Google Releases HEAL Framework, 4 Steps to Evaluate Whether Medical AI Tools Are Fair
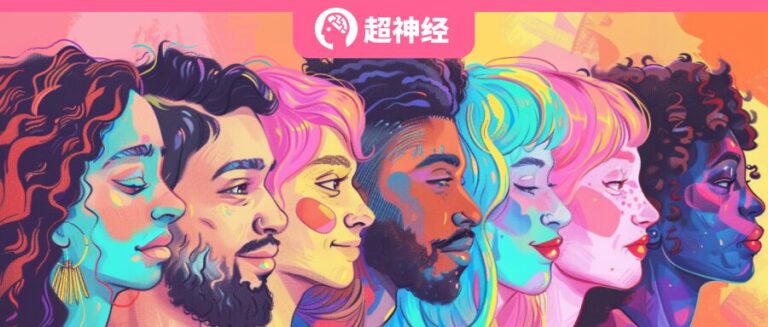
If you imagine maintaining a healthy state as a race, not everyone can start from the same starting line. Some people can run the entire course smoothly, and some people can get help immediately even if they fall. However, some people may face more obstacles due to their economic conditions, place of residence, education level, race or other factors.
"Health equity" means that everyone should have equal access to health care resources so that they can complete this race more calmly and achieve optimal health.Some groups (such as ethnic minorities, people of low socioeconomic status, or individuals with limited access to healthcare) are unfairly treated in terms of disease prevention, diagnosis, and treatment, which greatly affects their quality of life and chances of survival. There is no doubt that increasing attention to "health equity" should become a consensus worldwide to further address the root causes of inequality.
Nowadays, although machine learning and deep learning have made some achievements in the field of medical health, and have even stepped out of the laboratory and into the clinical frontline, while people marvel at the powerful capabilities of AI, they should pay more attention to whether the implementation of this type of emerging technology will exacerbate the inequality of health resources.
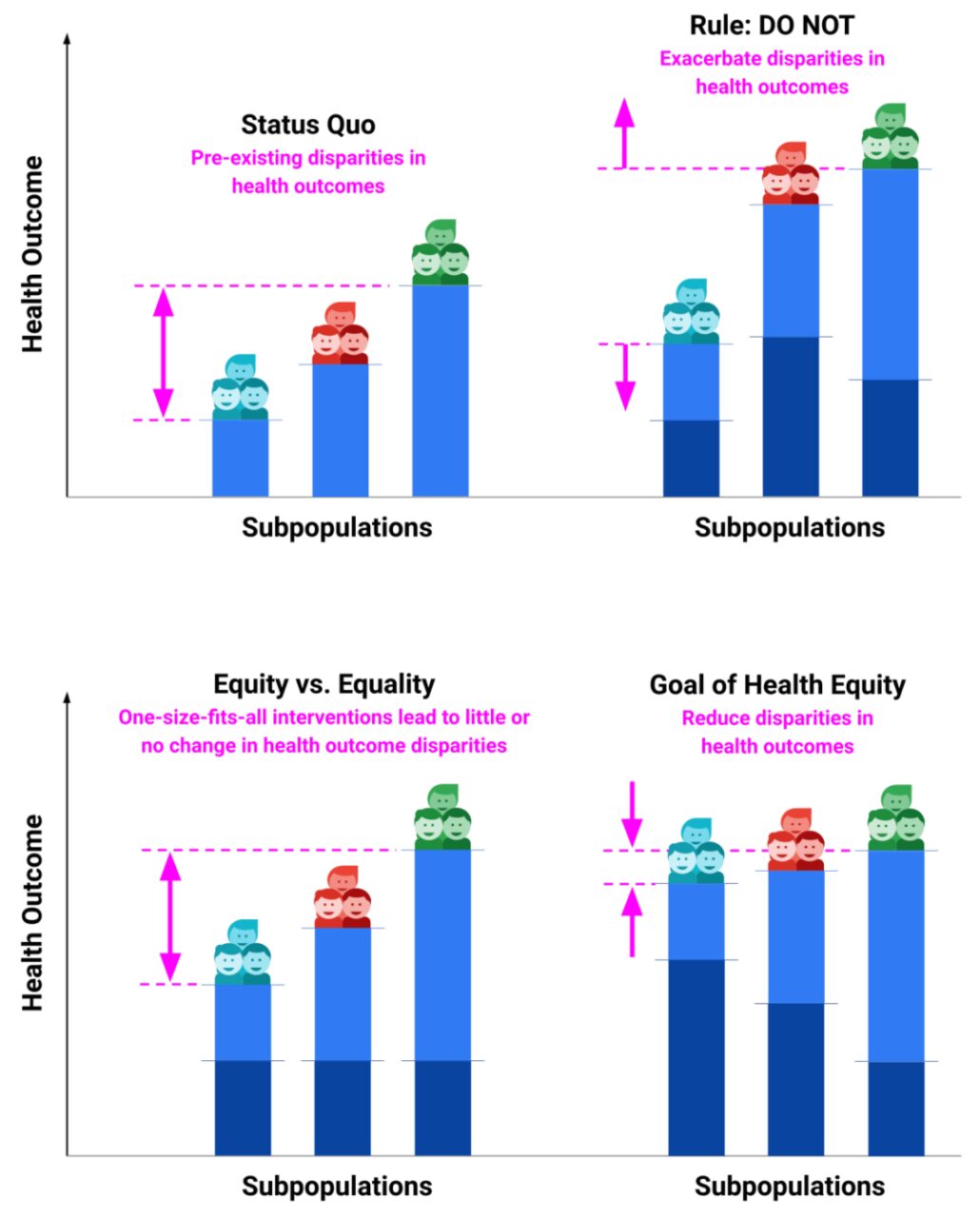
*Light blue bars indicate pre-existing health outcomes
* Dark blue bars illustrate the impact of interventions on pre-existing health outcomes
To this end, the Google team developed the HEAL (The health equity framework) framework, which can quantitatively evaluate whether machine learning-based healthcare solutions are "fair."Through this approach, the research team seeks to ensure that emerging health technologies are effectively reducing health inequalities, rather than inadvertently exacerbating them.
HEAL framework: 4 steps to evaluate fairness of AI tools in dermatology
The HEAL framework consists of 4 steps:
- Identify factors associated with health inequities and define AI tool performance metrics
- Identify and quantify pre-existing health disparities
- AI tool performance testing
- Measuring the potential of AI tools to prioritize health equity disparities

Step 1: Identify factors associated with health inequities in dermatology and identify metrics to evaluate the performance of AI tools
