Reshaping the Performance Boundaries of Lithium Batteries, Kang Jianqiang's Team From Wuhan University of Technology Proposed a Simplified Electrochemical Model Based on Ensemble Learning
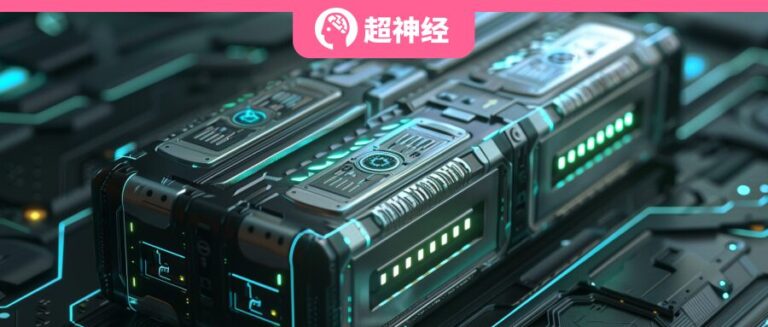
In July 2022, the ageless male god Jimmy Lin had a car accident. As a professional racing driver, the Tesla Model X he was driving suddenly deviated from the predetermined track during driving and crashed into the roadside barrier. The vehicle then caught fire and caught fire again during the towing process of the rescue vehicle. In the end, the entire car was burned to only the frame. Once this accident was reported, it once again aroused people's high attention to "new energy vehicle collision fire".
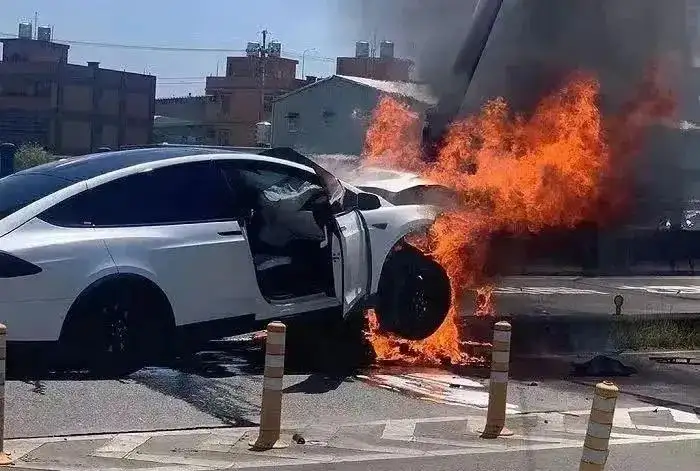
It is understood thatTesla cars mostly use lithium-ion batteries.With the advantages of high energy density, high power density, long cycle and small memory effect, it has been widely used in the field of electric vehicles in recent years. However, the explosion power of lithium batteries is like a small dynamite pack. Uncontrolled battery thermal runaway will cause explosion and fire. Because lithium ions will produce combustion-supporting gases such as oxygen after ignition, this will lead to subsequent secondary combustion and repeated combustion. Once a fire occurs, it is difficult to extinguish. Therefore,How to ensure the safe operation of lithium batteries is a difficult problem that must be solved in the field of electric vehicles.
Based on the chemical mechanism inside the battery, the electrochemical battery model can effectively model the migration process of lithium ions, and by predicting data such as voltage, it can determine the critical state of the battery, ensure the real-time monitoring of the internal state of the battery by the embedded system, avoid sudden thermal runaway caused by overcharging and discharging, aging, increased internal resistance, etc., and maintain the safe operation of the battery. However, the traditional electrochemical model has many parameters and complex calculations, which limits its widespread application in actual battery management systems.
In this regard,Kang Jianqiang's team from Wuhan University of Technology proposed a simplified electrochemical model of integrated learning + FIE.The ensemble learning model is based on machine learning and integrates the discrete-time realization algorithm (DRA), fractional-order Pade´ approximation (FOM), and three-parameter parabolic approximation (TPM). By simplifying the migration process of lithium ions in electrode particles, the change of lithium ion concentration on the surface of electrode particles can be accurately predicted.
Under constant current and dynamic conditions,The ELM proposed by the researchers can achieve more accurate voltage prediction than single DRA, FOM, and TPM models, and its computational complexity is much lower than that of the quasi-two-dimensional model (Pseudo-2D, P2D).
In addition, the researchers also used the first-order inertial element (FIE) to simplify the migration of lithium ions in the electrode solution and accurately predict the lithium ion concentration in the electrolyte near the positive and negative current collectors.
Research highlights:
- A simplified electrochemical model of ensemble learning + FIE is proposed. The ensemble learning integrates DRA, FOM and TPM, and 3,500s of 1C constant current discharge can be completed in 0.1676s.
