CVPR Best Student Paper! A Large Dataset of 10 Million Images and 450,000+ Species, the Multimodal Model BioCLIP Achieves Zero-shot Learning
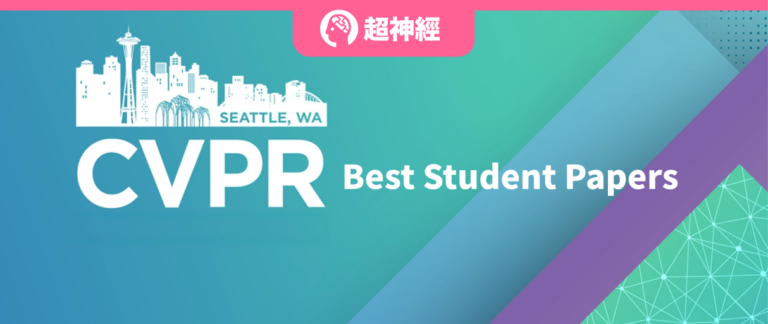
Different from the traditional academic field that attaches great importance to journal publication, in the computer world, especially in the fields of machine learning, computer vision, artificial intelligence, etc., top conferences are king. Countless "hot research directions" and "innovative methods" will flow out from here.
As one of the three most academically influential conferences in the field of computer vision and even artificial intelligence, this year's International Conference on Computer Vision and Pattern Recognition (CVPR) broke previous records in terms of conference scale and the number of accepted papers.
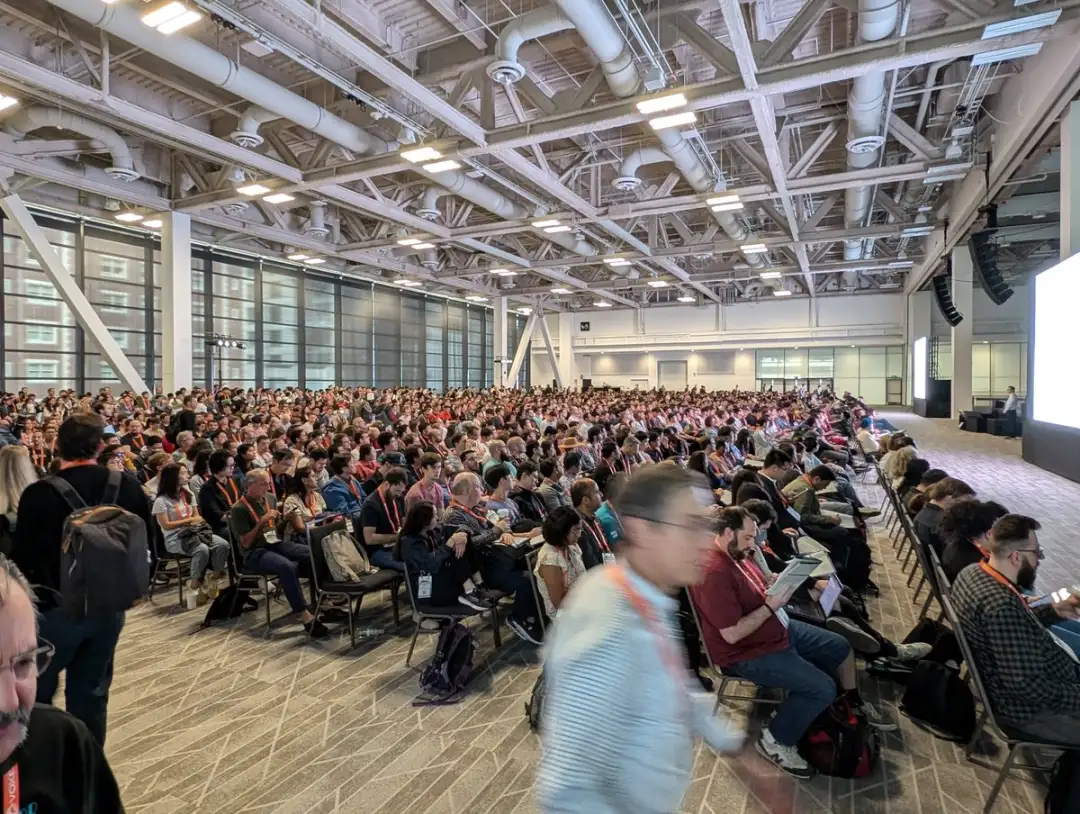
According to the latest official announcement of CVPR,CVPR 2024 has become the largest and most attended conference in the history of the conference.As of June 19, the number of on-site participants has exceeded 12,000.
