Blood Routine Tests, Urine Tests and Other Indicators Can Identify Ovarian Cancer! Liu Jihong's Team From Sun Yat-sen University Led the Team, and Four Major Medical Schools Jointly Built an AI Fusion Model
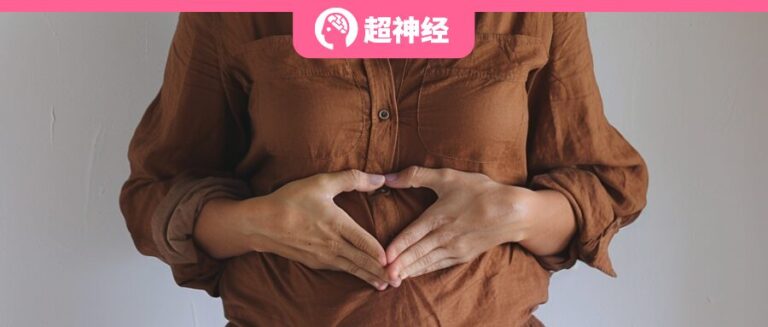
According to the "Guidelines for the Diagnosis and Treatment of Ovarian Cancer (2022 Edition)" issued by the National Health Commission, the annual incidence of ovarian cancer in my country ranks third among female reproductive system tumors, second only to cervical cancer and uterine body malignant tumors, and the mortality rate ranks first among female reproductive tract malignant tumors. Its 5-year survival rate is closely related to the stage of the disease at the time of diagnosis. According to data released by the National Cancer Institute of the United States, in the early local infection stage, the 5-year survival rate of ovarian cancer is 92.4%, while the 5-year survival rate in the metastatic stage drops to 31.5%.
The ovaries are deep in the pelvic cavity, and ovarian lesions are often asymptomatic in the early stages. When symptoms appear, patients with 70% are already in the late stages. Therefore, early diagnosis of ovarian cancer is of great significance.

Recently, Professor Liu Jihong's team from the Department of Gynecology at the Cancer Prevention and Treatment Center of Sun Yat-sen University, in collaboration with Southern Medical University, Tongji Hospital affiliated to Tongji Medical College of Huazhong University of Science and Technology, and Obstetrics and Gynecology Hospital affiliated to Zhejiang University School of Medicine, has conducted a study to address the current difficulties in early diagnosis of ovarian cancer and the lack of effective tumor markers.An artificial intelligence fusion model MCF for ovarian cancer diagnosis was constructed, and the risk of ovarian cancer can be calculated by inputting routine laboratory test data and age.The relevant results have been published in The Lancet Digital Health.

Paper address:
https://doi.org/10.1016/S2589-7500(23)00245-500245-5/fulltext#%20)
Follow the official account and reply "ovarian cancer" to get the full paper
Research highlights:
* The study collected data from three hospitals in my country and used a multi-criteria decision-making classification fusion (MCF) framework to develop the model
* The model is more accurate in identifying ovarian cancer than traditional biomarkers such as CA125 and HE4
* Study demonstrates the potential of a low-cost, easily available, routine laboratory test to be an effective diagnostic tool for ovarian cancer
Bringing together 3 hospitals, 10,000 patients, 98 related examinations and data
The researchers collected data from the Cancer Center of Sun Yat-sen University, Tongji Hospital affiliated to Tongji Medical College of Huazhong University of Science and Technology, and the Obstetrics and Gynecology Hospital affiliated to Zhejiang University School of Medicine between January 1, 2012 and April 4, 2021.Data on 98 laboratory tests and clinical characteristics of more than 10,000 patients (women with ovarian cancer and benign uterine adnexal lesions/normal physical examination).
The data of participants from Tongji Medical College of Huazhong University of Science and Technology (3,007 people in total) were selected as the training set, and five-fold cross-validation was performed on the training set. The two external validation sets were from Women's Hospital of Zhejiang University School of Medicine (5,641 people in total) and Cancer Center of Sun Yat-sen University (2,344 people in total).
MCF: Fusion of 20 base classification models

The study recruited a large number of participants from three different regions of my country. The median age of ovarian cancer diagnosis in the three cohorts was 51-56 years old. However, large-scale data also brings some problems. For example, multi-center data are heterogeneous, which is not conducive to building a robust artificial intelligence model, and there are some defects, including a significant imbalance between the number of ovarian cancer patients and control participants, inconsistent units, and a large number of missing values (48.5% for the internal validation set).
In order to solve these data problems and ensure the robustness of the model,The researchers did a lot of data cleaning work.include:
* When building the model, 98 laboratory test items were listed as candidate input features. For laboratory test items with different units, the units were unified.
* Missing data were imputed using the MICE (multivariate imputation by chained equations) algorithm.
* To reduce the differences in data distribution among institutions, the Box-Cox algorithm was used to reconcile the data, and then the data were normalized by min-max standardization.
* In order to solve the problem of data imbalance, an adaptive comprehensive sampling method is used with a balancing ratio of 0.5.
In addition, the MCF framework is a variant of the H-MCF (Hierarchical Prediction Scheme Based on MCF) proposed by the research team in previous work.The researchers established 176 basic classification models and combined the feature selection method with the machine-learning classifier. Through five-fold cross-validation, they selected the top 20 basic classification models from the 176 models.Among them, the feature selection process will identify the 20 most important features for the classifier to use to generate a basic classification model.
The researchers then estimated the weight of each model based on multi-criterion decision-making theory and finally fused their predictions to reach a consistent classification.

