AI Becomes the "key Man" in Football Games: DeepMind Releases TacticAI, With Tactical Layout Practicality up to 90%
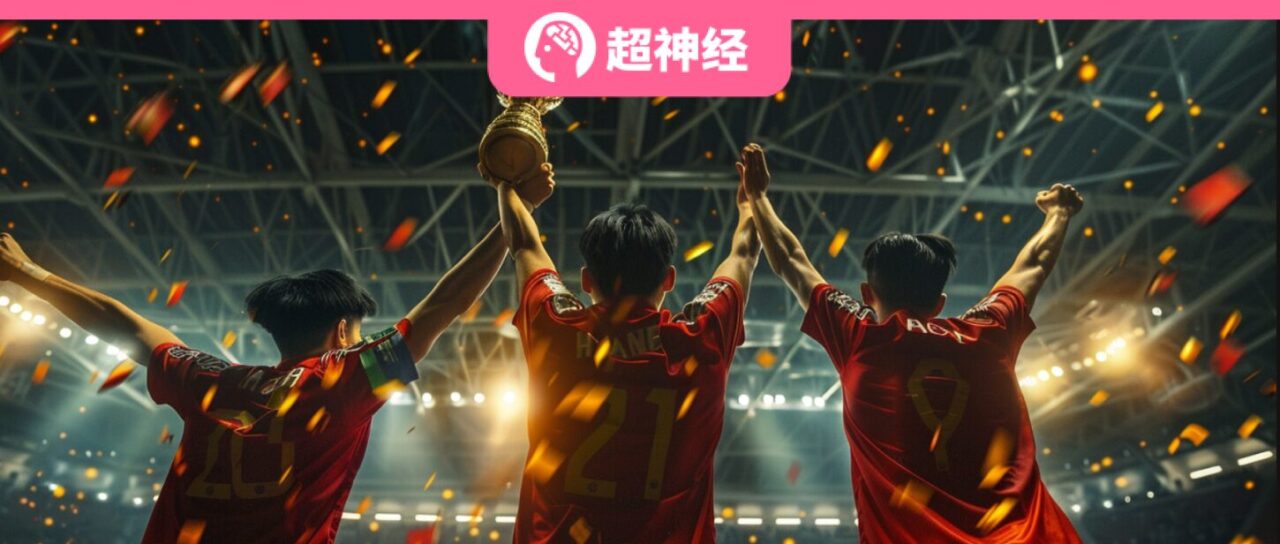
In the just-concluded World Cup qualifiers, the Chinese national football team defeated Singapore 4:1 at home in Tianjin, sweeping away the haze of being tied by the opponent in the previous game when they were leading, and also ushered in the team's first victory in 2024. At present, the Chinese team is temporarily ranked second in Group C, retaining the hope of advancing to the top 18.
While enjoying the joy of victory, some fans also reviewed the game data and found that the ratio of corner kick opportunities obtained by both sides in the entire game was 10:1. The Chinese national team was far ahead, but failed to successfully score a goal. Instead, it missed an empty goal, which was really regrettable.

In fact, "set pieces" such as corner kicks can provide players with a brief interruption in high-intensity running confrontations. Deploying troops in the opponent's penalty area is often an excellent opportunity to implement the coach's tactics. Many classic games in football history have confirmed its importance.
On May 7, 2019, the second leg of the Champions League semi-final was held at Anfield. After a huge 0:3 deficit in the first half, Liverpool scored three goals in a row, and finally Arnold's unexpected corner kick assisted to complete the winning goal.
At that time, Arnold started to walk away from the corner flag area. Everyone thought he was going to give the corner kick to Shaqiri on the side, but he quickly returned and kicked the corner kick to Origi in the penalty area, eventually completing a 4:3 reversal and perfectly interpreting the charm of the corner kick tactics.

