Appeared in the Top Astronomy Journal MNRAS! The Shanghai Astronomical Observatory of the Chinese Academy of Sciences Used AI to Discover 107 Neutral Carbon Absorption Lines With a Detection Accuracy of 99.8%
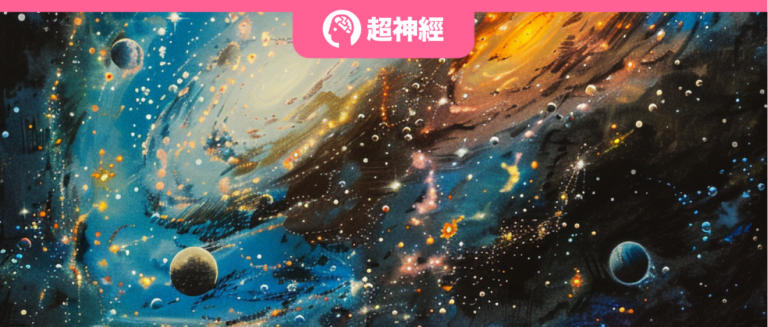
When people look up at the starry sky, the distant starlight has actually traveled through billions of years of time and space, telling ancient stories. As a key probe of cold gas clouds in early galaxies, neutral carbon absorption lines are like witnesses of the history of the universe. Their existence provides a window for people to peek into the mysteries of the stars.
In the process of stellar evolution, the materials released by stellar explosions contain rich chemical elements. These elements undergo nuclear fusion reactions inside the stars and diffuse into the surrounding space with the explosions. Among them, interstellar dust including carbon, oxygen, silicon and other elements also accumulates in the interstellar medium with the diffusion of the explosions, which not only provides an important material basis for the formation of new stars and planetary systems, but also plays a key role in the cooling and condensation of the interstellar medium.
Studies have shown that in different interstellar media, the absorption lines of neutral atomic carbon (CI) at wavelengths of 1560 and 1656 can be used to detect the abundance of cold gas, thereby revealing the formation of molecular clouds, interstellar dust, and stars. However, the current sample size of quasar spectra containing CI absorption lines is too small to be a powerful tool for understanding the overall chemical abundance evolution of the early universe and the evolution of galaxies.
Recently, an international team led by Ge Jian, a researcher at the Shanghai Astronomical Observatory of the Chinese Academy of Sciences, used deep learning methods to search for neutral carbon absorption lines (C Ⅰ absorption lines) in the data released by the Sloan Sky Survey Phase III, revealing the mystery of the composition of cold gas clouds in galaxies in the early universe.107 examples of neutral carbon absorption lines in the early universe were discovered.This discovery not only refreshes people's understanding of the evolution of galaxies in the early universe, but also proves the great potential of artificial intelligence in astronomical research. The relevant research results have been published in the Monthly Notices of the Royal Astronomical Society (MNRAS).
Research highlights:
- This study used a modified deep learning algorithm to search for CI absorption lines using Mg II absorption lines as markers.
- The study found 107 examples of neutral carbon absorption lines in the early universe, nearly twice the number of samples obtained previously.
- This study can detect more weaker signals than before, providing a new research method for future studies of the early evolution of the universe and galaxies.
