New Achievement of Fudan Institute of Brain Science: Pianno, a Spatial Transcriptome Semantic Annotation Tool, Was Developed Based on Semantic Segmentation
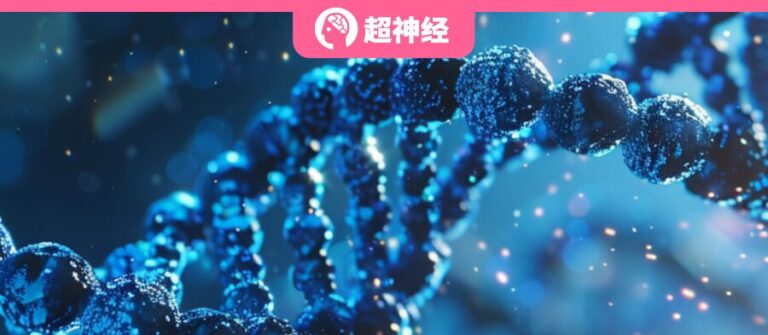
Since being selected as the Technology of the Year by Nature Methods in 2020,"Spatial transcriptomics" has become one of the hottest revolutionary technologies in the field of life sciences today.Simply put, this technology can obtain tissue spatial information and transcriptome data, accurately analyze gene expression patterns within tissues, as well as biological characteristics such as the spatial position relationship of cell populations from the time and space dimensions. It is of great value in research in the fields of disease research, growth and development, organ structure, and species evolution.
As spatial transcriptomics continues to gain popularity in the academic research field, spatial transcriptomics technologies such as 10x Visium, Slide-seq, and Stereo-seq have emerged. These latest achievements and progress are completely changing human research on gene expression patterns in tissues. However, simply obtaining the gene expression profile of specific physical coordinates in a tissue cannot fully understand the complexity of the biological system. To understand its reason, it is necessary to identify the biological identity of each spatial point in the tissue.
Currently, machine learning-based methods have been widely used to identify clusters of spatial points and interpret their biological identities using marker genes.However, these methods are often limited by their lack of ability to make explicit connections to known structures within the cluster.In addition, manual annotation is often used to assist in identifying known structures, but this method is often limited by the researchers' expertise and subjective judgment and cannot be applied to large-scale analysis.
In response to the above challenges, Zhu Ying's team from the Institute of Brain Science at Fudan University recently published a research result titled "Pianno: a probabilistic framework automating semantic annotation for spatial transcriptomics" in "Nature Communications".The research team borrowed the idea of "semantic segmentation" from computer vision, proposed the concept of "spatial transcriptome semantic annotation", and developed the spatial transcriptome semantic annotation tool Pianno.The ability to automatically define structures or cell types for spatial points within tissues can combine information from multiple dimensions to enhance the interpretation of complex biological systems.
Research highlights:
* Pianno has a unique automatic labeling mode that is applicable to data generated by various spatial transcriptomics technologies
* Pianno demonstrates superior performance compared to state-of-the-art spatial clustering methods, providing new insights into spatial transcriptomics data

Paper address:
https://doi.org/10.1038/s41467-024-47152-4
Dataset: Public data, rigorous calculations
The datasets used in this study are mainly public datasets from different spatial technology platforms, including the human dorsolateral prefrontal cortex dataset dlPFC, the adult mouse cerebral hemisphere coronal section dataset Stereo-seq, the mouse hippocampus preprocessing dataset Slide-seqV2, the human pancreatic ductal adenocarcinoma dataset ST, the human breast cancer dataset Visium, the mouse primary visual cortex dataset scRNA-seq, the snRNA-seq datasets of multiple human cortical regions, and the DAPI staining images of the mouse olfactory bulb.
In the study, in order to avoid the destruction of the original biological features by image processing technologies such as noise reduction, smoothing, and sharpening,The research team built a Bayesian classifier based on the raw counts to fine-tune the initial annotations.At the same time, the research team applied a high-order Markov random field (MRF) prior model. In the context of spatial transcriptomics, since the gene expression and spatial position of each site must be considered together, the research team also adopted the spatial Poisson point process (sPPP) model.
Pianno: Innovative new tool for automated spatial transcriptome semantic annotation
The research team proposed a new tool called Pianno based on the Bayesian framework.The tool combines Markov random fields (MRFs) with spatial Poisson point processes (sPPPs), making full use of the ability of sPPPs to model the distribution of RNA-seq count data while taking into account the location information of spatial points. It can automatically annotate the biological identity of each point in spatial transcriptome data using a predefined list of marker genes.

