Better Than the Five Advanced Models, the GNNWLR Model Proposed by Du Zhenhong's Team at Zhejiang University: Improving the Accuracy of Mineralization Prediction
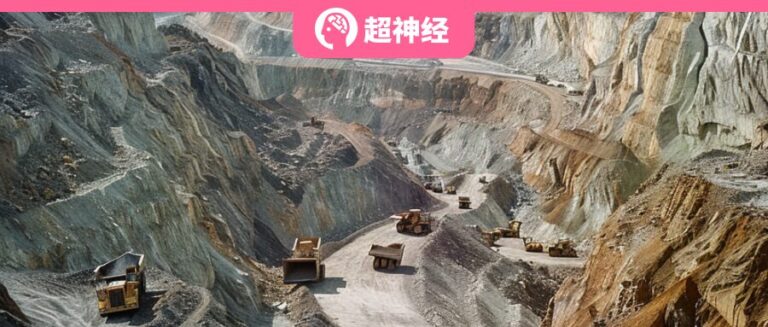
Qatar World Cup has invested about 229 billion US dollars since it was awarded the right to host the event in 2010 and will be held successfully in 2022. In comparison, the total cost of the previous seven World Cups was only about 40 billion US dollars. The extravagant style of this sporting event is ultimately due to the profound heritage of Qatar. As the saying goes, "If you have a mine at home, you will not be worried." Qatar is able to spend money freely to create a feast of audio-visual feasts with its rich mineral resources.
Mineral resources, for individuals, mean inexhaustible wealth, which is fascinating; for society, they are an important pillar of economic and social development, and are related to national economy, people's livelihood and national security. However, mineral resources are not easily available. They are hidden hundreds of meters underground, and it often takes a lot of hard work to explore and find the precious mineral resources.
With the continuous development of mineral exploration, the industry has gradually formed a research route guided by "ore-forming system-exploration system-prediction and evaluation system". Although artificial intelligence plays an increasingly important role in the mineral resource prediction and evaluation (MPM) system, its application still has certain limitations, which often makes it difficult for geologists to believe the final results.
In order to improve the interpretability of mineralization prediction models and the spatial non-stationarity caused by geological factors in the mineralization process,A research team from Zhejiang University proposed a new geospatial artificial intelligence method - geographically neural network-weighted logistic regression (GNNWLR).
The model integrates spatial patterns and neural networks, and combined with Shapley's additive interpretation theory, it can not only significantly improve the accuracy of predictions, but also improve the interpretability of mineral predictions in complex spatial scenarios.

Research highlights
* A geographic neural network weighted logistic regression model GNNWLR is proposed
* GNNWLR outperforms other advanced models in mineral resource prediction and evaluation
* GNNWLR overcomes spatial heterogeneity and nonlinear effects
* GNNWLR improves the interpretability of artificial intelligence for mineralization mechanisms

Paper address:
https://doi.org/10.1016/j.jag.2024.103746
Follow the official account and reply "ore-forming prediction" to get the complete PDF
Competing for the global MPM test site: Meguma, Nova Scotia, Canada
The study focuses on the Meguma Group, which covers an area of about 7,800 square kilometers in western Nova Scotia, Canada, and is mainly covered by grasslands and forests. The Meguma Terrane consists of two layers of strata, the lower layer is the Goldenville Group, which is mainly composed of metamorphic sandstone. The upper layer is the Halifax Group, which is composed of shale complex rocks.
Due to the Acadian orogeny and the emplacement of Devonian granites, a series of NE-SW trending fold structures have been formed in the area, which has become a testing ground for a variety of mineral resource prediction and evaluation systems.

There are 20 turbidite gold deposits in the study area.The study used six characteristic layers, including anticline structural factors, contact factors between the Goldenville and Halifax formations, and chemical elements such as copper (Cu), lead (Pb), arsenic (As) and zinc (Zn).
Among them, the study conducted a multiple-ring buffer analysis on the anticline and the contact surface between the Goldenville and Halifax formations, assigning corresponding weights at intervals of 0.5km, forming a total of 16 buffer rings. At the same time, the study also performed inverse distance weighted interpolation (IDW) on 671 lake sediment samples containing chemical elements. Finally, the study gridded the entire study area and unified all feature layers into 1km x 1km grid data.
Since 20 positive samples are still too small relative to the entire study area, the ratio of positive and negative samples is prone to imbalance. Therefore, the study also analyzed the buffer zone, divided the 20 positive sample points into a 2km radius, and then performed raster analysis.
Finally, the study obtained a total of 245 positive samples, indicating the presence of mineral deposits, and the others indicated the absence. At the same time, the study randomly selected the same number of data as the positive samples from the negative sample set and merged them with the positive samples to create the training set and validation set.
GNNWR Model: Efficient Ensemble of Neural Networks
Since the model structure of geographic neural network weighted regression (GNNWR) uses mean square error, directly applying it to mineral resource prediction and evaluation may bring convergence challenges. Studies have shown that cross entropy has more practical advantages than mean square error. Therefore,The study used a loss function specifically designed for logistic regression - binary cross-entropy (BCE).
In this context, GNNWLR first calculates the distance between the spatial coordinates of each data point and the spatial coordinates of other data points in the training dataset, and uses this as input to perform dropout regularization and prevent overfitting.
Secondly, the study performed a dot product operation on the spatial weight vector output by the neural network, the coefficients obtained by the least squares method, and the values of the independent variables, and then applied the logistic regression function to generate the final predicted value.
Finally, the study used a binary cross entropy loss function to calculate the loss relative to the actual value and used it to guide the neural network to perform negative feedback adjustments.

