The Latest Insights From Fei-Fei Li’s AI4S Team: 16 Innovative Technologies, Covering Biology/materials/medical Care/diagnosis…
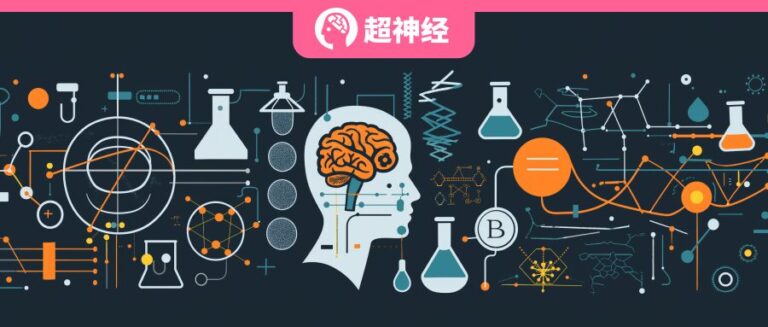
Not long ago,Stanford University's Human-Center Artificial Intelligence (HAI) Research Center has released the "2024 Artificial Intelligence Index Report".As the seventh masterpiece of Stanford HAI, this 502-page report comprehensively tracks the development trends of global artificial intelligence in 2023. Compared with previous years, the research scope has been expanded to cover basic trends such as AI technology, public perception of AI technology, and political dynamics surrounding its development, and predict future AI development trends.

Philosopher John Etchemend (left) co-leads
The most eye-catching part of this report is the newly added chapter:Explore the profound impact of artificial intelligence in science and medicine.The report shows the brilliant achievements of AI in the scientific field in 2023, as well as the important innovative results of AI in the medical field, including breakthrough technologies such as SynthSR and ImmunoSEIRA. In addition, the report also carefully analyzes the trend of FDA approval of AI medical devices, providing valuable reference for the industry.
Follow the official account and reply "HAI2024" to download the full report
AI: An engine for accelerating scientific research
The 2024 Artificial Intelligence Index Report states thatIn 2023, the industry produced 51 well-known machine learning models, while academia contributed only 15. In addition, 108 newly released basic models came from industry and 28 from academia.
Although the pace of development in academia is significantly slower than that in industry, it is important to note that AI was not officially used in scientific discovery until 2022. From AlphaDev, which optimizes algorithm sorting efficiency, to GNoME, which revolutionizes the material discovery process, we have witnessed the emergence of more important and relevant AI applications.
Today, AI has blossomed in fields such as materials science, climate change, and computer science. Fortunately, China is leading the way in this round of change.According to the "China AI for Science Innovation Map Research Report" compiled by the Chinese Institute of Scientific and Technological Information and the Ministry of Science and Technology's Next Generation Artificial Intelligence Development Research Center, my country ranks first in the number of papers published in AI-driven scientific research, and domestically produced AI scientific research basic software is becoming increasingly mature, providing researchers with rich data sets, basic models and specialized tools.
In general, AI has a diverse range of applications in science and is driving the development and progress of science at an unprecedented rate. However, it should be noted that at the current stage of development of AI for Science,Problems such as shortage of comprehensive talents, difficulty in reusing technical solutions, and poor quality of research data in vertical disciplines have gradually been exposed.
For example, in the discussion around "Should AI talents engage in scientific research or should scientific research talents learn AI", researchers with interdisciplinary knowledge backgrounds stand out. They not only have a deep insight into their research field, but are also able to quickly master various AI tools and technologies. However, their scarcity is imaginable, and the cultivation of comprehensive talents is not something that can be achieved overnight. Therefore, how to quickly build a communication bridge between AI and scientific research is an important issue related to the large-scale promotion of AI for Science.
At the same time, there is no need to elaborate on the rich fields covered by scientific research. Different research groups may have different needs for AI tools due to slightly different research directions. When it is difficult for every team to have researchers with interdisciplinary backgrounds, lowering the threshold for using AI tools and simplifying the model fine-tuning process may also be able to accelerate the promotion of AI in the field of scientific research to a certain extent.
Accelerate updates, self-iteration and advancement of technology
The advancement of AI technology has promoted the breadth and depth of its application, while also placing higher and higher requirements on algorithms. At present, most algorithms have reached a stage where it is difficult to rely on human experts to further optimize them, resulting in the continuous aggravation of computing bottlenecks. However, scientists have never stopped exploring the field of algorithms.
AlphaDev
Recreating AlphaGo's Masterstroke
Sorting algorithms are fundamental tools for computer systems to arrange data items in an orderly manner. To achieve innovative breakthroughs in this field, Google DeepMind took an innovative approach and explored the relatively understudied field of computer assembly instructions.Through the AlphaDev system, DeepMind can directly find more efficient sorting algorithms from the CPU assembly instruction level.
The AlphaDev system consists of two core components: the learning algorithm and the representation function.
The learning algorithm is an extension of the advanced AlphaZero algorithm, combining deep reinforcement learning (DRL) and random search optimization algorithms to perform large-scale instruction search tasks; the representation function is based on the Transformer architecture, which can capture the underlying structure of the assembly language and convert it into a special sequence representation.
Using the AlphaDev system,DeepMind has successfully discovered fixed-length short sequence sorting algorithms, namely Sort 3, Sort 4, and Sort 5, that are superior to current manually tuned algorithms, and has integrated the relevant code into the LLVM standard C++ library.In particular, in discovering the Sort 3 algorithm, AlphaDev took a seemingly counterintuitive approach that was actually a shortcut, reminiscent of the “37th move” that AlphaGo used in its match against legendary Go player Lee Sedol—an unexpected strategy that ultimately led to victory.

