Improving the Accuracy of House Price Prediction! The First Author of the Paper Explains: Neural Networks Provide a New Explanation for the Spatial Heterogeneity of House Prices
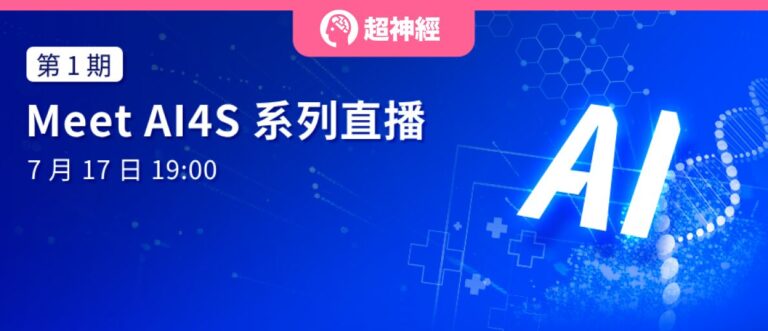
In July 2023, the "China AI for Science Innovation Map Research Report" compiled by the Chinese Institute of Scientific and Technological Information, the Ministry of Science and Technology's Next Generation Artificial Intelligence Development Research Center and relevant research institutions was officially released. The data showed thatmy country has the highest number of AI for Science papers published and is actively promoting the development of AI for Science around the world.
In order to further promote the universalization of AI4S, the scientific research results of academic institutions will be further reduced in the dissemination barriers and shared with a wider range of industry scholars, technology enthusiasts and industrial units.HyperAI has planned the "Meet AI4S" series of live broadcasts, inviting researchers or related units who are deeply engaged in the field of AI for Science to share their research results and methods in the form of videos.
The first episode of the "Meet AI4S" series will be officially launched at 19:00 on July 17. We are honored to invite Ding Jiale, a doctoral student in remote sensing and geographic information systems at Zhejiang University, who will give a speech titled "Neural Networks Provide New Explanations for Spatial Heterogeneity of Housing Prices".The design ideas and application scenarios of the model are introduced in an easy-to-understand manner, and the spatial regression analysis method of geographically weighted regression is further shared.
Click to schedule a live broadcast:Meet AI4S Series Live Broadcast Episode 1 | Neural Networks Provide New Explanations for Spatial Heterogeneity of Housing Prices
Guest Introduction
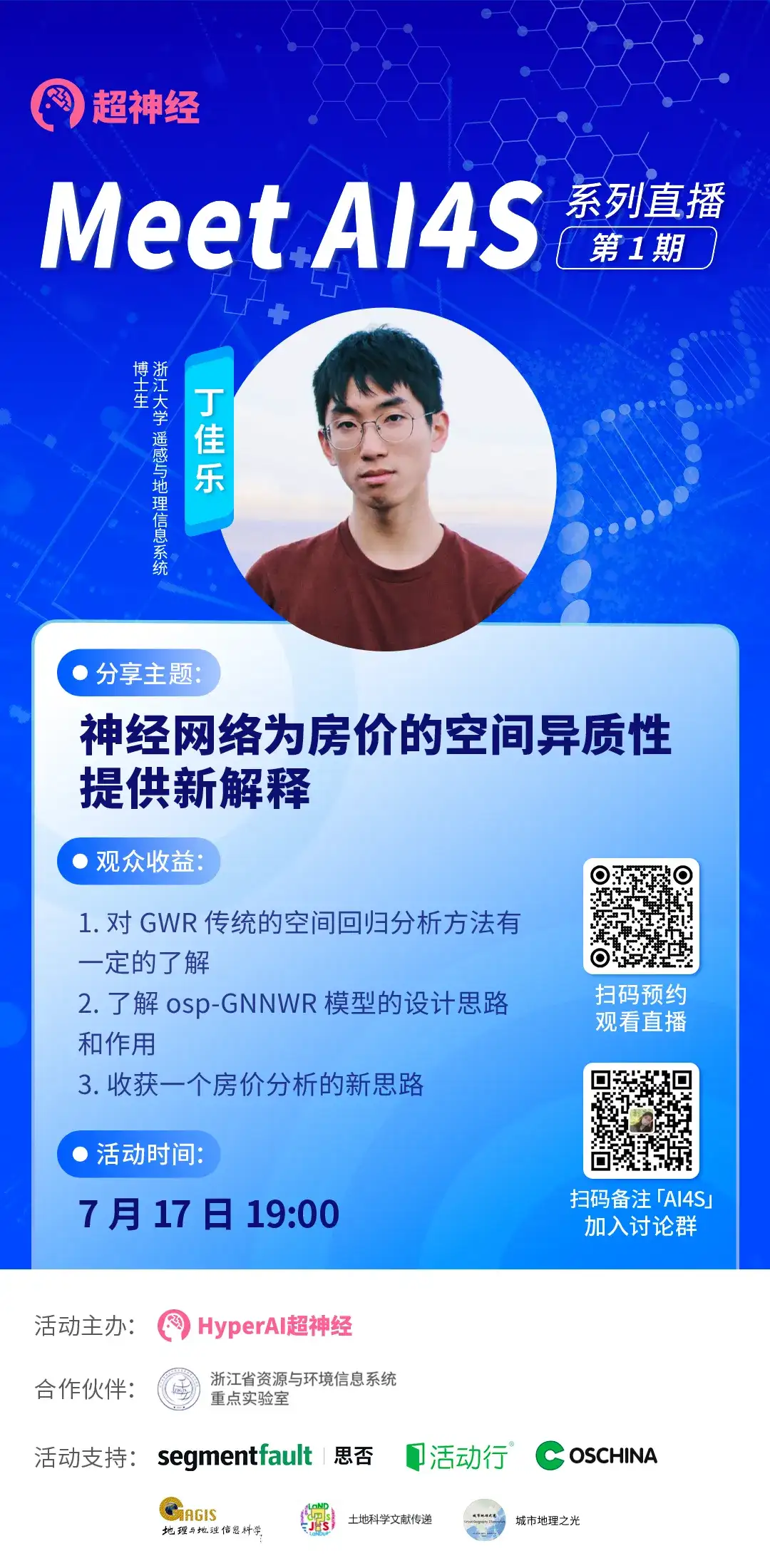
Introduction
In order to describe the spatial non-stationarity of the regression relationship between geographic elements at different spatial locations, spatial regression models such as geographically weighted regression give higher weights to samples that are spatially closer to each other to establish local regression relationships according to the first law of geography. However, in complex urban scenes, simple straight-line distance cannot fully reflect the true spatial proximity.
