New Breakthrough in Synthetic Biology! Luo Xiaozhou's Team From the Chinese Academy of Sciences Developed the ProEnsemble Machine Learning Framework: Optimizing the Combination of Promoters in Evolutionary Pathways
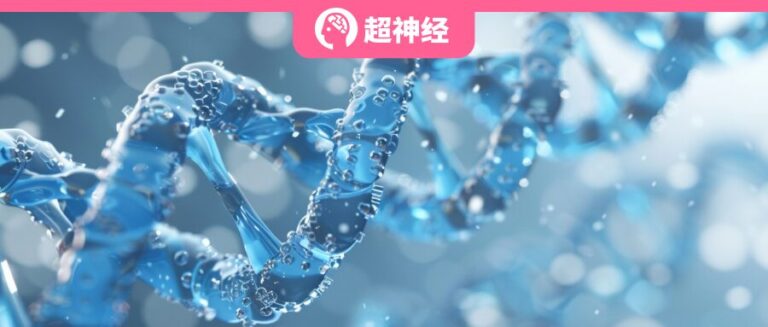
In the field of synthetic biology, researchers introduce enzyme genes from other organisms into a host and construct new metabolic pathways that allow the host to produce substances that it cannot synthesize on its own. This has been proven and widely used in the production of compounds such as biofuels, high-value chemicals and anti-cancer drugs.
However,The evolution of the above-mentioned metabolic pathways is not smooth, and an important limiting factor is the gene epistasis effect.
Geneticist Daniel Weinreich once said that the epistatic effect of genes is similar to when the effect of a single mutation is known, but the combined mutation produces an "unexpected surprise." Specifically, epistatic genes can inhibit the functional expression of a specific gene, which makes some gene mutations that help optimize metabolic pathways unable to function, causing uncertainty in the evolution of metabolic pathways.
Under natural conditions, due to the existence of gene epistasis, a slight modification of one enzyme may cause another enzyme to hinder the development of a metabolic pathway, resulting in a longer period of time for the enhancement of metabolic function or the discovery of new functions.Therefore, how to quickly achieve the effects required by thousands of years of natural evolution in a shorter time and with fewer iterations has always been a difficult point in research in this field.
To address the above problems, Luo Xiaozhou's team from the Institute of Synthesis, Shenzhen Institute of Advanced Technology, Chinese Academy of Sciences, used automated large-facility platform technology to determine a controllable evolutionary trajectory and achieved automatic and synchronous evolution of multiple key genes in metabolic pathways.At the same time, the ProEnsemble machine learning framework is combined to optimize promoter combinations, alleviate the impact of gene epistasis in evolutionary pathways, and create an efficient universal chassis.
Research highlights:
* Integrate the advantages of automation and machine learning to improve the speed and efficiency of chassis development, shorten the R&D cycle and reduce costs.
* Provides cutting-edge technology routes and new solutions for the field of biological intelligent manufacturing.

Paper address:
https://onlinelibrary.wiley.com/doi/full/10.1002/advs.202306935
Follow the official account and reply "metabolic pathway" to get the complete PDF
Automated platform accelerates synchronous evolution of metabolic pathways

This study proposed a pathway bottleneck design and solution strategy, taking naringenin as an example:
In the first stage, we used automated large-scale facility platform technology to allow the genes related to the synthesis of naringenin to be expressed at a low level (low copy number background), thereby constructing an artificial metabolic bottleneck for the synthesis of naringenin.
In the second stage, candidate mutants 4CL-11C1 and CHS-9H9 were screened for naringenin production comparable to that of the original mutant, thus eliminating the bottleneck of the naringenin pathway.
In the third stage, through artificial intelligence-mediated promoter engineering, mutants of single genes are placed back into the original pathway and the metabolic flux is balanced.
Our results demonstrate that, within the confines of a clear trajectory, artificial bottleneck creation and resolution strategies can enable efficient evolution of metabolic pathways, further confirming that epistatic effects may limit the boundaries of pathway evolution.
In addition, directed evolution of the three enzymes corresponding to the key gene of naringenin may induce an imbalance in the metabolic pathway. To this end, the researchers used the machine learning framework ProEnsemble to optimize the promoter combination of the evolutionary pathway, further optimize the expression of enzymes in each pathway, and increase the production of naringenin.
Dataset: Historical public data screening
Dataset 1:The researchers screened 42 reported promoters with a wide dynamic range from the literature, and finally screened 12 promoters with significant differences in strength and divided them into three categories: high strength, medium strength and low strength.

The PT7 promoter is a positive promoter, and the PBAD promoter is a negative promoter.
Dataset 2:The researchers screened about 1,000 mutants that could produce high naringenin concentrations using the Al3+ signal detection method and collected a balanced data set from them. Subsequently, 108 mutants with Al3+ signals above 0.2 were selected as high-yield representatives, and 50 samples with Al3+ signals below 0.2 were randomly selected, for a total of 158 mutants. Among them, the naringenin production of Top1's NAR1.0 strain was 4.44 times higher than that of the control group.
Model architecture: ProEnsemble optimized promoter combination
The researchers proposed a promoter combination prediction framework called ProEnsemble, which aims to establish the relationship between different promoter combinations and naringenin production, that is, encoding 12 different types of promoters, and the corresponding output is naringenin production.