- Provide strong technical support for the realization of future intelligent battery management system (BMS)
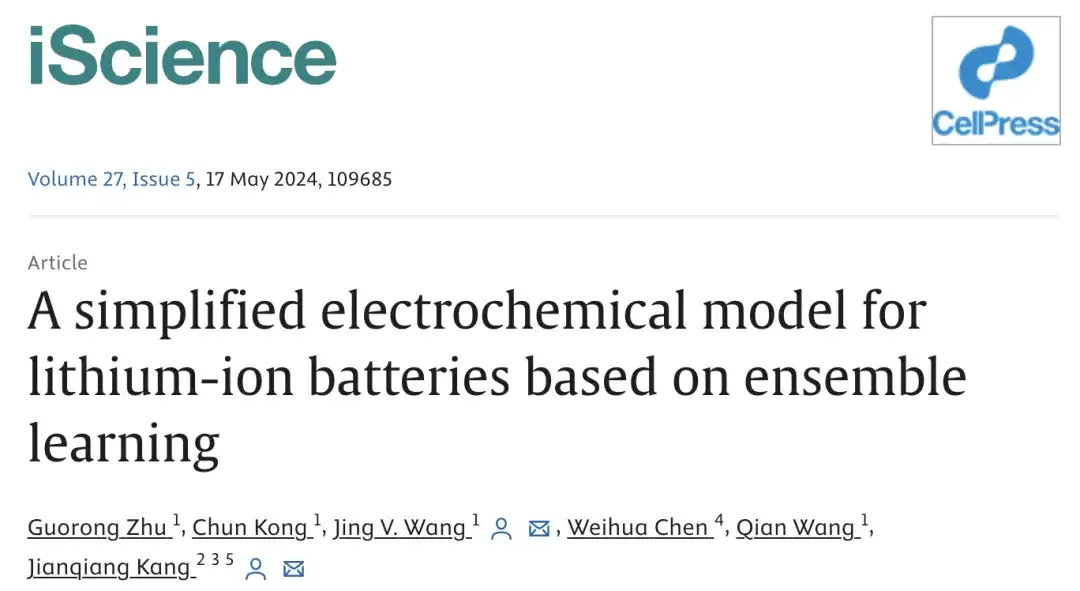
Paper address:
https://www.cell.com/iscience/pdf/S2589-0042(24)00907-6.pdf
Three experimental assumptions to simplify the complex structure of batteries
In order to speed up the calculation of electrochemical models and simplify the structure of traditional electrochemical models, the researchers proposed a simplified lithium-ion battery electrochemical model (single particle model) and made basic assumptions about it.
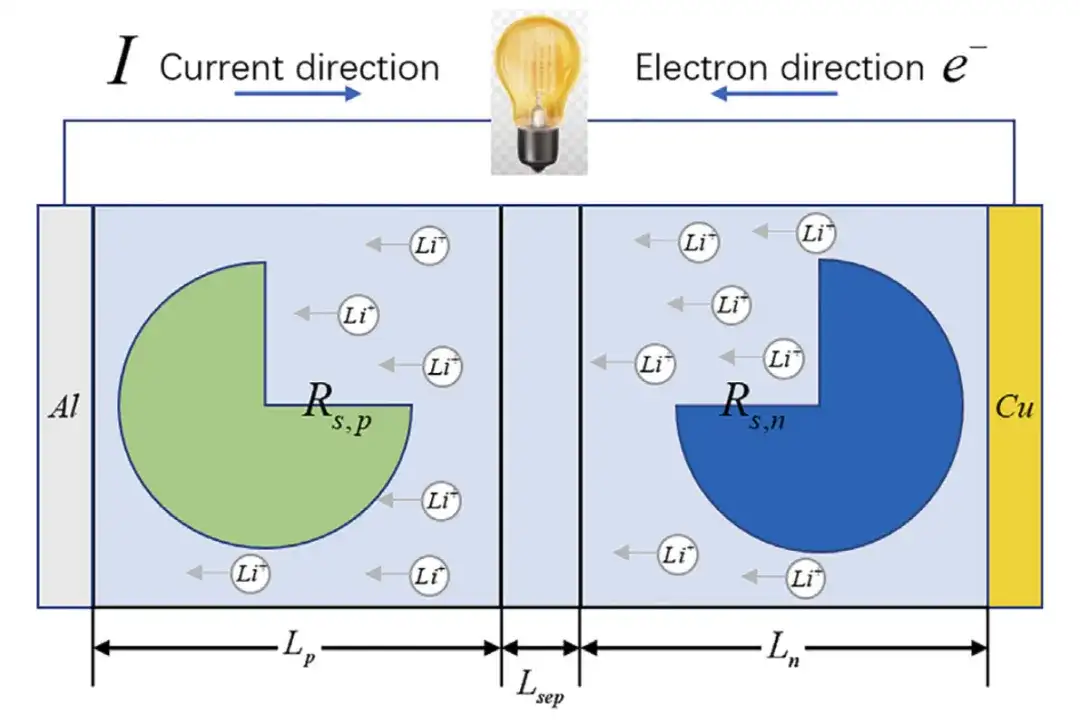
Assumption 1:The battery electrode is considered as a single spherical particle, and the electric potential at the same radius from the center of the sphere is equal;
Assumption 2:Solid phase diffusion is considered only in radial direction;
Assumption 3:The lithium ion pore wall flux (Ji) is uniform in the electrode particles.