We use a simple neural network model to optimize the expression of spatial proximity while retaining the spatial interpretability of the regression results, thereby achieving higher modeling accuracy.
In addition, we have also open-sourced a spatiotemporal intelligent regression model library, which contains the source code of the GNNWR, GTNNWR models and other derivative models, model usage tutorial notes, and published Python wheels.
Project address:
https://github.com/zjuwss/gnnwr
Live broadcast harvest
1. Talk with the core research members of the team to gain an in-depth understanding of the design ideas of the team's osp-GNNWR model and its operating logic and role in house price prediction.
2. Gain a deeper understanding of the usage and shortcomings of the traditional spatial instability modeling method - Geographically Weighted Regression (GWR).
3. Advance from a novice in housing price analysis, understand the underlying logic of the model in predicting housing prices, and gain new ideas for housing price analysis.
Paper Review
The osp-GNNWR model released by the team has been published in the International Journal of Geographical Information Science, a well-known journal in the field of geographic information science, under the title "A neural network model to optimize the measure of spatial proximity in geographically weighted regression approach: a case study on house price in Wuhan".
Paper address:
https://www.tandfonline.com/doi/full/10.1080/13658816.2024.2343771
Research highlights
* By introducing an optimized spatial proximity metric and integrating it into the neural network architecture, the applicability of geographically weighted regression in the study of spatial distribution of geographic processes such as housing prices is effectively improved
* Through the study of simulated data sets and empirical cases of housing prices in Wuhan, the model proposed in the paper is proven to have better global performance and can more accurately describe complex spatial processes and geographical phenomena
* Opens up new avenues for studying how to customize spatial proximity metrics to improve the performance of various geospatial regression models
Dataset acquisition
The research team used a spatially heterogeneous simulation data set and an actual data set containing 968 empirical cases of different housing prices in Wuhan, which greatly ensured the quality of the data.