The application scope of AlphaDev is not limited to sorting algorithms. DeepMind generalized its method and applied it to hash algorithms in the range of 9 to 16 bytes, achieving a significant speed increase of 30%. This shows that AlphaDev has broad potential and application value in optimizing underlying computing tasks.
Paper link:
https://www.nature.com/articles/s41586-023-06004-9
FlexiCubes
Generate high-quality 3D models using AI
From scene reconstruction to generative AI tracks, the new generation of AI models has achieved remarkable success in generating realistic and detailed 3D models. Since these models are usually created as standard triangular meshes, the quality of the mesh is crucial.Nvidia researchers have developed a new mesh generation method, FlexiCubes, which significantly improves the mesh quality in the 3D network generation pipeline and can be integrated with the physics engine to easily create flexible objects in 3D models.

The key idea of FlexiCubes is to introduce "flexible" parameters that allow precise adjustments in the process of generating the mesh.By updating these parameters during the optimization process, the quality of the mesh is greatly enhanced. This approach sets FlexiCubes apart from traditional mesh-based pipelines, such as the widely used Marching Cubes algorithm, allowing it to seamlessly replace optimization-based AI pipelines.
The high-quality meshes generated by FlexiCubes excel in representing complex details, enhancing the overall realism and fidelity of AI-generated 3D models. These meshes are particularly useful for physics simulations, making it possible for AI pipelines to accurately render details in complex shapes in fields such as photogrammetry and generative AI.
Paper link:
https://research.nvidia.com/labs/toronto-ai/flexicubes
Accelerate creation and improve efficiency beyond human effort
Synbot
AI-powered robot chemist
Deep in the depths of chemical laboratories, a revolution is quietly taking place - the synthesis of organic compounds is no longer a slow and tedious process, but is accelerated into reality through the magic of automation.At the heart of this transformation is the Synbot, an autonomous synthetic robot created by scientists at Samsung Electronics.

Specifically, Synbot consists of three layers:
* AI S/W layer:Lead the comprehensive planning process, equipped with retrosynthesis modules, experimental design and optimization modules, and use decision-making modules to guide the experimental direction;
* Robot S/W layer:Responsible for converting it into actionable commands for the robot through the recipe generation module and translation module;* Robot layer:Under the supervision of the online scheduling module, the various functions of the synthesis laboratory are modularized and the planned recipes are systematically executed, continuously updating the database until the predefined goals are achieved.
Research shows thatSynbot can perform an average of 12 reactions in 24 hours. Assuming that human researchers can perform two such experiments per day, Synbot is at least six times more efficient than its human counterparts.With the addition of Synbot, scientists are freed from tedious operations and can devote more energy to innovation and exploration.
Paper link:
https://www.science.org/doi/full/10.1126/sciadv.adj0461
GNoME
Reinventing the materials discovery process
Google DeepMind published an article in Nature saying,The AI tool GNoME (Graph Networks for Materials Exploration) based on material exploration has discovered 2.2 million new crystal predictions (equivalent to nearly 800 years of knowledge accumulated by human scientists), of which 380,000 are stable crystal structures.It is hoped that through experimental synthesis, some materials may trigger technological changes, such as the next generation of batteries and superconductors.