Every time a tactic is formulated, not only the ability characteristics of one's own players must be considered, but also the tactical characteristics of the opposing team must be summarized based on past game records. Both require the coaching staff to review a large amount of video data and unravel the details.
Fortunately, with the development of science and technology, a large amount of data resources have been accumulated in the field of football. Artificial intelligence conducts comprehensive statistics and analysis on athletes' strength, speed, shooting accuracy, etc., and generates data reports from them. This allows coaches to more intuitively identify athletes' strengths and weaknesses and develop more targeted training plans and game tactics, which greatly alleviates the pressure on coaches and managers.
Based on this,Google DeepMind and Liverpool Football Club team up to launch TacticAI football coaching artificial intelligence assistant.The system uses a geometric deep learning approach with predictive and generative models to provide professionals with insights into corner kick tactics. Compared to the actual layout, the tactics proposed by TacticAI were recognized by human experts in 90% situations.
Research highlights:
* The tactical layout proposed by TacticAI is favored by human expert evaluators in all cases of 90%.
* The accuracy of ball reception prediction is as high as 74%, and the shooting chance is increased by 13%.
* Provide reference for tactical layout research of set pieces or other activities with suspended match situations.

Paper address:
https://www.nature.com/articles/s41467-024-45965-x
Follow the official account and reply "角球" to get the complete PDF
Dataset: Four major data sources, collecting feature information in all aspects
The original dataset consists of 9,693 corner kicks collected from the Premier League in the 2020-21, 2021-22 and 2022-23 seasons, provided by Liverpool FC, including the following 4 data sources:
* Space-time player tracking framework data,The positions and speeds of all players and balls on the field were tracked during each game.
* Event stream data,The events or actions (e.g., passes, shots, and goals) that occurred in the corresponding tracking frames were annotated.
* Match team data,The players' personal data were recorded, including their height, weight and position.
* Miscellaneous match data,Includes match dates, stadium information, and field length and width.
The researchers screened and filtered the data, leaving 7,176 valid corner kicks. The data was randomly sampled and divided into a training set and a validation set in a ratio of 8:2, and the same split method was used in all subsequent tasks.
Dataset address:
Experimental Method: Predicting Corner Kick Outcomes via Geometric Deep Learning

First, in order to effectively utilize corner kick data, the researchers modeled the implicit relationships between players and converted the corner kick forms into a graphical representation.Each node represents a player (including features such as position, speed, height, etc.), the edges represent the relationship between them, and each node is updated by passing messages to adjacent nodes.

Next, the researchers analyzed the handling of a given corner kick, used geometric deep learning and took advantage of the approximate symmetry of the football field to generate four possible reflections of a given situation (original, H-flipped, V-flipped and HV-flipped). Geometric deep learning starts from symmetry and embeds geometric properties into machine learning to better characterize the intrinsic structure and change patterns of the data, and improve the representation, generalization and generalization capabilities of the algorithm.
Finally, all four reflection combinations are applied to the corner-kick graph neural network structure and further fed into TacticAI. TacticAI consists of three predictive and generative models, all of which use the same encoder-decoder architecture. The encoder has the same structure in all tasks, while the decoder model generates outputs of corresponding shapes according to the requirements of each benchmark task.The three models correspond to the three benchmark tasks of this study, namely, ball reception prediction, shot prediction, and generating tactical layout strategies.
In summary, in ball reception prediction, TacticAI can predict whether a corner kick will result in a shot with an accuracy of 71%. In shot prediction, the researchers found that the average shooting probability of the defending team dropped from about 75% for a real corner kick to about 69% after adjustment.The attacking team's shot probability increased from around 18% to around 31%.
Experimental conclusion: Four case studies on the practical effectiveness of TacticAI
To evaluate the actual effectiveness of TacticAI, the researchers invited five football experts, including three data scientists, one video analyst and one coaching assistant, to conduct a quantitative analysis of TacticAI based on four cases.
1. Authenticity of corner kick samples generated by TacticAI

(A.2) Rating value histogram
First, to evaluate the realism of the generated adjustments, the researchers synthesized a dataset and evaluated the differences between the synthetic corner kicks generated by TacticAI and the real corner kicks.
Specifically, the researchers assigned each sample a score of +1 if it was rated as real by a human, and 0 otherwise. The average score of the five reviewers for each sample was calculated.The results show that there is no significant difference in the average score between the actual corner kicks and the generated corner kicks (z = -0.34, p > 0.05). Therefore, the corner kick samples generated by TacticAI are authentic.
z and p are statistical indicators commonly used in hypothesis testing in statistics.
2. TacticAI predicts the receiver's credibility

