Good News for Patients With Drug-resistant Bacterial Infections! McMaster and Stanford University Team up to Develop New Antibiotics Using Generative AI
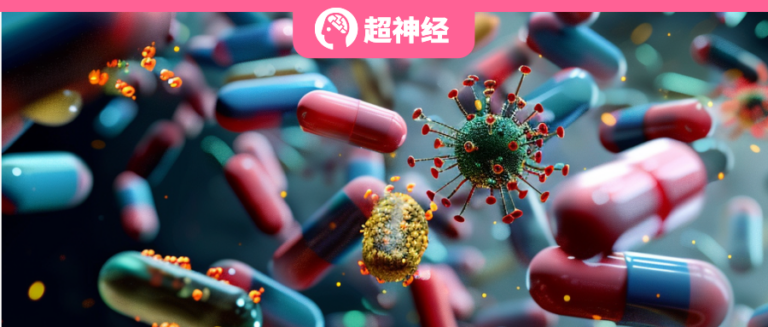
Since Alexander Fleming discovered penicillin in 1928, antibiotics, as one of the most important discoveries in human medical history, have completely changed modern medicine. However, in actual diagnosis and treatment,The abuse of antibiotics is becoming more and more serious.This phenomenon is particularly prominent in some third- and fourth-tier cities where supervision is lax, which has also led to the development of drug resistance in bacteria.
A study published in The Lancet in 2022 suggested thatNearly 5 million people died from drug-resistant bacterial infections in 2019, a figure expected to reach 10 million by 2050.The same study also revealed that antimicrobial resistance (AMR) is now the third leading cause of death worldwide, after heart disease and stroke, and surpassing mortality from HIV/AIDS, breast cancer and malaria.
In the face of the problem of bacterial resistance, it is urgent to accelerate the development of novel antibiotics on the basis of strictly controlling the abuse of antibiotics. Today, although artificial intelligence methods can help discover new antibiotics, there are still many limitations. For example, the predictive models that evaluate the specific properties of molecules do not scale well in large chemical spaces.
To address this issue, researchers from McMaster University and Stanford University published a paper titled "Generative AI for designing and validating easily synthesizable and structurally novel antibiotics" in Nature Machine Intelligence, a Nature subsidiary.
The study developed a new generative artificial intelligence model, SyntheMol, which can design new, easily synthesized compounds based on the chemical space of nearly 30 billion molecules.The research team used SyntheMol to design molecules that can inhibit the growth of the superbug Acinetobacter baumannii (A. baumannii). They synthesized 58 molecules and verified that 6 of them with novel structures showed antibacterial activity against Acinetobacter baumannii and other bacterial pathogens.
Research highlights:
* SyntheMol, a generative AI model, can design new compounds that are easy to synthesize from a chemical space of nearly 30 billion molecules
* The model not only creates new drug structures, but also provides detailed formulas for chemical synthesis
* Experimental results show that generative AI has the potential to design novel, synthesizable and effective small molecule antibiotic candidates from a wide range of chemical spaces

Paper address:
https://www.nature.com/articles/s42256-024-00809-7
Follow the official account and reply "synthetic antibiotics" to get the full PDF
Dataset: Contains 13,524 unique molecules
This study first performed physical screening on three different chemical libraries to be used as training datasets.
Chemical library 1 contains 2,371 molecules from the Pharmakon-1760 library (360 FDA-approved drugs and 400 internationally approved drugs) and 800 natural products isolated from plants, animals, and microorganisms.
Chemical library 2 is the Broad Drug Repurposing Hub database, which contains 6,680 molecules, most of which are FDA-approved drugs or clinical trial candidates.
Chemical Library 3 is a synthetic small molecule screening set of 5,376 molecules randomly sampled from a larger chemical library at the Broad Institute.
For each database, the researchers conducted two biological replicates and calculated the average standardized OD600 value for each compound. They also calculated the mean μ and standard deviation σ of these OD600 values, and then used the threshold μ − 2σ to binarize these values, marking all values below the threshold as active and all values equal to or above the threshold as inactive. The researchers then merged the three binarized libraries and deleted duplicate compounds with conflicting activity labels.
After the above processing,The final data set contained 13,524 unique molecules, of which 470 (3.5%) were active compounds and 13,054 (96.5%) were inactive compounds.
Model architecture: Monte Carlo tree search guided by property prediction model
The superbug A. Baumannii is known for being difficult to eradicate and can cause pneumonia, meningitis and infected wounds. The World Health Organization (WHO) has identified it as one of the most dangerous antibiotic-resistant bacteria in the world.The experiments revolved around potential antibiotic candidate compounds targeting this superbug.

