Deconstructing Ocean Hypoxia: Zhejiang University GIS Laboratory Integrates Machine Learning and Satellite Mapping to Release a Comprehensive Modeling Framework for Global Sea Surface Dissolved Oxygen
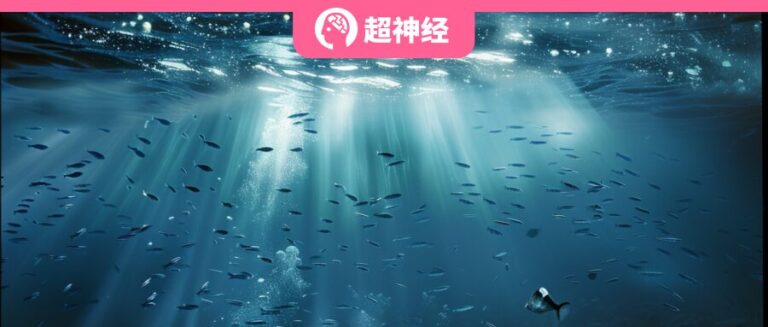
Many years ago, marine expert Eric Prince discovered an anomaly while tracking fish tags: blue marlin usually dive to a depth of 800 meters to hunt in the southeastern United States, but only move on the surface of the ocean in Costa Rica waters.Why did the marlin, an expert in diving, suddenly stop diving?Eric Prince, a long-time billfish researcher, decided to investigate this anomaly.
In fact, this is a self-protection response of marine organisms in the face of changes in the marine environment, and Eric Prince's exploration this time has further brought the "ocean hypoxia" phenomenon behind it to the public's attention.It is precisely because the oxygen content in the deep waters of Costa Rica is decreasing and the oxygen-deficient area is gradually expanding that the marlin have to move on the surface to avoid suffocation.
Areas where a large number of marine life have died due to lack of oxygen are usually called "dead zones" in the ocean.But in fact, the negative impact of ocean hypoxia is not only on marine life itself, but also affects fisheries and even social economy.Nowadays, with the intensification of global environmental problems, ocean hypoxia is becoming more and more serious.
In 2019, the World Conservation Union (IUCN) stated in its report on marine hypoxia that the current areas of low oxygen concentration in the ocean are expanding. Compared with the 45 hypoxic marine areas in the 1960s, there are now more than 600 areas affected by low oxygen conditions. The report pointed out that in the same period,The amount of oxygen-depleted water in the world's oceans has increased fourfold.
In order to better maintain the health of marine ecosystems and protect fishery resources, it is crucial to carry out scientific measurements of ocean dissolved oxygen levels.
However, the current cost of field observations in the ocean is high, the existing observation data is unevenly distributed in space, and the methods for measuring dissolved oxygen concentration are diverse, so the quality of the data obtained also varies greatly. This has brought certain challenges to the study of changes in dissolved oxygen levels in the global ocean.
In response to this, researchers from Zhejiang University's GIS Laboratory proposed a new method that combines machine learning technology with satellite products.A comprehensive global sea surface dissolved oxygen modeling framework DOsurface-Pred Framework was developed, and a large-scale sea surface dissolved oxygen dataset SSDO covering the period from 2010 to 2018 was generated based on this framework.The results show that even in oxygen-rich surface areas, dissolved oxygen levels are decreasing, and this decrease is mainly attributed to changes in sea surface temperature (SST).
Research highlights:
* Proposed a comprehensive modeling framework for global ocean surface dissolved oxygen
* Introduced the SHAP interpreter to identify key variables and their impact on predicted dissolved oxygen results
* Helps to understand the high dynamic changes of dissolved oxygen in the global ocean and explore the laws and causes of deoxygenation