Construction of the osp-GNNWR model
The construction of the osp-GNNWR model is divided into two steps: the first step is to obtain the optimized spatial proximity measure (OSP); the second step is to further combine OSP with GNNWR to finally construct the osp-GNNWR model.
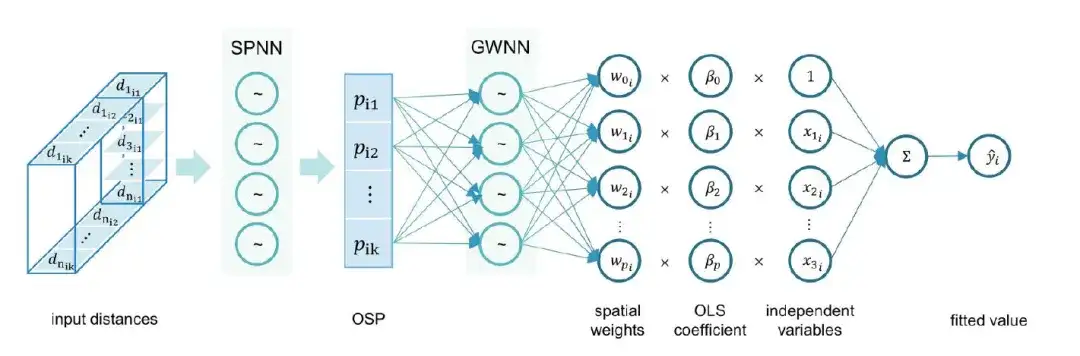
First, in order to obtain more accurate spatial proximity measurements in complex geographic analysis, the research team integrated multiple distance measurement methods including Euclidean distance, Manhattan distance, and travel time to optimize spatial proximity (OSP).
Subsequently, the researchers further combined OSP with GNNWR and finally constructed the osp-GNNWR model. The GNNWR model combines ordinary least squares (OLS) and neural networks to estimate spatial non-stationarity based on a concept similar to geographically weighted regression (GWR), which is suitable for solving spatial non-stationarity problems in various fields with complex geographical processes.
Therefore, the osp-GNNWR model finally constructed has potential advantages in depicting the spatial heterogeneity of real-world geographical processes. In short, the model will improve the accuracy of house price prediction, help the management and construction of the real estate environment, and improve people's housing happiness index.
Zhejiang Provincial Key Laboratory of Resources and Environmental Information Systems
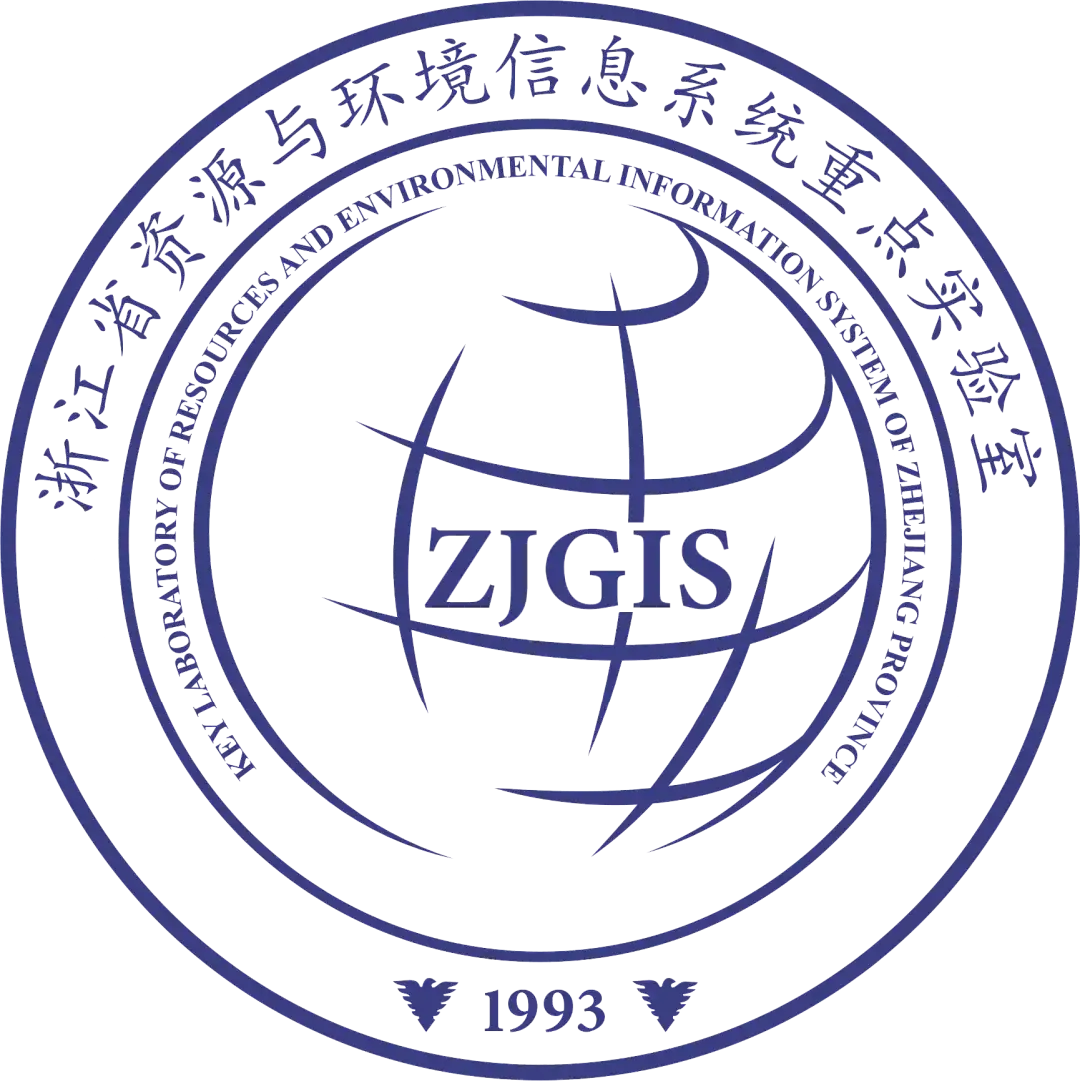
The Zhejiang Provincial Key Laboratory of Resources and Environmental Information Systems was approved for establishment in November 1993 and completed and opened in April 1995. It is mainly oriented to national high-tech fields such as digital earth and geographic information systems, remote sensing and global positioning system technologies. The laboratory has undergraduate programs in geographic information science, and master and doctoral programs in remote sensing and geographic information systems.