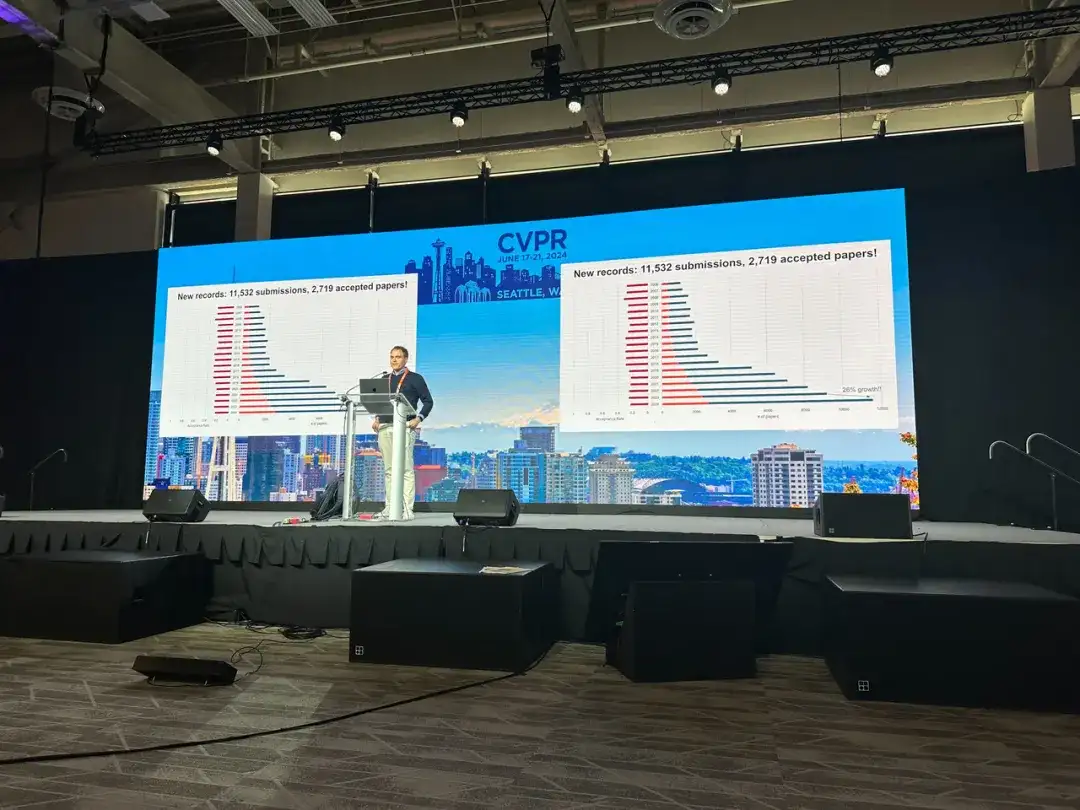
In addition, as a leading computer vision event, CVPR accepts the latest research in the current visual field every year. Among the 11,532 valid papers submitted this year, 2,719 papers were accepted, compared with CVPR 2023.The number of received papers increased by 20.6%, while the acceptance rate decreased by 2.2%.These data show that the popularity, competition and quality of winning papers of CVPR 2024 have increased.
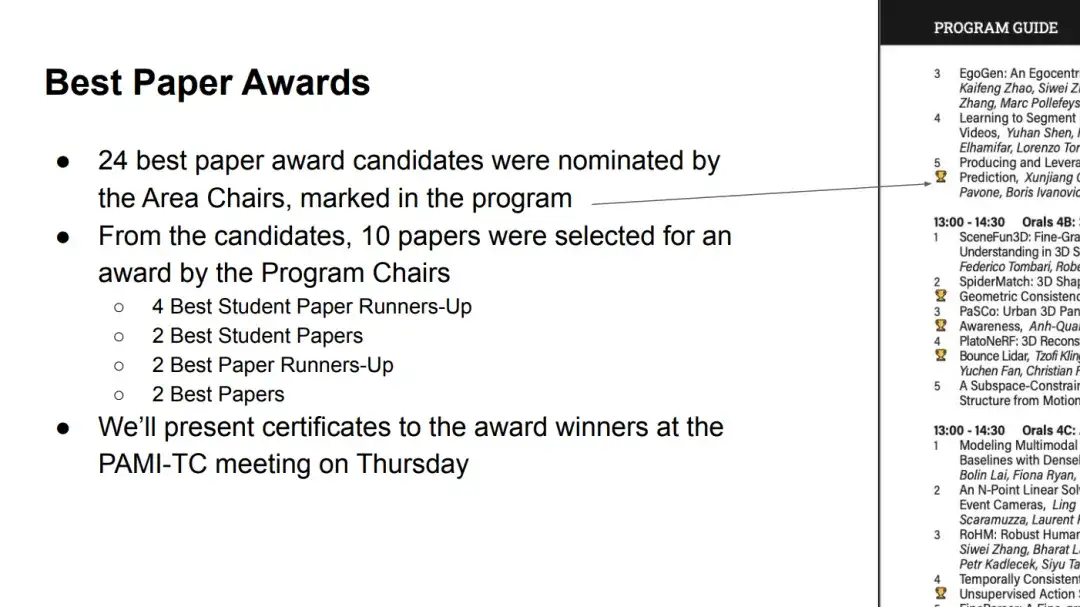
At midnight on June 20th, Beijing time, CVPR 2024 officially announced the best paper awards for this session. According to statistics, a total of 10 papers won awards.Among them, there are 2 best papers, 2 best student papers, 2 best paper nominations and 4 best student paper nominations.