The spatial transcriptome data input by Pianno consists of spatial coordinates, initial marker gene list and raw gene counts.Each pattern provides at least one known token.
The annotation process consists of an initial segmentation step and a refinement step:
In the initial segmentation step,The spatial expression of each gene is converted into a grayscale image. For each target pattern, a pattern image is created by aggregating the grayscale images of marker genes associated with the pattern, and then additional candidate marker genes for each pattern are determined to update the initial marker list. The updated marker list will be integrated into the subsequent refinement step, taking into account their unique expression patterns in the initially annotated structure.
In the refinement step,A Bayesian classifier is constructed to evaluate the posterior probability that each spatial point belongs to different modes, and then the annotation is updated based on the posterior probability.
Pianno provides two methods for updating annotations:
* For continuous patterns in semantic annotation, it is recommended to use the probability distribution as a pattern image and return it to the pattern detector for updating the annotation; * For scattered or sharp image patterns, it is recommended to update the label directly based on the probability value because it can retain detailed information.
In general,Pianno simplified the annotation process and adopted a heuristic approach to use an initial single marker gene to identify additional marker genes, which can minimize the input of the number of known markers.
Research results: excellent performance and strong applicability
In this study, the research team verified the performance, accuracy, and adaptability of Pianno, and further demonstrated Pianno's capabilities by comparing it with existing methods.
In the comparison with clustering-based tools for anatomic structure annotation, the research team evaluated the performance of Pianno using 12 samples from the dlPFC dataset and compared it with CellAssign, another annotation method based on markers but without spatial information. In addition, the unsupervised clustering method Leiden algorithm and 5 spatial clustering methods (SpaGCN, SEDR, BayesSpace, DeepST and STAGATE) were also considered in the evaluation process.

The assessment found thatPianno's performance achieved the highest agreement with manual annotations by experienced researchers based on morphological features and markers.Eleven out of the 12 samples outperformed the other test methods.

In addition, the research team further comprehensively evaluated the superior performance of Pianno through other classification indicators, such as accuracy (ACC), macro-averaging precision (P), macro-averaging recall (R), macro-average F1 score (F1) and normalized mutual information (NMI), as shown in Figure e above.Pianno-related indicators are all at a high level.

The team then evaluated Pianno's ability to predict the spatial distribution of cell types. In this round of validation, the team used a Stereo-seq dataset of coronal sections of adult mouse hemibrains and compared the results with the cell type distribution inferred by different strategies, including unsupervised clustering after cell segmentation, and three spatial deconvolution tools based on the integration of spatial and single-cell transcriptomics.
The study found that Pianno's predictions of the distribution of excitatory neuron subtypes showed patterns comparable to Tangram and RCTD, and were highly consistent with their known locations in each layer.These results demonstrate the robustness and accuracy of Pianno in predicting complex cell type distributions in spatial datasets, especially in situations where unsupervised methods face challenges.
The research team then further evaluated Pianno's performance in annotating various shape structures in spatial transcriptome data on different platforms and compared it with STAGATE.

The research team used Pianno to annotate the anatomical structures in the Stereo-seq dataset of the mouse olfactory bulb, which contains 10,747 spatial points covering both tissue-covered and background areas.
Pianno was able to perform background subtraction and structure annotation simultaneously within a few minutes. In contrast, when the number of clusters was set to the number of structures, STAGATE was unable to identify clusters corresponding to all anatomical structures.
The research team also evaluated Pianno's performance in annotating complex and dispersed structural tissues in view of the high heterogeneity of the tumor microenvironment. This round of testing analyzed the microenvironment of two human pancreatic ductal adenocarcinoma samples and two breast cancer samples.