(B.2) The corresponding histogram of the average score of each sample
Second, for predicting the receiver, if at least 1 receiver appeared in TacticAI's first 3 predictions, the reviewer rated TacticAI's prediction as +1, otherwise it was rated as 0.
After averaging the predicted ratings of the sample catchers, the researchers found that there was no statistically significant difference in the average ratings of the predicted catchers for the actual sample and the generated sample (z = 0.97, p > 0.05).
There are individual differences in the ratings of catch predictions by different evaluators, and in this case, TacticAI is still able to maintain a high top-3 accuracy, which shows its high confidence in the task of predicting catchers.
3. Effectiveness of TacticAI for corner kick retrieval

Third, experts conduct a similarity analysis between the corner kicks retrieved by TacticAI and the reference corner kicks, scoring them with a score of +1 if they are similar, and 0 otherwise.
Finally found thatThere were no significant differences between the distribution of ratings across evaluators, indicating a high degree of agreement among them on the effectiveness of TacticAI’s ability to retrieve corner-like shots (F1,4 = 1.01, p > 0.1).
F1,4 and p are statistical indicators used to judge consistency or no significant difference.
4. The practicality of TacticAI’s strategy adjustment

Finally, the researchers evaluated the practicality of TacticAI's player adjustment suggestions in real life. Specifically, each reviewer was given 50 tactical adjustments and the corresponding real corner kick settings, and the reviewers rated each adjustment as significantly improving the tactic +1, significantly worsening it -1, and not significantly different 0.
The results showed that the average score of all five reviewers was 0.7 ± 0.1. Among them, the human reviewers found TacticAI's recommendation 90% to be favorable. In addition, their ratings were also highly consistent (F1,4 = 0.45, p > 0.05),This suggests that this usefulness is widely recognized among human experts.
In summary, TacticAI can efficiently complete the three benchmark tasks and is practically effective in corner kick prediction, retrieval, and tactical adjustment.
Breaking the traditional football pattern, AI becomes the "forward" of the new era
Futurist and trend observer Richard van Hooijdonk once said: “For the current generation, everything they do and experience includes some kind of technological element. In order to keep sports attractive to this generation and future generations, we have no choice but to continue to integrate technology.”When we focus on technological innovation in the world of football, it is not difficult to find that AI is no longer just a secret weapon for coaching teams. It is quietly reshaping the layout of the entire football industry with its ubiquitous influence.
From player selection to daily training to tactical planning, we can see AI in more and more links. For example:
* Adidas designed the Al Rihla match ball for the 2022 World Cup.The built-in inertial measurement unit can accurately detect the kick point of the football. By combining the player's body tracking and ball tracking data, it can monitor whether the attacker in an offside position touches the ball. (Click here for detailed report: 4 goals and 1 point, the technology and hard work behind Argentina's defeat)
* AiSCOUT launches AI-assisted scouting platform,It breaks the geographical and resource limitations of the traditional selection mechanism and provides scouts with data on football players' athletic ability, cognitive ability, technical ability, etc., so that scouts can select players more accurately.
* Zone7 launches AI player injury prediction platform,Using artificial intelligence to identify and predict player injuries can help athletes and coaches find the best balance between optimal movement and injury risk.
* ChyronHego launches AI-assisted refereeing platform,Through AI-driven ball tracking, limb tracking and skeletal modeling technology, the referee's "eagle eyes" are upgraded to "electronic eyes", accurately capturing the player's limb position at key passing moments, helping the referee make more accurate and timely decisions.
From player discovery and injury prevention to referees and coaching assistants, AI has spread to every aspect of football. An unprecedented era of football intelligence is coming. Will the "rich-poor gap" between clubs affect the AI content of players' regular training and further aggravate the Matthew effect between teams? I am afraid that all parties in the entire ecosystem need to think more fully.
References:
https://zhuanlan.zhihu.com/p/447351207
https://zhidao.baidu.com/question/161044184.html
https://soft.zhiding.cn/software_zone/2022/0622/3141934.shtml
https://www.sports-idea.com/news/12456.html
https://www.sohu.com/a/624410973_100111861