GNoME is an advanced graph neural network (GNN) model. The input data is mainly in the form of graphs, forming connections similar to those between atoms, which makes it easier for GNoME to discover new crystalline materials. It is reported that GNoME can predict the structure of new stable crystals, then test them through DFT (density functional theory), and feed the obtained high-quality training data back into model training.
At this stage,The new model will increase the accuracy of predicting material stability from about 50% to 80%, and the discovery rate of new materials from less than 10% to more than 80%.(Click to view the full report: 800 years ahead of humans? DeepMind releases GNoME, using deep learning to predict 2.2 million new crystals)
Accelerate change and calmly deal with the "gray rhino" of the ecological environment
GraphCast
Producing the most accurate global weather forecast
GraphCast, released by Google DeepMind, is a weather forecasting system based on machine learning and graph neural networks (GNNs). It uses an "encode-process-decode" configuration, has a total of 36.7 million parameters, and can make predictions at a high resolution of 0.25 degrees longitude/latitude (28 km x 28 km at the equator).The model covers the entire surface of the Earth. At each grid point, the model predicts five Earth surface variables (including temperature, wind speed, wind direction, mean sea level pressure, etc.), as well as six atmospheric variables at 37 different altitudes, including specific humidity, wind speed, wind direction, and temperature.

In the comprehensive basic test,Compared with HRES (High Resolution Forecast), GraphCast provides more accurate forecasts for nearly 90% out of 1,380 tested variables.According to comparative analysis, GraphCast can also identify severe weather events earlier than traditional forecasting models. (Click here for the full report: Hailstorm Center collects data, large models support extreme weather forecasts, and "Storm Chasers" are on stage)
Flood Forecasting
Artificial intelligence transforms flood forecasting
In 2018, Google launched the Google Flood Forecasting Initiative, using AI and powerful computing power to create better flood prediction models and collaborate with governments in many countries.Google's research team has developed a river forecasting model based on machine learning that can reliably predict floods five days in advance. When predicting flood events that occur once in five years, its performance is better than or equivalent to the current prediction of flood events that occur once a year. The system can cover more than 80 countries.
This study constructed an advanced river prediction model by adopting the application of two Long Short-Term Memory Networks (LSTM).The core architecture of the model is based on the encoder-decoder framework.Specifically, the Hindcast LSTM module is responsible for processing historical meteorological data, while the Forecast LSTM module processes forecasted meteorological data. The output of the model is the probability distribution parameters for each forecast time point, which can provide a probability forecast of the flow of a specific river at a specific time point.

