The Accuracy of Lithium Battery Life Prediction Has Been Improved by 20%! The Team From Shanghai Jiaotong University Released the semi-supervised Learning Method PBCT to Extract Hidden Information From Unlabeled Data
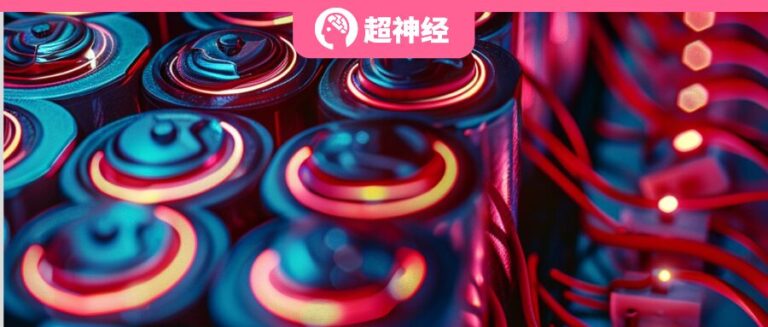
Lithium batteries have the advantages of high energy density, fast charging and discharging speed, and long service life. They are currently widely used in energy storage power systems such as hydropower, thermal, wind and solar power stations, as well as power tools, electric vehicles, military equipment, aerospace and other fields, playing a vital role in modern society.
However, with the large-scale use of lithium batteries, their potential dangers have gradually emerged - they have poor overcharge and discharge resistance, and are very likely to cause fires or even explosions when overcharged or short-circuited. In recent years, spontaneous combustion of electric bicycles and new energy vehicles has frequently occurred due to improper charging or battery aging.
In order to ensure the safe and sustainable operation of the battery system, people need to accurately predict the life of lithium batteries in order to effectively manage their health status.Traditional prediction methods based on physical and semi-empirical models are susceptible to errors and have very limited accuracy.With the development of artificial intelligence technology, data-driven prediction methods have improved in accuracy. However, the accuracy of their models is often constrained by the scarcity of labeled data.
In response to the above challenges, the team led by Associate Professor Wan Jiayu from the Puyuan School of Future Technology at Shanghai Jiao Tong University published a research paper titled "Semi-supervised learning for explainable few-shot battery lifetime prediction" in the internationally renowned journal Joule.A semi-supervised learning technique called partial Bayesian co-training (PBCT) is proposed.By making full use of the low-cost and abundant unlabeled data generated throughout the life cycle of lithium batteries, extracting hidden information, and deepening the understanding of the underlying data patterns, the accuracy of battery life prediction can be significantly improved.
Compared with existing methods, PBCT not only significantly improves the accuracy of life prediction, but also requires almost no additional data collection costs.
Research highlights:
* Predicting battery life using limited data via semi-supervised learning
* Bayesian Co-training (PBCT) semi-supervised learning method performs well in terms of accuracy and interpretability
* Significantly reduce economic costs by reducing the need for labeled data

Paper address:
https://doi.org/10.1016/j.joule.2024.02.020
Follow the official account and reply "Lithium battery life" to get the complete PDF
Dataset: 124 batteries, 3 sub-datasets
The dataset used in this study is an open source dataset provided by Severson et al., which contains 124 1.1 Ah nominal capacity LiFePO4/graphite A123 APR18650M1A cylindrical cells with an average life of 806 cycles (ranging from 150 to 2,300 cycles).
According to different calendar aging and fast charging protocols, the dataset used in this study is divided into three sub-datasets, with 41 batteries (dataset 1), 43 batteries (dataset 2), and 40 batteries (dataset 3).Each dataset contains 20 input features extracted based on domain knowledge.
Model Architecture: Building a High-Performance Full View Model
The architecture of the PBCT method is shown in the figure below, in which two models are established.That is, partial-view model and complete-view model.

Since the full view model takes all features into account, it is prone to overfitting and may lead to poor prediction accuracy.So the researchers created a partial view model to estimate pseudo labels for unlabeled data based on a subset of important input features.
Subsequently, according to the maximum a posteriori optimization principle, it is jointly trained again with the full view model, so that the partial view model acts as a guide to help derive the high-performance full view model that is finally used.

This allows the full view model to gain useful insights from both labeled and unlabeled data, leading to more reliable predictions.
