How Do AI Practitioners Do Science? Zhou Hao From Tsinghua University AIR: Cross-border Exploration From Text Generation to Protein Design
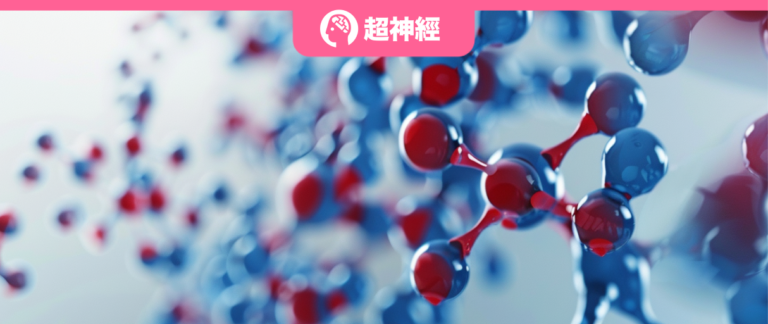
Recently, at the "AI for Science" forum of the Beijing Zhiyuan Conference,Zhou Hao, associate researcher at the Institute of Intelligent Industries of Tsinghua University, gave a speech on the theme of "Generative Artificial Intelligence for Scientific Discovery".HyperAI has organized and summarized Professor Zhou Hao’s in-depth sharing without violating the original intention.
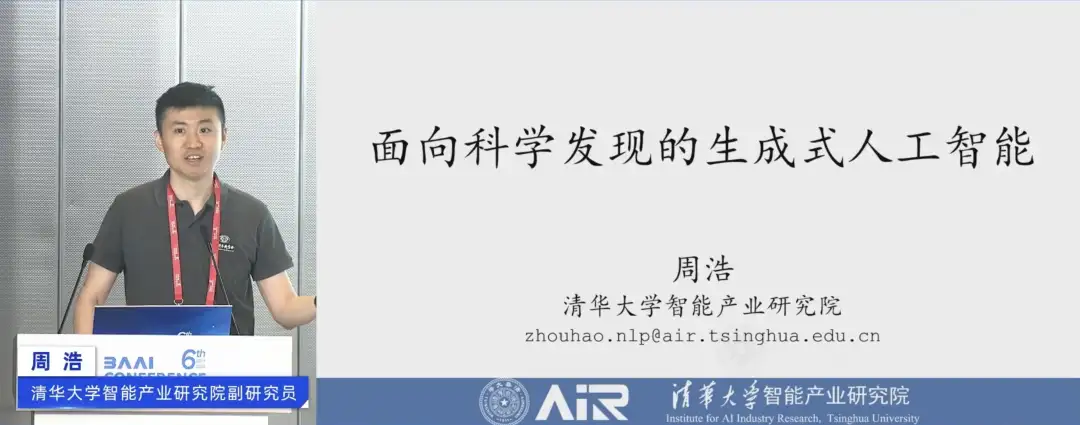
Cross-border exploration from text generation to molecular design
In this speech, Professor Zhou Hao mainly elaborated on three aspects: generative artificial intelligence for complex symbols, the challenges faced by micro-sample generation, and current specific research content.
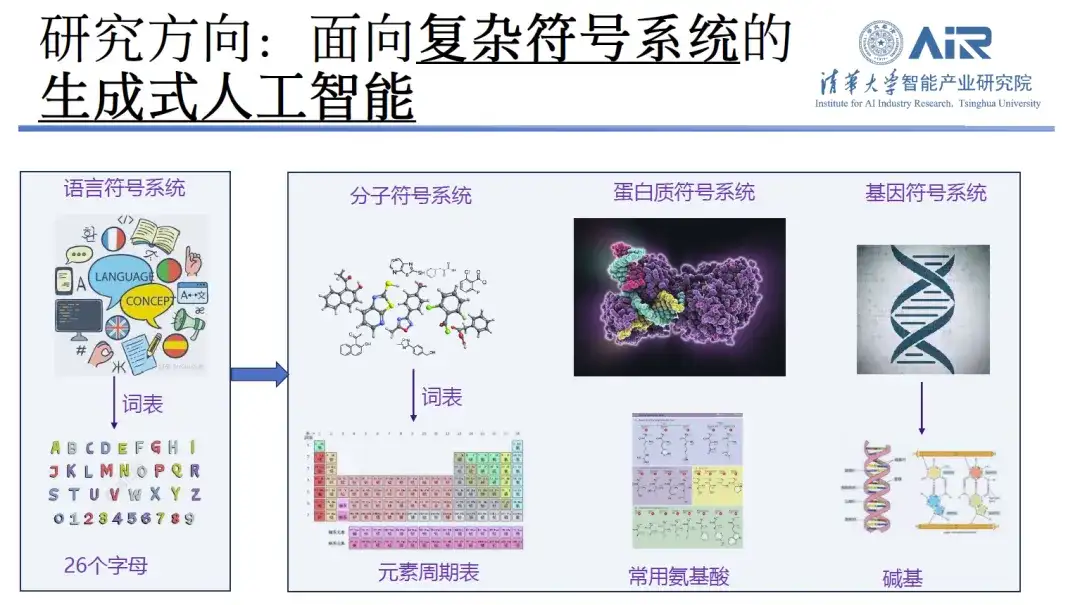
When introducing his research direction, Professor Zhou Hao said that he has been committed to natural language processing including text generation and machine translation in the past 10 years.Its research focus gradually shifted from content creation to molecule generation and protein design.In his view, if the text processing work in the past is regarded as a complex language symbol system, in which the vocabulary consists of 26 letters, then the current work is equivalent to expanding these 26 letters to a wider range of fields such as the periodic table, amino acids, bases, etc. His research team has accumulated rich experience in these technologies.
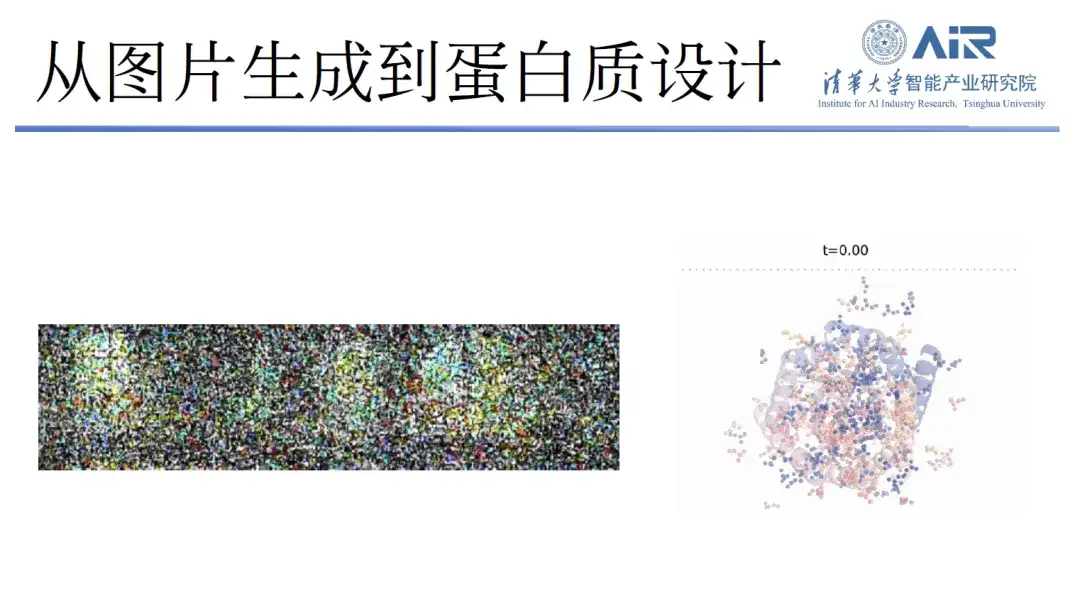
From AI focused on content creation to AI dedicated to scientific discovery,What is the connection between the two? In fact, artificial intelligence can generate complete images from noise, and many North American research teams have already used similar methods to design proteins. By randomly arranging the amino acids of proteins in space, and then through a series of generative designs from 0 to 2,000 steps, it is possible to design an amino acid sequence that looks quite reasonable.