The laboratory conducts research work at three levels: basic theoretical methods, core key technologies, and major engineering applications. Major basic research focuses on the basic theories and original methods of Earth system big data, surface environmental change processes, and human-land coupling. Core key technology research focuses on cutting-edge directions such as spatiotemporal big data storage management, high-performance GIS, three-dimensional GIS visualization, and intelligent GIS deep analysis and mining. It has been used to improve the application efficiency and value of ultra-massive, high-precision, and highly complex spatiotemporal data, and solve the current "neck" bottleneck problem in the field of geographic information and remote sensing; major engineering application research focuses on natural resources, oceans, surveying and mapping, agriculture, forestry, transportation, environmental protection, disaster prevention and mitigation, and other fields. It carries out software development and results transformation for major national strategies and social application needs, aiming to solve practical application needs.
Meet AI4S Live Series
HyperAI (hyper.ai) is China's largest search engine in the field of data science. It focuses on the latest scientific research results of AI for Science and tracks academic papers in top journals such as Nature and Science in real time. So far, it has completed the interpretation of more than 100 AI for Science papers.
In addition, we also operate the only AI for Science open source project in China, awesome-ai4s.
Project address:
https://github.com/hyperai/awesome-ai4s
In order to further promote the popularization of AI4S, further reduce the dissemination barriers of scientific research results of academic institutions, and share them with a wider range of industry scholars, technology enthusiasts and industrial units, HyperAI has planned the "Meet AI4S" video column, inviting researchers or related units who are deeply engaged in the field of AI for Science to share their research results and methods in the form of videos, and jointly discuss the opportunities and challenges faced by AI for Science in the process of scientific research progress and promotion and implementation, so as to promote the popularization and dissemination of AI for Science.
Click to schedule a live broadcast: