Solving the Problems of Data Silos, Computing Consumption, and Error Accumulation, Su Rui From Shanghai Artificial Intelligence Laboratory: FengWu-GHR Achieves Multiple Breakthroughs in AI Weather Forecasting
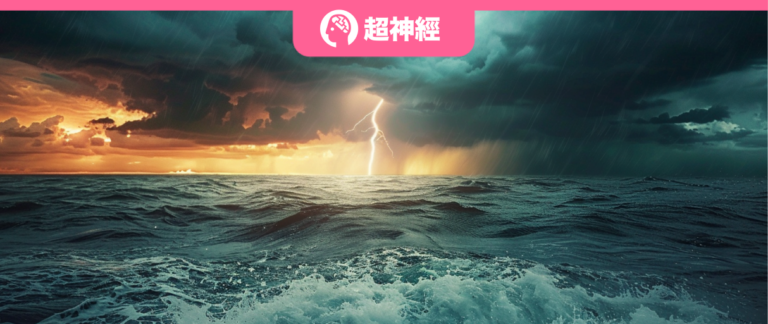
“Before the emergence of AI methods, it took 10 years to improve the weather forecasting skills by one day, but after the introduction of AI, the forecasting skills can be improved in a few months.”
At the "AI for Science" forum of the 2024 Beijing Zhiyuan Conference, Su Rui, a young researcher at the Shanghai Artificial Intelligence Laboratory, reviewed the historical development of AI weather forecasting, discussed in depth the challenges faced in this field, and comprehensively introduced his team's research results FengWu-GHR.
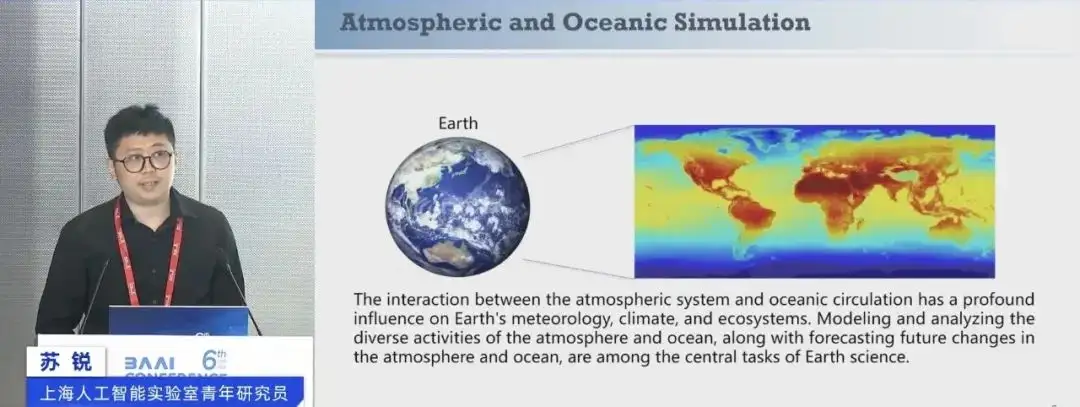
HyperAI has compiled and summarized Mr. Su Rui’s in-depth sharing without violating the original intention. Let’s decipher the latest developments in AI meteorology!
today,The topic I will share with you is "Exploring the Future, Intelligently Controlling Weather - Frontier Progress of Artificial Intelligence in Earth Science Research."
The so-called earth science research is mainly the study of the atmosphere, ocean, biosphere, lithosphere and the interaction, exchange and circulation process between them. In fact, the circulation of the atmosphere and the ocean will have a great impact on the earth's meteorology, climate, ecosystem, etc. Simulating and analyzing the changes in the atmosphere and ocean, and then predicting weather and climate, etc., is crucial to the sustainable development of mankind.
AI forecast vs. digital forecast
In the past, physics-based digital forecasting models have made great progress in research, but their development speed is still slow and the demand for computing is very large. With the successful application of deep learning and artificial intelligence in various fields, more and more research institutions are beginning to try to use data-driven methods for weather forecasting.
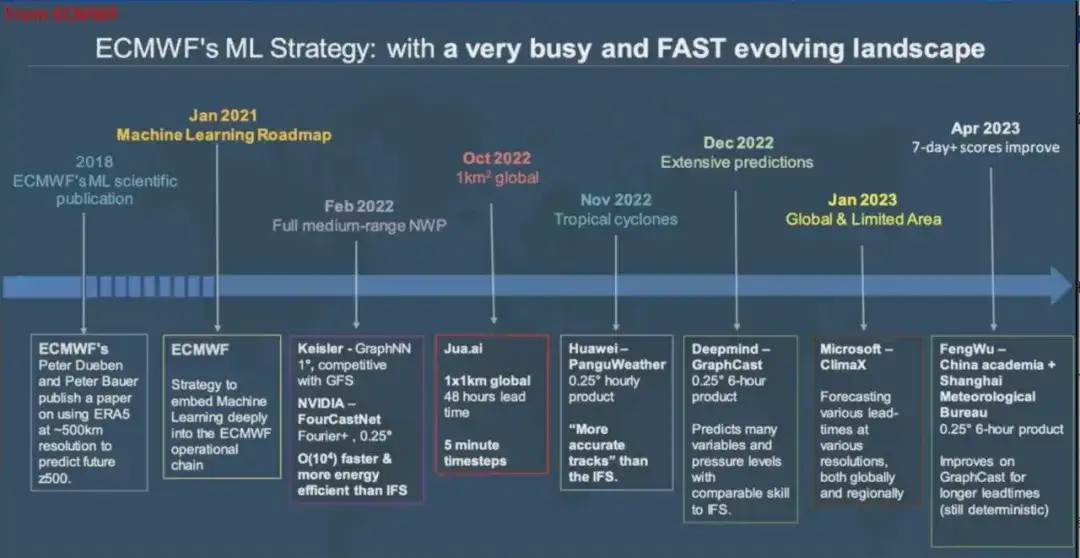
For example,The European Centre for Medium-Range Weather Forecasts is an internationally recognized authority.Since 2018, we have taken the lead in trying to use deep learning technology for weather forecasting, but due to the low-resolution meteorological data at the time, the effect of this attempt was mediocre.
February 2022NVIDIA launches FourCastNet weather modelFor the first time, forecasts were made based on 0.25° high-resolution meteorological data, but the model still did not surpass the physical digital forecast model used by the European Centre for Medium-Range Weather Forecasts, and this model can only predict a small number of meteorological elements.
November 2022Huawei launches Pangu weather modelThe announcement that the model outperformed the European Centre for Medium-Range Weather Forecasts' IFS model on high-resolution meteorological data was seen as a major breakthrough.
One month later,Deepmind The company launched the GraphCast weather model.The main feature of this model is that it can predict more different meteorological elements.
April 2023Our team (Shanghai Artificial Intelligence Laboratory) launched its own large-scale meteorological model FengWu,Compared with all previous models, FengWu has achieved significant improvements in performance.
AI-driven FengWu model achieves optimal typhoon trajectory prediction capability
Rolling Forecast, the inspiration for FengWu model
If we unfold the earth into a plane and grid the plane, we will divide the longitude and latitude of the world into 0.25° spatial resolution (equivalent to a scale of about 25 kilometers). This means that the world is divided into about 720×1440 grid points, and each grid point is divided into 37 different levels in vertical height, involving 169 variables such as temperature, humidity, wind speed, sea surface temperature, surface wind speed, etc. Weather forecasting is based on the global weather element field to predict the changes in the weather element field in the future.
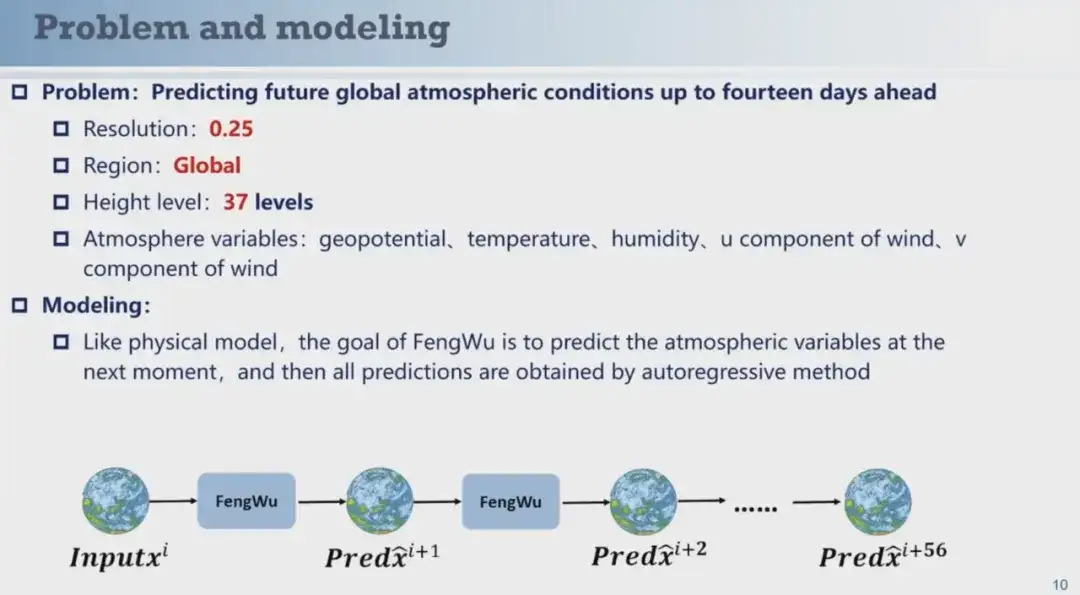
Our team analyzed the hourly global meteorological field data for the past 40 years and found that the global meteorological field at each moment is actually a natural annotation of the field at the previous moment. Therefore, without the need for additional annotation data, we only need to predict the relationship between the meteorological field at two adjacent time points to predict future changes in the meteorological field.This is the original inspiration for the FengWu model.
Specifically, after the FengWu model predicts the meteorological element field at the next moment, it uses it as input to predict the meteorological element field at the next moment, and so on.Such a rolling forecast can produce the meteorological element field to be predicted for the next 14 days.
Two major advantages: long-term forecasting skills + high computing efficiency
The FengWu model has two major advantages.One is to have long-term advance forecasting skills,It can achieve a forecast capability of 10.75 days. In fact, before the emergence of AI methods, the physics-based digital forecast model could improve the forecast skill by 1 day every 10 years on average. After the introduction of AI, the forecast skill can be improved in a few months.
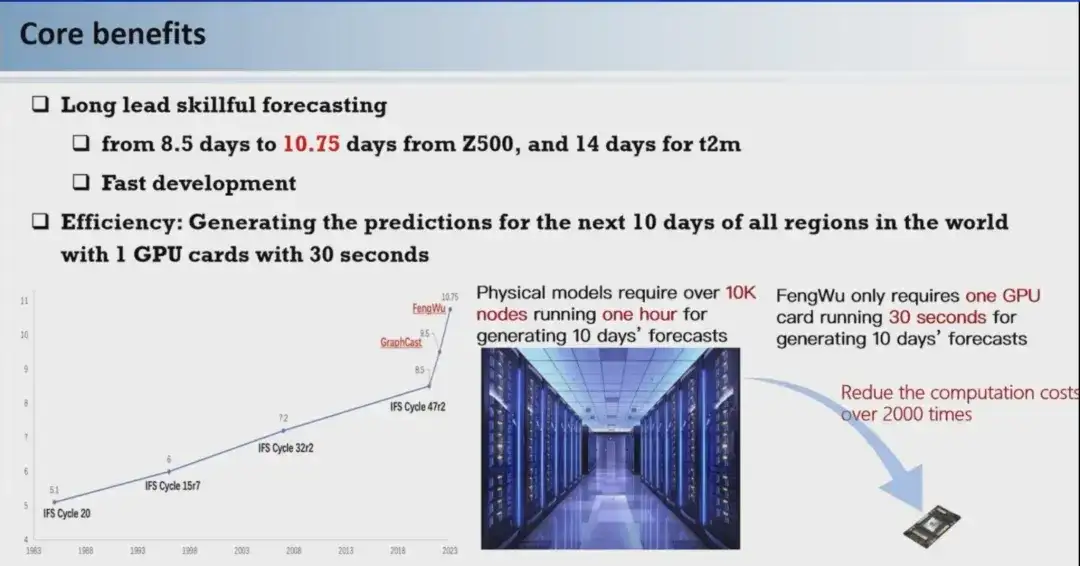
Another advantage of the FengWu model is computational efficiency.In the past, the physics-based digital forecasting model required 10,000 computing nodes to run for one hour to generate forecast results for the next 10 days. However, the FengWu model only needs one GPU to run for 30 seconds to complete the forecast results in the same period of time, which is more than 2,000 times faster than traditional methods.
Mixed feelings: FengWu's strengths and challenges in typhoon forecasting
In order to evaluate the ability of the FengWu model in typhoon trajectory prediction, our team tested it using typhoon data after 2023, and compared the test results with those of the European Centre for Medium-Range Weather Forecasts, the Japan Meteorological Agency, the U.S. Weather Bureau and other institutions.
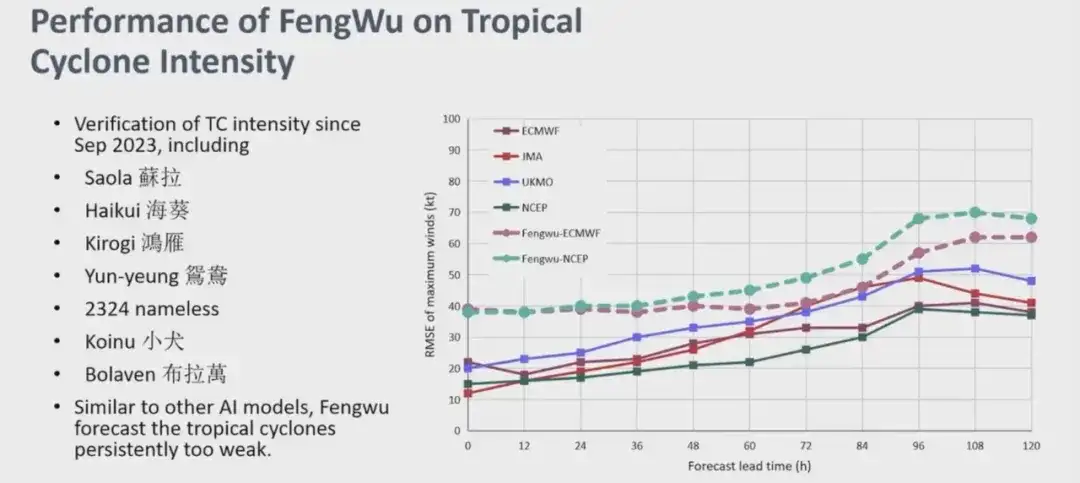
The results show that when the typhoon track is predicted 0-120 hours in advance,The FengWu model has the smallest error in predicting typhoon positions at each node.
Compared with traditional physical methods,There is still a gap in AI’s ability to predict typhoon intensity.This is because all current AI-based models are trained in a data-driven manner. Since there is relatively little data on extreme weather events such as typhoons, AI models tend to smooth the results when predicting extreme weather, resulting in weak performance in typhoon intensity predictions.
FengWu-GHR: For the first time, AI forecast resolution has been increased to 0.09°
Urgent issues to be addressed: high resolution and long-term error accumulation
In fact, after completing the development of the FengWu model, we received feedback from many meteorological experts. One of the feedbacks was that although FengWu can already achieve 0.25° high-resolution prediction,But they still hope to obtain higher-resolution weather forecasts.Another feedback is,The problem of error accumulation caused by long-term forecasts needs to be further resolved.
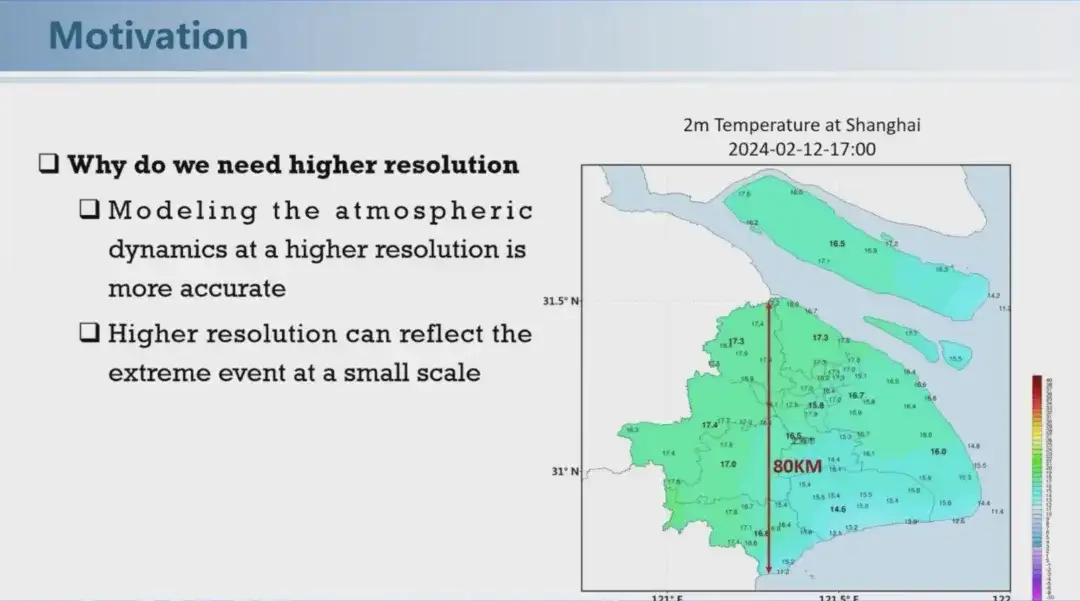
Why do we need more refined and higher-resolution weather forecasts?
Taking the graph of Shanghai's surface temperature as an example, we can see that although Shanghai is not large, the temperature difference between different regions is obvious. With a north-south distance of only 80 kilometers, if we use a 0.25° meteorological forecast model for prediction, we may only get about 3 grid point data, which is not enough to describe the details of weather distribution. Higher-resolution forecast data can provide more accurate atmospheric motion simulation, which in turn brings more refined forecast results.
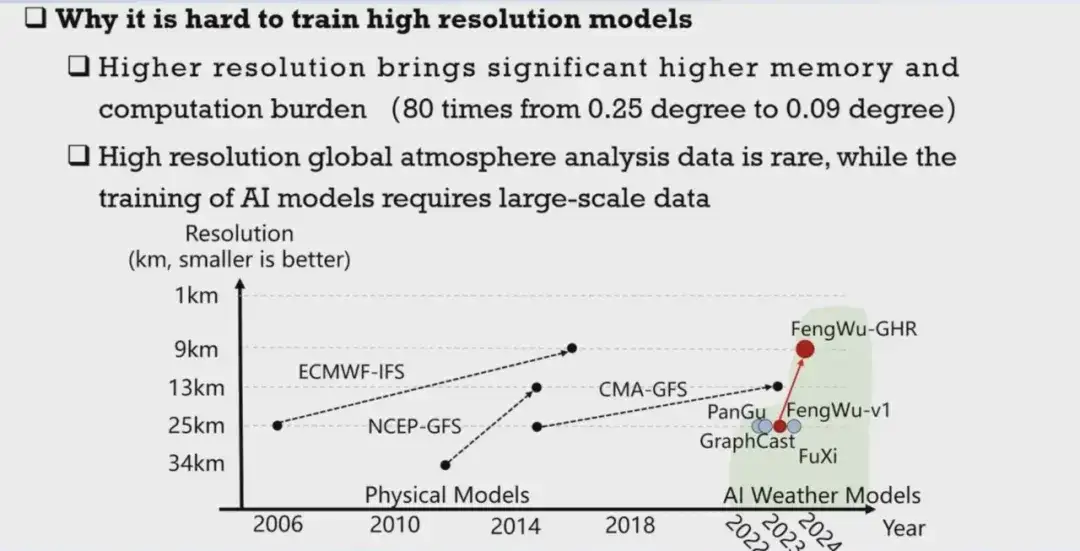
To address this, we launched the FengWu-GHR model, which is the first AI weather forecast model implemented at a high resolution of 0.09°. Its specific implementation process is not easy.
First, increasing the resolution from 0.25° to 0.09° will increase the amount of computation and memory consumption by more than 80 times. Second, higher-resolution meteorological analysis data is very scarce, but AI models require a large amount of data for training, which makes it extremely difficult to train a high-resolution AI meteorological model from scratch.
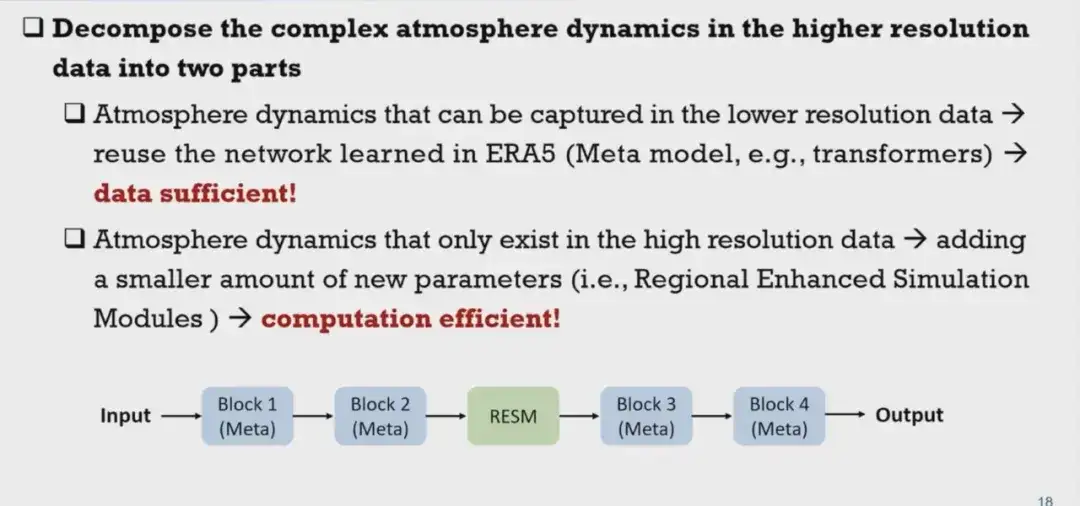
To address these issues, we attempt to decompose the high-resolution atmospheric motion into two different components.
First, a model (meta-model) is trained using a large amount of low-resolution data. Then, the high-resolution meteorological data is decomposed into multiple low-resolution meteorological data, and the meta-model is used to predict each meteorological data. Finally, these prediction results are spliced together to obtain high-resolution meteorological prediction results.
However, this approach does not fully utilize the nonlinear relationship in high-resolution data. Therefore, on this basis, we introduced a new module and a small number of parameters, and trained the module using high-resolution data to better capture the nonlinear coupling relationship between high-resolution regions.
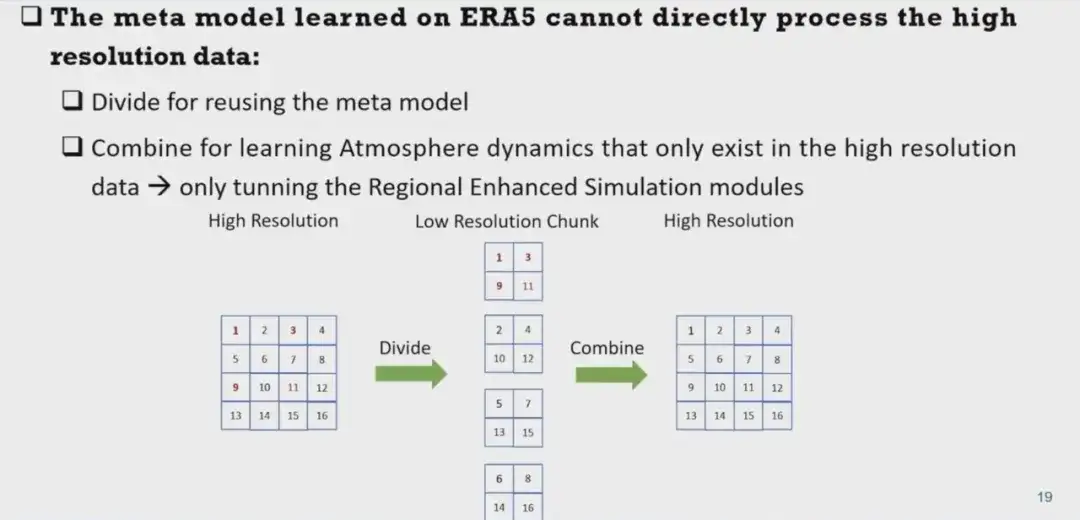
Specifically, the icon on the left side of the above figure is the original high-resolution field, which is divided into 4 different low-resolution fields, and then predicted by the language model. After combining, the prediction of the high-resolution field is obtained, and finally the newly added module is used to capture its nonlinearity.