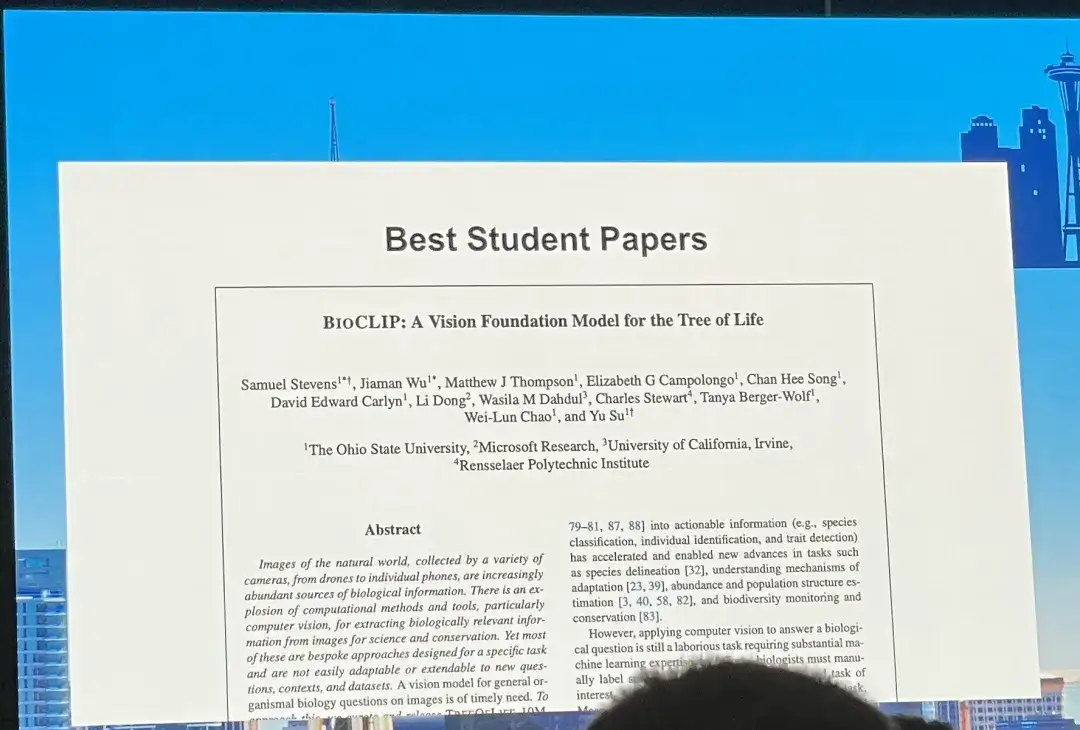
in,“BIoCLIP: A Vision Foundation Model for the Tree of Life” was named the best student paper.In this regard, Sara Beery, assistant professor at MIT's Computer Science and Artificial Intelligence Laboratory, commented that the authors and team were "well-deserved" winners, and the first author of the paper, Samuel Stevens, was the first to express his gratitude on the platform.