Overall,Pianno showed a level of consistency with manual annotation by professional pathologists, demonstrating its great potential in annotating irregular and complex structures, especially in heterogeneous tumor microenvironments.This provides valuable assistance to pathologists in understanding the complexity of tumor biology and is expected to provide new ideas for providing personalized treatment strategies.
Combining artificial intelligence with complex biology has great potential
According to the Institute of Brain Science of Fudan University, the research project has been funded by the key project of the National Key R&D Program "Biological and Information Integration (BT and IT Integration)", the major project of Science and Technology Innovation 2030 - "Brain Science and Brain-like Research", the National Natural Science Foundation, the Shanghai Science and Technology Major Project and the Zhangjiang Laboratory.
It is understood that the Fudan University Brain Science Institute was established in April 2006. It is a school-wide neuroscience research entity of Fudan University and one of the key scientific and technological innovation platforms built in the second phase of the Ministry of Education's "985 Project". It is a "two-in-one" construction project with the National Key Laboratory of Medical Neurobiology.
Since its establishment, the Institute of Brain Science of Fudan University has achieved fruitful results. The institute has repeatedly responded to major international and national needs, undertaken major scientific research projects, and produced important research results. According to its official website, researchers at the institute have presided over and participated in a series of major scientific research projects, including the Ministry of Science and Technology's "973 Program", "863 Program", Science and Technology Innovation 2030 "Brain Science and Brain-like Research", the National Key R&D Program, the National Science and Technology Major Project "Major New Drug Creation", etc.
In fact, in addition to the Institute of Brain Science at Fudan University, many laboratories and companies have also begun to pay attention to spatial transcriptome technology.
For example,Zhang Shihua's team from the Institute of Mathematics and Systems Science, Chinese Academy of Sciences, developed the STA-series of tools. In 2022, the team released STAGATE, an artificial intelligence tool for identifying spatial substructures of biological tissues that is suitable for different spatial transcriptome technologies and different biological tissues. After entering 2023, the team has released a number of results around spatial transcriptome technology - * A new integrated analysis tool STAligner was established for multi-slice spatial transcriptome data of biological tissues from different technologies, different developmental time points, and different disease conditions. * STAMarker, a spatial domain-specific variable gene identification method based on deep learning saliency maps, simultaneously realizes spatial domain identification and corresponding spatial variable gene identification, and is expected to provide an effective method for fine-grained analysis of spatial transcriptome data. * In collaboration with the teams of Yang Yungui and Cai Jun from the Beijing Institute of Genomics, Chinese Academy of Sciences (National Center for Bioinformatics), the three-dimensional spatial transcriptome map STAPR of Mediterranean planarians during regeneration was mapped, and multiple key regulatory factors for regeneration were systematically identified.

Professor Zhang Xiaofei's research group at the School of Mathematics and Statistics of Central China Normal University has developed a computational method called ENGEP.Using k-nearest neighbor weighted regression and ensemble learning strategies, the expression of unmeasured genes in the spatial transcriptome can be accurately predicted. In addition, ENGEP can also accurately predict the expression patterns of unmeasured genes in space, which is of great significance for enhancing spatial transcriptomics data.
There is no doubt that AI's empowerment in spatial transcriptomics and even biology has not only improved research efficiency, but also provided new solutions to scientific research difficulties. As pointed out in the discussion section of the paper, the value brought by Pianno - it may replace the existing labor-intensive manual annotation, and provide efficient, accurate and low-cost forms in an automated way to bring changes to spatial transcriptomics, and will also promote new developments in biology.
References:
1. https://news.fudan.edu.cn/2024/0407/c2474a139894/page.htm
2. https://bfse.cas.cn/sxyqyjc/kyjz/202311/t20231110_4985132.html
3. https://kjc.ccnu.edu.cn/info/1009/3744.htm