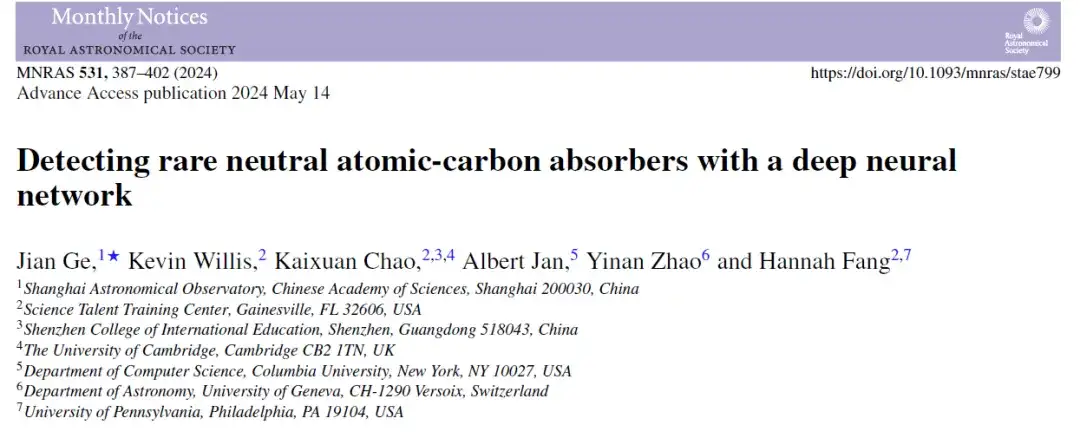
Paper address:
https://doi.org/10.1093/mnras/stae799
Dataset: Using Mg II absorption line as a marker, generate 5 million random samples
Since the C Ⅰ absorption line is difficult to detect, this study narrowed the search scope and only studied QSOs (Quasi-Stellar Objects) known to have Mg Ⅱ absorption lines, using the Mg Ⅱ absorption line as a signpost for discovering absorption by other atomic species.This helped reduce the total number of search targets to about 14,000.
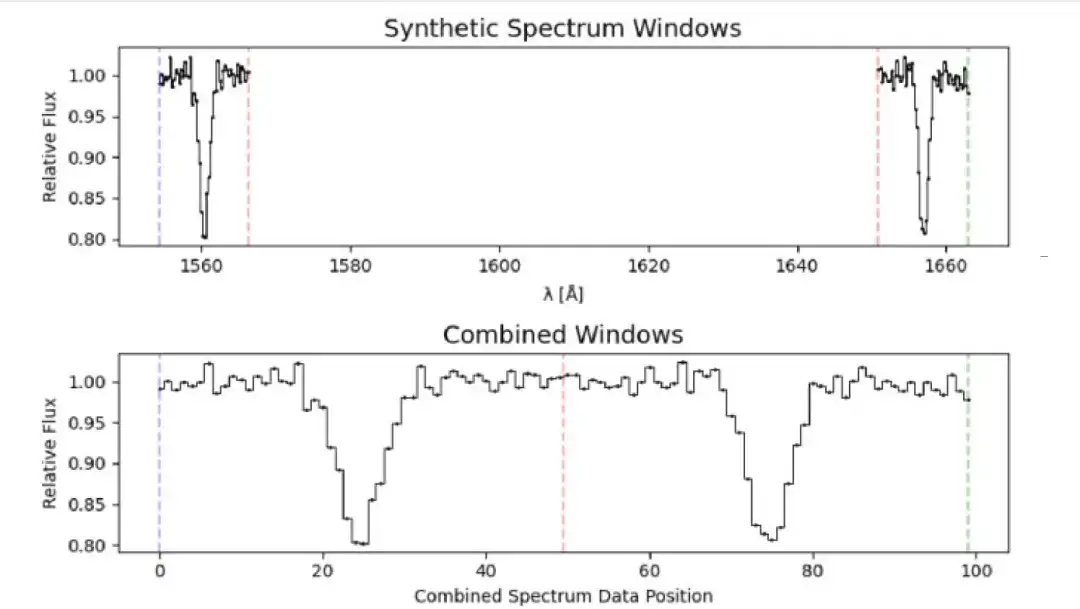
Considering that the two C Ⅰ absorption lines are usually very weak and rare, they are far apart from each other at rest wavelengths of 1560 and 1656 Åare, making the search difficult for deep neural networks.This study innovatively proposed the "fake doublet method".A small part of the spectral region around the two C I absorption lines can be extracted to form a pseudotype C I double absorption line.