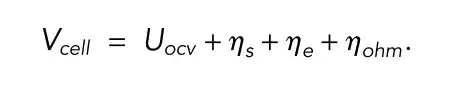
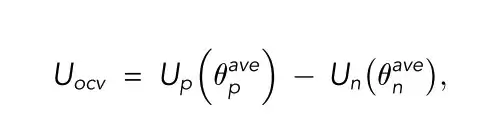
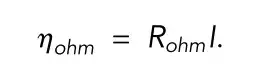
Vcell is the cell terminal voltage; Uocv is the open circuit voltage; η is the overpotential; Rohm is the total ohmic resistance; I is the current; ⍬ave is the average solid phase stoichiometry
In the single-particle model, the battery terminal voltage is considered to be only related to the open-circuit voltage and the reaction overpotential, which greatly reduces the computational complexity of the model.
Integrated learning + FIE to predict lithium ion concentration in solid electrodes and electrolytes
The researchers proposed an integrated learning + FIE model.Among them, integrated learning can be used to predict the lithium ion concentration in the positive and negative electrode solid particles, and FIE can be used to predict the lithium ion concentration in the electrolyte phase.
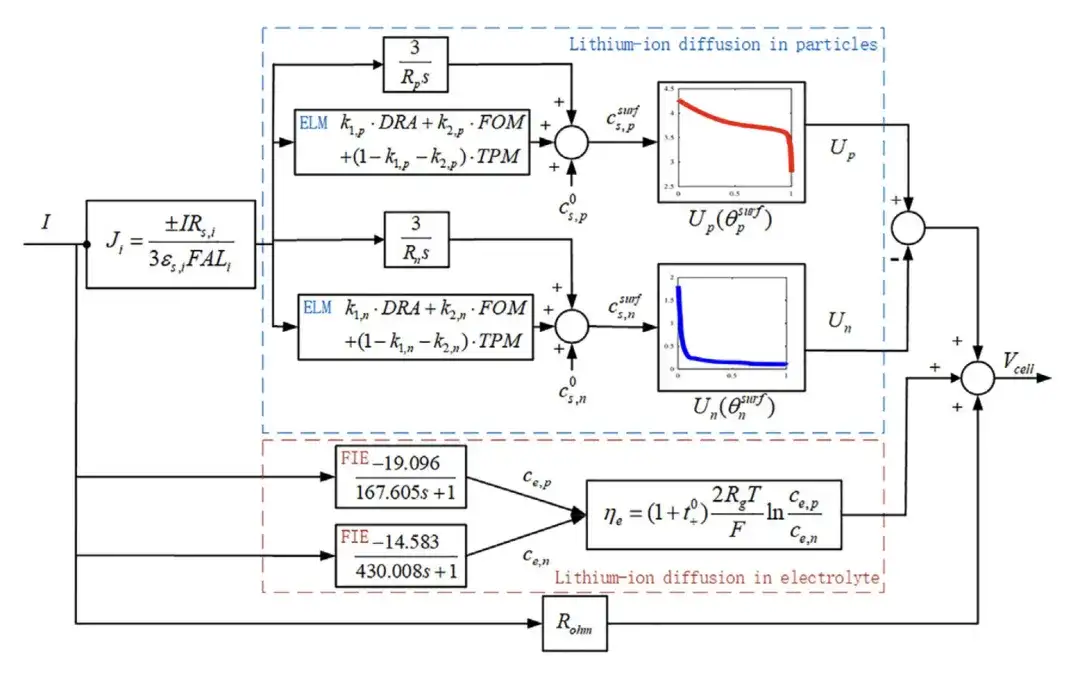
Ensemble learning: integrating three models to more accurately predict the lithium ion concentration of solid-phase electrodes
Ensemble Learning Model (ELM), as a typical machine learning technique, can achieve better prediction performance than any single model by combining multiple models.In previous literature, the discrete-time realization algorithm (DRA), fractional-order Pade´ approximation (FOM), and three-parameter parabolic approximation (TPM) have shown a certain accuracy in predicting the lithium ion concentration on the surface of electrode particles.