Research results: PBCT opens up a new path for battery life prediction
In the performance evaluation experiments, the researchers used Dataset 1, Dataset 2, and Dataset 3 for experiments. Each set of experiments was further divided into offline and online scenarios, and the root mean square error (RMSE) of the test data was used to evaluate the accuracy of the training model. Due to the scarcity of labeled training data in the experimental setting, the researchers used classic regression methods to deal with few-sample situations, such as Lasso and Elastic net as baselines.
The experimental results corresponding to the offline scenario are shown in Figures A to C below.The proposed PBCT method outperforms the baseline methods given different amounts of labeled training data.When the number of labeled training data is 10, PBCT outperforms Lasso by 9.8%, 21.9%, and 18.3% on datasets 1 to 3, respectively.
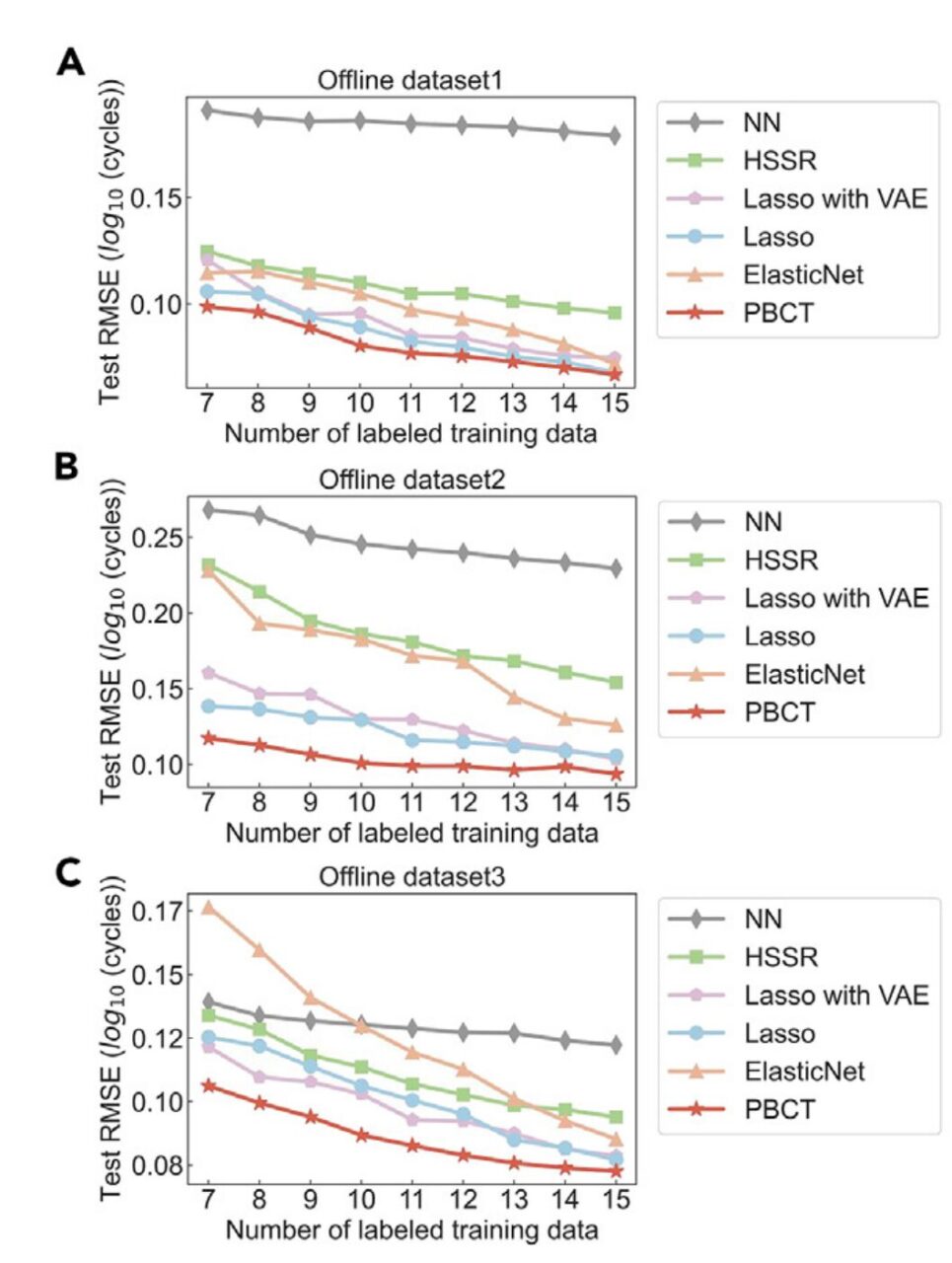
The experimental results in the online scenario are shown in Figures D to F below. The PBCT method outperforms the baseline method in all cases of datasets 2 and 3, and also outperforms the baseline method in most cases when the number of labeled training samples in dataset 1 is scarce.