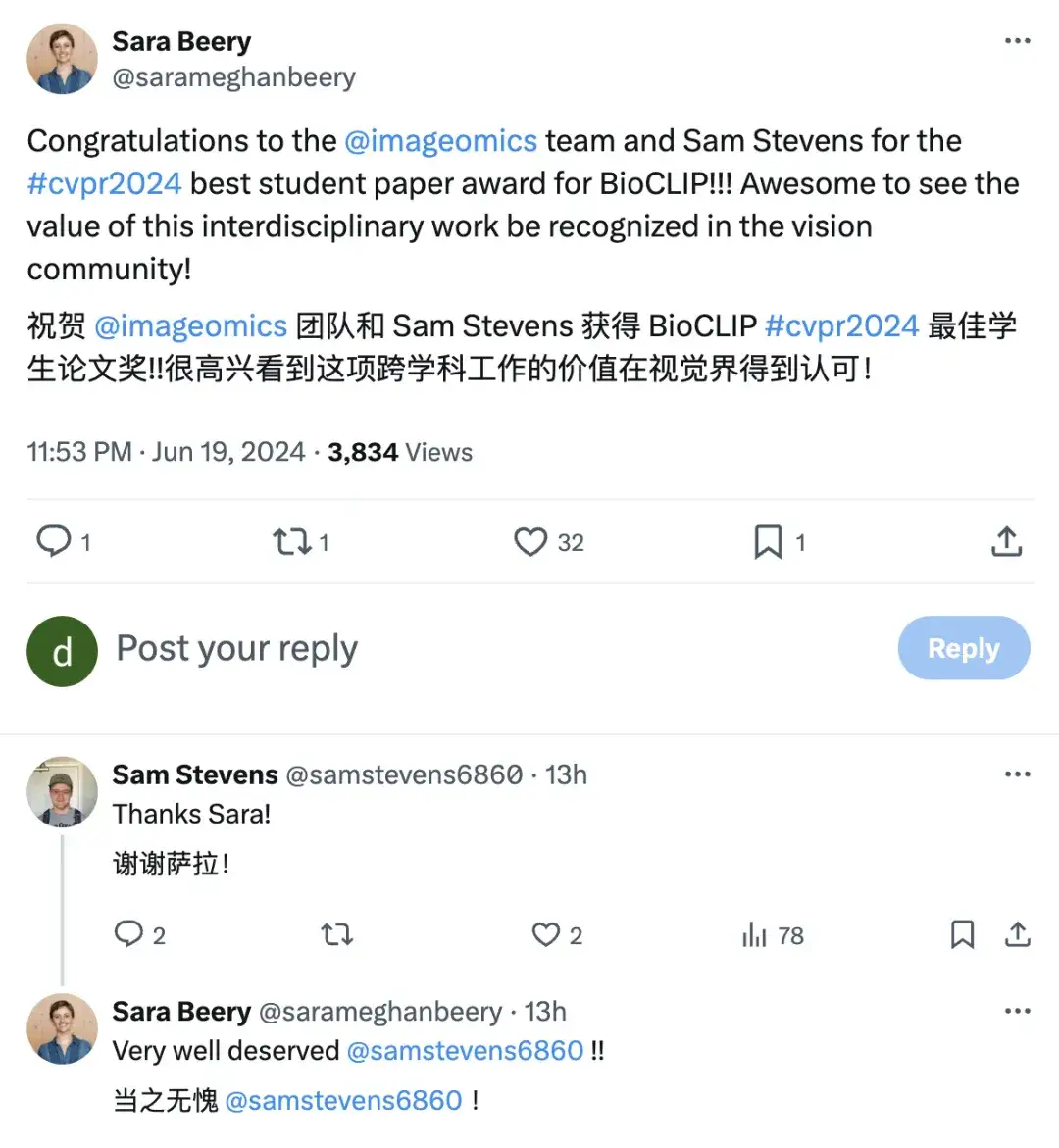
HyperAI will comprehensively interpret "BIoCLIP: A Vision Foundation Model for the Tree of Life" from the aspects of data set, model architecture, model performance, etc., and summarize other achievements of Sam Stevens for everyone.
Download address:
https://arxiv.org/pdf/2311.18803
Creating the largest, most diverse biological image dataset
The largest biological image dataset for machine learning is iNat21, which contains 2.7 million images and covers 10,000 species. Although the classification breadth of iNat21 has been greatly improved compared with general-purpose datasets such as ImageNet-1k, 10,000 species are still rare in biology. The International Union for Conservation of Nature (IUCN) reported more than 2 million known species in 2022, with more than 10,000 species of birds and reptiles alone.
To address the problem of species category restrictions in biological image datasets,The researchers built a dataset called TreeOfLife-10M containing 10 million images.Spanning 450K+ species, it has achieved a revolutionary breakthrough in dataset size and species diversity.
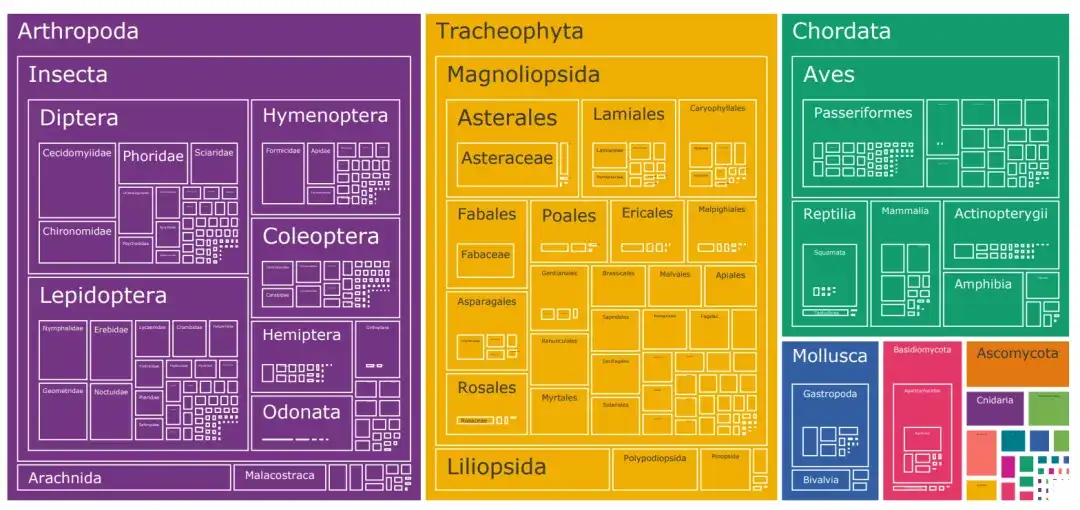
The dataset combines biological images from iNaturalist, BIOSCAN-1M, and the Encyclopedia of Life (EOL).