In order to further improve the prediction accuracy of lithium ion concentration in electrode particles, the researchers integrated DRA, FOM and TPM using the weighted average method and proposed the ELM model, whose output equation is as follows:

*ELM output equation; k1, k2 are the weight coefficients of the ensemble learning model
Experiment 1: Predicting the lithium ion concentration on the surface of the negative electrode solid phase electrode particles
Taking the P2D model as the baseline control group, the five different models, DRA, FOM, TPM, ELM, and P2D, were compared to predict the changes in lithium ion concentration on the surface of negative electrode particles.
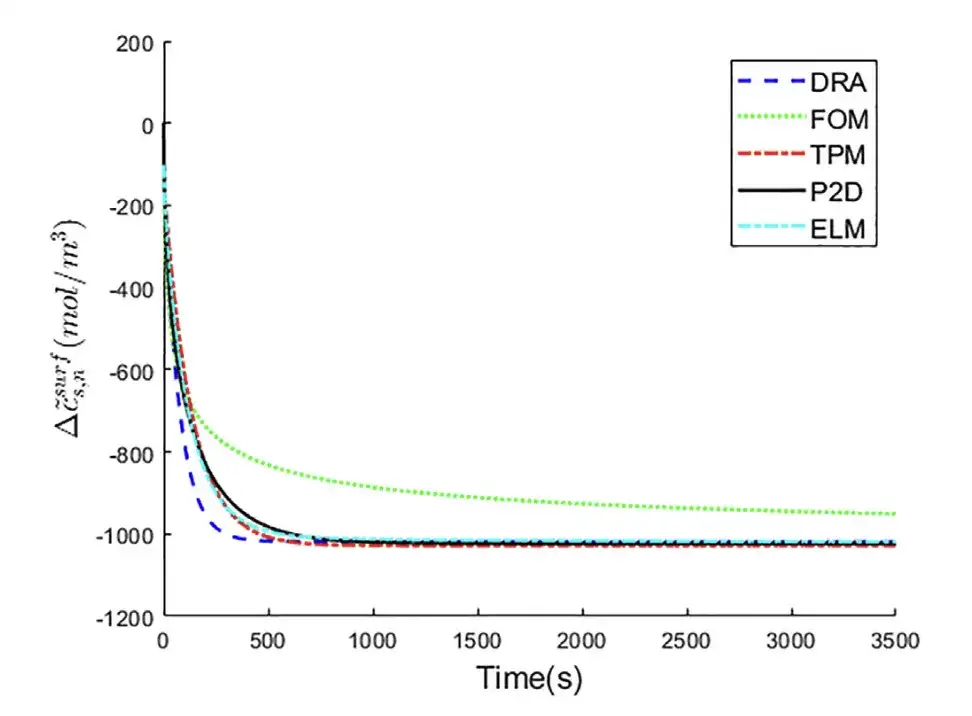
It can be seen that the ELM curve is closer to the P2D model curve.That is, ELM achieved the best prediction accuracy.

The loss function is defined as the root mean square error (RMSE) between the output of the ELM model and the true value of the particle surface △cs,n~surf. It can be seen thatELM has the lowest RMSE, which is only 11.51 mol/ m3.
Experiment 2: Predicting the lithium ion concentration on the surface of the positive solid electrode particles
Taking the P2D model as the baseline control group, the five different models, DRA, FOM, TPM, ELM, and P2D, were compared to predict the changes in lithium ion concentration on the surface of the positive electrode particles.
