Based on Clinical Data From 627 Patients in the United States and Japan, Google Confirms the Effectiveness of AI-assisted Lung Cancer Screening in the Population
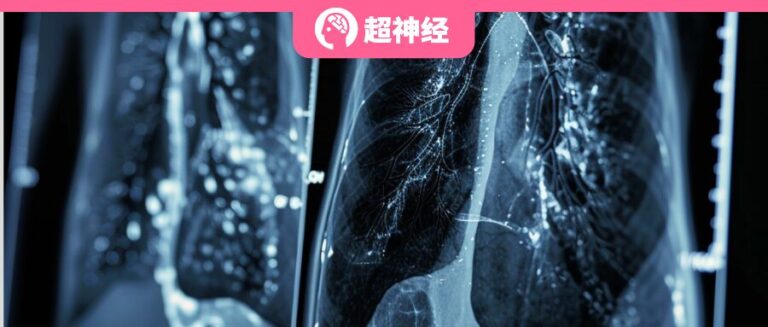
In February 2024, the National Cancer Center compiled the report "Cancer Incidence and Mortality in China in 2022" based on the latest data from tumor registration and follow-up monitoring. The report shows that in my country, lung cancer is still the leading cause of malignant tumor morbidity and mortality.Although the medical community is paying increasing attention to lung cancer, there are still many clinical problems in the diagnosis and treatment of lung cancer, such as delayed diagnosis and overtreatment.In the past decade, AI is leading the direction of technological development at an unprecedented rate due to the improvement of algorithms, the increase in computing power, the expansion of available data and the significant growth in the scope of applications.
As one of the world's most advanced technology giants in the field of AI research and development, Google has made great achievements in the research of AI and medical applications. Recently, Atilla Kiraly, a software engineer at Google Research Center, and Rory Pilgrim, a product manager, studied how machine learning models can effectively convey research results to radiologists.To evaluate the impact of generalized artificial intelligence-assisted systems on lung cancer screening (LCS) workflows in specific workflow settings, devices, and country-specific guidelines and scoring/management protocols.The research paper has been published in the journal Radiology AI.
Research highlights:
* Developed and optimized AI-assisted lung cancer screening workflow and tested it in the U.S. and Japan
* With the assistance of artificial intelligence, the specificity can be increased by 5%-7% without a significant decrease in sensitivity
* With the assistance of artificial intelligence, the average screening time for each case can be reduced by 14 seconds, and the doctor's diagnostic confidence is greatly enhanced

Paper address:
https://doi.org/10.1148/ryai.230079
Follow the official account and reply "Google lung cancer" to get the full PDF
Study scope: Artificial intelligence-assisted screening, a retrospective multinational study in the United States and Japan
The study used five independent datasets (DS_CA, DS_NLST, DS_US, DS_JPN) and analyzed a total of 627 low-dose chest CT cases (including 141 cancer-positive cases) in the United States and Japan.The US study involved 330 patients, including 191 men and 139 women, with a median age of 63. The Japanese study involved 297 patients, including 217 men and 80 women, with a median age of 58. Positive cases were defined as lung cancer diagnosed by pathology within two years, and negative cases were defined as no confirmed cancer diagnosis for at least two years.

at the same time,The study also included 6 U.S. board-certified thoracic radiologists and 6 Japanese board-certified radiologists with experience in reading CT chest examinations.In the study, CT scans of all cases were reviewed once by 12 doctors with and without AI assistance, resulting in a total of 7,254 interpretations. For country-specific scoring systems, radiologists from the United States used the Lung-Rads score (version 1.1) from the American College of Radiology guidelines, and doctors from Japan used the Sendai scoring system.
Among the 141 cancer-positive cases, 124 were from the United States and 17 from Japan. Among them, the nodule situation of the positive cases in the United States showed that there were 69 solid nodules (56%), 15 partially solid nodules (12%), 20 non-solid nodules (15%), and 29 unclassified nodules (23%).
Similarly, among the positive cases in Japan, 6 (35%) were solid nodules, 6 (35%) were partly solid nodules, and 5 (29%) were non-solid nodules. In the cancer-negative cases, the number of nodules of different sizes was also selected to match the proportion of previous cases with the proportion of cancer cases.
Model analysis: Based on the improvement of existing models, statistical analysis capabilities are greatly improved
The first step in the research was to improve a previously developed machine learning model with additional training data and architecture.
The lung cancer screening system consists of 13 models that work in coordination to first segment the lungs, get an overall assessment, and locate three suspicious areas. This information is then used to assign a suspicious rating to each area. The system uses Google Kubernetes Engine (GKE) deployed on Google Cloud to ingest images, run machine learning models, and provide results.
Simply put, the system output provides suspicion rating and ROI (Region of Interest) for doctors to make decisions based on the scoring guidelines in their region.