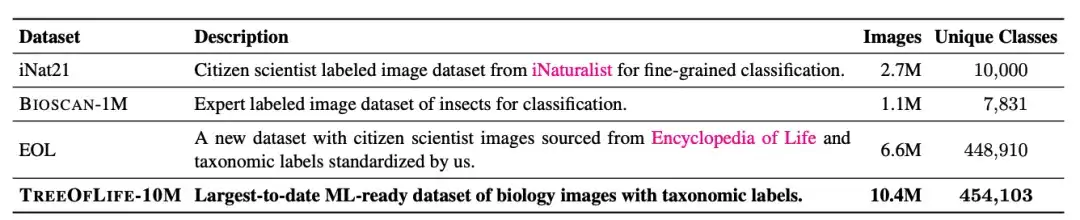
In addition to the 10,000 species categories covered by iNat21, the researchers downloaded 6.6 million images from EOL to expand TreeOfLife-10M to cover an additional 440,000 taxa. At the same time, to help the base model learn extremely fine-grained visual representations of insects, the researchers also included BIOSCAN-1M, a state-of-the-art dataset containing 1 million laboratory insect images, covering 494 different families and 7,831 species classifications.
TreeOfLife-10M Download address:
https://go.hyper.ai/Gliol
Multimodal model BioCLIP: Improving generalization ability based on CLIP
Compared with general tasks, the label space of biological computer vision is richer. Not only is the number of classification annotations huge, but the annotations are also interconnected in the hierarchical classification system. This undoubtedly brings huge challenges to training basic models with high species coverage and strong generalization capabilities.
Drawing on hundreds of years of experience in biological research, the researchers believe that if the underlying model can successfully encode the structure of the annotation space, then even if a particular species has not been seen, the model may be able to identify its corresponding genus or family and give a corresponding representation. This hierarchical representation will help achieve few-shot or even zero-shot learning of new taxa.
Based on this, the researchers chose CLIP, a multimodal model architecture developed by OpenAI.And use CLIP's multimodal contrastive learning objective to continuously pre-train on TREEOFLIFE-10M.
Specifically, CLIP trains two unimodal embedding models, the visual encoder and the text encoder, to maximize the feature similarity between positive pairs and minimize the feature similarity between negative pairs, where the positive pairs come from the training data and the negative pairs are all other possible pairs in the batch.
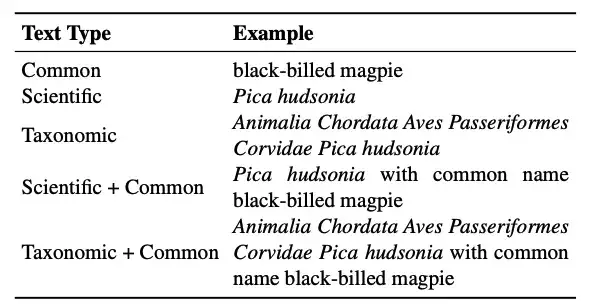
In addition, an important advantage of CLIP is that its text encoder accepts free-form text, which can cope with the diverse class name formats in the biological field. Regarding the text form in this study, the researchers mainly considered:
* Taxonomic name:The standard seven-level biological classification is Kingdom, Phylum, Class, Order, Family, Genus, and Species. For each species, the classification system is "flattened" by concatenating all the annotations from the root to the leaves into a string, which is the classification name.
* Scientific name:Consists of genus and species.
* Common name:Taxonomic names are usually Latin, which are not common in general image-text pre-training datasets. In contrast, common names such as "black-billed magpie" are more common. It should be noted that there may not be a one-to-one mapping between common names and taxonomic groups. A species may have multiple common names, or the same common name may refer to multiple species.
In practical applications, there may be only one type of annotation input. In order to increase flexibility during reasoning,The researchers proposed a mixed text type training strategy.That is, in each training step, each input image is paired with text randomly selected from all available text types. Experiments show that this training strategy not only maintains the generalization advantage of categorical names, but also provides more flexibility during reasoning.

As shown in Figure a above, the taxonomic groups or classification labels of two different plants, Onoclea sensibilis (d) and Onoclea hintonii (e), are exactly the same except for the species.