In this study, the researchers also compared the most common geographically weighted regression (GWR), support vector machine (SVM), random forest (RF), geographically weighted logistic regression (GWLR), geographically weighted support vector regression (GWSVR), and random forest (GWRF) models.
Specifically, the study used five-fold cross-validation to randomly divide 20 deposits into 5 sequences, each with 4 deposits, and then performed a buffer analysis with a buffer radius of 2km on these 4 deposits to obtain positive samples for each sequence. Similarly, the study also randomly selected negative samples from the negative sample pool by matching the number of positive samples, and each negative sample appeared only once in the five-fold cross-validation.
According to the five-fold cross-validation theory, four sample sets are used for training and one sample set is used for validation. This process is repeated five times, with each sequence serving as a validation set. Finally, the training set and validation set obtained by the five-fold cross-validation are merged.
From the results,Due to the effective integration of neural networks in the GNNWLR model, GNNWLR significantly outperforms other models and shows excellent fitting and prediction capabilities in mineral classification, with an AUC of 0.913, which is 5%-16% higher than other models.Meanwhile, GWRF and GWSVR are also significantly better than RF and SVM, which may be because they are both combined with geographically weighted regression (GWR), which can more accurately describe the local relationship between spatial variables.

The MPM plots for all models also intuitively show that the mineral prospectivity of Nova Scotia varies greatly in space, with the Northeast region scoring higher overall, consistent with the actual location of the deposits.When faced with gold resources far away from concentrated areas, GNNWLR can discover more deposits that are easily overlooked by other models.
For example, the score of GNNWLR in “Region 1” is as high as 0.985, while the corresponding scores of GWSVR, GWRF, GWLR, SVM, RF and GWR models are only 0.288, 0.438, 0.471, 0.133, 0.383 and 0.290.

In addition, the RF and SVM models exhibited sudden jumps in “Region 2” and “Region 3”, which affected their accuracy and reliability. The GNNWLR, GWLR, and GWR models take into account the spatial proximity and heterogeneity of mineralization factors, which can prevent the occurrence of sudden changes commonly seen in traditional machine learning models. It was observed that GNNWLR exhibited excellent ability in capturing the complex nonlinear relationships between these factors, especially those associated with spatial variation.
therefore,GNNWLR demonstrates a relatively seamless transition in predicting mineral prospectivity, showing higher accuracy and consistency with empirical data.
SHAP can quantitatively analyze the factors affecting mineralization
To improve the interpretability of model evaluation, this study integrated and used the positive sample set of the entire dataset to calculate the mineral prospect characteristics of relevant locations in GNNWLR.
The results show that As has the greatest impact on the model output and is positively correlated with the SHAP value. The larger the As value, the higher the SHAP value and the greater the possibility of mineralization. This may be because As is a low-temperature hydrothermal element and is often associated with gold deposits. Similarly, Zn has a negative impact on many mining areas, while Cu has the least significant impact. Among them, As and Pb are low-temperature hydrothermal elements associated with minerals such as realgar and galena, and Zn and Cu are medium-temperature hydrothermal elements that form minerals such as sphalerite and chalcopyrite.In summary, the formation of gold deposits in this area is closely related to low-temperature hydrothermal processes.

By evaluating the impact of mineralization with different characteristics in different areas, the study found that the mineralization in "Area 4" is strongly correlated with anticlines and Pb, and there are two mineral deposits in "Area 5". The northern deposit is positively affected by the four elements Cu, Pb, Zn, and As, indicating that the low area has both medium-temperature hydrothermal and low-temperature hydrothermal mineralization; the southern deposit is positively affected by Zn and As, indicating that medium-temperature hydrothermal fluids are the main ones.
Combined with the drilling data of the Nova Scotia Department of Natural Resources, there are 39 geological drilling records related to gold in the northern deposits of "Region 5", involving a variety of low-temperature and medium-temperature hydrothermal minerals, and only 4 geological drilling records related to gold in the southern deposits of "Region 5". The deposit area mainly contains medium-temperature hydrothermal minerals such as sulfides and arsenopyrite. The mineralization of "Region 6" is closely related to the anticline contact, which also confirms the inference of the mineralization type based on the spatial distribution of SHAP values.

In summary, the model based on SHAP value can quantitatively analyze various factors affecting mineralization results in the entire spatial domain, has excellent interpretability and is consistent with the principles of earth science.At the same time, the study also compared the spatial distribution of SHAP values and regression coefficients. The results show that the spatial distribution of regression coefficients does not completely conform to geological laws. Therefore, SHAP values are more meaningful than traditional regression coefficients and are easier for scholars to refer to.

Professor Du Zhenhong of Zhejiang University: Focusing on spatiotemporal big data and artificial intelligence research
The research team led by Professor Du Zhenhong of the School of Earth Sciences at Zhejiang University has long been engaged in scientific research in remote sensing and geographic information systems, spatiotemporal big data and artificial intelligence, and has achieved a series of results in the basic theory and key technology research of spatiotemporal big data analysis in the fields of geography, ocean, geological disasters, etc. He is leading the team to fully integrate GIS, remote sensing, computer science with geography, ocean, geology, etc., and explore a new chapter in the development of data-driven earth science.
References:
1.https://www.zast.org.cn/art/2022/12/8/art_1675105_58963288.html