Then, a 100-element-long 1D flux array consisting of two 12Å windows connected together provides a clear view of local spectral features and signal-to-noise, while excluding the entire wavelength range between absorption lines, thus reducing the sample size and computational requirements. Afterwards, the deep learning program can easily search for Mg II and Ca II doublet absorption lines in it.By appropriately training the neural network, it is possible to search for unsaturated CI doublet absorption lines in quasar spectra.
Due to the uncertainty of the absorption red shift value in the Mg II absorption line, the spectra used in the actual search may have a wavelength deviation of up to about ±0.25 Å. For this reason, this study applied the same range of random offsets to the CI absorption line in each generated sample, and a total of 5 million positive and negative samples were generated with the same number of positive and negative samples. Among them, the positive samples contain two CI absorption lines, and the variance parameter is randomly sampled from a uniform distribution in the range of 0.05-0.8 Å; the negative samples do not contain CI absorption lines, and the variance parameter is randomly sampled from a uniform distribution in the range of 0.2-1.0 Å.
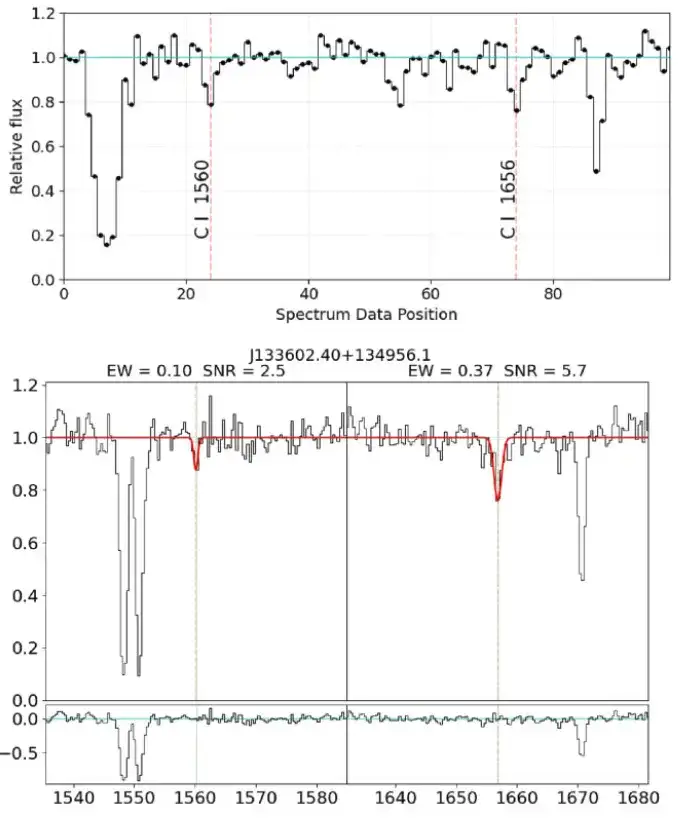
To simulate the noise in the training dataset, this study randomly draws samples from a Gaussian distribution and assigns a signal-to-noise ratio (SNR) to each spectrum by sampling from a triangular distribution. This process results in an average SNR of about 8.0 for the training set, which is very close to the average SNR of 8.4 for 100,000 QSO spectra in SDSS DR12.In this study, the signal-to-noise ratio of the synthetic dataset was intentionally biased toward lower values to enhance the model's ability to detect weak CI absorption lines.
Model construction: The model accuracy rate is as high as 99.8%, proving that convolutional neural networks are very effective
The convolutional neural network model in this study is designed to identify two CI absorption lines in each input spectrum. The model consists of several key components, including a single convolutional layer, a batch normalization layer, a flatten layer, and three dense layers.