As shown in Figure 2b, the text encoder is an autoregressive language model that can naturally encode hierarchical representations of taxonomy, where the order representation Polypodiales can only depend on higher orders, absorbing information from Kingdom, Phylum and Class tokens. These hierarchical representations of taxonomic labels are input into the standard contrastive pre-training target and matched with image representations (d) and (e).
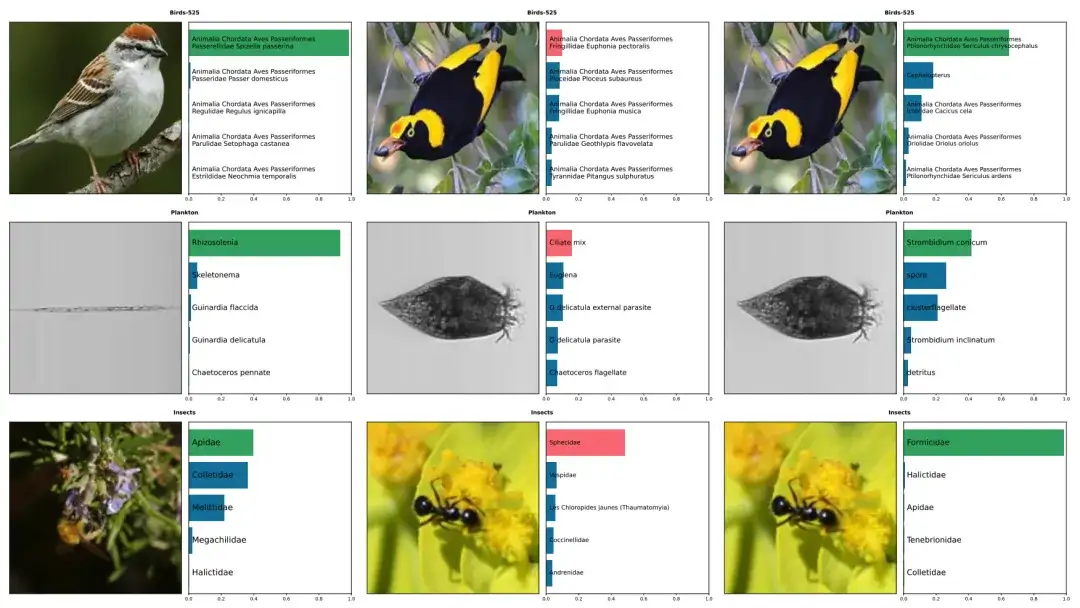
The above figure shows the prediction examples of five species, including birds, plankton, and insects, by BioCLIP and CLIP. The correct annotations are in green, and the incorrect annotations are in red. The left column shows the correct predictions by BioCLIP. The middle and right columns show images that were incorrectly annotated by CLIP but correctly annotated by BioCLIP.
BioCLIP performs well on zero-shot and few-shot tasks
The researchers compared BioCLIP with a general vision model.BioCLIP performs well in both zero-shot and few-shot tasks, and significantly outperforms CLIP and OpenCLIP,The average absolute improvement in zero-shot and few-shot tasks is 17% and 16% respectively. Intrinsic analysis further shows that BioCLIP learns a more fine-grained hierarchical representation that conforms to the tree of life, explaining its excellent generalization ability.
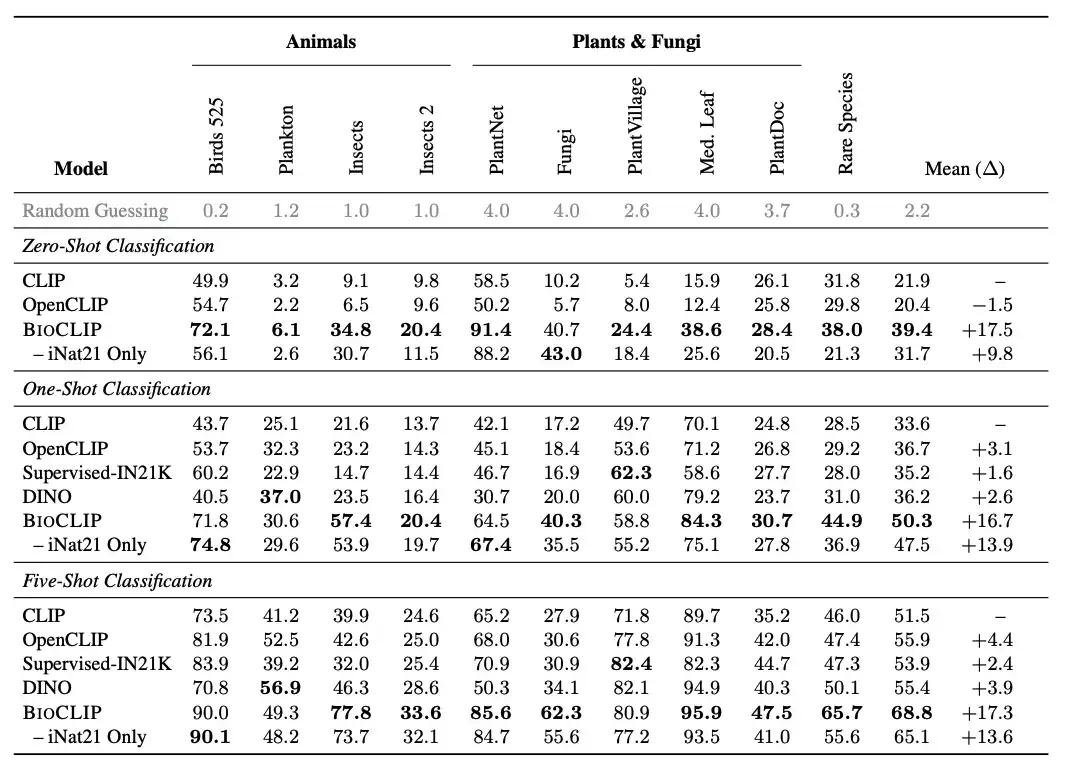
Specifically, the researchers introduced a new assessment task "RARE SPECIES", which collected about 25K species from the IUCN Red List, which are classified as Near Threatened, Vulnerable, Endangered, Critically Endangered, or Extinct in the Wild. The researchers selected 400 such species with at least 30 images in the EOL dataset and then removed them from TreeOfLife-10M.Created a new test set of rare species,There are 30 images for each species.