Specifically, the root mean square error (RMSE) of 13 conventional predictors was evaluated by performing ten-fold cross validation on the above dataset containing 158 mutants.
Subsequently, through forward model selection, the predictors with the smallest errors are integrated in turn, and the integrated model with the smallest RMSE is selected as the final prediction model.The best model is a combination of Gradient Boosting Regressor, Ridge Regressor and Gradient Boosting.
The research results showed that the naringenin production of the top 5 strains predicted by the ProEnsemble model was higher than 700 mg/L, which was more efficient and accurate than random sampling (5 high-yield strains in 960 samples).
However, the unbalanced distribution of the dataset may limit the predictive ability of the model, resulting in the yield of the top 5 strains not exceeding that of the NAR1.0 strain.
Model optimization: Balanced distribution of data to enhance model performance
The researchers further expanded the training set from another 1,500 clones and optimized the model using data sets with naringenin levels above 400, 500, 600, 700, and 800 mg/L.

Finally, after adding 27 datasets with concentrations above 600 mg/L to the initial dataset, the model performed best, and the Pearson correlation coefficient (PCC) increased from 0.74 to 0.82. The results showed the importance of balanced distribution of datasets to enhance model performance.

By testing the naringenin production in different strains, the researchers found that the top 5 strains predicted in the second round can all efficiently synthesize naringenin. The highest yield of NAR2.0 is 1.21 g/L, which is 16% higher than NAR1.0.5.16-fold higher than the initial construct without promoter optimization.
It is worth noting that more than 99.11% strains in the random promoter library had a yield of less than 1 g/L, which indicates that the ProEnsemble integrated model has the potential to mine high-yield strains.
Experimental conclusion: Universal chassis can efficiently synthesize flavonoids

In order to further verify the feasibility of the scheme proposed by the institute, the researchers achieved the efficient synthesis of flavonoids such as genistein, sakuratin and hesperidin through the naringenin chassis. The yield of genistein reached 72.32 mg/L, the yield of sakuratin was 223.39 mg/L, and the yield of hesperidin was 82.50 mg/L. The yield of each flavonoid was higher than the level reported in the literature, which provides a new idea for the production of high value-added compounds.
China’s synthetic biology industry is still in its infancy
In recent years, developed countries such as Europe and the United States have taken measures to promote the development of synthetic biology and its related manufacturing industries. The Chinese government has also attached great importance to this field and listed synthetic biology as a disruptive technology that will lead my country's industrial transformation. The optimization of metabolic pathways closely related to it has become a hot topic of concern for more and more researchers.
In the context of the AI and big data era, the automated learning, flexibility, and powerful data processing capabilities of machine learning technology have provided new directions for the optimization of metabolic pathways and brought new vitality to synthetic biology.
In fact, there are already pioneers in this emerging industry in China. The author of this article, Luo Xiaozhou, founded a company dedicated to the research and development of synthetic biology technology in 2019 - Senruis Biotech (Shenzhen) Co., Ltd. The company uses big data and AI technology for biosynthesis, and with the scientific research resources of colleges and universities, it has quickly developed and implemented some high-value-added product pipelines, successfully overcome the difficulties of many synthetic biology production processes, and completed the construction of chassis cells for subdivided categories.
In addition, in January this year, Dr. Luo Xiaozhou's team also proposed an enzyme kinetic parameter prediction framework EF-UniKP, which is based on a pre-trained large language model and a machine learning model, and achieves accurate prediction of enzyme kinetic parameters and efficient mining of specific enzymes. It is understood that the research team is currently developing further cooperation with Senruis Biotechnology (Shenzhen) Co., Ltd., which is expected to promote the implementation and transformation of this technology. (Click here for details: Luo Xiaozhou's team from the Chinese Academy of Sciences proposed the UniKP framework, a large model + machine learning to predict enzyme kinetic parameters with high precision)
It can be said that Dr. Luo Xiaozhou has perfectly implemented the "integration of industry and research". While deepening his research on synthetic biology, he is also promoting the implementation of excellent results in the industry. Facing the booming development of the global synthetic biology industry, Luo Xiaozhou said that although my country has made initial achievements in the synthetic biology industry, it is still in its infancy. Therefore, further strengthening the research and development of core technologies and ensuring the deep integration of scientific research results and industrial practice are the key to narrowing the gap between my country and developed countries in the synthetic biology industry.
References:
1.http://cn.chinagate.cn/news/2018-11/16/content_72414672_2.htm
2.https://new.qq.com/rain/a/20230918A03TY700
3.https://sheitc.sh.gov.cn/dsxxjyzl/20231129/7321884958b14651abeac020f7802f8b.html
4.https://www.develpress.com/?p=4755
5.http://www.isynbio.org/news-detail.aspx?detail=8217&parm=1772
6.https://www.cn-healthcare.com/article/20221028/content-574249.html
7.https://isynbio.siat.ac.cn/view.php?id=814