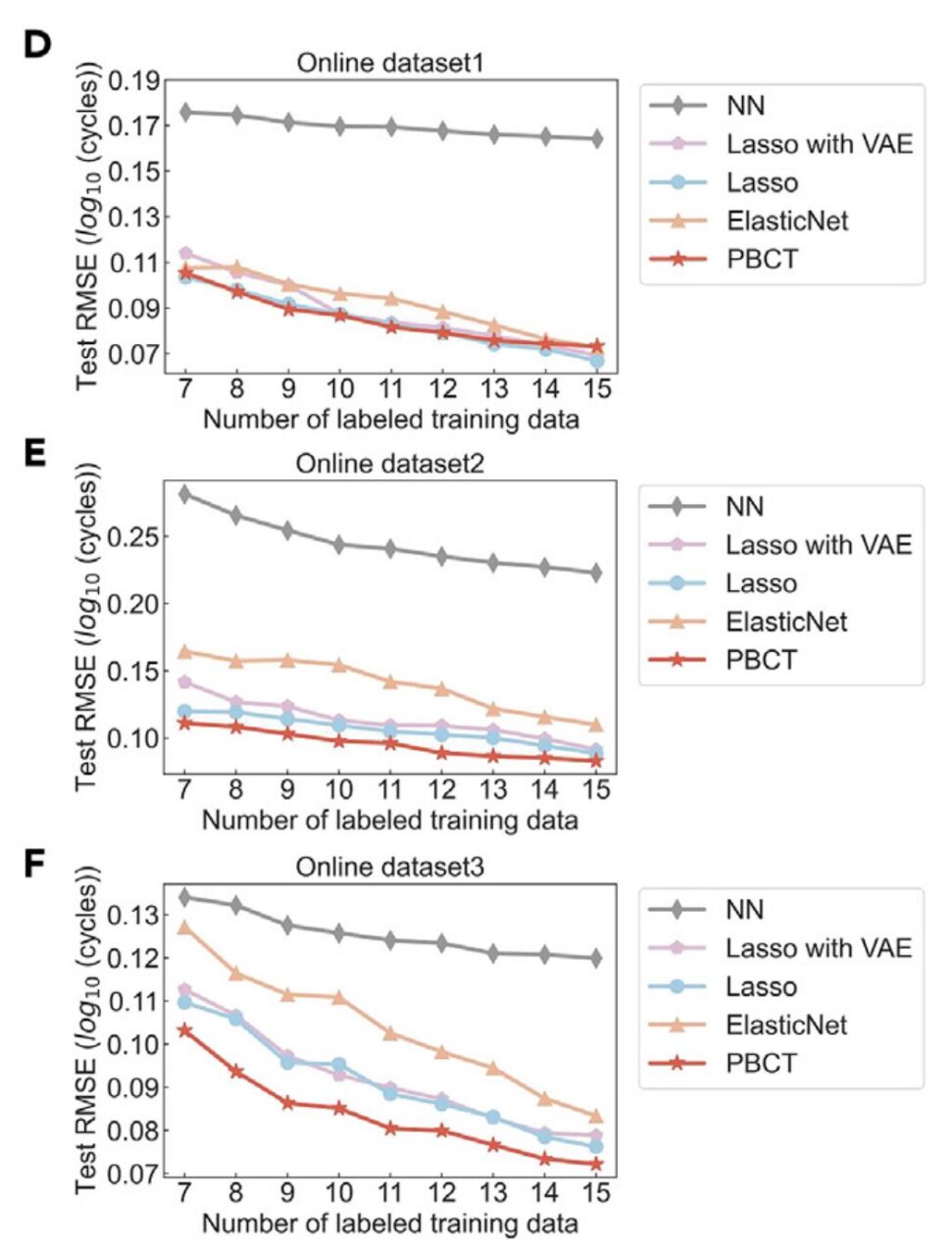
In the economic analysis experiment, in order to quantitatively demonstrate the advantages of the proposed PBCT method in battery life prediction,Based on the results of offline scenario experiments, the researchers analyzed the time and cost of data sampling required for each method.