The results of the study showed thatThe model outperforms the current world-leading modelling system, the Copernicus Emergency Management Service Global Flood Awareness System (GloFAS).This finding confirms the potential and reliability of the proposed model in the field of river forecasting and provides a new technical means for flood warning and water resources management. (Click here to view the full report: Google's flood prediction model is published in Nature again, beating the world's No.1 system and covering 80+ countries)
AI: Leading a New Era in Medicine
The 2024 Artificial Intelligence Index Report shows that AI technology has achieved results in many fields, including medical imaging, medical question-answering, and medical diagnosis. In fact, the application of AI in the field of medical health has long been known to people. Through machine learning algorithms, AI can analyze large amounts of medical data to help doctors diagnose diseases more accurately. For example, in cancer detection, AI can identify tiny abnormalities in medical images, thereby improving the success rate of early diagnosis.
In addition, AI also plays an important role in drug development.on the one hand,AI has deepened our understanding of drug targets and compound synthesis, optimized the steps of drug discovery, and greatly increased the chances of successful new drug launches.on the other hand,AI technology is used to shorten the new drug development cycle, save costs, and significantly improve drug development efficiency and corporate competitiveness.
It is worth noting that the "2024 Artificial Intelligence Index Report" also summarizes artificial intelligence-related medical devices, and the number of approvals for AI-related medical devices by the US Food and Drug Administration (FDA) continues to increase. In 2022, the FDA approved 139 AI-related medical devices, an increase of 12.1% from the previous year. This number has increased more than 45 times since 2012, showing the widespread use of AI in real-world medical applications.
Although the application of AI technology in actual medical care has brought many opportunities, it also faces a series of challenges that need to be addressed, such as AI ethical issues, data privacy protection, technical bottlenecks, supervision and accountability, interdisciplinary cooperation, clinical applicability, etc. In particular,AThe “black box” nature of the I model makes its decision-making process difficult to explain, which is a major challenge for medical diagnosis that requires a high degree of transparency and traceability.Lack of explainability may affect doctors' trust in AI-assisted diagnosis results.
Therefore, in addition to technological iteration, how to fill in the gaps in policies, standards, supervision, security, etc., and how to break the "black box" characteristics of themselves still require the government and relevant companies to jointly promote solutions.
Medical imaging: providing more comprehensive and in-depth solutions
The application of AI technology in the field of medical imaging is becoming more diverse and in-depth. From assisting diagnosis to improving workflows to promoting personalized medicine, AI is becoming an indispensable tool for medical imaging.
SynthSR
Convert high-resolution images and repair lesions
Developed by MIT’s Computer Science and Artificial Intelligence Laboratory, SynthSR trains a super-resolution convolutional neural network (CNN) using an open access dataset of 1 mm isotropic high-field MRI scans and precise segmentation of 39 regions of interest (ROIs) within the brain.The technology focuses on low-field (0.064-T) T1- and T2-weighted brain MRI sequences, while using magnetization-prepared rapid gradient echo (MPRAGE) acquisition technology, aiming to produce high-quality images with 1-mm isotropic spatial resolution.

The advanced features of SynthSR are:It can convert clinical MRI scan data of different directions, resolutions and contrasts into 1mm isotropic MPRAGE images, and repair lesions in the process.
The converted synthetic MPRAGE images can be directly applied to existing brain MRI 3D image analysis tools, such as image registration or segmentation.No additional training is required.In addition, by comparing the brain morphometric data of synthetic images with actual high-field strength images, the study further verified the application potential of LF-SynthSR in the field of quantitative neuroradiology.
Paper link:
http://arxiv.org/pdf/2012.13340v1.pdf
CT Panda
Early pancreatic cancer screening
In view of the hidden location of pancreatic cancer and the lack of obvious manifestations in plain CT images,Alibaba DAMO Academy, in collaboration with research teams from more than a dozen medical institutions around the world, used AI to screen pancreatic cancer in asymptomatic people, built a unique deep learning framework, and ultimately trained the PANDA model for early detection of pancreatic cancer.
The PANDA model is an advanced medical image analysis tool that uses a combination of deep learning techniques to improve the efficiency and accuracy of pancreatic lesion detection. The model first uses a segmentation network (U-Net) to accurately locate the pancreatic region, and then uses a multi-task convolutional neural network (CNN) to identify abnormalities in the image. Finally, a two-channel Transformer model is used to classify the detected abnormalities and identify the specific type of pancreatic lesions.
The core advantage of this technology is that it can use AI algorithms to magnify and identify tiny lesion features in plain scan CT images that are difficult to identify with the naked eye.This not only achieves efficient and safe detection of early pancreatic cancer, but also effectively solves the problem of high false positive rate in previous screening methods.