As shown in the figure above, BioCLIP significantly outperforms the baseline CLIP model and the CLIP model trained with iNat21 in zero-shot classification, especially in unseen classifications (see the Rare Species column).
Fruitful results, exploring the scientific research behind the best BioCLIP
"BioCLlP: A Vision Foundation Model for the Tree of Life" was jointly released by Ohio State University, Microsoft Research, University of California, Irvine, and Rensselaer Polytechnic Institute.The paper's first author, Dr. Samuel Stevens, and corresponding author, Jiaman Wu, are both from Ohio State University.
Although Samuel Stevens modestly describes himself on his personal website as "not a person who takes himself very seriously", judging from his fruitful scientific research results and unremitting efforts in recent years, he is obviously a person who takes scientific research seriously.
It is understood that Samuel Stevens has been engaged in computer work since 2017. The multimodal model BioCLlP is a research result he published in December 2023 and was accepted by CVPR 2024 in February 2024.
In fact, computer vision work like BioCLlP is just one of his research directions. He has a wide range of interests and has conducted a series of research in areas such as AI for crypto and various LLM projects.
For example, he participated in the "MMMU: A Massive Multi-discipline Multimodal Understanding and Reasoning Benchmark for Expert AGI".A new benchmark MMMU (Massive Multi-Task Language Understanding) is proposed.As one of the most influential large model evaluation benchmarks in the industry, MMMU focuses on leveraging knowledge in specific fields (science, health and medicine, humanities, etc.) for advanced perception and reasoning, requiring multimodal models to be able to perform tasks similar to those faced by experts.
The researchers used it to evaluate 14 open source LMMs and the proprietary GPT-4V (ision), and found that even the advanced GPT-4V only achieved an accuracy of 56%, which shows that there is still a lot of room for improvement in the model. In this regard, the researchers expressed the hope that the benchmark will inspire the community to build the next generation of multimodal base models to achieve expert-level artificial general intelligence.
MMMU: https://mmmu-benchmark.github.io
Of course, his passion and open attitude towards scientific research are also key factors in his success. Yesterday, the news that BioCLlP was named the best student paper came out, and Dr. Samuel Stevens immediately expressed his views to the outside world through social platforms: "If you want to talk about computer vision of animals, multimodal basic models or AI for Science, please send me a private message!"
