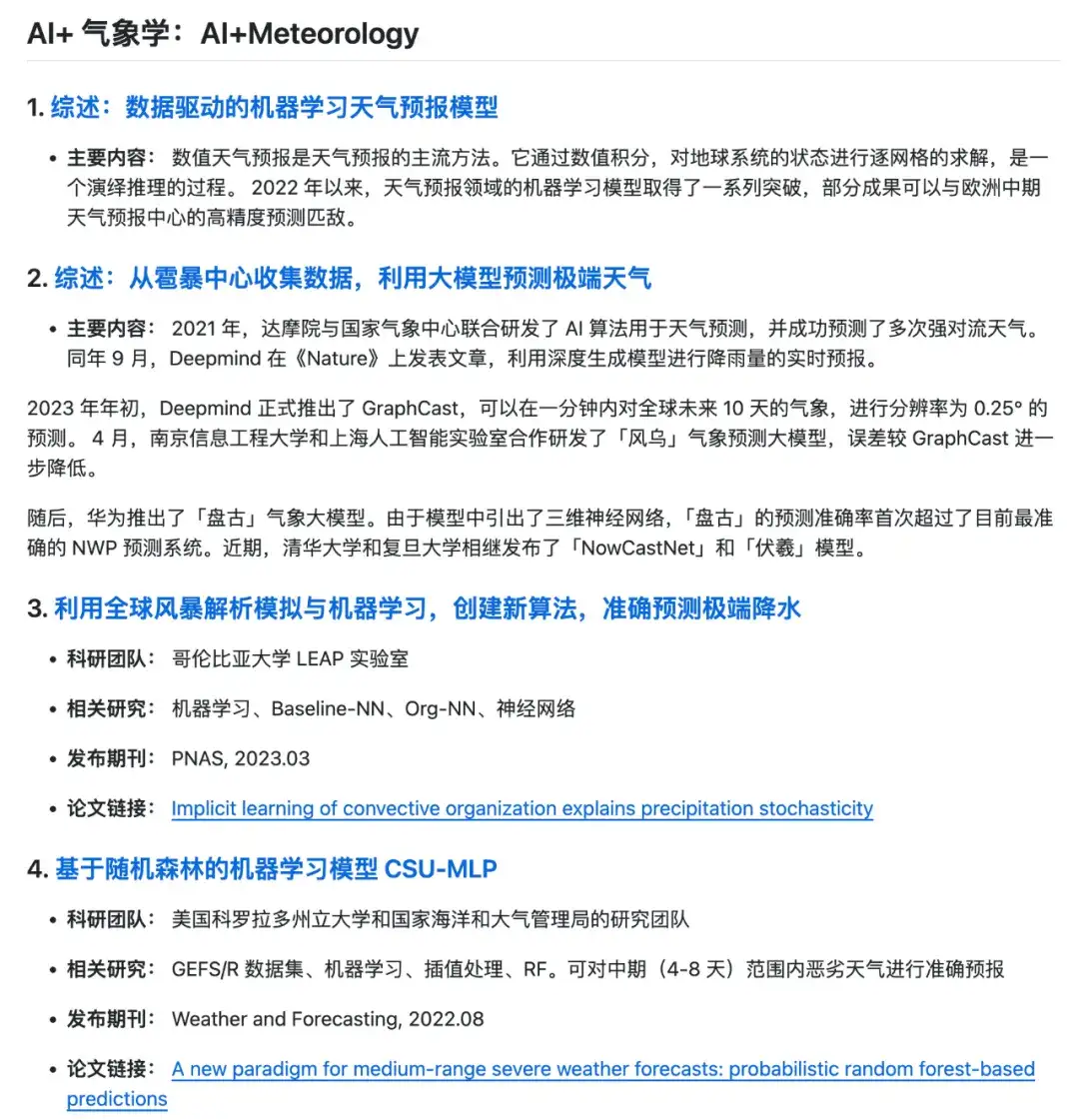
HyperAI continues to track AI4S cutting-edge achievements.The open source project "awesome-ai4s" brings together more than 100 AI4S paper interpretations.The AI+meteorology topic is summarized as follows:
https://github.com/hyperai/awesome-ai4s
References:
https://iap.cas.cn/gb/kxcb/kpwz/202004/t20200417_5551357.html
https://www.metax-tech.com/en/ndetail/12503.html
http://paper.people.com.cn/rmzk/html/2024-04/29/content_26056178.htm
https://www.eurekalert.org/news-releases/1007746