In the validation test, PANDA's sensitivity was 34.1% higher than that of ordinary radiologists, and its specificity was 6.3% higher than that of ordinary radiologists.In a large-scale real-world test involving approximately 20,000 patients, PANDA achieved a sensitivity of 92.9% and a specificity of 99.9%.(Click here to view the full report: 31 missed diagnoses were identified among 20,000 cases, and Alibaba Damo Academy took the lead in releasing "plain scan CT + large model" to screen pancreatic cancer)
Medical diagnosis: Develop personalized, accurate diagnosis and treatment plans
From improving diagnostic efficiency and accuracy to providing personalized treatment plans, AI technology has great potential in the field of medical diagnosis, helping to improve the quality of medical services and patient experience.
Coupled Plasmonic Infrared Sensors
Empowering neurodegenerative disease diagnosis
In the field of neurodegenerative disease diagnosis, the lack of effective tools for detecting preclinical biomarkers has made the early diagnosis of diseases such as Parkinson's syndrome and Alzheimer's disease a major challenge. Although traditional detection methods such as mass spectrometry and enzyme-linked immunosorbent assay (ELISA) are helpful to a certain extent, they have limitations in identifying changes in the structural state of biomarkers.
To address this problem,A research team from EPFL has developed an innovative diagnostic method that combines neural network technology, plasmonic infrared sensors using surface-enhanced infrared absorption (SEIRA) spectroscopy, and immunoassay technology (ImmunoSEIRA) to enable quantitative analysis of the stage and progression of neurodegenerative diseases.

The ImmunoSEIRA sensor uses an array of gold nanorods modified with antibodies targeting specific proteins, which can capture target biomarkers from very small amounts of samples in real time and perform structural analysis on them. Subsequently, neural networks are used to identify misfolded proteins, oligomers, and fibril aggregates, achieving an unprecedented level of high accuracy detection. This method provides a new technical means for the early diagnosis and accurate assessment of neurodegenerative diseases.
CoDoC
Logical integration between AI and doctors’ diagnosis
Google DeepMind has developed a medical assistance artificial intelligence system called CoDoC, which is designed to provide in-depth interpretation and analysis of medical images. Through learning, the system can decide when to rely on its own judgment and when to adopt the doctor's opinions.
Specifically, the DeepMind team explored various application scenarios where clinicians use AI tools to assist in interpreting medical images. For any theoretical case in a clinical setting, the CoDoC system only requires three inputs for each case in the training dataset:* first,A confidence score for the predicted AI output, which ranges from 0 (definitely no disease) to 1 (definitely disease);* Secondly,clinicians’ interpretation of medical images;
* at last,The objective existence of disease.
It is worth noting thatThe CoDoC system does not require direct access to the medical images themselves.

In addition, DeepMind conducted a comprehensive test of the CoDoC system using multiple real-world de-identified historical data sets. The test results show that combining human medical expertise with the predictions of AI models can provide the most accurate diagnosis solution.Its accuracy exceeds that achievable using either method alone.This finding highlights the importance of AI working in collaboration with human experts and provides new perspectives for improving the accuracy and reliability of medical imaging diagnoses.
Medical Q&A: Improve diagnostic accuracy, optimize treatment plans, and enhance patient service experience
In 2020, researchers proposed MedQA, a medical question-answering system based on knowledge graphs, which uses knowledge graphs to represent and store structured and semi-structured data in the medical field, and then retrieves or generates answers from the knowledge graphs through graph search, reasoning, or matching techniques. Since the release of MedQA, AI's ability to answer medical knowledge questions has also received more widespread attention.
GPT-4 Medprompt
Accuracy exceeds 90%
GPT-4 Medprompt developed by the Microsoft Research team surpassed 90% in accuracy for the first time on the MedQA dataset (U.S. Medical Licensing Examination questions).Beyond BioGPT and Med-PaLM and other fine-tuning methods. The researchers also said that the Medprompt method is universal and can be applied not only to medicine, but also to electrical engineering, machine learning, law and other professions.
Medprompt is a combination of multiple prompting strategies, including:* Dynamic few-sample selection:The researchers first used the text-embedding-ada-002 model to generate vector representations for each training sample and test sample. Then, for each test sample, the most similar k samples were selected from the training sample based on vector similarity.* Self-generated thought chain:The Chain of Thought (CoT) method is to let the model think step by step and generate a series of intermediate reasoning steps. Compared with the chain of thought examples handcrafted by experts in the Med-PaLM 2 model, the chain of thought rationales generated by GPT-4 are longer and the step-by-step reasoning logic is more fine-grained.
* Option shuffle integration:When GPT-4 is doing multiple-choice questions, there may be a bias, that is, no matter what the options are, it will tend to always choose A, or always choose B. This is position bias. To reduce this problem, the researchers chose to shuffle the original order of the options and then let GPT-4 make multiple rounds of predictions, using a different order of the options in each round.