Paper Locationsite:
https://pubs.acs.org/doi/10.1021/acs.est.3c08833
SSDO dataset address:
https://go.hyper.ai/BBlqA
Follow the official account and reply "sea surface dissolved oxygen" to get the complete PDF
Dataset: Satellite + field measurement data
The data used in this study include satellite data, fixed vessels, and conductivity meter field measurements.
Satellite datasets include sea surface temperature (SST), sea surface salinity (SSS), chlorophyll-a (Chl-a), sea surface wind (SSW), and sea level anomaly (SLA). SST data comes from the OISST dataset, SSS data comes from the CCI project of the European Space Agency (ESA), Chl-a data comes from MODIS Aqua and Terra satellites, SSW data is obtained by VAM method combined with multi-satellite microwave wind and instrument observations with cross-platform cross-calibration, and SLA data comes from the AVISO project.
Fixed vessel and conductivity meter field measurements are obtained from the Ocean Station Data (OSD) and the high-resolution Conductivity-Temperature-Depth (CTD) sub-database in the 2018 World Ocean Database (WOD).
The field measurement data used in this study are shown in the figure.There are 28,044 records in total, including 241 records from 2019.

(a) Spatial distribution of field measurement data
(b) Time distribution of field measurement data
(c) Data distribution of different ocean site measurements
(d) Correlation matrix analysis among seven main ocean hydrological variables
Model architecture: three major components of DOsurface-Pred Framework

The researchers proposed an interpretable machine learning framework for embedding spatiotemporal information.The framework consists of three main parts: Spatio-temporal Information Embedding Module, Backbone Regression Module and SHAP Explainer Module.
Part I,Using multiple satellite and field measurements of spatiotemporal information data, namely, sample Xi= {Xi spatial,
Xi temporal, Xi satellite1, ..., Xi satellite n} as input. Through the spatiotemporal information embedding module, the space and month information are converted into polar coordinates, and the converted X‘i={X‘i spatial, X‘i temporal, X‘i satellite1, ..., X‘i satellite n} global representation.
Then, X‘i is passed on to the second part.Different models were evaluated using multi-step gridsearch cross-validation.

The above table is the performance evaluation table of backbone models.The performance of the DOsurface-Pred framework is improved compared to models that do not adopt this framework.
In addition, all tree-based machine-learning models outperformed the benchmark multiple linear regression model. These models are ranked in descending order of performance: ET, RF, GBDT, XGBoost, MLP. Among them,The ET model performed best in all evaluation indicators, with an RMSE value of 11.67 μmol/kg. The model had better generalization ability in dissolved oxygen (DO) modeling and could reduce the overfitting phenomenon of the model.
In the third part,The researchers used the SHAP interpretability method to evaluate the impact of sample feature values on model output results. Through this framework, prediction results were generated under the optimal model.
SHAP can quantify the contribution of different eigenvalues to the prediction results, making the model output easier to understand and thus identifying key variables and their impact on dissolved oxygen prediction results.
Experimental conclusion: SST is the main reason affecting the dissolved oxygen content in the sea surface
DOsurface-Pred Framework can accurately assess the global sea surface dissolved oxygen concentration.Based on this framework, the researchers generated a large-scale sea surface dissolved oxygen dataset spanning 2010-2018, calling it the SSDO product.

(a,b) RMSE and bias error (c,d) Total uncertainty and its components (M, R, P) in different years and months
To evaluate the results generated by the optimal model, the researchers performed uncertainty estimation and spatiotemporal validation.
First, the errors and uncertainties of SSDO products are evaluated. The experimental results show that the three errors (measurement error M, representation error R and prediction error P) together cause the total uncertainty.The total uncertainty was estimated to be ±13.02 μmol/kg.