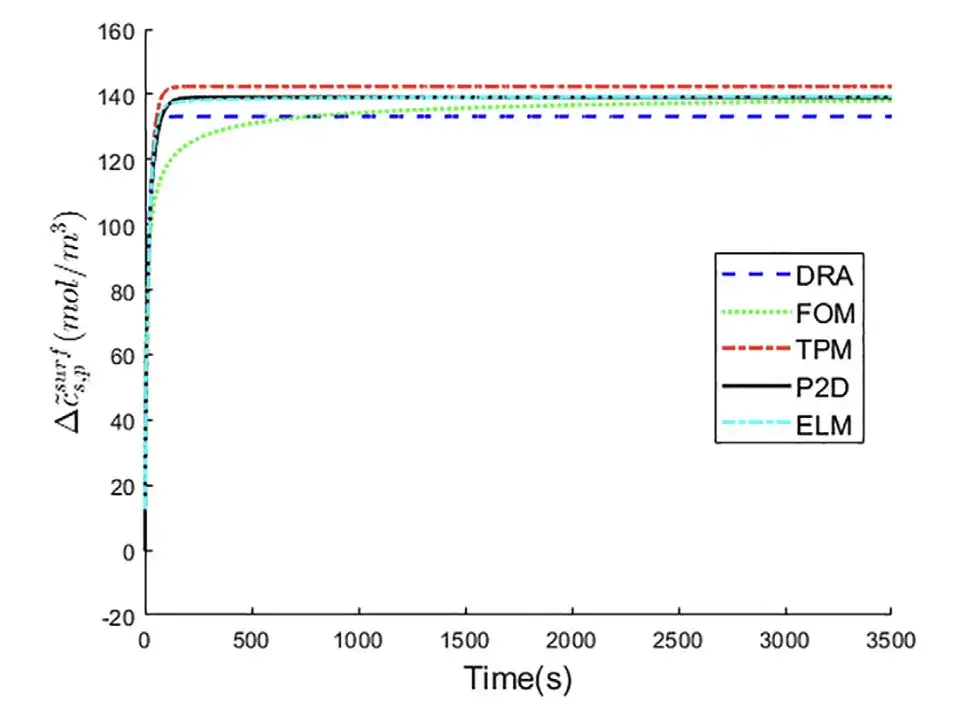
It can be seen thatThe curve of ELM is closer to that of P2D model.It shows the best △cs,p~surf prediction performance.

As can be seen from the above table,ELM has the smallest prediction error, RMSE is only 0.6 mol/m3, and MAPE is only 1.66%.
FIE: More accurate prediction of lithium ion concentration in electrolyte phase
Since the lithium ion concentration in the electrolyte near the positive and negative current collectors directly affects the battery voltage, and thus affects the battery state.The researchers proposed FIE to fit the changes in lithium ion concentration in the electrolyte near the positive and negative electrode current collectors.The P2D model was used as a baseline control group to compare it with the changes in lithium ion concentrations in the positive and negative electrode electrolyte phases predicted by FIE.
Experiment 1: Predicting the change in lithium ion concentration in the electrolyte near the negative electrode current collector
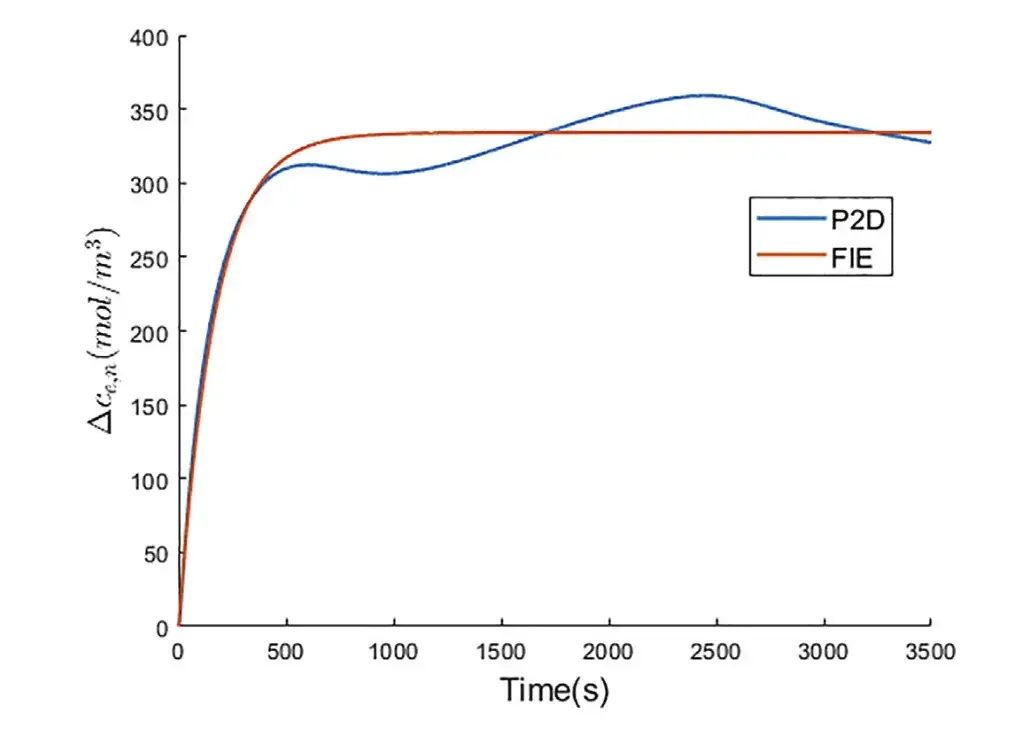
The figure above shows that the two curves are close. In the P2D model, due to the uneven flow of lithium ions on the surface of the electrode particles along the thickness direction of the electrode, the △ce,n curve fluctuates after the relaxation time. The researchers previously assumed that the lithium ion flux on the surface of the electrode particles is constant under constant current. Therefore, △ce,n remains constant after the relaxation time.