As shown above, first, the researchers screened a training set of about 13,000 molecules and performed growth inhibition tests to determine their biological activity against A. baumannii.These screening data were then used to train a property prediction model to predict antibacterial activity.
To generate molecules,The researchers selected a chemical space consisting of nearly 30 billion molecules.Each molecule can be synthesized through one of 13 chemical reactions that combine two or three molecules from about 132,000 building blocks.
The generative AI model SyntheMol uses Monte Carlo tree search (MCTS) guided by a property prediction model to search the vast combinatorial chemical space.To search for promising antibiotic candidate compounds active against A. baumannii.
In each MCTS expansion, SyntheMol builds molecules by selecting Roots and combining them with Reactions. The generated molecules are then evaluated by the property prediction model and provide feedback to the MCTS algorithm. As the MCTS expansion proceeds, SyntheMol learns which combinations of Roots and Reactions can produce molecules with high property prediction scores. This is shown in the figure below:

After a set of expansions, SyntheMol outputs all generated compounds and the specific synthetic schemes needed to synthesize each molecule—including the order of Roots and Reactions.
After 20,000 iterations, the researchers screened out a group of high-scoring compounds with novel structures and a wide variety.The compounds were synthesized and experimentally tested in vitro against phylogenetically diverse bacterial species.
Research results: SyntheMol helps chemists synthesize antibiotics and create formulations
In the experiment, the researchers applied SyntheMol to discover potential antibiotic candidate compounds against A. baumannii using antibiotic property prediction models, and mainly used Chemprop within SyntheMol to present the results.
In the process of 20,000 MCTS deployments (less than 8.5 hours),SyntheMol and Chemprop evaluated 452 million intermediate nodes, which contains a variety of combinations of molecular building blocks and generates 24,335 complete molecules,Of these, 2,868 had a Chemprop antimicrobial prediction score of at least 0.5. This outperformed an AI-based virtual screening approach, in which Chemprop scored 10 million randomly sampled REAL molecules (8 hours) and identified only 374 molecules with a score of at least 0.5 (only 13% from SyntheMol).

Chemprop Antimicrobial Model Score
SyntheMol generated high molecules in all MCTS expansions, but these high molecules were mainly concentrated in the early expansions. In the first 2,000 expansions, 2,868 (36%) Chemprops were generated, of which 1,035 (10%) had a score of at least 0.5. Although 20,000 expansions only explored a portion of the chemical space of nearly 30 billion molecules, these results show thatSyntheMol quickly generated many of the top-scoring compounds.
The researchers selected 70 compounds that were most likely to kill bacteria and worked with Ukrainian chemical company Enamine to synthesize them. The company was able to effectively produce 58 of these compounds, and the researchers further verified the biological activity of these 58 compounds against A. baumannii. The experimental results showed thatThe six molecules shown in the figure below (Enamine 10, 23, 28, 31, 40 and 43) showed excellent antibacterial activity.That is, the minimum inhibitory concentration (MIC) was ≤ 8 μg ml−1. This represents a hit rate of 10%, which is almost three times the hit rate of 3.5% in the training set.

also,These six novel molecules also showed antibacterial efficacy against several other phylogenetically different bacterial pathogens (Klebsiella pneumoniae, methicillin-resistant Staphylococcus aureus, etc.).Specifically, when used in combination with outer membrane perturbing agents such as SPR 741 or colistin, all six molecules showed broad-spectrum activity against multiple Gram-negative bacteria including Bacillus baumannii, Escherichia coli, and Klebsiella pneumoniae, and one of the molecules, Enamine 40, was also active against Pseudomonas aeruginosa.
As single agents, these molecules inhibited the growth of the Gram-positive bacterium methicillin-resistant Staphylococcus aureus, and Gram-negative isolates of LPS-deficient and colistin-resistant Bacillus baumannii. The experimental results are shown in the figure below:

