Meet AI4S Live Preview | New Ideas for House Price Analysis: Neural Networks Directly Address Spatial Heterogeneity in Complex Geographical Environments
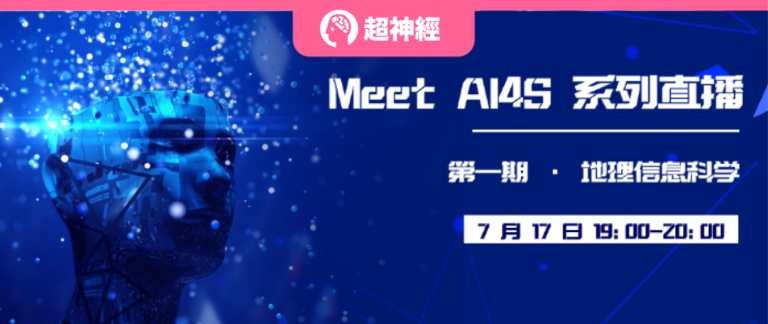
In recent years, the real estate market has experienced ups and downs, and housing prices have become an important factor affecting residents' happiness. Most families need to face the difficult decision of "whether to buy a house, when to buy a house, where to buy a house, and what house to buy". The answer to each question is closely related to the fluctuation of housing prices to some extent.
In recent years, the differentiation of housing prices between cities in my country has become increasingly prominent. Even within the same jurisdiction of the same city, housing prices in different areas can vary greatly due to differences in community environment, school districts, supporting commercial facilities and other factors.This is the "spatial heterogeneity" often mentioned in geographic information research.Capturing the spatial specificity of housing prices is crucial for predicting their changing trends.
In view of this,Researchers from the GIS Laboratory of Zhejiang University built the osp-GNNWR model.The spatial proximity measure (OSP) is combined with the geographic neural network weighted regression method, and the neural network method is innovatively introduced to improve the accuracy of the model in predicting housing prices.
HyperAI is honored to invite the first author of the paper, Ding Jiale, a doctoral student in Remote Sensing and Geographic Information Systems at Zhejiang University,On July 17 at 19:00, in the form of online live broadcast,Introduce the design ideas and application scenarios of the model, and further share the spatial regression analysis method of geographically weighted regression.
Click to schedule a live broadcast:
Guest Introduction
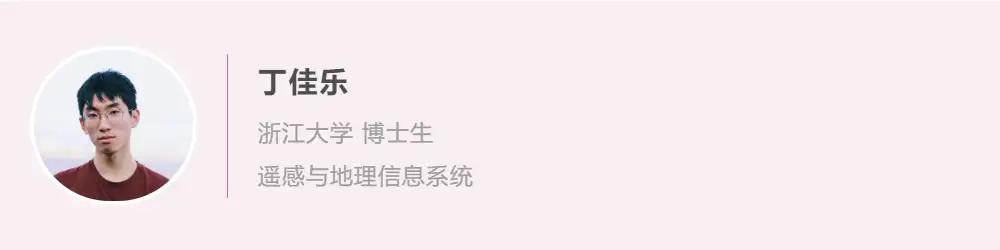
Share topic:
Neural network provides new explanation for spatial heterogeneity of housing prices
Contents:
In order to describe the spatial non-stationarity of the regression relationship between geographic elements at different spatial locations, spatial regression models such as Geographically Weighted Regression (GWR) give higher weights to samples that are spatially closer to each other to establish local regression relationships based on the first law of geography. However, in complex urban scenes, simple straight-line distance cannot fully reflect the true spatial proximity.
We use a simple neural network model to optimize the expression of spatial proximity while retaining the spatial interpretability of the regression results, thereby achieving higher modeling accuracy.
In addition, we have also open-sourced a spatiotemporal intelligent regression model library, which contains the source code of the GNNWR, GTNNWR models and other derivative models, model usage tutorial notes, and published Python wheels.