*Relaxation time is a measure of the battery's recovery capacity, characterizing the battery's ability to recover from a polarized state to an equilibrium state.
Experiment 2: Predicting the change in lithium ion concentration in the electrolyte near the positive electrode current collector
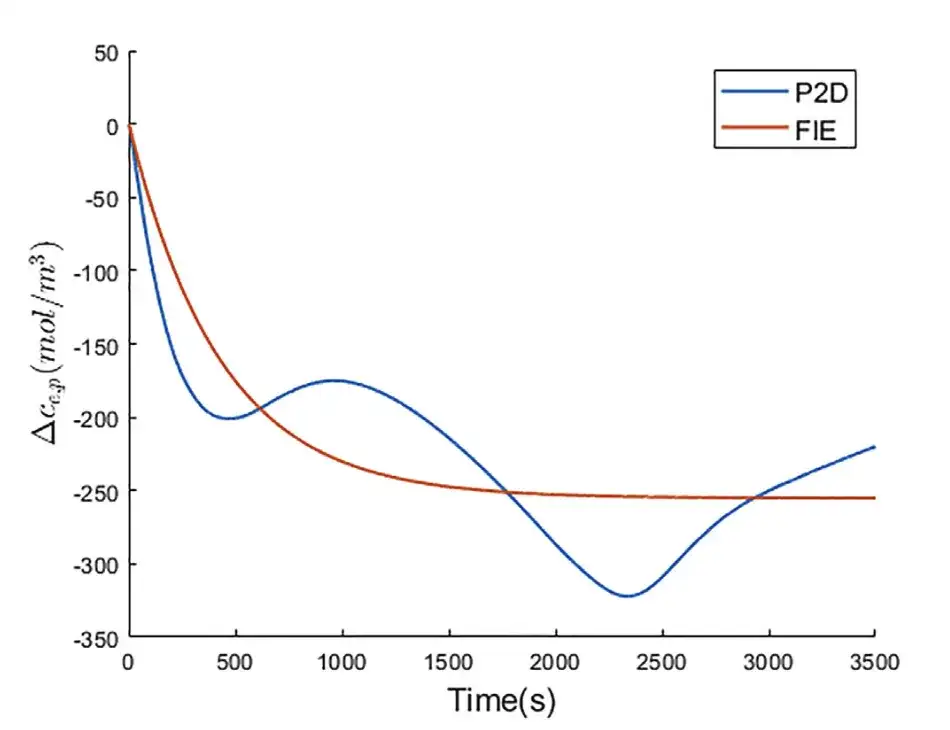
As shown in the figure above, in the P2D model, the fluctuation of △ce,p is greater than that of △ce,n because the lithium ion flux on the surface of the positive electrode particles is more uneven than that on the surface of the negative electrode particles. Compared with the P2D model, FIE achieves accurate prediction of △ce,p with an RMSE of 39.136 mol/m3.
In summary,The ensemble learning proposed by the researchers can accurately predict the lithium ion concentration of the solid electrode, with better prediction ability than a single model. The FIE model can also accurately predict the lithium ion concentration of the electrolyte phase.
Ensemble learning predicts voltage more accurately
Experiment 1: Comparison of voltage predictions by different models
The researchers conducted constant current discharge simulations on LiMn2O4/Carbon batteries at 0.5C, 1C and 2C rates, and compared the results and errors of voltage prediction using five models: ELM, DRA, FOM, TPM and P2D.