Research has shown that Medprompt outperforms the top-ranked Flan-PaLM 540B in 2022 by 3.0, 21.5, and 16.2 percentage points in the multiple-choice section of several well-known medical benchmarks, including PubMedQA, MedMCQA, and MMLU, respectively. Its performance also surpassed the state-of-the-art Med-PaLM 2 at the time.
MediTron-70B
Best open source large language model for healthcare
Since GPT-4 Medprompt is a closed source system, it limits its free use among the wider public. To address this issue,Researchers at the Swiss Federal Institute of Technology in Lausanne developed the MediTron-70B based on this system, aiming to provide aAn open source, high-performance large-scale language model for the medical field.
MediTron is a deep learning algorithm.Built on the Llama 2 architecture and fine-tuned using Nvidia's Megatron-LM distributed trainer,An extended pre-training was also performed on a comprehensive medical corpus of carefully selected PubMed articles, abstracts, and internationally recognized medical guidelines.

The MediTron series includes two models: MediTron-7B and MediTron-70B.The performance of MediTron-70B has surpassed that of GPT-3.5 and Med-PaLM, and is close to the level of GPT-4 and Med-PaLM-2.
To promote the development of open source medical LLMs, the development team has made public the medical pre-trained corpus used and the weight code of the MediTron model. MediTron-70B has the highest score among open source models on MedQA, an achievement that marks an important progress in the field of open source medical LLMs.
Paper link:
https://arxiv.org/pdf/2311.16079.pdf
MedAlign
Reduce the burden of healthcare administration
Current electronic health record (EHR) question answering datasets for text generation tasks in healthcare do not adequately capture the complexity that clinicians face in information needs analysis and document processing.
To fill this gap, a team of 15 clinicians from different fields of expertise launched MedAlign - a benchmark dataset based on EHR data. This dataset contains 983 real-world clinical questions and their instructions, as well as answers provided by 303 clinicians. The instruction-response pairs are constructed by analyzing 276 longitudinal EHR data.
This work not only addresses the lack of an evaluation benchmark for the practicality of LLMs in complex clinical tasks, but also promotes the research progress of natural language generation in the healthcare field by providing a realistic and comprehensive command response dataset.

On the MedAlign dataset, the researchers tested six large language models from different general domains and evaluated the accuracy and quality of the responses generated by each large model by clinicians.
The results show thatThe GPT-4 model variant that has undergone multi-step optimization has achieved an accuracy of 65.0%, which is generally preferred over other LLMs. As the first benchmark dataset to broadly cover EHR applications, MedAlign marks an important step forward in using artificial intelligence technology to reduce the administrative burden of healthcare.
Paper link:
https://arxiv.org/pdf/2308.14089.pdf
Medical research: Using AI to build the strongest line of defense for human health
With the continuous advancement of technology, the application of AI technology in the field of medical research has become more extensive and in-depth. Today, scientists are using the power of AI to deeply explore the code of human genes and use AI to help us build a solid medical defense line.
AlphaMissence
Effectively identify pathogenic missense mutations in genes
Based on AlphaFold, the Google DeepMind team further developed a new AI model - AlphaMissense.The model combines the high-precision protein structure model provided by AlphaFold and the constrained evolution algorithm extracted from related sequences. The training process of AlphaMissense is divided into two stages:
* The first stage is similar to the training of AlphaFold, focusing on enhancing the weights of the protein language model;
* The second phase focuses on fine-tuning the model to more accurately match pathogenicity, assigning a benign or pathogenic label to the mutation based on its frequency in the population.