Although there are still certain limitations on the length of proteins involved in this research, recent research results have significantly expanded these limitations and also implied the huge potential of this technology, which may be an important reason why Professor Zhou Hao chose this field.
Multiple challenges faced by AI practitioners when conducting scientific research
Afterwards, Professor Zhou Hao shared with everyone the three major challenges facing artificial intelligence (AI for Science) in the scientific field from the perspective of practitioners in the field of computer science or AI.
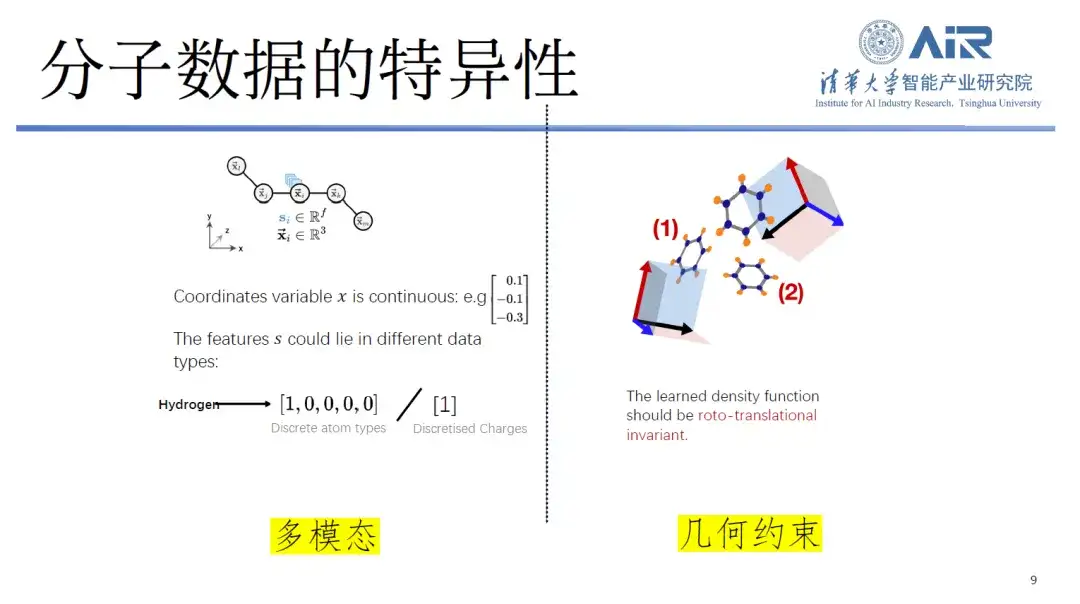
First, the specificity of molecular data.Generally speaking, text and symbols are processed as discrete, and images are continuous signals between 0 and 1, but molecular data contains both discrete and continuous elements.
For example, when storing molecules in computers, researchers usually represent them as atomic coordinates and atomic types, where atomic coordinates are continuous and atomic types are discrete, forming a multimodal data that is difficult to process. In addition, molecules also have geometric constraints, such as invariance to rotation and translation, which are not common in text or image processing.
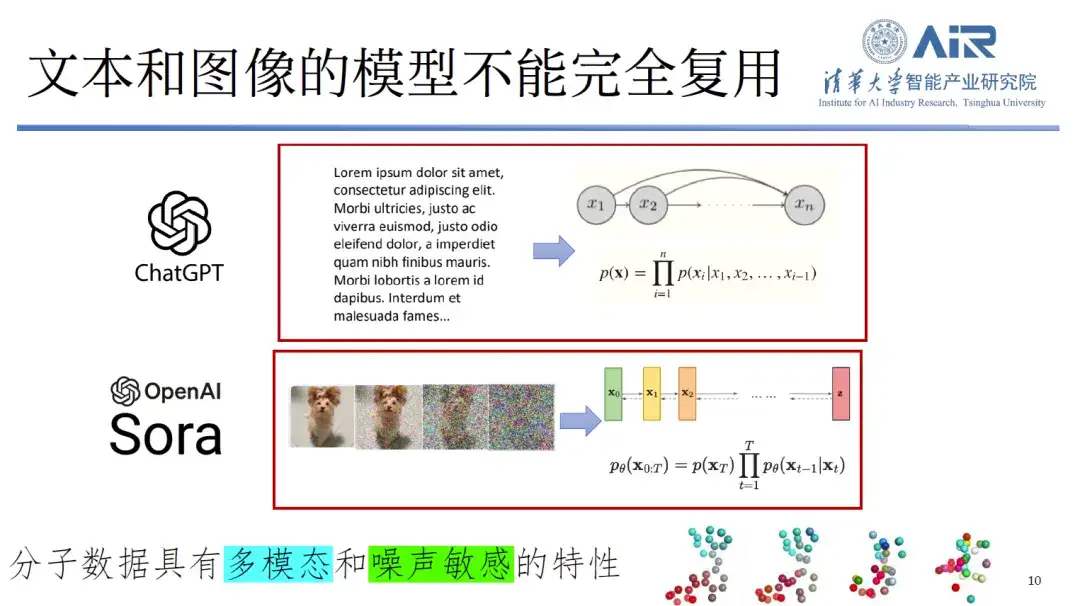
Second, text and image models cannot be fully reused in the protein field.Molecular data not only has multimodal characteristics, but is also extremely sensitive to noise. For example, if noise is added to a picture of a dog, people can still recognize that it is a picture of a dog. However, if even a tiny amount of noise is added to molecular data, it may cause people to be unable to recognize the identity of the molecule, resulting in a large amount of information loss. Therefore, traditional processing methods are not fully applicable to this new data type.
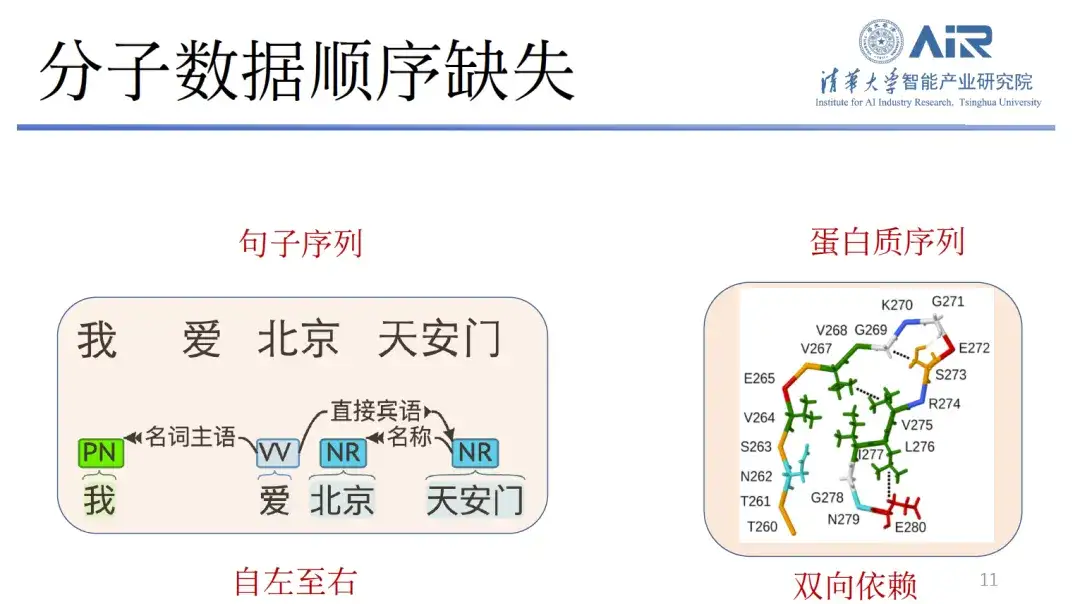
Third, the molecular data are missing in sequence.Text has very little reliance on left-to-right reading, so it can generate new text from left to right through GPT. However, proteins have very strong bidirectional dependencies, and their front-to-back, left-to-right, and right-to-left order is not easy to determine. If we directly use text or image models to generate molecular structures, we will face great difficulties.