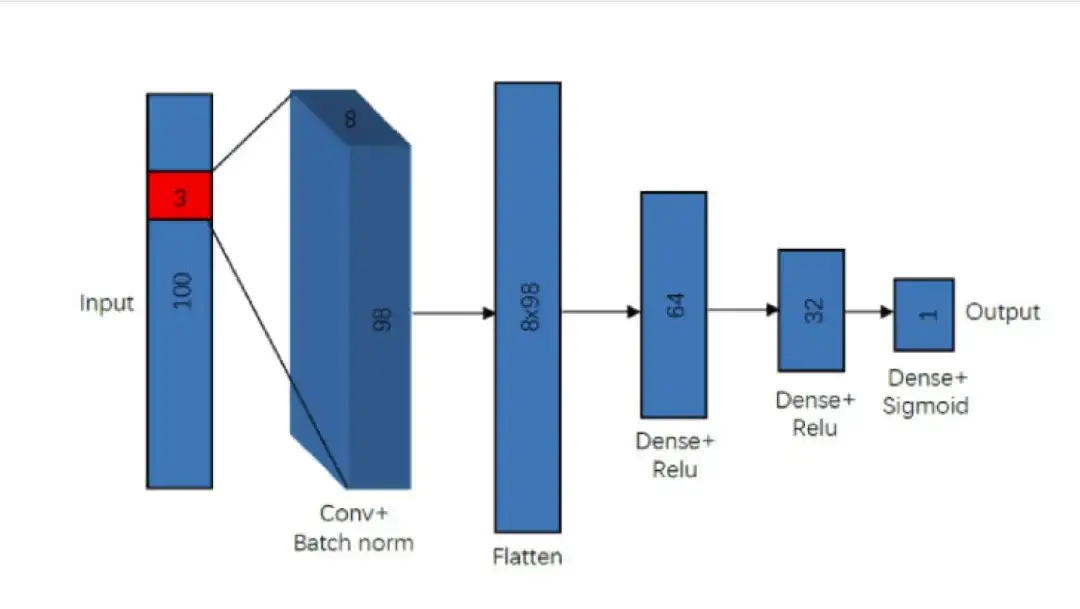
Before inputting into the model, the study normalized the noise of each spectrum, effectively eliminating the influence of model noise. After noise normalization, the study also divided the result by 30 and added 0.5 to keep the flux value in the range of 0 to 1, which ensured that the data of the first layer (convolution) of the model was normalized and partially helped the normative consistency of the second layer (Batch normalization).
The convolutional layer is mainly used to detect spectral lines and their positions.After extensive experiments and testing, we found that a single convolutional layer with 8 filters and a kernel size of 3×3 was sufficient.
After convolution,The samples are passed through a batch normalization layer to ensure that the data is in the correct value range for the subsequent dense layer. The Flatten layer is mainly used to "flatten" the input, that is, to convert the multi-dimensional features output by the convolutional layer into a one-dimensional vector.
The first two dense layers of the model use a linear activation function (ReLU) and are assigned a dropout layer. At the same time, the output layer is also a dense layer with only one neuron, using the Sigmoid activation function. This relatively simple design provides excellent detection accuracy, while the training and search speeds are also very fast.
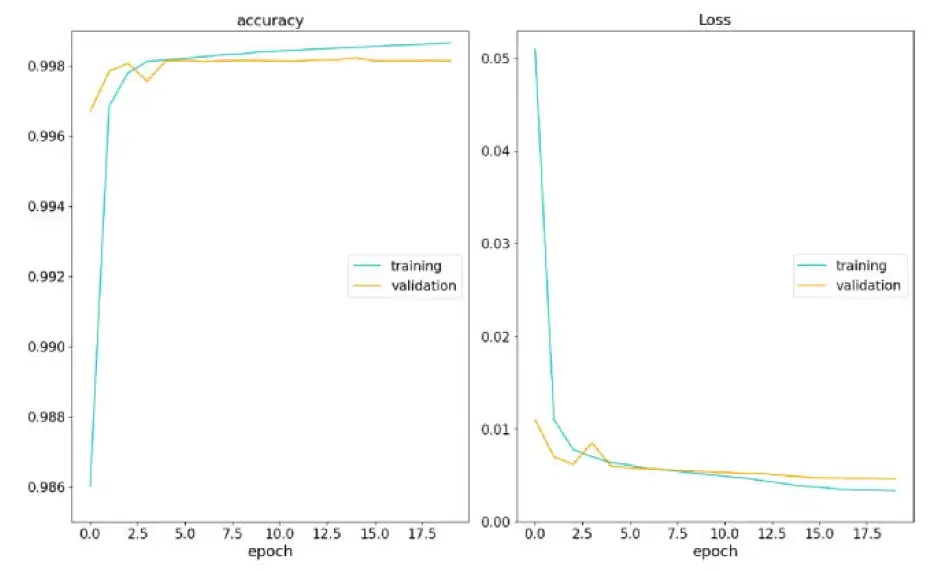
After that, the model was run for a total of 20 iterations. In each iteration, all training samples were passed through the model in groups of 32.Overall, the model is 99.81% accurate.This high accuracy demonstrates that convolutional neural networks are very effective in detecting CI absorption lines in spectra.