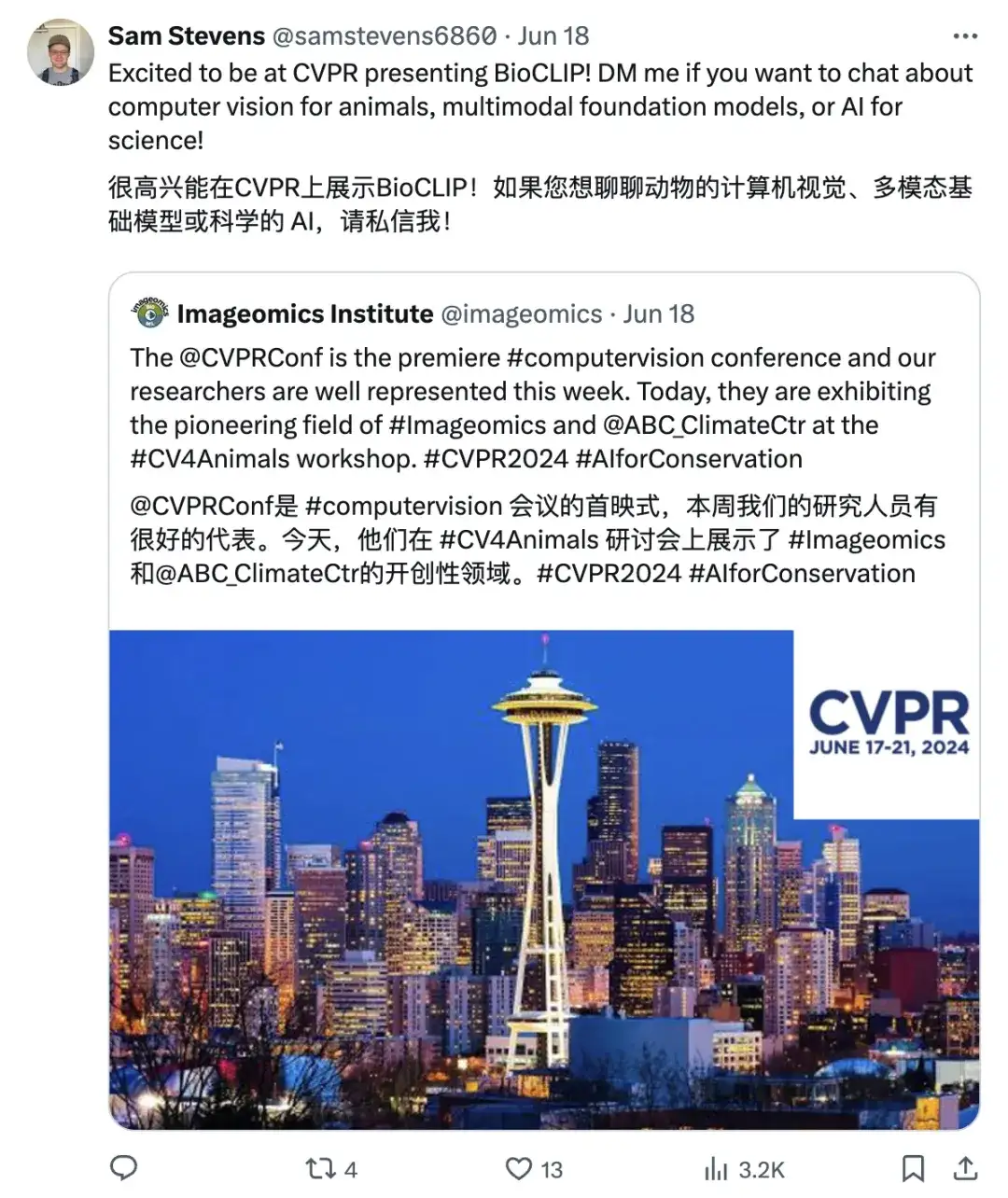
It is worth mentioning that Dr. Samuel Stevens not only forges ahead in scientific research, but also does not forget to support the younger generation. His personal website also shares advice for beginners: "If you want to get started with machine learning and artificial intelligence, you may want to start with Coursera's machine learning course and Andrej Karpathy's Neural Networks: Zero to Hero. Both courses are of very high quality and should provide a lot of value compared to other free resources."
References:
1. https://samuelstevens.me/#news
Finally, I recommend an activity!
Scan the QR code to sign up for the 5th offline gathering of the "Meet AI Compiler" Technology Salon↓
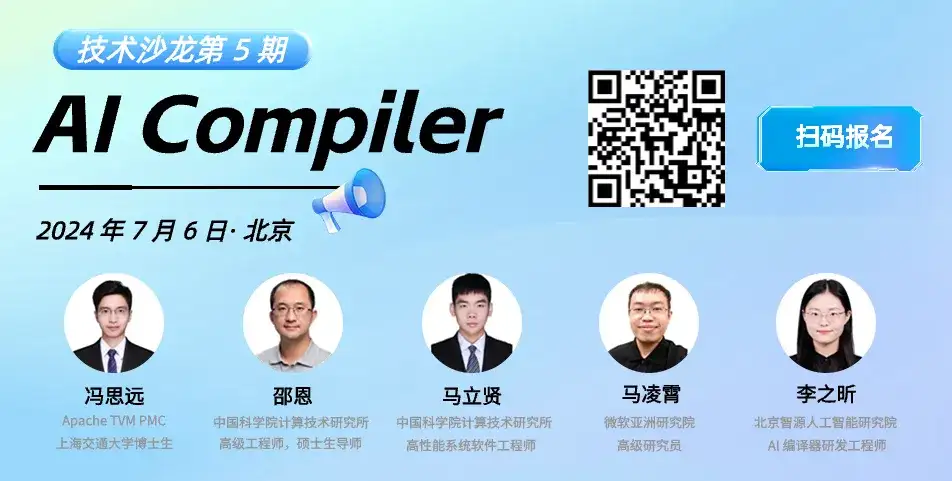