In order to meet the above challenges,Professor Zhou Hao's team conducted in-depth research in data structure, generation algorithm and base construction.
Starting from the data structure, find the intrinsic data characterization space
Only retain the dihedral angle degrees of freedom to reconstruct the 3D structure representation of the molecule
“How to determine the eigenspace of a molecule or target data structure is a problem that computer scientists must solve.”Professor Zhou Hao said that the three-dimensional structure representation of molecules is very important, and it can be said that structure is function. In the past, researchers mainly built molecular models by recording the coordinates and types of atoms to obtain the required information. However, the structure of molecules is large and contains a lot of redundant information. If we use the past method to model, from the perspective of computer science, this is not an observation in the intrinsic space of the molecule.
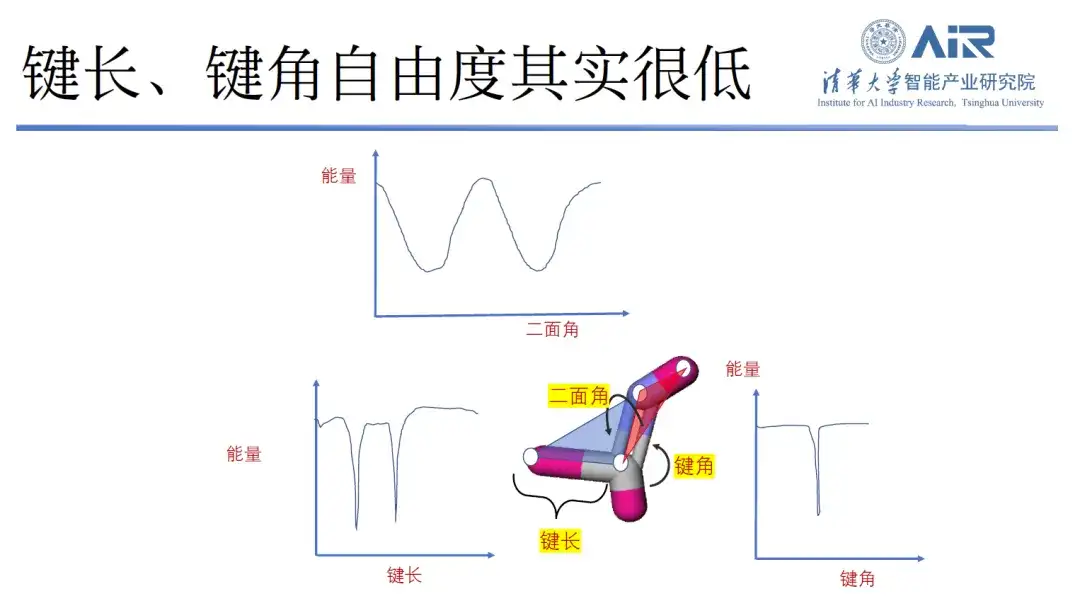
In fact, by analyzing the bond length, bond angle and dihedral angle of molecules, it is found that the peak values of molecular bond length and bond angle are small and the degrees of freedom are limited, while the dihedral angle has more degrees of freedom. Therefore, Professor Zhou Hao's team designed a new method.That is, while retaining the dihedral angle degree of freedom, other redundant degrees of freedom are removed.
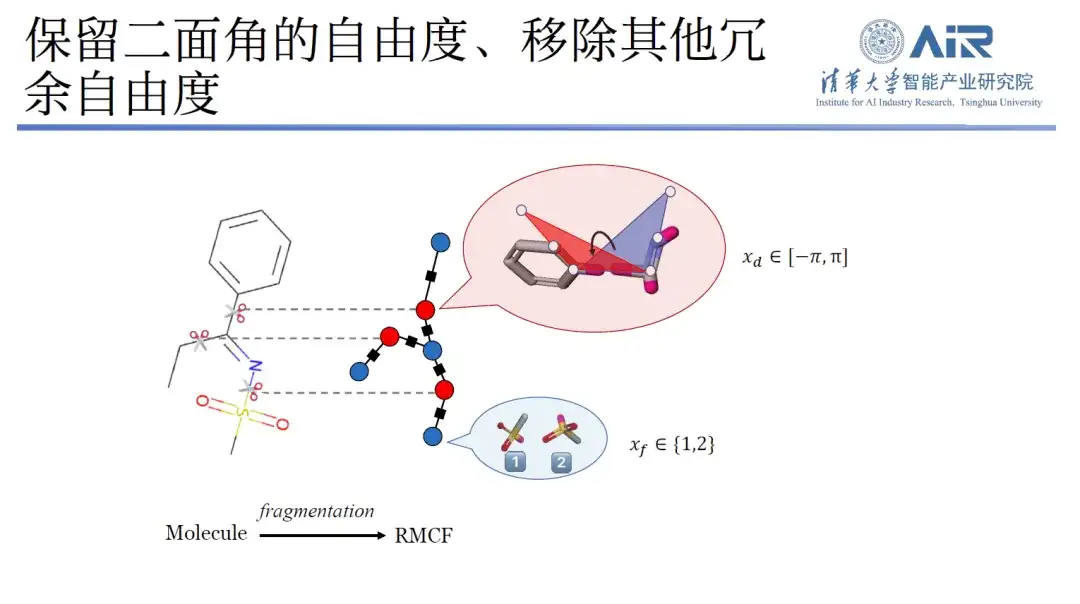
Specifically, the research can convert the three-dimensional structure into a two-dimensional representation, and through molecular fragmentation processing, minimize the degrees of freedom within each molecule and maximize the degrees of freedom between fragments. Using dynamic programming technology, the min-max problem can be easily solved, and then all molecules can be cut into the target data structure using an algorithm.