Research results: 107 CI absorption lines were selected, and CNN has unlimited potential in exploring weak signals
In this study, the trained CNN was finally used to search a dataset of 14,509 quasar spectra from the Mg II catalog, focusing on quasars with redshifts between 1.3 < Z(abs) < 2.7.
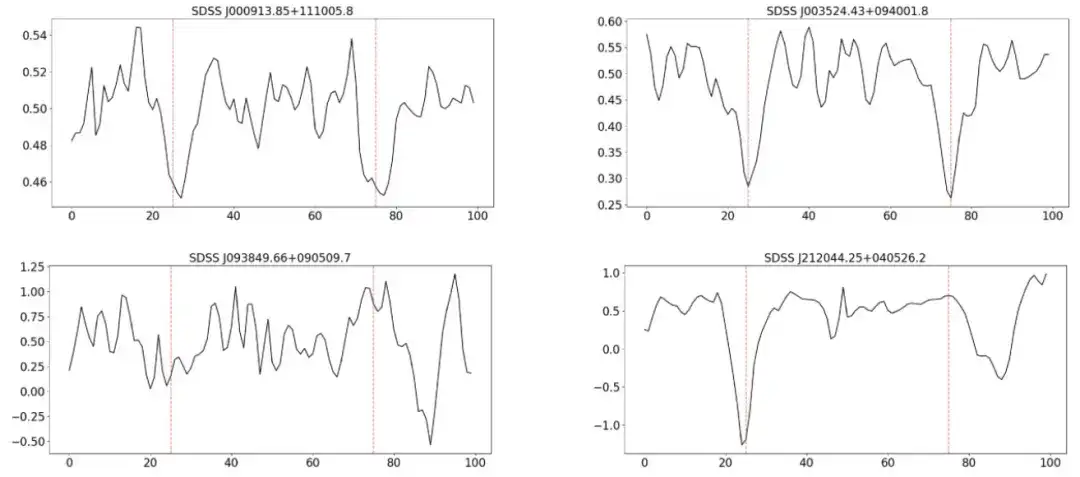
The steps to detect and select absorption lines are as follows:
Initial CNN recognition
The CNN was deployed as a binary classifier, and 14,509 quasar spectra were evaluated in this study, with each spectrum being scored between 0 and 1. Spectra with scores above a threshold of 0.5 were classified as CI absorption line candidates, and a total of 2,056 candidates were selected for further analysis.
Manual inspection and line verification
The CI absorption lines were further validated by manual inspection, focusing on their precise wavelengths and differences from neighboring absorption features. When a CI line was well located, but its dual counterparts deviated significantly, these were also excluded.Finally, the candidate samples were reduced to 400.