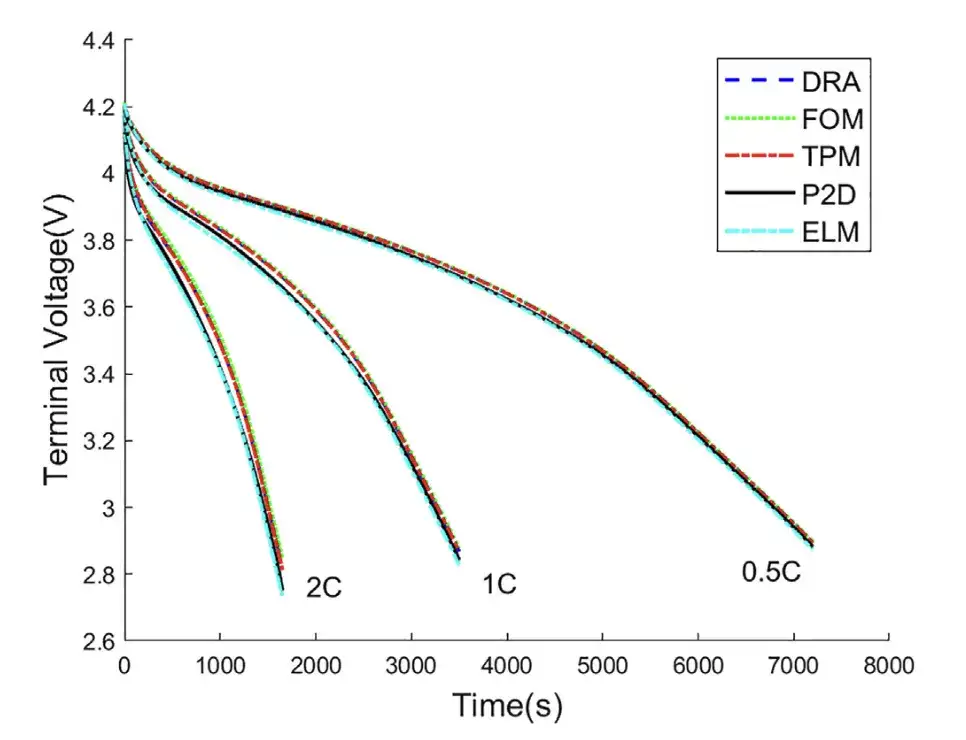
According to the above figure, under 0.5C discharge rate, the voltage curves of DRA, FOM, TPM, and P2D models are very close to the P2D model. Under 1C and 2C discharge rates,The ELM voltage curve is closer to the P2D voltage curve.

As shown in the table above, the ELM model has the smallest RMSE and MAPE, and the lowest voltage error. As the discharge rate increases, the voltage errors of these four models increase. In summary,The proposed ELM achieves better voltage prediction over a wider current range.
Experiment 2: Comparison of computational complexity of different models
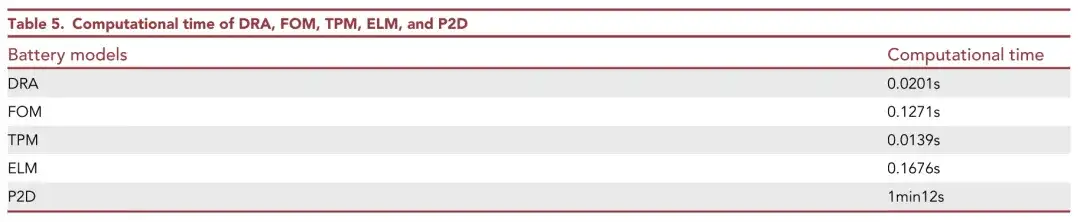
The researchers conducted a 1C rate constant current discharge simulation and compared the calculation time of different models. Since DRA and FOM only have two second-order state space equations, the calculation speed is faster. FOM is slightly slower, and ELM only takes 0.1676s to complete 3,500s of 1C constant current discharge. The calculation speed of the P2D model is much slower than other models.
Discharge test is an important means to evaluate battery performance.
Experiment 3: Verifying the effectiveness of the ELM model under dynamic conditions
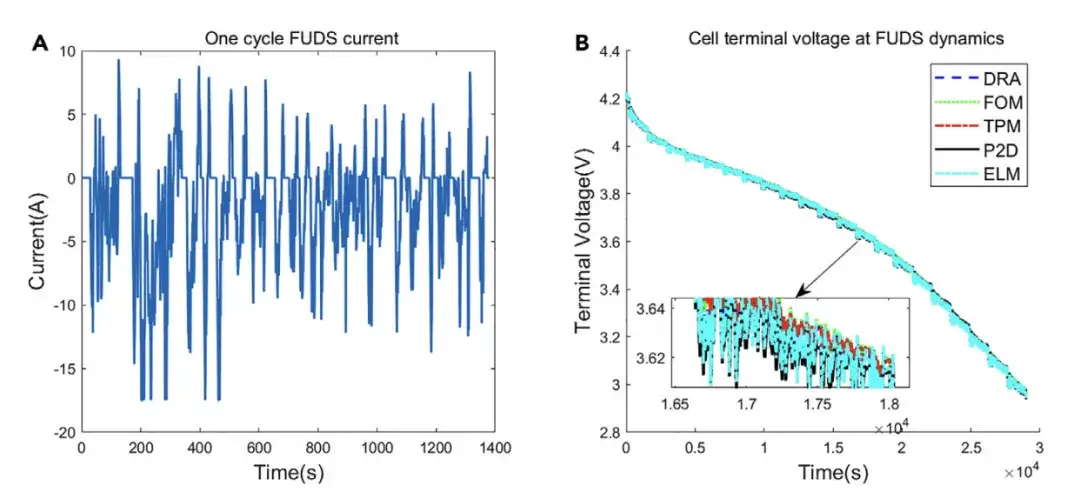
To verify the effectiveness of the ELM model under dynamic conditions, the researchers conducted FUDS dynamic simulations on different models. The results show that since the current change rate is small most of the time in FUDS dynamics, the voltage curves of DRA, FOM, TPM and ELM are close to the P2D model.