(a) Scatter density plot of predicted and measured PFL values (b) Spatial locations of matched PFL points, numbered to correspond to the plot numbers provided below (ch) Temporal trend of oxygen anomaly at each location, compared with PFL measured anomaly values
Second, the researchers further verified the accuracy and time series of the SSDO using an independent buoy measurement dataset.The results show that the prediction results have a good fit with the PFL database, with an R² value of 0.86.
In addition, the researchers conducted a comparative assessment of long-term buoy observation locations in different ocean regions.The results show that the predictions are consistent with the spatial variability and long-term trends measured by buoys.These evaluations reliably validate the prediction results and help analyze their applicability in different scenarios.
The researchers also conducted a statistical analysis of the SSDO products, and the results showed that the SSDO data had a similar spatial distribution to the WOD long-term record data.Under the influence of the continuous expansion of the hypoxic zone, the dissolved oxygen level at the sea surface has also become undersaturated. Even at the sea surface where the dissolved oxygen is fully exchanged, the dissolved oxygen shows a downward trend of 0.22 μmol/kg per year on average.In addition, the interannual variation of sea surface dissolved oxygen shows correlation with typical ocean variation phenomena.

(a) Evaluating the impact of global feature averages on model outputs
(b) Evaluate the impact of local features on model output
(c, d) Analysis of the impact of SST and SSS on model output
(e,f) Spatial distribution of the impact of SST and SSS characteristics
The researchers used the SHAP interpretability method to further reveal the driving mechanism of ocean factors such as temperature and salinity on dissolved oxygen in the sea surface.Temperature (SST) and salinity (SSS) were the main controlling factors, showing a negative impact on dissolved oxygen, with SST having the greatest impact on dissolved oxygen levels.This assessment helps improve the reliability of modeling and provides quantitative results of explainable factors for exploring the changes in the temporal and spatial distribution of dissolved oxygen in the ocean and the causes of deoxygenation.
In summary, the researchers used the DOsurface-Pred framework to generate the SSDO dataset and introduced the SHAP interpretability method.It was confirmed that even in the oxygen-rich sea surface areas, the dissolved oxygen content showed a downward trend, and this decrease was mainly attributed to SST changes.
AI and the Ocean, Exploring the Unknown Mysteries of the Deep Sea
Dan Laffoley, senior advisor for marine science and conservation at IUCN’s Global Marine and Polar Program, once said:"Dissolved oxygen concentrations in the ocean continue to decline, exacerbating stress on marine ecosystems already facing rising temperatures and acidification."
At present, in addition to accelerating global and industry-wide carbon emission reductions, in-depth research on the phenomenon of ocean hypoxia and analysis of the important factors that affect the earth's life can also enable people to have a more accurate understanding of the reasons for the extinction or survival of different organisms, and further "prescribe the right medicine."
In this process, the capabilities of emerging technologies such as the Internet of Things and AI have gradually been more deeply applied. In addition to the sea mark dissolved oxygen analysis framework mentioned above, some scholars have also conducted research on marine debris control and marine biodiversity protection based on ocean data such as satellite remote sensing, drones, buoys, sonar, and underwater optics.
For example, in 2019,The United Nations Environment Programme has launched a countermeasure project called CounterMEASURE to address plastic pollution in the Mekong and Ganges rivers.The project used drones to capture aerial images of the Mekong River basin and developed an identification and monitoring model to pinpoint the sources and paths of plastic waste in the Mekong River basin with an accuracy rate of 83.9%.
In addition, The Ripper Group in Australia,We have also developed a product that uses drones and machine vision technology to identify sharks.This product is not only used to prevent coastal areas from being disturbed by sharks, but also plays an important role in protecting shark ecology.
It is true that human society’s high carbon emissions have already had a serious impact on the global climate and ecological environment. Among them, as the origin of life on Earth, the protection of the marine ecological environment is also urgent. We look forward to the fact that in the near future, AI-driven environmental protection measures will be able to address the symptoms and root causes while revealing the truth about rising sea temperatures and decreased dissolved oxygen concentrations, and restore the ocean to its clarity from the source.
References:
1.https://mp.weixin.qq.com/s/bUbYptqccBXC2T9dvkfOfA
2.https://www.cdstm.cn/gallery/hycx/qyzx/201909/t20190904_923957.html
3.http://www.cbcgdf.org/NewsShow/4854/10658.html