Don't Worry About Space Objects "colliding With the Earth"! The Chinese Academy of Sciences Team Proposed a New Method to Identify Coronal Mass Ejections, Which Is Close to Human Identification Results
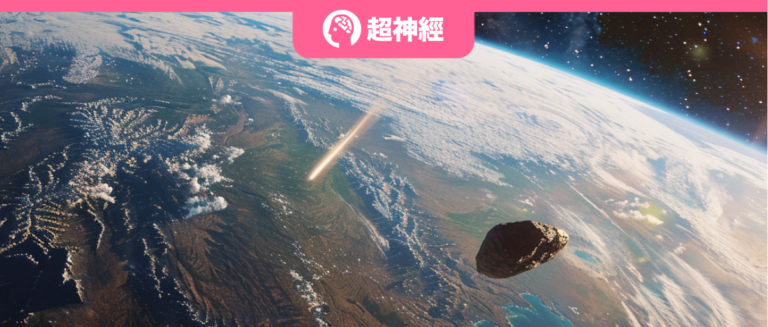
Coronal mass ejections (CMEs) are large-scale plasma masses ejected from the sun into interplanetary space. They are a form of energy released by the sun and one of the main factors affecting space weather. Large coronal mass ejections can affect communications, navigation, aviation activities, power grid operations, etc. In order to avoid security threats and asset losses,Accurately determine whether a coronal mass ejection will "hit" or "miss" the Earth, and predict the arrival time of a coronal mass ejection.The relevant research is of vital importance.
Based on the data from the LASCO coronagraph on the Solar and Heliospheric Observatory (SOHO) satellite, researchers at NASA's CDAW Data Center manually compiled a catalog of CME observations from 1996 to the present. The catalog records the time, position angle, angular width, speed and other physical quantities of each CME event, providing important basic data for CME-related research. However, in the face of massive amounts of data, manual identification is time-consuming and laborious, and automatic identification of CMEs has become a more active research direction in this field.
A research team from the Key Laboratory of Solar Activity and Space Weather, National Space Science Center, Chinese Academy of Sciences, published a paper titled "An Algorithm for the Determination of Coronal Mass Ejection Kinematic Parameters Based on Machine Learning" in The Astrophysical Journal Supplemental Series. The paper proposed a CME identification and parameter acquisition method based on machine learning. Compared with the manual CME catalog and some classic automatic identification methods, this method is efficient and fast, can identify relatively weak CME signals, and provide accurate CME morphology information.
Research highlights:
* The CME identification and parameter automatic acquisition method based on machine learning is highly efficient and fast, and can identify relatively weak CME signals.
* The parameters obtained by this method are close to the results of manual recognition by humans
* The CME structure detected by this method can also be used in other work such as CME arrival time prediction and CME 3D reconstruction.

Paper address:
https://iopscience.iop.org/article/10.3847/1538-4365/ad2dea
Follow the official account and reply "coronal mass ejection" to get the full PDF
Dataset: Accurately classify images
The researchers obtained CME-related event records (including CME appearance, end time and notes) from the CDAW CME catalog website, and downloaded daily differential images of LASCO C2 and C3 coronagraphs from 2013 to 2018. The selected time range covers half of the 24th solar cycle, which can provide representative and balanced CME and non-CME samples from the solar maximum to the solar minimum. In addition, the data for the rest of the 24th solar cycle were retained for verification.
The researchers chose the NASA/CDAW website as the data source because the data it provided met the needs of training CNN models for image classification and further tracking CME characteristics.
Based on the records of each CME event, the researchers performed iterations on each image:If the exposure time of an image is between the appearance time and the end time of a recorded entry, the image is marked with the corresponding note of the entry; conversely, if the exposure time of an image does not match any record, the image is marked as “No-CME”.
Research Methodology: Three Steps to Confirm Core Parameters
The CME identification and parameter acquisition method based on machine learning is divided into three steps:

first,After the image was correctly classified, the researchers extracted the convolutional feature map from the last convolutional layer of the neural network and applied the PCA algorithm (principal component analysis) to the feature map to obtain information about the same object. They then used the Otsu algorithm (an algorithm for determining the image binary segmentation threshold) and morphological operations to obtain accurate CME pixel labels.
Secondly, scan each frame of the image sequence and use a trajectory matching algorithm to track the propagation trajectory of the CME leaving the Sun in the coronagraph field of view.

at last,Based on the trajectory obtained in the previous step, the kinematic parameters of the CME, such as velocity, CPA (central position angle) and AW (angular width), are derived.
Research results: The CME identification and parameter automatic acquisition method based on machine learning is efficient and fast
To evaluate the performance of the method in real observations, the researchers selected representative events with different velocities and AW from 2010 to 2012 and analyzed them in ascending order of AW. The AW of the selected CMEs ranged from 78° to 360° and the velocity ranged from 288 to 1205 km s-1 At the same time, the researchers compared the results of their newly proposed method with other classic CME automatic tracking catalogs (namely CACTus, CORIMP and SEEDS).
Event 1: The CME event that occurred on February 14, 2012.
The CME event was launched from the west side of the coronagraph field of view on February 14, 2012. The following figures show the detection images of CACTus, CORIMP, SEEDS and the method proposed in this study from top to bottom.