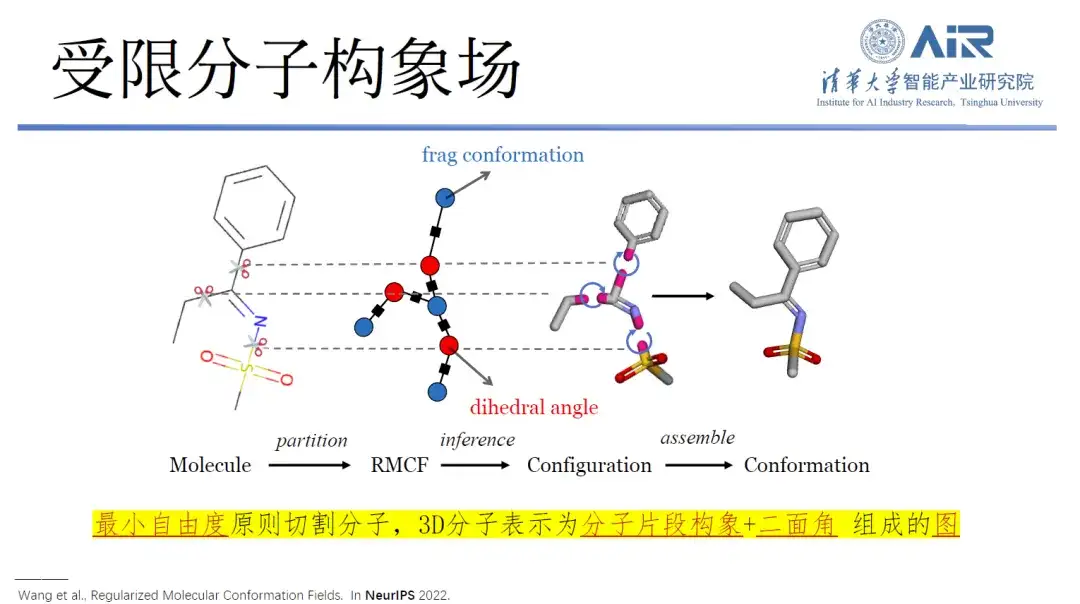
Paper title:Regularized Molecular Conformation Fields
Paper link:https://neurips.cc/virtual/2022/poster/53277
"With this new data structure, if molecules need to be generated in the future, related research will be able to construct the molecular space with very little data. This idea is extremely important!"
From real space to spectral space, efficiently capture protein geometry and chemical information
In addition to molecular research, Professor Zhou Hao's team is also interested in the study of protein structure and function.
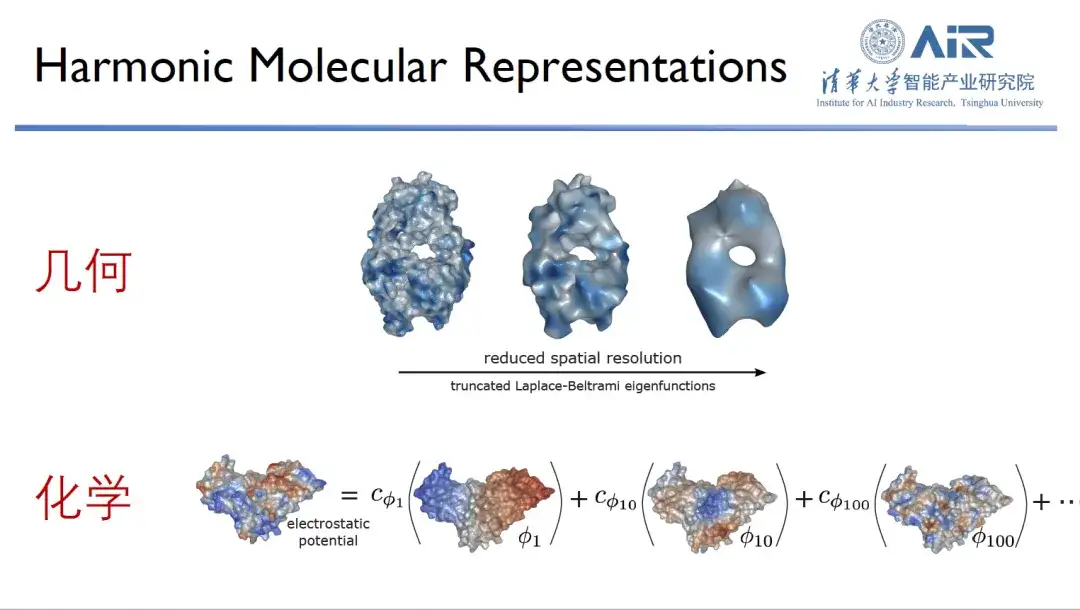
When studying proteins, researchers usually observe them from two dimensions: geometric information and chemical information. It is well known that the shape and surface chemical information of proteins are crucial to their function, and only when the two complement each other can they perform optimally.
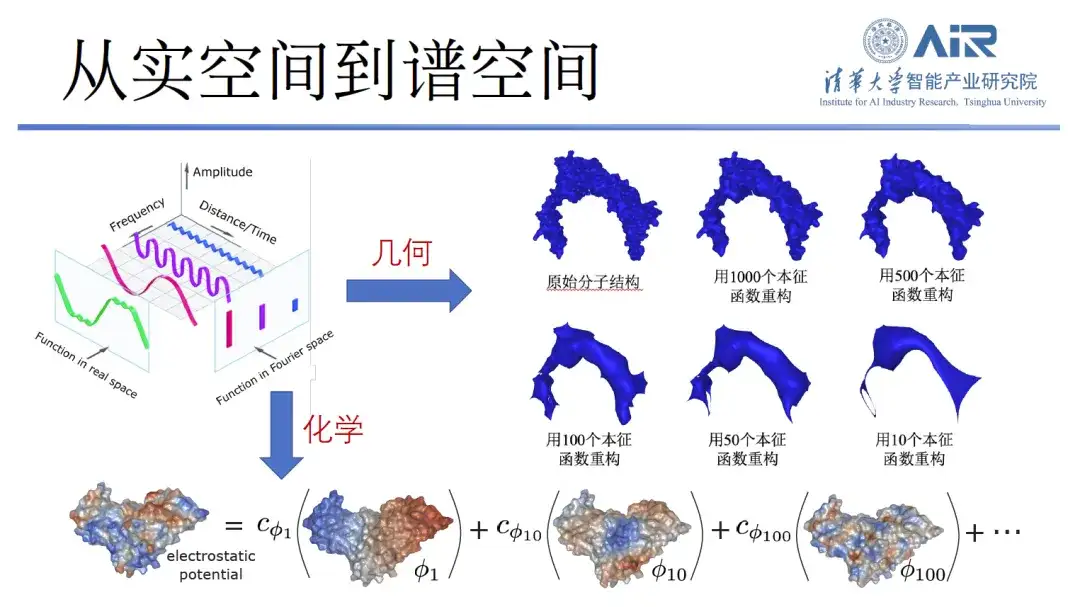
In order to efficiently represent the chemical and geometric information of proteins,Professor Zhou Hao's team transforms proteins from real space to spectral space, and then uses eigenfunctions to represent proteins. For example, 10 eigenfunctions are used to capture the low-frequency information of proteins, thereby analyzing their general outline. In addition, the more eigenfunctions there are, the more high-frequency information can be captured. By using 1,000 eigenfunctions, almost all protein information can be captured.
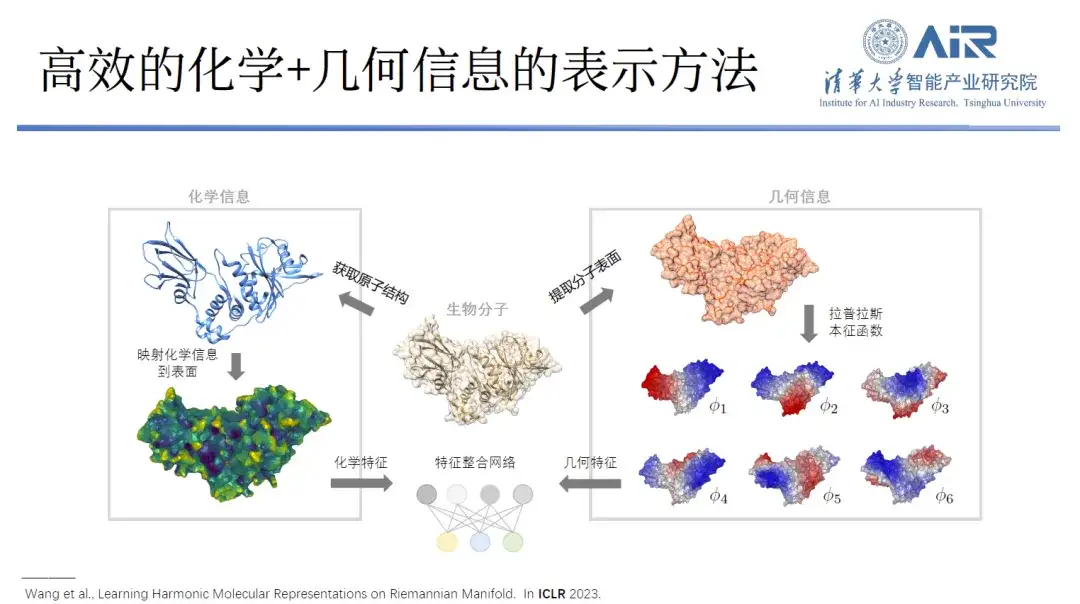
Paper title:Learning Harmonic Molecular Representations on Riemannian Manifold
Paper link:https://iclr.cc/virtual/2023/poster/10900
"The advantage of this approach is that it can replicate not only the geometric information of the protein, but also its chemical information."Each eigenfunction can be regarded as a new space, and the chemical information on the protein surface can be mapped to this eigenspace. Both geometric information and chemical information can be expressed in the same space, and the complex real space problem is converted into a simple spectral space problem.