The results of the study show thatAlphaMissense successfully predicted 71 million missense mutations in human protein-coding genes.Missense mutations are genetic variations that can affect protein function and may lead to a variety of diseases including cancer.AlphaMissense was able to classify 89% variants, of which approximately 57% were judged as likely benign variants (Likely benign), 32% were judged as likely pathogenic variants (Likely pathogenic), and the remaining variants were classified as uncertain nature (Uncertain).
This classification capability far exceeds that of human annotators, who can only confirm 0.11 TP3T among all missense mutations. The high efficiency and accuracy of AlphaMissense provide a powerful tool for the research and clinical diagnosis of genetic diseases.
Paper link:
https://www.science.org/doi/10.1126/science.adg7492
EVEscape
Early warning system for pandemics
A research team from Harvard Medical School and Oxford University jointly developed an innovative universal modular framework, EVEscape.Ability to predict the virus's escape potential without relying on sequencing data or antibody structure information during the pandemic.
EVEscape's accuracy in predicting SARS-CoV-2 pandemic mutations is comparable to that of high-throughput deep mutation scanning (DMS) technology, and its application is not limited to SARS-CoV-2 but can be extended to other virus types. This early warning system provides guidance for public health decision-making and preparedness, helping to minimize the negative impact of the pandemic on human health and socioeconomics.

The EVEscape framework consists of two main parts:* One part is a model for generating evolutionary sequences,The model provides insights into possible viral mutations, similar to the model used in the EVE (Evolutionary Virus Escape) project;
* Another part is a database containing detailed biological and structural information about the virus.By integrating these two components, EVEscape is able to predict the characteristics of viral variants before they actually emerge.
Through a retrospective analysis of the SARS-CoV-2 pandemic, the research team confirmed the effectiveness of EVEscape in predicting mutations with pandemic escape potential, months earlier than methods that rely on traditional antibody and serum experiments, while maintaining considerable accuracy. Early identification of potential escape mutations using EVEscape can provide key information for the design of vaccines and treatments, thereby more effectively controlling the spread of the virus.
Paper link:
https://doi.org/10.1038/s41586-023-06617-0
Human Pangenome Reference
First draft of human pan-genome mapped
In the early 21st century, the Human Genome Project successfully released the preliminary draft of the human reference genome, marking a breakthrough in humanity's understanding of its own life blueprint. However, due to the limitations of sequencing technology at the time, the draft had several unfilled blank areas.
In 2023, an international consortium of 119 scientists from 60 institutions, led by the University of Washington School of Medicine and the University of California, used artificial intelligence technology to develop the first updated and more representative draft of the human pan-genome.
The draft used advanced "long-read sequencing" technology to conduct in-depth analysis of 94 genome samples from 47 individuals with different ancestral backgrounds around the world.Subsequently, the measured long DNA fragments were assembled into a more complete genome sequence through a customized algorithm. The results showed that the draft reached 99% in coverage of the expected sequence, and also exceeded 99% in terms of structure and base pair accuracy.

Compared with the old workflow based on GRCh38, the new draft is more efficient when analyzing short-read data.The discovery error of small genetic variants was reduced by 34%, while the detection rate of haplotype structural variants was improved by 104%, adding 119 million base pairs.In addition, the new draft also revealed two important new components that regulate gene expression: HIRA and SATB2. These findings are of great significance for a deeper understanding of the structure and function of the human genome.
2024, AI leads the future of scientific research
Artificial intelligence is becoming a core driving force for scientific and medical progress with its amazing potential. In 2024, the rapid development of AI is bringing revolutionary changes to scientific research and medicine, with a speed and impact far greater than ever before. AI not only accelerates the accumulation of knowledge and the cycle of innovation, but is also redefining our understanding and solution of complex problems.
In the field of scientific research,AI algorithms and models are helping scientists process and analyze huge data sets, revealing the deep insights hidden behind the data. They have shown great advantages in simulating and predicting the behavior of complex systems, thus making groundbreaking discoveries in many basic science fields such as physics, chemistry, and biology.
In the medical field,AI-assisted diagnostic tools are becoming more accurate, able to detect signs of disease early and provide patients with more timely treatment. At the same time, the application of AI in personalized medicine can customize more accurate treatment plans for patients by analyzing individual genetic information and biomarkers, greatly improving treatment outcomes and patient quality of life.
also,The role of AI in drug development cannot be underestimated.By predicting the activity of molecules and the side effects of drugs, it greatly shortens the cycle of new drugs from laboratory to market, reduces R&D costs, and accelerates the process of bringing new drugs to market.
It can be said that every step of progress in AI is like a stone thrown into the long river of human wisdom, causing ripples and pushing the boundaries of scientific research and medicine forward. Humans who are good at using tools will eventually use the power of these stirrings to move towards a new era of greater intelligence and health.