Accelerating Catalyst Design, He Yulian's Research Group at Shanghai Jiaotong University Automatically Extracts Knowledge Based on AutoML
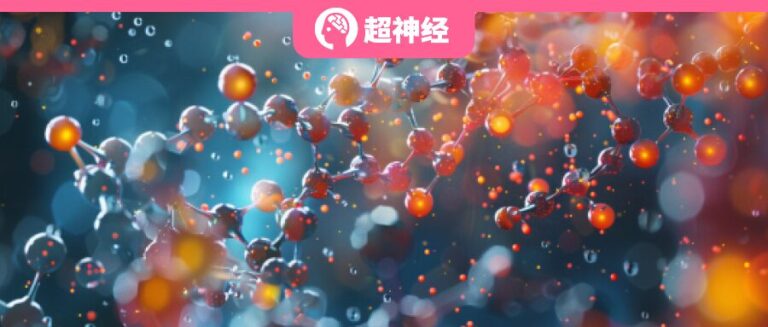
In daily life, "catalysis" is one of the most common chemical reactions. For example, the essence of wine and vinegar is the process of starch in grains being converted into alcohol and acetic acid under the catalysis of microbial enzymes.
To put it in more academic terms - a substance that can change the reaction rate of reactants in a chemical reaction (either increase it or decrease it) without changing the chemical equilibrium, and whose own mass and chemical properties do not change before and after the chemical reaction is called a catalyst.
In the chemical industry, processes above 85% all rely on catalysts to accelerate the reaction rate. The importance of designing new and efficient catalysts to the entire industry is self-evident.In the process of understanding and identifying the best catalyst, one of the most informative features is the chemical adsorption energy E of the reactants on the catalyst surface.ads The inherent complexity of chemical reactions makes it difficult to clearly determine Eads There are significant difficulties in determining the key physical quantities.
Recently, the research group of Assistant Professor Yulian He from the Joint Institute of Shanghai Jiao Tong University published a research paper titled “Interpreting Chemisorption Strength with AutoML-based Feature Deletion Experiments” in the top international comprehensive journal Proceedings of the National Academy of Sciences of the United States of America (PNAS).This study aims to determine theads A new method is proposed for the automatic extraction of knowledge from a high-throughput density functional theory (DFT) database based on feature removal experiments based on automated machine learning (AutoML).
Research highlights:
* Feature removal experiments based on automated machine learning (AutoML) to automatically extract knowledge from high-throughput density functional theory (DFT) databases
* The study demonstrates that the local geometric information of adsorption sites on the surface of binary alloy catalysts has an important influence on the chemical adsorption energy Eads The significant impact of AutoML feature removal experiments demonstrates the stability, consistency, and potential of
* This research result is of great significance in catalyst design optimization and has a significant impact on methodology

Paper address:
https://www.pnas.org/doi/10.1073/pnas.2320232121
Follow the official account and reply "Automatic Machine Learning" to get the complete PDF
High-quality datasets with rigorous science
A high-throughput density functional theory calculated dissociative chemisorption energy dataset was chosen as a benchmark for this study. The data quality was verified by reproducing the adsorption energies using the same DFT protocol suggested by Mamun et al.
This database contains DFT-calculated E values of various adsorbates on binary alloy surfaces.ads The researchers then selected chemical adsorption reactions involving more than 10 adsorbents from a dataset of 88,587 entries, retaining only five diatomic molecular adsorbents (H2 , O2 , N2 , CO and NO), as shown in the following table, totaling 8,418 entries.

The main purpose of restricting the adsorbents to diatomic molecules is to reduce the complexity caused by the adsorbent structure and unify the adsorbent description so that the machine learning model can focus on the surface behavior of the alloy involved (i.e., the catalyst).
Knowledge extraction methods guided by automatic machine learning (AutoML)
Previously, researchers tended to use machine learning (ML) methods, especially explainable artificial intelligence (XAI), to discover new insights about catalytic reactions. However, with the rapid development of AI technology in the field of chemistry, the models and specific feature explanations provided by XAI may not meet the level of clarity and certainty required by chemical researchers. Therefore,This study proposes an alternative, namely an automated machine learning (AutoML) guided knowledge extraction approach.As shown below:

Rather than delving into the inner workings of machine learning algorithms, the researchers bundled together many comparable machine learning models for collective analysis. Specifically, the researchers built their physics insights on a simple and fundamental principle — assuming that "critical" physical quantities should significantly affect the predictability of a physical model; therefore, removing these quantities would reduce the model's effectiveness, and vice versa.
first step, an initial benchmark feature set (Ftotal) is constructed and validated to ensure its descriptiveness, and models using this feature set should show acceptable predictive performance.
Step 2, remove internally correlated features from Ftotal to examine any changes in the predictability of the model.
This approach has three benefits:
1. Physical insights are gathered by comparing the performance of different sets of features, so physical considerations are explicitly incorporated. Through carefully designed experimental setups, changes in predictability can be linked to physical hypotheses;
2. Reduce the randomness of the model by analyzing the statistics of comparable models;
3. This approach avoids understanding the detailed mathematical structure of machine learning algorithms during the knowledge extraction process, thus avoiding the trade-off between model complexity and interpretability.
Research results: Local geometric information of adsorption sites is the key physical quantity
Through customized AutoML-based feature removal experiments,This study found that for binary alloy catalyst surfaces, the local geometric information of the adsorption site is the key factor determining the Eads The key physical quantity is not the intrinsic electronic or physicochemical properties of the alloy catalyst.
Specifically, the study combined feature removal experiments with the neural network-based explainable artificial intelligence (XAI) tool instantiated variable selection (INVASE) to summarize the prediction of Eads The optimal feature set for DFT, which contains 21 intrinsic physical quantities F21 that are inherent and not calculated by DFT, was used to achieve a mean absolute error (MAE) of 0.23 eV in about 8,400 chemical adsorption reactions on more than 1,600 alloy surfaces.
The table below shows the detailed information of F21, including 1 adsorbent feature, 3 geometric features, 7 physicochemical features, and 10 electronic features.