Designing a generative model for aptamers based on a generative algorithm
Although the most compact and intrinsic molecular and protein spaces have been found, the next question after successfully identifying these spaces is:How to use generative artificial intelligence to effectively obtain target molecules.
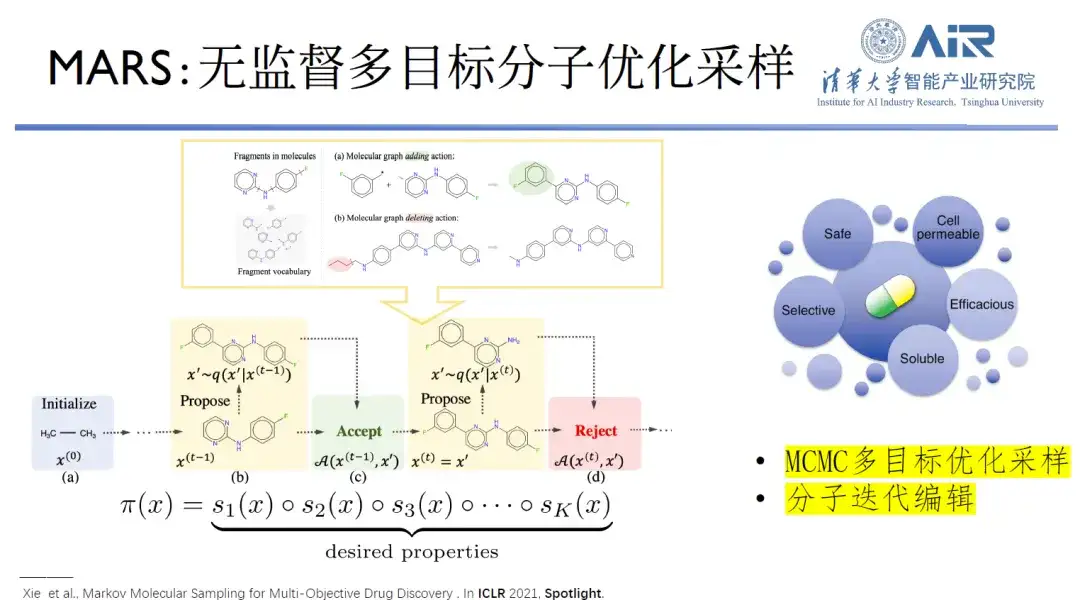
Paper title:MARS: Markov Molecular Sampling for Multi-objective Drug Discovery
Paper link:https://iclr.cc/virtual/2021/poster/3352
In order to find the most suitable molecular generation model,Professor Zhou Hao's team developed a model called MARS, which uses unsupervised multi-objective molecular optimization sampling to perform 2D molecular design. The molecular design process needs to meet multiple design goals, which is a problem of sampling in a complex high-dimensional space. The Markov Chain Monte Carlo (MCMC) framework is used to edit molecules. If the detailed balance conditions are met, any target molecule can be generated.
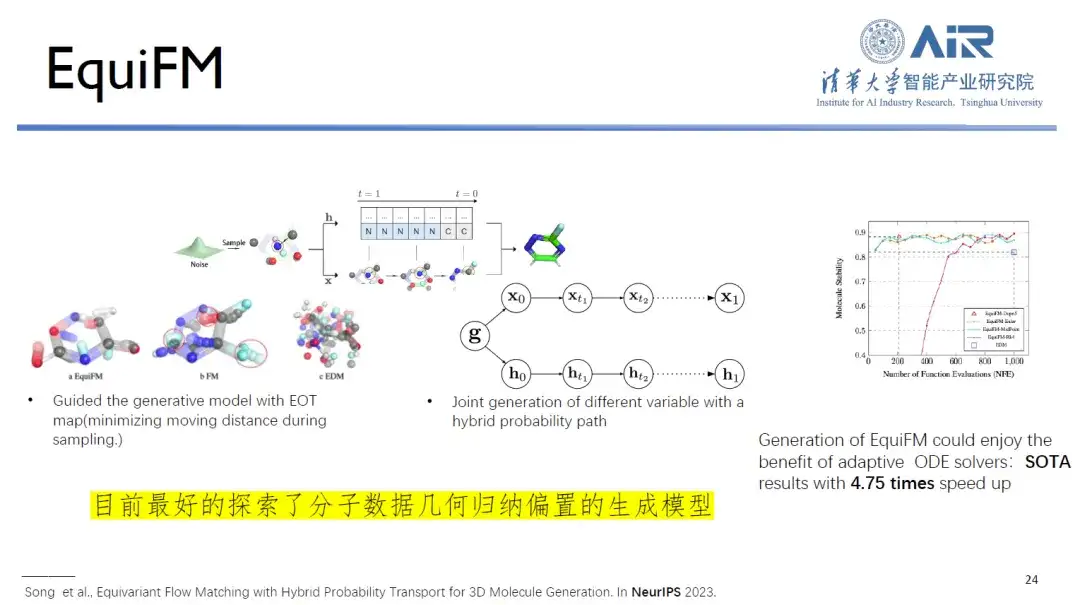
Paper title:Equivariant Flow Matching with Hybrid Probability Transport
Paper link:https://neurips.cc/virtual/2023/poster/70795
At the same time, EquiFM proposed by Professor Zhou Hao's team is currently the best generative model in exploring the geometric inductive bias of molecular data. It can achieve good performance in multiple molecular generation benchmarks and the average sampling speed is increased by 4.75 times.