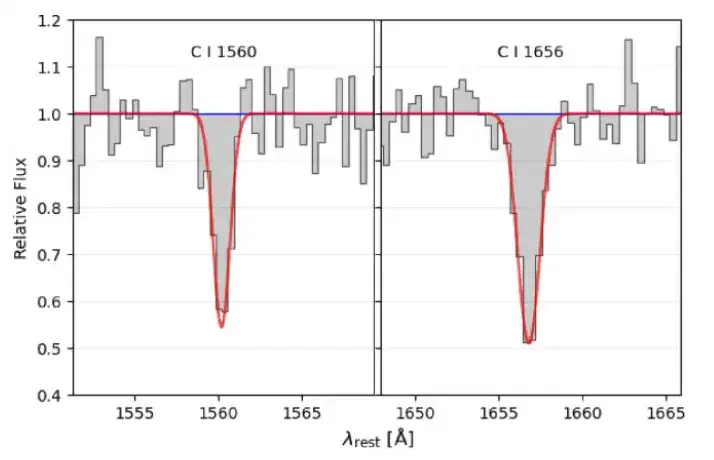
Detailed spectral line fitting and signal-to-noise ratio calculation
The study used a one-dimensional Gaussian model to fit the candidate CI absorption lines. Two key criteria are used here: first, although the static equivalent width W of λ1656 should be greater than λ1560, as long as λ1560 remains within the 3σ confidence interval, W(λ1560) is allowed to exceed W(λ1656); second, the minimum acceptable signal-to-noise ratios of λ1560 and λ1656 are 2.5 and 3, respectively. According to these criteria,The number of candidate samples was narrowed down to 142.
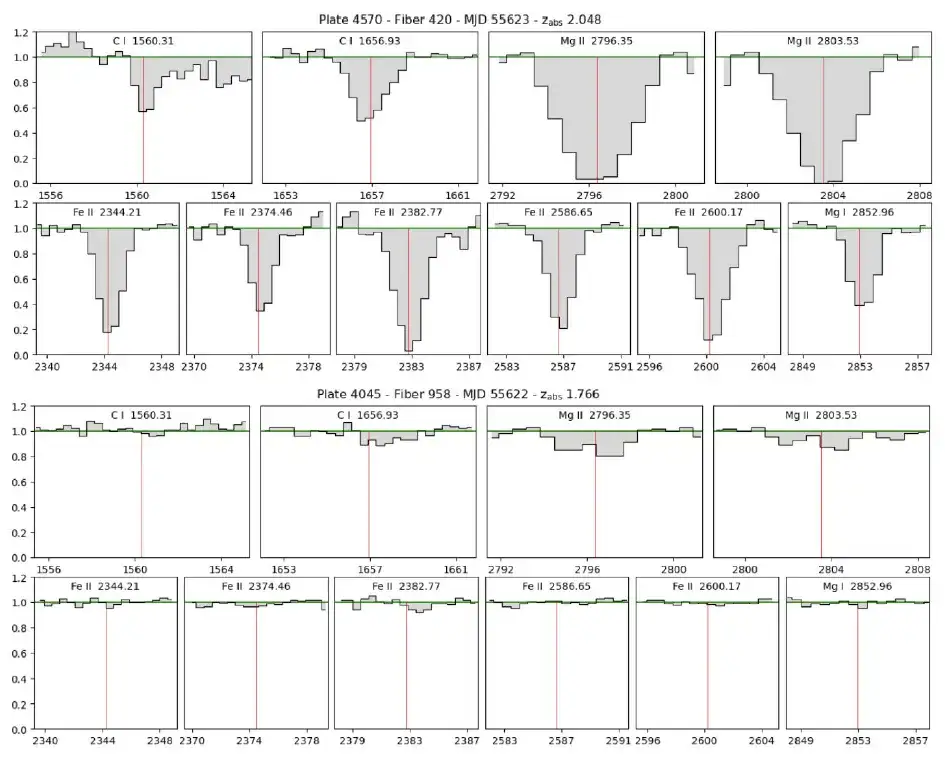
Visual inspection and spectral line cross-referencing
Each remaining candidate material was subjected to a final visual inspection, particularly for additional spectral lines when they matched the relative intensities of the CI line. Candidates where the CI line was prominent but all other spectral lines were absent were excluded from the study.The final candidate sample was narrowed down to a final list of 107 carbon absorbers tested.The table below shows some of the CI absorption lines.