As shown in the table above,ELM achieves the most accurate voltage prediction among these models.There is only an RMSE of 4.48 mV and a MAPE of 0.097%.
AI guarantees the safety of lithium batteries under green transformation
Under the general trend of "carbon neutrality and carbon peak", countries have begun to pay attention to the transformation of the energy system, and all walks of life have actively transformed towards low-carbon and sustainable development. Lithium batteries, as the first choice of environmentally friendly batteries, are widely used in energy storage power systems, electric bicycles, electric vehicles, military equipment, aerospace and other fields.
Although lithium batteries are widely chosen for their high energy density and long life, they can also seriously threaten the safety of the public if used improperly or have quality problems. For example, in June 2023, a fire broke out in an electric car store in Chinatown, Manhattan, New York City, killing four people and injuring three. The cause was the thermal runaway explosion of lithium batteries during charging.It is urgent to pay attention to the safety of lithium batteries.
In addition, the performance degradation of lithium batteries is affected by many factors such as ambient temperature and charging and discharging conditions. Traditional physical models are based on limited electrochemical laws and it is difficult to effectively evaluate the internal state of the battery. In this context, AI technology stands out with its powerful data processing and feature extraction capabilities.Some people in China have already conducted relevant research on AI+ lithium battery safety.
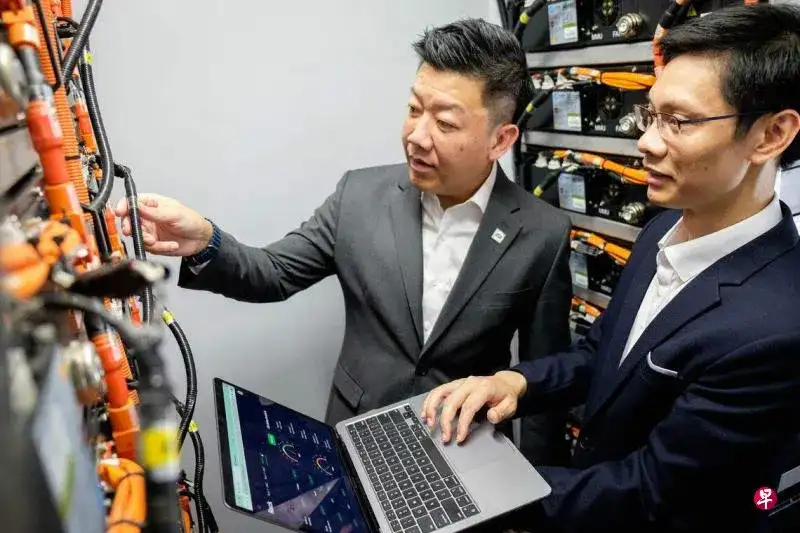
In 2023, Nanjing University and lithium battery manufacturer Durapower developed a Fire & Explosion Management System (FXMS) that helps enhance the safety of lithium batteries and extend their service life. The system uses digital twin technology to replicate real batteries and monitor battery performance using virtual models, which can predict the battery's status in the next five years.It helps staff determine when batteries need to be replaced, with a prediction accuracy of up to 95%.
The main function of digital twins is to collect real-time data from the real world, use machine learning and analysis technology to process the data, simulate and predict the reactions and situations that objects may produce in the real world, and then study their performance.
In March 2024, the team of Associate Professor Wan Jiayu from the Puyuan School of Future Technology at Shanghai Jiao Tong University proposed a semi-supervised learning technology called partial Bayesian co-training (PBCT), which makes full use of the low-cost and abundant unlabeled data generated during the life cycle of lithium batteries. By extracting hidden information from the data, it deepens the understanding of the underlying data patterns. Compared with existing methods,PBCT has achieved an improvement of up to 20% in life expectancy prediction accuracy.And there is almost no additional data collection cost. (Click here for details: Lithium battery life prediction accuracy improved by 20%! The Shanghai Jiaotong University team released a semi-supervised learning method PBCT to extract hidden information from unlabeled data)
From digital twins to semi-supervised learning, technological advances have spawned more innovative solutions and brought new possibilities for the future development of the energy sector.
References:
https://www.zaobao.com/news/singapore/story20231108-1448759
https://m.163.com/dy/article/J3