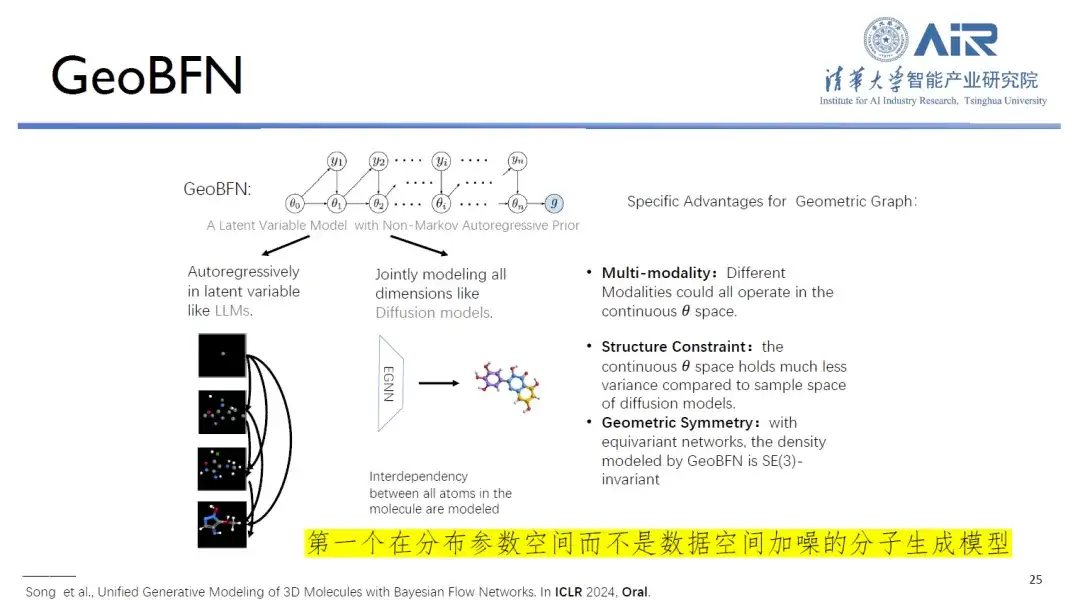
Paper title:Unified Generative Modeling of 3D Molecules via Bayesian Flow Networks
Paper link:https://iclr.cc/virtual/2024/oral/19764
In addition, the core of the GeoBFN molecular generation model is to transform all molecular data in the data space into the Gaussian mean variance space, thereby generating molecules with high legitimacy and close to the real distribution. In this regard, Professor Zhou Hao said:“This is currently the most suitable deep generative model for molecules and has great potential for development.”
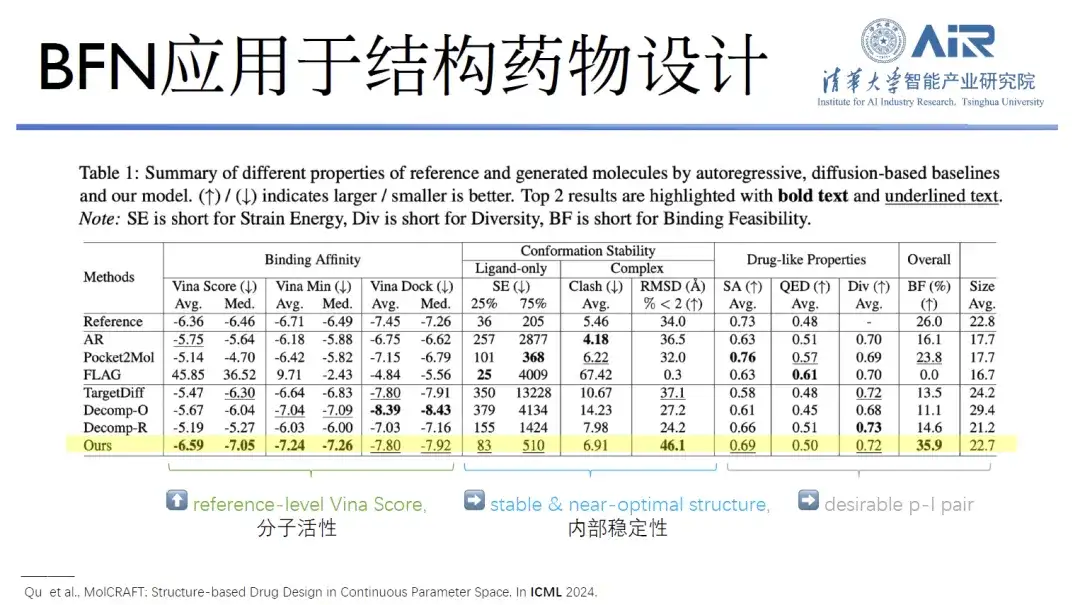
Paper title:MolCRAFT: Structure-Based Drug Design in Continuous Parameter Space
Paper link:https://icml.cc/virtual/2024/poster/34336
In addition to these works, Professor Zhou Hao's team also published a paper at the International Conference on Machine Learning (ICML) to explore the possibility of applying GeoBFN to structural drug design. The results showed that the molecules generated using this model have very stable conformations and good activity.
Starting from the foundation construction, establish a pre-training foundation rich in vast data knowledge
Finally, Professor Zhou Hao shared with everyone how to build a pre-training base rich in vast data knowledge starting from the base construction.
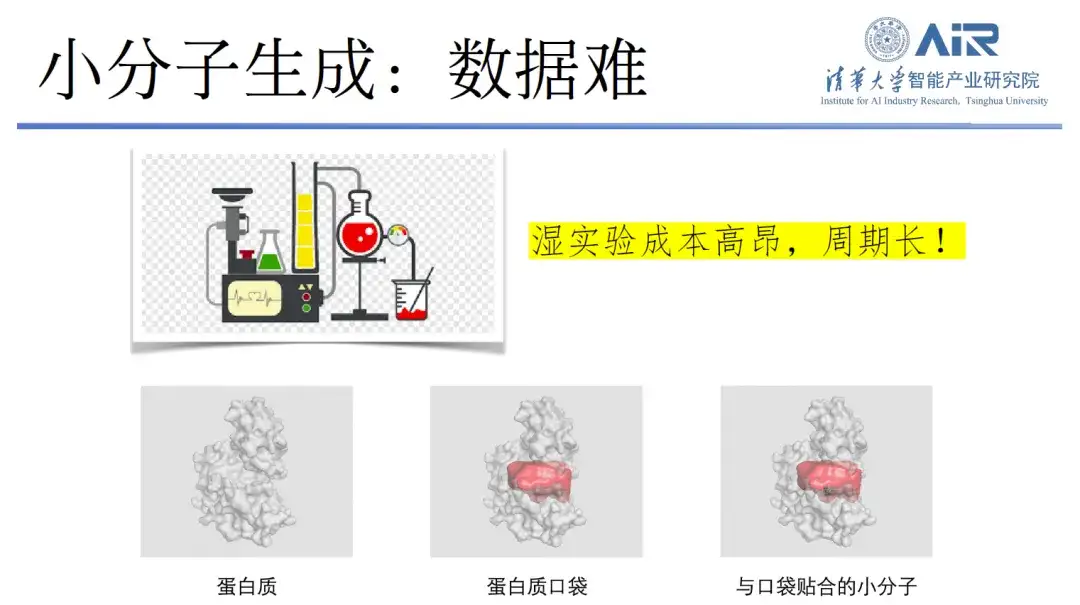
In existing research, experimental data on small molecule generation is very scarce, and trying to use computer science methods to solve this problem is an important idea.