at present,The SyntheMol model also has some shortcomings. It was not programmed to produce molecules with high water solubility. Four of the six antimicrobial molecules were insufficiently water soluble, and only two could be tested for toxicity in a mouse model.
While more research is needed to translate these generated molecules into viable antibiotic candidates and to improve generative AI approaches for the design of synthesizable molecules, these findings demonstrate the potential of generative AI to design structurally novel, synthesizable, and potent small-molecule antibiotic candidates from a broad range of chemical space.
It is more worth emphasizing thatThe model not only creates new drug structures, but also provides detailed recipes for chemical synthesis, allowing chemists to synthesize these drugs in the laboratory.
AI + antibiotic research and development has made breakthroughs
Scientists, public health agencies and governments around the world are warning that antibiotic resistance will be the next major global health crisis because bacteria and other microorganisms have been increasingly becoming resistant to antibiotics and causing more and more deaths since the 1960s.
Although the demand for new antimicrobial drugs is growing, the number of newly developed antibiotics is very small. On the one hand, it takes a long time to develop antibiotics.It takes scientists about 12 years to develop a new antibiotic, and another 3-6 years to discover any clinical candidates.On the other hand, due to the low profits from the research and development of antibiotics, many large pharmaceutical companies have withdrawn from the market.It was estimated that the cost of developing an antibiotic in 2017 was approximately $1.5 billion. However, compared to such a high cost, antibiotic drugs can only bring $46 million in revenue to pharmaceutical companies each year. If we consider revenue and cost alone, it will take about 32 years to recover the investment.
Today, the rapid development of artificial intelligence technology has brought new breakthroughs and paths to the research and development of antibiotics.
As early as 2020, MIT scientists discovered the "halicin" antibiotic molecule using a deep learning model, which demonstrated unprecedented broad-spectrum antibacterial capabilities.This is the first time that a new antibiotic has been discovered entirely using artificial intelligence methods.Halicin could kill some of the world's most dangerous bacteria, researchers say, in a study published in Cell, a leading life sciences journal.
In June 2023, researchers from McMaster University and Massachusetts Institute of Technology in Canada published a research paper titled "Deep learning-guided discovery of an antibiotic targeting Acinetobacter baumannii" in the journal Nature Chemical Biology. The research team used artificial intelligence algorithms toA new antibiotic, abaucin, has been discovered that can specifically kill the important drug-resistant bacterium A. baumannii.The research team also plans to use this AI model to identify antibiotics against other important drug-resistant bacteria, such as Staphylococcus aureus and Pseudomonas aeruginosa. (Click here for detailed interpretation: AI fights superbugs: McMaster University uses deep learning to discover a new antibiotic abaucin)

In December 2023, researchers from MIT developed a deep learning method to discover antibiotics.Using graph neural network Chemprop to identify potential antibiotics from a large chemical library, a new class of antibiotics was discovered.It can specifically kill the important drug-resistant bacteria A. Baumannii. (Click here for detailed interpretation: The curse of "super bacteria" may be broken, MIT uses deep learning to discover new antibiotics)
It is worth noting that although the above study also targeted drug-resistant bacteria A. Baumannii, generative AI was not used. Compared with traditional AI methods,The generative AI model in this study can directly design candidate antibiotic molecules and quickly explore vast chemical space.
In the domestic academic community, in June 2022, researchers from the Institute of Microbiology, Chinese Academy of Sciences published a research paper titled "Identification of antimicrobial peptides from the human gut microbiome using deep learning".Methods for using artificial intelligence to efficiently mine large gut microbiome datasets for peptides with antimicrobial properties are described.The researchers screened 2,349 candidate AMPs from the 4,409 representative genomes retrieved, and further identified 241 antimicrobial peptide sequences through gene expression data, relative abundance, and association with selected bacteria. They synthesized a final set of 216 peptides, 181 of which had antibacterial activity, with a hit rate of 83.8% - a finding that strongly demonstrates that machine learning models can effectively accelerate the progress of discovering new antibiotics.
same year,Professor Liu Bing from the First Affiliated Hospital of Xi'an Jiaotong University successfully developed the super antibacterial drug Drug X using the AI-assisted drug design service based on Huawei Cloud Pangu drug molecule big model.The drug achieves antibacterial effects by targeting microbial histone HU and inhibiting bacterial DNA replication. It is the first time in the world that a bacteriophage-encoded antibacterial inhibitor targeting bacterial histone HU has been discovered. The related research was published in the Proceedings of the National Academy of Sciences of the United States of America under the title "Bacteriophage protein Gp46 is a cross-species inhibitor of nucleoid-associated HU proteins".
On the industrial side,The main domestic antibiotic production enterprises include Lukang Pharmaceutical, North China Pharmaceutical, Kelun Pharmaceutical, Harbin Pharmaceutical Group, and Chuan Ning Biological, and overseas enterprises include Sanofi and Novartis.Many of them have clearly stated that they will introduce AI to optimize their business.
For example, Twinings Biopharma has established a strategic partnership with Shanghai Jinyu Technology Co., Ltd. to use AI to assist in synthetic biology research and development and to jointly develop new products to improve production methods and efficiency. In 2023, Sanofi announced that it would "all in on artificial intelligence and data science" and reached a cooperation with BioMap Biosciences to use BioMap's artificial intelligence platform to jointly develop cutting-edge AI modules for biotherapeutic drug discovery.
Of course, the discovery of antibiotic molecules is just the tip of the iceberg of the application of AI technology in the field of drug discovery. At present, scholars around the world are trying to use artificial intelligence technology to reduce drug research and development costs, shorten research and development time, improve research and development efficiency, and make new drug development fast and efficient.
References:
1.https://www.thepaper.cn/newsDetail_forward_20092860
2.https://www.chinagut.cn/articles/ss/a5871d7d61f64fdc9d902e34520654c7
3.https://www.sohu.com/a/374972885_498729
4.https://www.most.gov.cn/gnwkjdt/202206/t20220614_181127.html