The researchers selected the following factors - age, sex, race/ethnicity, and Fitzpatrick skin type (FST) - through a literature review and consideration of data availability.
FST is a system for classifying human skin based on its response to ultraviolet (UV) radiation, particularly sunburn and tanning. Ranging from FST I to FST VI, each type represents a different level of melanin production in the skin, eyes and hair, as well as sensitivity to UV rays.
In addition, the researchers chose top-3 agreement as a metric to evaluate the performance of the AI tool, which is defined as the proportion of cases in which at least one of the top three conditions suggested by the AI matched the reference diagnosis of the dermatology expert panel.
Step 2: Identify existing “health disparities” in dermatology
Health disparities indicators are specific measures that quantify and describe inequalities in health status between groups based on race, economic status, geographic location, sex, age, disability, or other social determinants.
Here are some common health disparities indicators:
Disability-adjusted life years (DALYs)DALY: reflects the number of years of healthy life lost due to illness, disability or premature death. DALY is a comprehensive indicator, which is the sum of years of potential life lost (YLLs) and years lived with disability (YLDs).
Years of life lost (YLLs): Expected number of healthy years lost due to premature death.
The researchers also conducted a sub-analysis of skin cancer to understand how the performance of the AI tool varied across high-risk conditions. The study used the Global Burden of Disease (GBD) categories of "non-melanoma skin cancer" and "malignant cutaneous melanoma" to estimate health outcomes for all cancers, and the "cutaneous and subcutaneous diseases" category for all non-cancer conditions.
Step 3: Measure the performance of AI tools
Top-3 agreement was measured by comparing the AI-predicted ranked pathologies with reference diagnoses on an evaluation dataset (subpopulations stratified by age, sex, race/ethnicity, and eFST).
Step 4: Testing the performance of AI tools in accounting for health disparities
To quantify the HEAL metric of the skin disease AI tool, the specific method is as follows:
For each subpopulation, two inputs are required:Quantitative measures of pre-existing health disparities and AI tool performance.
Calculate the inverse correlation R between health outcomes and AI performance across all subgroups for a given inequality factor (e.g., race/ethnicity). The larger the positive value of R, the more comprehensive the consideration of health equity.