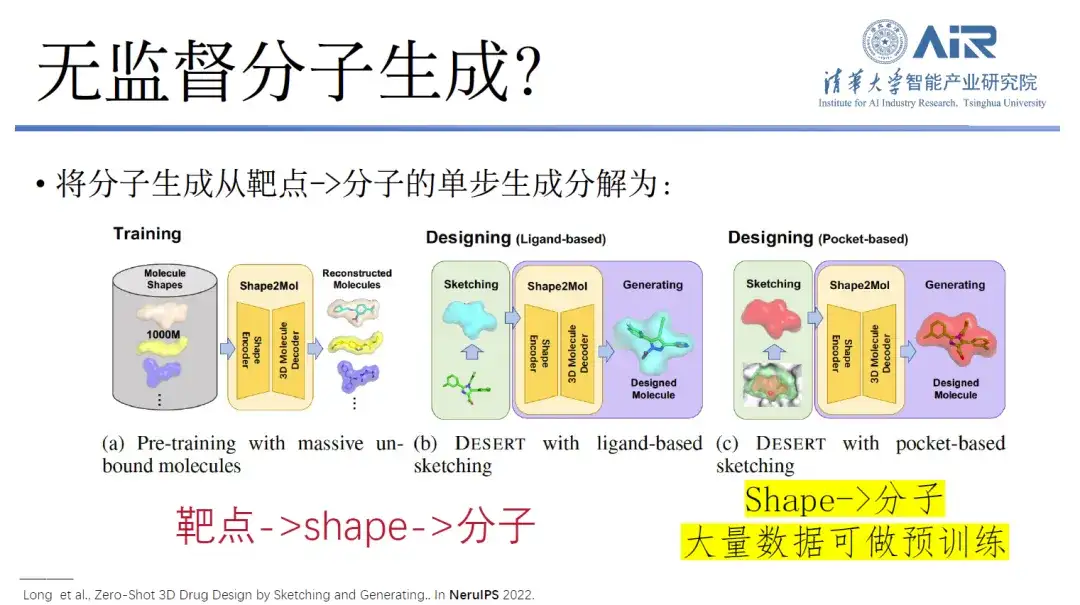
Paper title:Zero-Shot 3D Drug Design by Sketching and Generating
Paper link:https://neurips.cc/virtual/2022/poster/54457
In this regard, Professor Zhou Hao's team proposed a new idea.That is, the single-step generation of molecules from target to molecule is decomposed into a process from target to shape, and then from shape to molecule.In fact, although the amount of data from target to molecule is small, the amount of data from shape to molecule is very large. This data is enough to collect various shapes from target, and then make a large-scale pre-training model from shape to molecule. Finally, it is possible to quickly achieve the process from target to molecule, and even realize unsupervised or less-supervised drug molecule design.
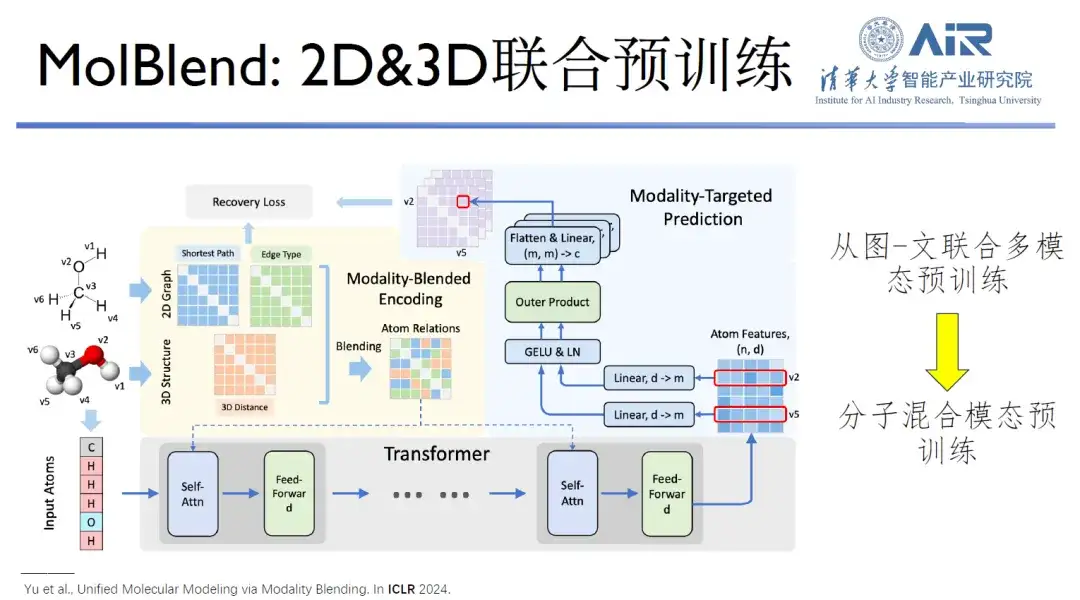
Paper title:Multimodal Molecular Pretraining via Modality Blending
Paper link:https://iclr.cc/virtual/2024/poster/17824
In addition, the MolBlend model they proposed realizes the joint pre-training of two-dimensional and three-dimensional molecules, which is a typical case of expansion from image and text pre-training to molecular pre-training.
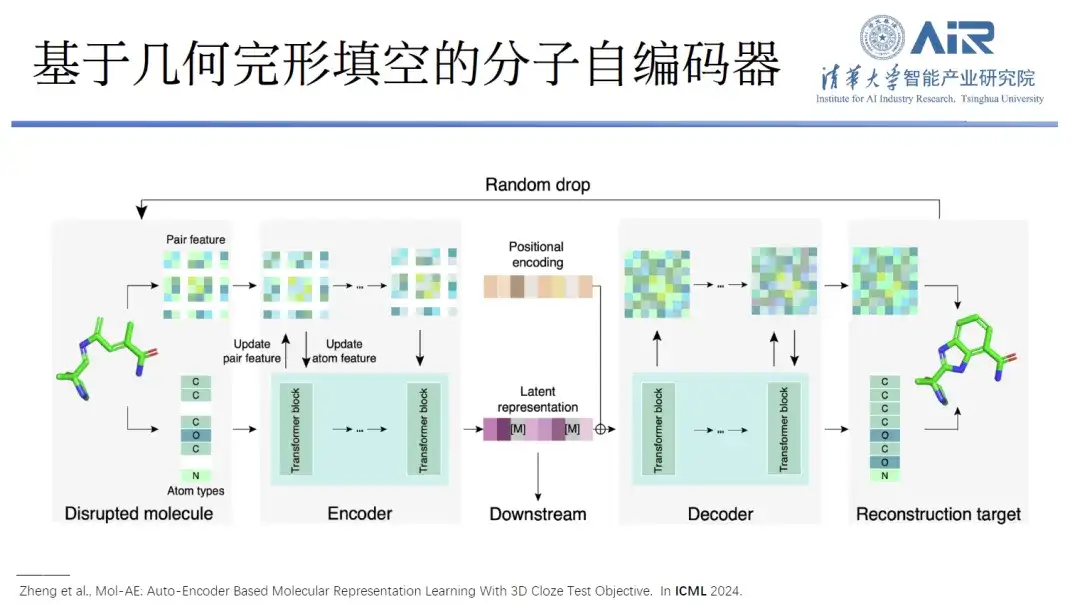
Paper title:Mol-AE: Auto-Encoder Based Molecular Representation Learning With 3D Cloze Test Objective
Paper link:https://icml.cc/virtual/2024/poster/33340
in addition,They also proposed a molecular autoencoder Mol-AE based on geometric cloze.With the new training objectives of the 3D Cloze Test, the proposed model can better learn the spatial relationships of atoms in real molecular structures. Compared with the most advanced 3D molecular modeling methods, Mol-AE achieves a significant performance improvement.