The researchers estimated the median cycle cost for 200 repeated experiments and found that the PBCT method requires less labeled training data and lower cycle cost to achieve the same accuracy, as shown in Figures A to C below:

As shown in Figure D, to achieve the target accuracy RMSE% 0.10, PBCT requires lower cycle cost compared to the Lasso method. In dataset2, to achieve the target accuracy RMSE% 0.10, PBCT requires 7,700.5 cycles (RMSE is 0.099 when cycles is 7,700.5), while Lasso requires more than 10,537 cycles (RMSE is 0.105 when cycles is 10,537).
* Cycles refers to the number of times a battery goes from being fully charged to being fully discharged and then fully charged again, and is used to measure battery life.
* RMSE stands for Root Mean Square Error, also known as Root Mean Square Deviation, and is a commonly used measure of the difference between measured values, with lower values being better.
Therefore, applying the PBCT algorithm alone can save up to 28,36.5 cycles, or $4,685.1, compared to the Lasso method, which is equivalent to 26.9% of the total economic cost of using the Lasso method.
In summary, compared with existing methods, PBCT has achieved an improvement of up to 20% in life prediction accuracy, and almost no additional data collection costs are required. Therefore, under the premise of the same prediction accuracy, PBCT can significantly reduce the data collection costs.
In addition, the study found that incorporating unlabeled data into the training process helps reveal key factors that affect battery life, which are often overlooked when analyzing only labeled data. Therefore, PBCT, a semi-supervised learning technique, has opened up a new path for efficient and interpretable data-driven battery state estimation.
Shanghai Jiao Tong University's Deep Energy Laboratory continues to overcome difficulties
According to official reports, the research results mainly come from the research team of Shanghai Jiao Tong University Pu Yuan School of Future Technology and Deep Energy Laboratory.
The research direction of this research group is mainly energy storage devices and materials, advanced manufacturing, and their cross-integration with artificial intelligence.So far, more than 80 SCI papers have been published in internationally renowned academic journals in the field of energy and materials, such as Science, Nature, and Cell, with a total of more than 11,000 citations and an H-index of 48. The research results have been reported by many well-known media at home and abroad.
Associate Professor Wan Jiayu of the research group has also won the American Vacuum Society's National Doctoral Student Award "Dorothy M. and Earl S. Hoffman Award" (one person per year worldwide), the "National Outstanding Self-funded International Student Scholarship" issued by the China Scholarship Council, and Stanford University's annual list of the top 21 TP3T scientists in the world (2020-2022). He serves as a young editorial board member of National Science Open, Materials Today Energy, eScience, Carbon Energy, China Chemical Express, Rare Metals and other magazines.
In addition to the research results mentioned above, the research team has continued to delve deeper and overcome difficulties in the field of lithium batteries.
In May 2023, the team published a research paper titled "Self-Assembly Monolayer Inspired Stable Artificial Solid Electrolyte Interphase Design for Next-Generation Lithium Metal Batteries" in the internationally renowned journal Nano Letters.
Lithium metal is an ideal negative electrode for high-energy lithium-ion batteries, but its high reactivity and fragile interface limit its application - especially in commercial carbonate electrolyte systems, the performance of lithium metal is poor, which seriously hinders its widespread application in secondary batteries.This study proposed a simple and effective strategy to immerse lithium metal in 3-mercaptopropylmethyldimethoxysilane (3MPDMS) solvent to form a uniform, inorganic-rich SEI layer, which in turn enabled the uniform deposition/stripping of lithium metal for more than 500 cycles at low overpotential in a carbonate electrolyte.
In June 2023, the team released its latest work results on the ultra-fast recycling of positive electrode materials in the field of lithium-ion battery recycling.The ultrafast repair method they developed can efficiently and directly repair the positive electrode material (LCO) of used batteries within 8 seconds. The electrochemical performance of the repaired positive electrode material is comparable to that of the newly prepared positive electrode material.This research on the repair of lithium-ion battery positive electrode materials provides an efficient and energy-saving method for the renewable development and resource recycling of lithium-ion batteries.

Under the leadership of the leading team, advanced technologies represented by artificial intelligence will continue to deepen in the fields of energy and materials in the future, promoting the sustainable development of the industry.
References:
1.https://www.cell.com/joule/abstract/S2542-4351(24)00103-X
2.https://news.sjtu.edu.cn/jdzh/20240326/195130.html
3.https://www.x-mol.com/groups/deepenergy/news/44033
4.https://newsletter.x-mol.com/groups/deepenergy/news/46631