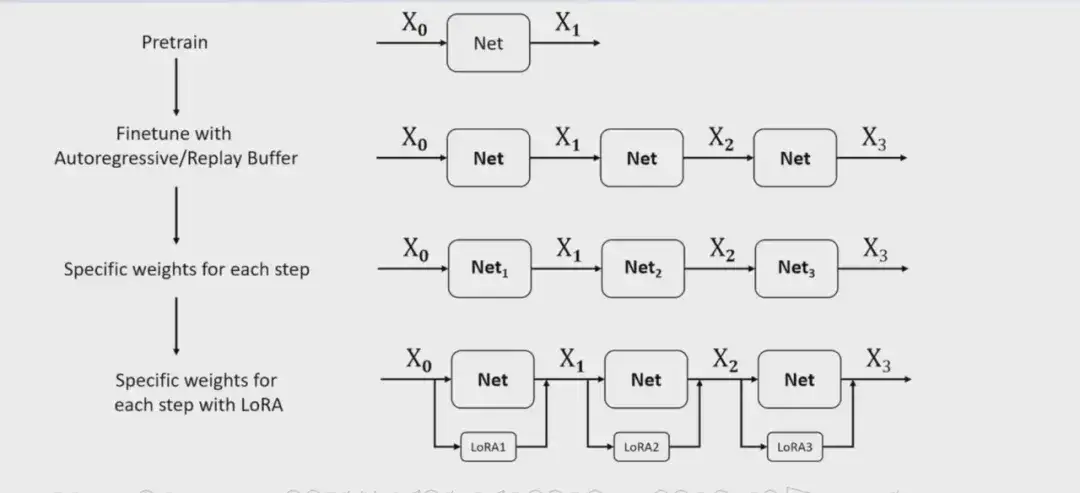
When dealing with the problem of cumulative errors in long-term forecasts,Pangu uses a separate training model at each prediction time point to solve this problem. This is an effective method, but its training cost is very high. Therefore, we added a LoRA module to each step of the prediction process and trained each step with a small number of parameters. This is equivalent to having a new model for each prediction step, but only a small number of parameters are required, which significantly reduces the computational cost.
Model evaluation: FengWu-GHR achieves further upgrade of weather forecast
Since only IFS has achieved a 0.09° resolution result, we use it as a reference standard to verify our test results.
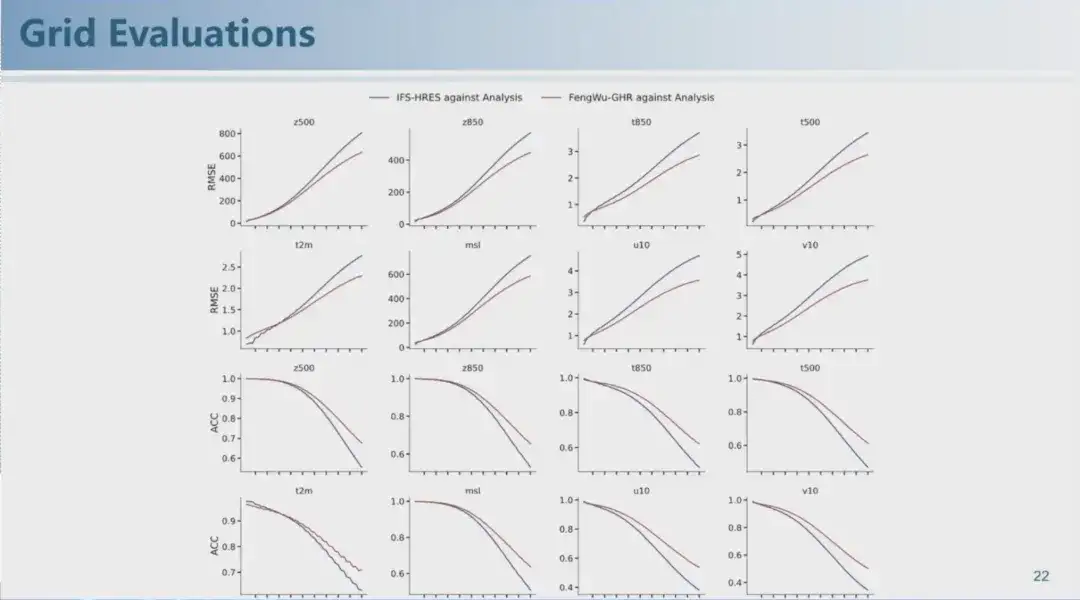
The results show that FengWu-GHR shows obvious advantages in RMSE and ACC indicators, with lower RMSE and higher ACC.