The researchers applied a proven feature removal method to Ftotal and determined the relative importance of the geometric, physicochemical, and electronic features of F21. The results are shown in the figure below: Removing electronic features from F21 resulted in ΔMAE ≈ 0.04 eV, making MAE = 0.30 eV, comparable to Ftotal.

Similar to Ftotal, although only three geometric features were selected, geometric information plays the most critical role in F21, as shown in Figure (b), with a ΔMAE of approximately 0.4 eV. Figure (c) shows that the removal of alloy physicochemical information from F21 has a greater impact than electronic features (ΔMAE ≈ 0.15 eV). In particular, the researchers found that a specific feature of alloy component B, atomic radius B, is particularly important. Regardless of the order of deletion, a ΔMAE of approximately 0.1 eV was observed when atomic radius B was removed. The importance of atomic radius B may be related to the "ligand" or "strain" effect in bimetallic nanocrystals. The introduction of a second metal B into the main metal matrix A may cause significant changes in the electronic state and/or lattice strain (compression or tension), thereby affecting the chemical adsorption intensity.
As summarized in Figure (d) above, the relative importance found on F21 is ranked as geometric > physicochemical > electronic, consistent with the findings of Ftotal.
In summary, this study demonstrates that the local geometric information of adsorption sites on the surfaces of binary alloy catalysts has a significant influence on the chemical adsorption energy Eads The results show the stability, consistency and potential of AutoML-based feature removal experiments. Compared with traditional interpretable models, this method avoids the trade-off between model complexity and interpretability, shifts the source of scientific insights from elucidating model behavior to evaluating feature set performance, minimizes the impact of human interference on conclusions, and extracts knowledge from the statistical behavior of the output.
This newly proposed AutoML-based feature analysis method is a powerful and flexible tool for revealing the importance of statistical features in complex physical sciences, even beyond the field of catalysis.
Catalysis towards an efficient future
Designing new catalysts is the key to solving many energy and environmental challenges. However, on the one hand, many catalytic reactions involve complex reaction mechanisms, including the generation and transformation of multiple intermediates and transition states, which may be affected by multiple factors, such as solvents, temperature, pressure, etc., making it very difficult to predict and understand the performance of catalysts; on the other hand, due to the complexity and uncertainty of catalyst synthesis, the cost of trial and error is often high, and traditional methods may require trying a variety of different materials and reaction conditions, which increases the time and cost of catalyst development.
In order to overcome these challenges and improve the design efficiency and performance of new catalysts, artificial intelligence technology needs to be introduced. Artificial intelligence can use big data and machine learning algorithms to analyze complex catalytic reaction mechanisms and accelerate the design and optimization process of catalysts. For example:
* Crystal structure prediction and design:Artificial intelligence can be used to predict and design the crystal structure of catalysts, thereby improving catalytic performance. In the past, scientists looked for new crystal structures by adjusting known crystals or testing new combinations of elements. Now, technologies such as deep learning can analyze large amounts of crystal structure data and discover patterns and trends to guide catalyst design.
* Chemical reaction prediction and optimization:Artificial intelligence can help predict the products and reaction pathways of chemical reactions and optimize reaction conditions to achieve the desired catalytic effect. For example, by training neural network models, scientists can establish a predictive model of the reaction mechanism and use it to guide experimental design.
* High-throughput material screening:Artificial intelligence can accelerate the high-throughput materials screening process and quickly identify candidates with potential catalytic properties from a large number of candidate materials.
* Intelligent experiment design and optimization:Artificial intelligence can help design and optimize experimental plans to maximize the synthesis efficiency and performance of catalysts. By combining machine learning and automated experimental technology, an intelligent experimental platform can be built to automatically execute experimental processes and adjust and optimize based on real-time data.
For example, in September 2023, researchers at Hokkaido University demonstrated an extrapolated machine learning approach to develop new multi-element reverse water-gas shift catalysts. Using 45 catalysts as initial data points and performing 44 cycles of a closed-loop discovery system (ML prediction + experiment), the researchers experimentally tested a total of 300 catalysts and identified more than 100 catalysts that had superior activity compared to previously reported high-performance catalysts.
The research, titled "Accelerated discovery of multi-elemental reverse water-gas shift catalysts using extrapolative machine learning approach," was published in Nature Communications.

In the future, artificial intelligence is expected to further improve the design and synthesis efficiency of catalysts, accelerate the discovery and application of new catalysts, and thus promote the development of the field of chemistry.
References:
1.http://www.sdqiying.com/cxinwenz/469/
2.https://www.zhihuiya.com/newknowledge/info_2859.html
3.https://www.ceshigo.com/article/11511.html
4.https://www.jiqizhixin.com/articles/2023-10-21-19