The model performance is significantly higher than traditional methods
The researchers quantified the prediction accuracy of the MCF model using AUC, accuracy, specificity, sensitivity, positive predictive value, negative predictive value, and F1 score. The results are shown in the figure below:

The researchers selected 52 features (51 laboratory test indicators and age) from the top 20 basic classification models, of which about 90% features were significantly associated with the risk of ovarian cancer, and constructed similar consistent feature rankings based on SHAP (Shapley Additiveexplanation, a technique for evaluating and explaining model predictions). The prediction AUCs of individual features ranged from 0.477 (AFP) to 0.886 (CA125), and were generally consistent with their rankings.
in,51 laboratory test indicators include routine blood tests, urine tests, biochemical tests, etc.For example, platelet PLT, fibrinogen determination FIB, CRP to assess the degree of inflammation, ALB to measure serum albumin level, erythrocyte sedimentation rate ESR, urine pH value, etc. The following figure A shows the importance of the test indicators.

The AUCs of MCF on the internal validation set and two independent external validation sets were 0.949 (95%CI 0.948-0.950), 0.882 (0.880-0.885), and 0.884 (0.882-0.887), respectively.
The researchers also compared MCF with traditional tumor markers in ovarian cancer detection.For all three validation sets for distinguishing ovarian cancer, the AUC, sensitivity, and F1 score of MCF were higher than those of traditional tumor markers.As shown in the following figure:

For the classification of advanced ovarian cancer and control groups, MCF achieved an AUC of 0.985 in the internal validation set, 0.972 in the first external validation set, and 0.943 in the second external validation set. For the classification of early ovarian cancer and control groups, MCF achieved an AUC of 0.879 in the internal validation set, 0.823 in the first and second external validation sets, and 0.810 in the first and second external validation sets, respectively.
The results showedThe AUC and sensitivity of the MCF model for identifying ovarian cancer patients, especially those in early stage ovarian cancer, were significantly higher than those of traditional ovarian cancer markers CA125, HE4 and their combination.Moreover, the risk of ovarian cancer can still be predicted accurately in a population where some indicators are missing, which shows that the MCF model has good stability and good compatibility with real-world data.
In addition, this study also found that in addition to tumor markers, other routine laboratory tests, such as DD dimer and platelet count, also made a significant contribution to the diagnosis and prediction of ovarian cancer, suggesting that the pathophysiological processes related to these test indicators may play an important role in the development of ovarian cancer, and their potential mechanisms deserve further exploration.
AI empowers primary healthcare development
According to the "Statistical Bulletin on the Development of my country's Health Care Industry in 2022", although there are 979,768 primary medical and health institutions in my country, accounting for 94.85% of the total number of medical and health institutions in the country, in terms of the number of diagnosis and treatment, the number of diagnosis and treatment in primary medical and health institutions is 4.27 billion, accounting for only 50.7% of the total annual diagnosis and treatment. It can be seen that the number of primary medical and health institutions in my country accounts for a high proportion, but the number of diagnosis and treatment still has a lot of room for growth.
In addition, according to statistics from the National Cancer Center, in my country's cancer medical service market, public tertiary hospitals undertake more than 80% of cancer treatment tasks. Most of these tertiary hospitals are located in provincial capitals, but they have to receive patients from all over the world at the same time, and the pressure on doctors can be imagined.
However, the mature application of artificial intelligence in recent years has brought endless imagination to the medical industry and provided new ideas for primary care. The ovarian cancer diagnosis model MCF constructed in this study has been open sourced. The risk value of ovarian cancer can be calculated by inputting the corresponding laboratory test data and age, which undoubtedly provides important support for the popularization of this model in primary medical institutions.
The deployment of AI-assisted diagnosis in primary health care institutions is extremely important. The "Opinions on Further Deepening Reforms to Promote the Healthy Development of Rural Medical and Health Systems" previously issued by the State Council also mentioned the need to accelerate the deployment and application of AI-assisted diagnosis in rural medical and health institutions.
The application of artificial intelligence in primary health institutions can not only process medical information into structured data, solve the problems of "data islands" and data quality, and lay the foundation for the interconnection and sharing of medical information in the region; it can also improve the level of primary diagnosis and treatment through auxiliary consultation, auxiliary diagnosis, chronic disease management and other functions, reduce the probability of missed diagnosis and misdiagnosis, and allow more places to enjoy high-quality diagnosis and treatment.
References:
1.https://www.sysu.edu.cn/news/info/2331/1091611.htm