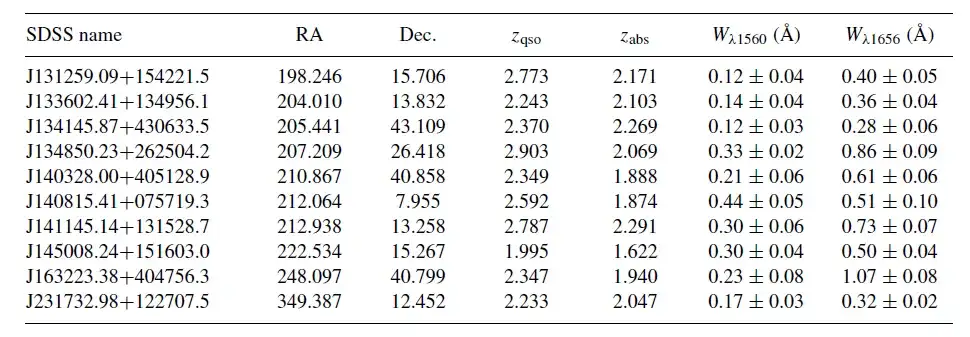
This study lists 10 carbon absorbers in the final catalog, with detailed information including target name, coordinates, redshift and static equivalent width. The results show that the strongest carbon absorber W(λ1656) is 1.92 Å, while the weakest carbon absorber has a static equivalent width of 0.1 Å. At the same time, the CNN training method enables the overall CI absorption lines to achieve lower equivalent widths and can detect CI absorption lines at lower redshifts.
The study also showed that the CNN method can be effectively used to search for two weak carbon absorption lines with broad wavelengths. Considering that many other lines in quasar spectra or other lines with similar continuous spectra (such as stellar spectra) are widely separated from each other and are important in various studies, the method can be used to search for any combination of absorption or emission lines.
AI applications in astronomy help humans reach the stars
In fact, Professor Ge Jian’s latest research has only uncovered the tip of the iceberg of AI technology applications in astronomy. As astronomy continues to develop, the challenges people face are becoming increasingly complex, from massive data management to precise navigation of deep space exploration to detailed research on distant galaxies, all of which require solutions that go beyond traditional methods.
The introduction of AI technology can not only process and analyze huge data sets generated by astronomical observations, but also play a key role in pattern recognition, predictive modeling and automated observations, greatly expanding the boundaries of our understanding of the universe.
In the past few years, researchers have begun to use AI to understand the universe more and more. In 2022, computer scientists from the U.S. Department of Energy's Argonne National Laboratory collaborated with institutions such as the University of Chicago, the University of Illinois at Urbana-Champaign, NVIDIA, and IBM to combine AI and supercomputers toA month's worth of data was processed in less than 7 minutes, and 4 gravitational wave signals generated by black hole mergers were identified.
In 2023,Musk officially established xA company, whose goal is to understand the true nature of the universe.Musk once said in an interview, "In a sense, an artificial intelligence that cares about understanding the universe is unlikely to exterminate humanity because we are an interesting part of the universe." In May of this year, xAI received more than US$6 billion in Series B financing, which also made the valuation of the company, which was established less than 10 months ago, reach approximately US$18 billion.
April 2024The Artificial Intelligence Working Group of the National Astronomical Observatory of the Chinese Academy of Sciences released a new generation of astronomical model "Star Language 3.0".Built on the Tongyi Qianwen open source model, it has been successfully connected to the Mini Sitian telescope array at the Xinglong Observatory of the National Astronomical Observatory. This is a classic example of the implementation of large models in the scientific field, and it is also the first application of large models in the field of astronomical observation.
In the vast universe, the unknown seems to always outweigh the known, but AI exploration has already begun to show its prowess. We have reason to believe that as technology continues to mature, AI will reveal more mysteries about the universe in the future, help humans understand the universe we live in more deeply, and lead us to the stars.