The HEAL metric of the AI tool is defined as: p(R > 0), which estimates the probability that the AI prioritizes pre-existing health disparities through the R distribution of 9,999 samples. A HEAL index of more than 50% means a higher probability of achieving health equity, while a HEAL index of less than 50% means a lower probability of achieving equitable performance.
Dermatology AI tool review: Some subgroups still need improvement
Race/ethnicity: The HEAL indicator is 80.5%, indicating a high priority for addressing health disparities that exist among these subgroups.
Gender: The HEAL indicator is 92.1%, indicating that gender has a high priority in considering health differences in AI tool performance.
Age: The HEAL index is 0.0%, indicating a low likelihood of prioritizing health disparities across age groups. For cancer conditions, the HEAL index is 73.8%, while the HEAL index for non-cancer conditions is 0.0%.

The researchers performed a logistic regression analysis that showed that age and certain dermatological conditions (such as basal cell carcinoma and squamous cell carcinoma) had a significant impact on the AI's performance, while other conditions (such as cysts) were less accurate.
In addition, the researchers conducted an intersectional analysis, using a segmented GBD health outcome measurement tool to expand HEAL analysis across age, gender, and race/ethnicity, with an overall HEAL index of 17.0%.Focusing specifically on the intersection of low rankings in health outcomes and AI performance, we identified subgroups in need of improved AI tool performance, including Hispanic women aged 50+, Black women aged 50+, White women aged 50+, White men aged 20-49, and Asian Pacific American men aged 50+.
That said, improving the performance of AI tools for these groups is critical to achieving health equity.
More than just health equity: The broader picture of AI fairness
It is obvious that health inequality exists significantly among different racial/ethnic, gender and age groups, especially with the rapid development of high-tech medical technology, the imbalance of health resources has even intensified. AI has a long way to go in solving related problems. But it is worth noting that the unfairness brought about by technological progress is actually widespread in all aspects of people's lives, such as the inequality in information access, online education and digital services caused by the digital divide.
Jeff Dean, head of Google AI and a "programmer god", once said that Google attaches great importance to AI fairness and has done a lot of work in data, algorithms, communication analysis, model interpretability, cultural differences research, and large model privacy protection. For example: * In 2019, Google Cloud's Responsible AI Product Review Committee and Google Cloud's Responsible AI Transaction Review Committee suspended the development of credit-related AI products to avoid aggravating algorithmic unfairness or bias. * In 2021, the Advanced Technology Review Committee reviewed research involving large language models and believed that it could continue with caution, but this model could not be officially launched before a comprehensive review of AI principles was conducted. * The Google DeepMind team once published a paper exploring "how to integrate human values into AI systems" and integrate philosophical ideas into AI to help it establish social fairness.
In the future, in order to ensure the fairness of AI technology, intervention and governance will be needed from multiple angles, such as:
* Fair data collection and processing:Ensure that the training data covers diversity, including people of different genders, ages, races, cultures, and socioeconomic backgrounds. At the same time, avoid data selection due to bias and ensure that the data set is representative and balanced.
* Eliminating algorithmic bias:During the model design phase, proactively identify and eliminate algorithmic bias that could lead to unfair outcomes. This may involve careful selection of input features to the model or using specific techniques to reduce or eliminate bias.
* Fairness Assessment:Fairness evaluation should be performed before and after model deployment. This includes using various fairness metrics to assess the impact of the model on different groups and making necessary adjustments based on the evaluation results.
* Continuous monitoring and iterative improvement:After the AI system is deployed, its performance in the actual environment should be continuously monitored to promptly identify and address any possible unfairness. This may require regular iteration of the model to adapt to environmental changes and new social norms.
As AI technology develops, relevant ethical standards and laws and regulations will be further improved to allow AI technology to develop within a fairer framework. At the same time, more attention will be paid to diversity and inclusiveness. This requires considering the needs and characteristics of different groups in every link, such as data collection, algorithm design, and product development.
In the long run, the true meaning of AI changing lives should be to better serve people of different genders, ages, races, cultures, and socioeconomic backgrounds, and reduce unfairness caused by the application of technology. As public awareness continues to improve, can more people participate in the planning of AI development and make suggestions on the development of AI technology to ensure that the development of technology is in line with the overall interests of society.
The grand blueprint for fairness in AI technology requires joint efforts from multiple fields such as technology, society, and law. We must ensure that advanced technology does not become a driver of the "Matthew effect."