In the first three rows of the above figure, the detected CME regions are shown in different colors and symbols. In the detection map of CACTus, the detected CME region is limited by the white straight line. In the detection map of CORIMP, the red dots indicate the trajectory of the strongest outermost front, and the yellow dots indicate the overall detected structure. In the detection map of SEEDS, the blue dots indicate the location of the leading edge, and the red dots indicate the approximate outline of the leading edge created using the segmentation technique. In the last row, the method proposed in this study, the blue in the figure indicates irrelevant background, while the warmer color indicates that the pixel is more likely to be part of the CME.
In the northwest quadrant, the results of this study showed brighter lines and spots.These are small and faint traces of transient eruptions that can only be detected using the method proposed in this study, demonstrating its ability to detect small and weak signals.
Event 2: The CME event that occurred on January 15, 2012.
The researchers selected and displayed several frames from the detection results of CACTus, CORIMP, SEEDS and the newly proposed method from top to bottom for comparison.

All methods detected the main body of the CME.However, the method proposed in this study discovered weaker or smaller CME features besides the main body, while other methods failed to detect them.From the above figure, we can see that the proposed method successfully separates the CME region from the background.
Event 3: The CME event that occurred on March 8, 2011.
This CME event occurred on March 8, 2011. The detection graphs of CACTus, CORIMP, SEEDS and the method proposed in this study are shown below:

Among these methods, the method proposed in this study and CORIMP were the first to detect the CME at 04:00, while the other methods identified the CME after 12 minutes.
Based on the above results, we can conclude that compared with the CME manual catalog and some classic automatic identification methods,The CME identification and automatic parameter acquisition method based on machine learning proposed in this study is highly efficient and fast, and can identify relatively weak CME signals and provide accurate CME morphology information.The tracking method is relatively intuitive, and the parameters obtained are close to the results of manual recognition by humans. In addition, the CME structure detected by this method can also be used in other work such as CME arrival time prediction and CME three-dimensional reconstruction.
Artificial intelligence saves overburdened astronomers
In recent years, with the advancement of technology, a huge amount of data has been generated in astrophysics research. For example, in addition to the CME-related research mentioned above, the famous Sloan Digital Sky Survey began in 2000 and observed about 3 million celestial bodies, with a data volume of about 40 TB. The Dark Energy Survey, which is currently in operation, has released 80 TB of scientific-grade spectral data in its first batch, which is almost equivalent to the data volume of the entire project of the previous completed sky survey observations.
For astronomers to find valuable information for research from the massive data obtained from large-scale comprehensive survey telescopes or observatories is like fishing for stars in the universe. How to efficiently process this data has become an important challenge facing modern astronomy. Due to the outstanding advantages of artificial intelligence in the analysis and processing of massive data, it has naturally entered the field of vision of astronomers and become a powerful assistant to help them "reduce the burden".
As early as 2017,NASA announced that the neural network developed by Google's artificial intelligence team has discovered two new exoplanets.One of the planets is numbered "Kepler-90i", and the star system it is in has 8 planets, the same number of planets as the solar system. However, Kepler-90i is 30% larger than the Earth and is a super-Earth.
At the 2021 World Artificial Intelligence Conference,Ma Huateng, Chairman of Tencent, revealed that Tencent will jointly launch the "Star Exploration Project" with the National Astronomical Observatory.Using AI technology to search for pulsars and explore the universe. Based on the leading computer vision technology of Tencent Youtu Lab and the computing power of Tencent Cloud, the two parties will use "cloud + AI" to help China's Sky Eye FAST process the huge amount of data received every day, and find clues to pulsars through visual AI analysis, assisting the search for pulsars in fast radio bursts and close binary star systems.
June 2022Portuguese researchers have developed the artificial intelligence software SHEEP to automatically identify whether the observed target is a star, galaxy, quasar, supernova or nebula.Unlike other pattern recognition software, SHEEP first calculates redshift data as an additional feature of the classification pattern, and then classifies the cataloged celestial bodies by combining the observed images with the coordinate data. The relevant results were published in the journal Astronomy & Astrophysics.
Nowadays, more and more researchers are using AI technology as a powerful exploration tool to provide rich and complex data, classify galaxies, screen data to obtain signals, discover pulsars, identify unusual exoplanets, etc., thereby promoting innovation in the field of astronomy. There is no doubt that AI will stimulate new vitality and create new possibilities.
References:
1.https://iopscience.iop.org/article/10.3847/1538-4365/ad2dea
2.https://mp.weixin.qq.com/s/7frn8XvRJ-QWHCFwtsixzw
3.http://www.stdaily.com/index/kejixinwen/202207/d42b187caded4c7db61582566d7a6ce6.shtml
4.https://www.most.gov.cn/gnwkjdt/202206/t20220624_181299.html