In the second step, the researchers used three datasets, DS_CA, DS_US, and DS_NLST, to develop and train the model.
Training set:
* DS_CA is data from a Canadian hospital system, which consists of diagnostic CT examinations from 2010 to 2017 and is used to train the final classification model.
* Nonscreening CT of DS_US patients was used for additional training.
* DS_NLST consisted of 26,722 patients, and a subset obtained by random split at the patient level was used to develop the AI system.
Test set:
* The first test dataset is 15% randomly split from the total cases of DS_NLST.
* The second test dataset screened CT cases from DS_US, a dataset from a hospital system in Illinois, USA, separating 1,1792 unidentified cases from 5,055 patients.
* The third dataset DS_JPN is from Sendai Kousei Hospital, Japan, and consists of 301 patients who underwent LDCT screening between 2006 and 2018.
To improve the AI system's ability to detect cancer at an early stage, the study included cancer-positive cases up to two years before diagnosis cancer_in_2. In cancer_in_2, all negative cases were followed up for at least two years to ensure that no cancer was confirmed, and positive cases were all diagnosed by imaging within 2 years.
The primary objective of the study was to calculate sensitivity and specificity by integrating physicians' level of suspicion (LoS) scores for cancer, scanning all possible numerical thresholds, deriving receiver operating characteristic curves for LoS, and calculating AUC. Secondary objectives were to explore the sensitivity/specificity of physicians using local scoring systems and case management decision thresholds, which were calculated based on binary thresholds of urgency.
For example, “6-month follow-up CT” is considered less urgent than “3-month follow-up CT”, which in turn is less urgent than “suspected malignancy”. Similarly, the same analysis was performed on the response of the scoring system and the response of the AI system, and the AUC differences were compared using ORH analysis.
In the results, p value < 0.05 indicated that the AUC difference comparison was statistically significant, and p value < 0.0125 or < 0.01 indicated statistical significance after Bonferroni correction, respectively.
Study results: AI is effective, but it can still miss lesions
In studies in the United States and Japan,Relative to unassisted approaches, AI assistance increased sensitivity for all physicians in scoring systems and case management options.Among them, studies on cases in the United States and Japan showed that both LoS and AUC improved by 0.023, and artificial intelligence assistance achieved higher sensitivity and specificity in all Lung-RADS scores.

Sensitivity and specificity scores were higher in all case management categories with AI assistance.The AI-assisted specificity of the American and Japanese cases increased by 5.5% and 6.7%, respectively.However, the specificity of biopsy recommendation in positive screening decreased by 1.1%.
The study also focused on the analysis of the patient population in the US National Lung Cancer Screening Trial, and the results showed that the specificity was still improved by 3.4%. The results of the Japanese case study also showed that with the assistance of artificial intelligence,On average, the screening time for each case can be reduced by 14 seconds.And it greatly enhances the doctor's diagnostic confidence.

In terms of auxiliary positioning, the AI system marked the nodules that deserve the most attention in 89% and 75% cancer cases in studies in the United States and Japan, respectively, but in other cases AI may miss some nodules that deserve the most attention.
For example, in one case, a physician marked the case as suspicious and the AI system marked it as negative, and further comparison identified smaller subsolid nodules that had not been diagnosed as cancer with at least two years of negative follow-up; on further radiologist review, they appeared to be minimally invasive adenocarcinomas and are being further observed.
In conclusion,The system demonstrated efficacy in a retrospective study across two countries, PACS systems, and patient populations.Because AI-assisted interpretation of challenging lung cancer screening cases reduces unnecessary follow-up and has the potential to reduce overuse of follow-up imaging, avoid frequent lung biopsies, and reduce the burden on the healthcare system.
Google's lung cancer research continues
In fact, Google has a long history of research on lung cancer.
As early as May 7, 2019, Google announced at the 2019 Developer Conference that its artificial intelligence technology can detect lung cancer a year earlier than doctors, increasing the patient's chance of survival by 40%. Google will also work to transform these technologies into practical medical solutions to improve the treatment and prognosis of lung cancer patients.
Just over ten days after the 2019 Developer Conference ended, Google AI researcher Daniel Tse collaborated with researchers from Stanford, New York University and other institutions to publish their latest deep learning model in the journal Nature Medicine. Tests showed that the AI program had an accuracy rate of 94% in judging human diseases, which was better than the six radiologists who participated in the test, and these human doctors had about 8 years of clinical experience.
Since then, Google has not stopped its exploration of lung cancer. Based on the continuous development of the above machine learning model, Google has finally further verified the effectiveness of the model in multiple countries, multiple experts, and multiple patients. This may also mean that the actual application of artificial intelligence in the field of lung cancer will also accelerate, and will truly benefit mankind in the near future.