Project address:
https://github.com/zjuwss/gnnwr
By watching this sharing session, you will learn:
1. Have a certain understanding of GWR's traditional spatial regression analysis method
2. Understand the design ideas and functions of the osp-GNNWR model
3. You can gain a new idea for house price analysis
Zhejiang Provincial Key Laboratory of Resources and Environmental Information Systems
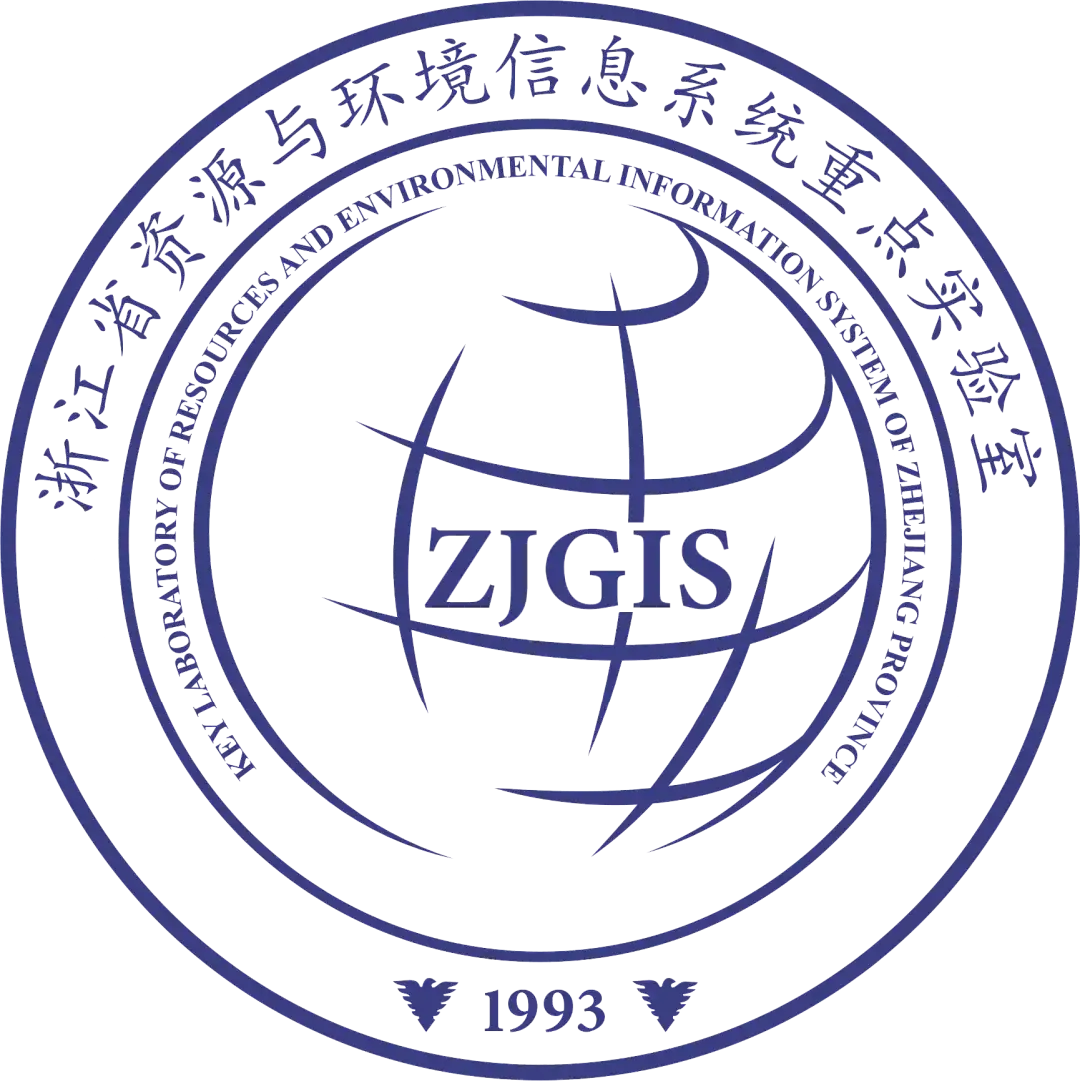
The Zhejiang Provincial Key Laboratory of Resources and Environmental Information Systems was approved for establishment in November 1993 and completed and opened in April 1995. It is mainly oriented to national high-tech fields such as digital earth and geographic information systems, remote sensing and global positioning system technologies. The laboratory has undergraduate programs in geographic information science, and master and doctoral programs in remote sensing and geographic information systems.
The laboratory conducts research at three levels: basic theoretical methods, core key technologies, and major engineering applications.
Major basic research focuses on the basic theories and original methods of Earth system big data, surface environmental change processes and human-land coupling. * Core key technology research focuses on cutting-edge directions such as spatiotemporal big data storage management, high-performance GIS, three-dimensional GIS visualization, and intelligent GIS deep analysis and mining, to improve the application efficiency and value of ultra-massive, high-precision, and highly complex spatiotemporal data, and solve the current bottleneck problems in the field of geographic information and remote sensing.
Major engineering application research focuses on natural resources, ocean, surveying and mapping, agriculture, forestry, transportation, environmental protection, disaster prevention and mitigation and other fields, and carries out software development and results transformation in response to major national strategies and social application needs, aiming to solve practical application needs.
Meet AI4S Live Series
HyperAI (hyper.ai) is China's largest search engine in the field of data science. It focuses on the latest scientific research results of AI for Science and tracks academic papers in top journals such as Nature and Science in real time. So far, it has completed the interpretation of more than 100 AI for Science papers.
In addition, we also operate the only AI for Science open source project in China, awesome-ai4s.
Project address:
https://github.com/hyperai/awesome-ai4s
In order to further promote the universalization of AI4S, the scientific research results of academic institutions will be further reduced in the dissemination barriers and shared with a wider range of industry scholars, technology enthusiasts and industrial units.HyperAI has planned the "Meet AI4S" video column, inviting researchers or related units who are deeply engaged in the field of AI for Science to share their research results, methods and ideas in the form of videos.Together we will discuss the opportunities and challenges that AI for Science faces in scientific research progress and implementation, and promote the popularization and dissemination of AI for Science.