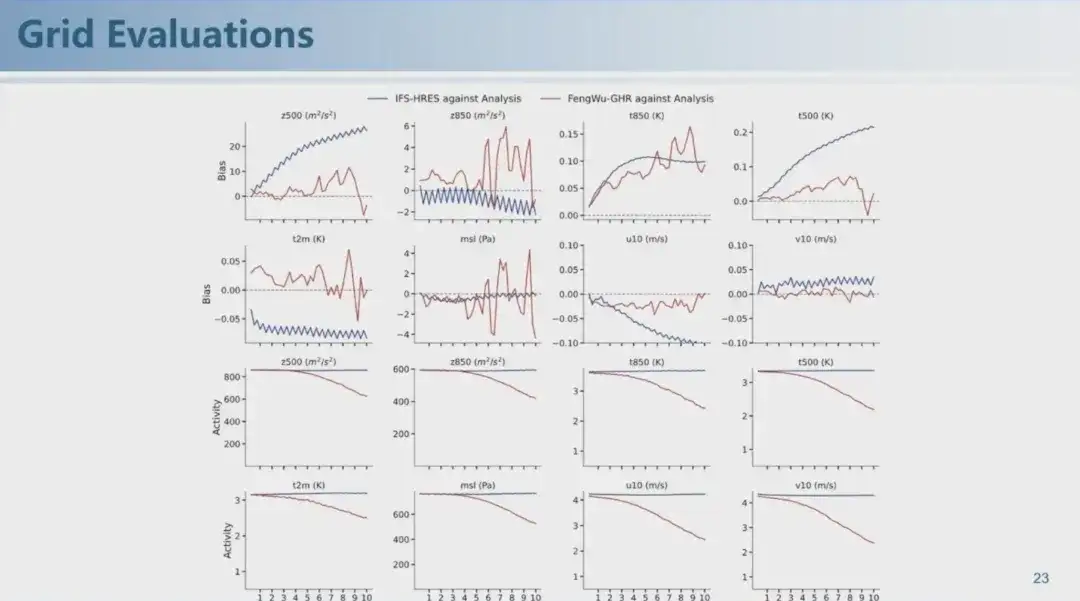
The indicator Bias is used to measure the deviation of the prediction results. FengWu-GHR is closer to 0 and has better test results. The Activity indicator is used to measure whether the prediction results become more ambiguous as the prediction time increases. The results show that the prediction results of FengWu-GHR gradually tend to be smooth, and the model still does not solve the smoothing effect on extreme weather prediction.
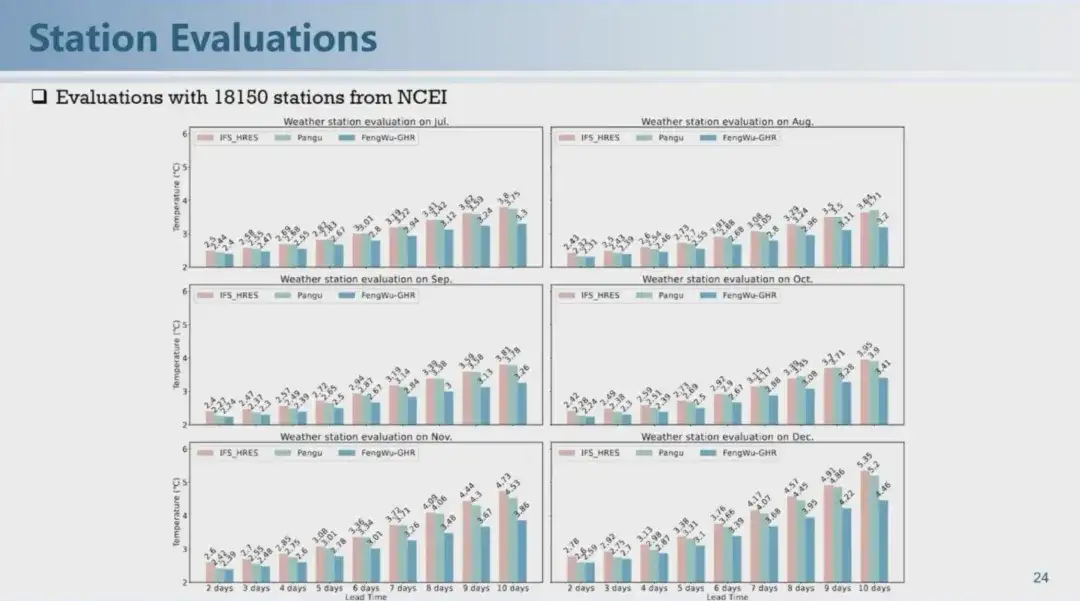
By analyzing the data of the field and the real-time observation data of 18,000 different meteorological stations, we use the data from July to December 2022 to test the model, and compare it with IFS_HRES and Pangu model.FengWu-GHR has an advantage in forecasting results obtained at any number of days in advance.