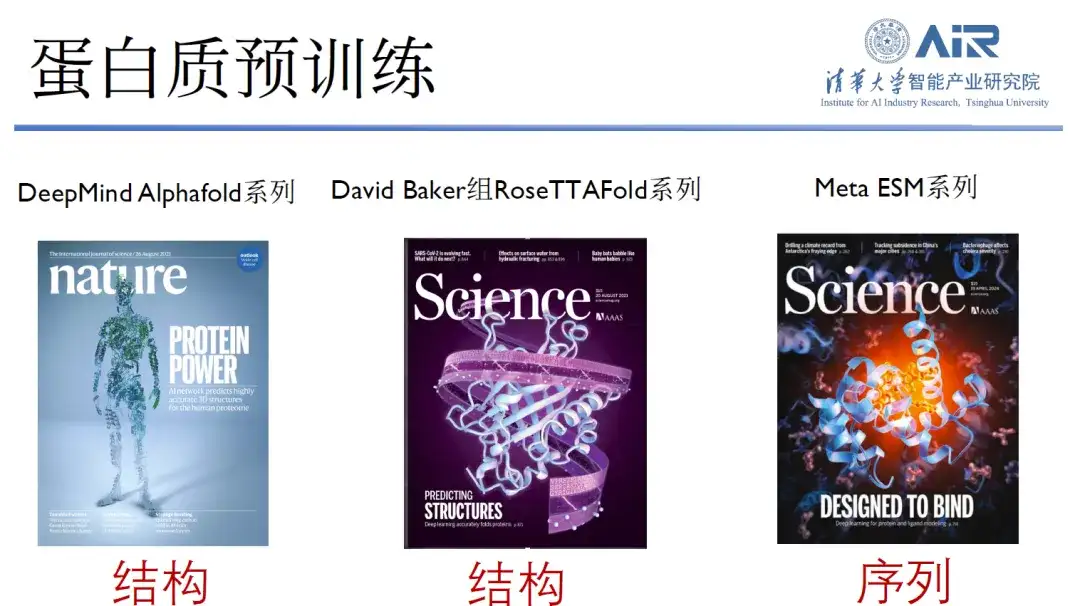
The general pre-training research of proteins is also a direction they have chosen. It is understood that the general pre-training of proteins is mainly divided into three categories: DeepMind Alphafold series, David Baker's RoseTTAFold series, and Meta ESM series.Professor Zhou Hao’s team has currently developed the ESM-AA model.
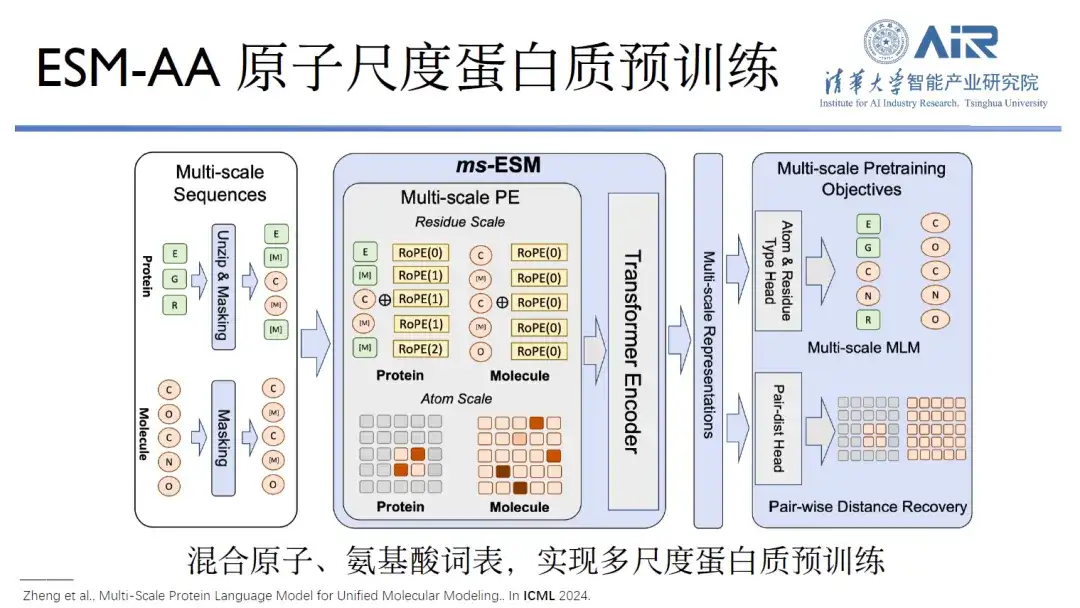
Paper title:Multi-Scale Protein Language Model for Unified Molecular Modeling
Paper link:https://icml.cc/virtual/2024/poster/35119
This is because the upgrade from Alphafold2 to Alphafold3 has built an all-atom base, as has the RoseTTAFold series. Only the ESM series has not yet built an all-atom base. Professor Zhou Hao's team has been working on this since September last year, combining the atomic and amino acid vocabularies to achieve multi-scale protein training. In the joint task of protein and small molecules, ESM-AA outperforms individual pre-trained bases, such as ESM, other protein pre-training or small molecule pre-training bases.
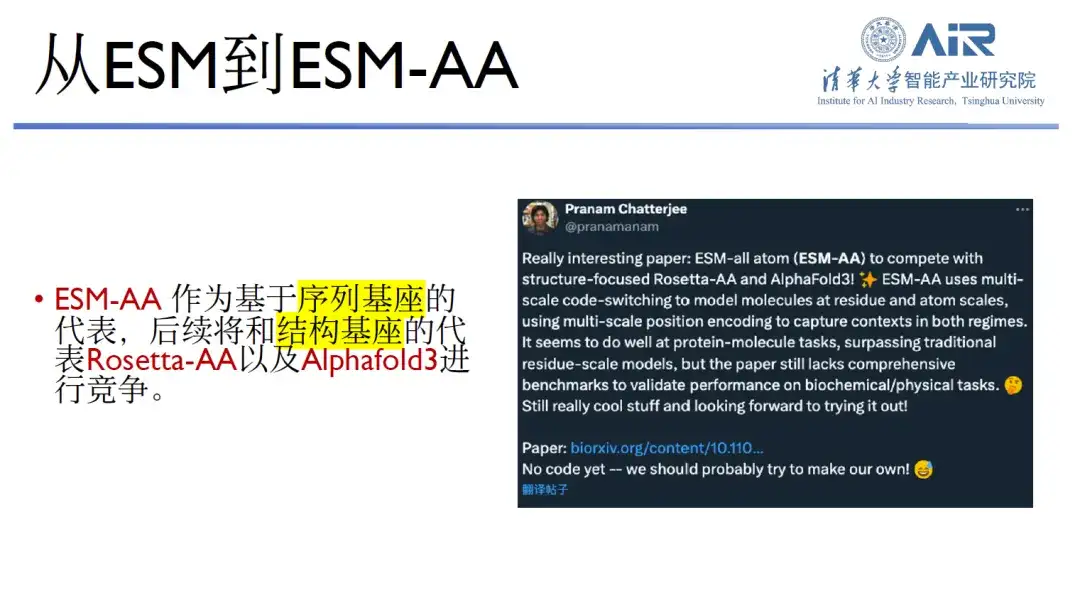
This pre-trained base has also received widespread praise on Twitter. As a representative of sequence bases, ESM-AA will compete with RoseTTAFold and Alphafold3, representatives of structural bases. "I think this is also our future goal," said Professor Zhou Hao.
About Professor Zhou Hao
Zhou Hao, born in 1990, PhD, associate researcher at Tsinghua University. His research direction is generative artificial intelligence for complex symbolic systems, with major applications including ultra-large-scale language models, molecular generation, protein design, and new material discovery.
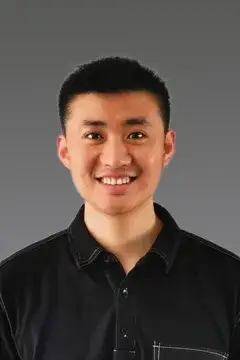
He was a research scientist and deputy director at ByteDance. He led the R&D teams in the text generation platform and AI-assisted drug design of ByteDance. The R&D products are used in more than 20 countries around the world, with more than 1 billion users. He has long served as the field chair of top AI conferences such as ICML, NeurIPS, ICLR, and ACL, and has published more than 80 papers at important international AI conferences. He won the 2019 Outstanding Doctoral Dissertation Award of the Chinese Artificial Intelligence Society, the Best Paper Award (1/3350) of the top international conference in the field of natural language processing, ACL 2021, and the 2021 NLPCC Young Emerging Scholar Award of the Chinese Computer Society.