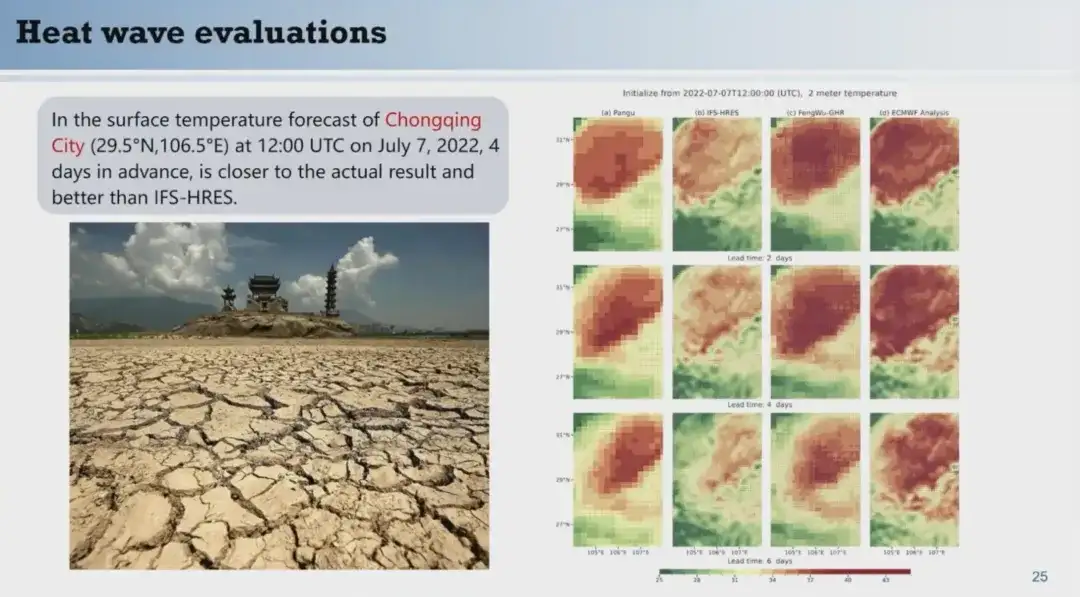
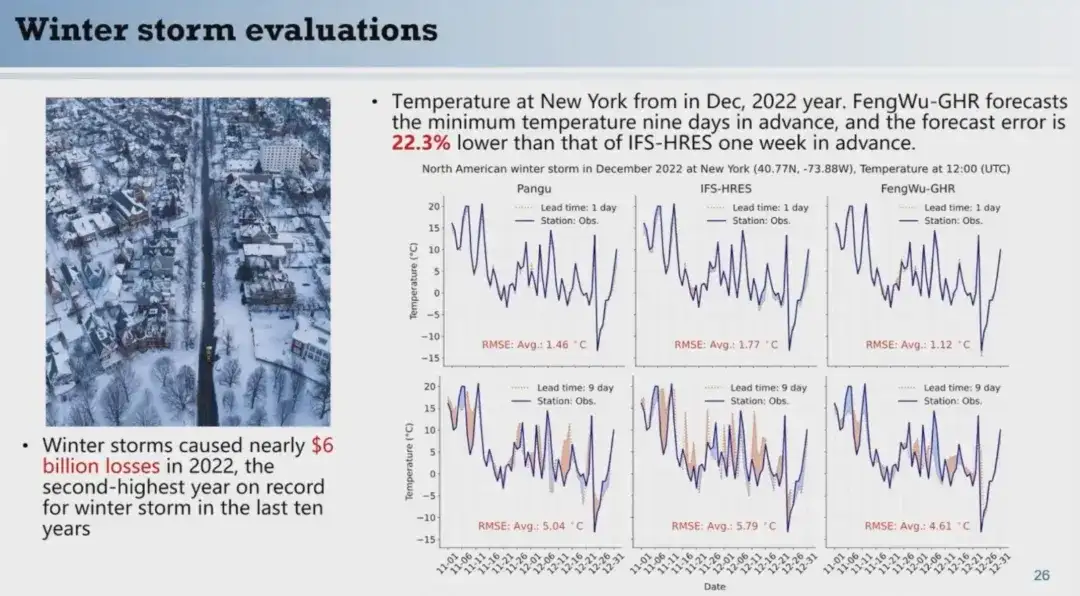
In addition, FengWu-GHR also has advantages in heat wave prediction and cold wave prediction.
Today we are talking about the issue of medium-term meteorological forecasts. In fact, there are many different scales of forecasts in the field of atmosphere, including 1-3 days, long-term, seasonal climate forecasts, etc. At present, we are mainly focusing on the research of medium-term meteorological forecast capabilities, but in the future, we hope to conduct in-depth research to explore whether it is possible to expand from medium-term forecasts to climate-level forecasts, and further study climate evolution and future development trends.