To Fully Explore the Active Ingredients of Natural Medicines, Professor Liu Shao's Team From Central South University Built the IMN4NPD Platform
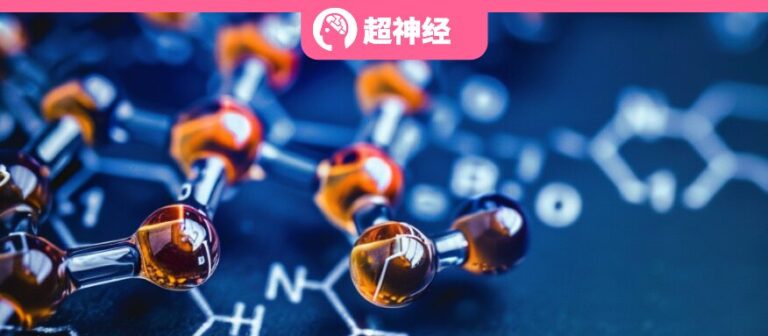
In 1806, the 23-year-old German pharmacist Sertürner isolated monomeric morphine from poppy for the first time, and modern natural drug chemistry research started from then on. On this basis, German chemist Friedrich Wǒhler successfully achieved the artificial synthesis of urea in 1828, which also marked the official birth of organic chemistry. It can be said that it is the continuous research of human beings on bioactive natural products (NPs) that has led to the establishment of organic chemistry.
The so-called bioactive natural products (NPs) are actually material entities that have evolved over a long period of time in nature. They are an important source of research and development of bioactive substances and practical drugs. In the process of drug development, NPs have made great contributions to the innovation of drugs for cancer and infectious diseases. However, to this day, NPs still face technical barriers in screening, separation, characterization, and optimization. Among them, separating NPs from complex mixtures is one of the most severe challenges, which has also become a major bottleneck in drug research.
To address this bottleneck, Professor Liu Shao's team from the Department of Pharmacy, Xiangya Hospital, Central South University, innovatively established an integrated molecular networking workflow for NP dereplication (IMN4NPD) that can comprehensively explore the pharmacological components of natural medicines.It not only speeds up the dereplication of extensive clusters in molecular networks, but also provides annotations for self-loops and paired nodes that are often overlooked in existing research methods. The relevant research results were recently published in Analytical Chemistry, a journal of the American Chemical Society (ACS).

Paper address:
https://doi.org/10.1021/acs.analchem.3c04746
Follow the official account and reply "Natural Medicine" to get the complete PDF
IMN4NPD: integrating multiple computational tools to drive molecular networks based on spectral similarity
The core working principle of IMN4NPD is molecular networking driven by spectral similarity.It helps researchers quickly identify specific classes of compounds by integrating and coordinating multiple computational tools such as NPClassifier, molDiscovery and t-SNE networks, while also simplifying the annotation of molecular network nodes.
* NPClassifier: A natural product structure classification tool based on deep neural networks
* molDiscovery: A mass spectrometry database search method
Generally speaking, the IMN4NPD workflow can be divided into 3 steps:
first step,The raw LC-MS data were preprocessed to generate molecular networks or feature-based molecular networks. Subsequently, SIRIUS, a deep neural network-based NP classification tool, was used to systematically classify the compound classes through NPClassifier.
Step 2,This study conducted dereplication experiments based on MS/MS spectral databases through GNPS (Global Natural Product Social Molecular Networking), and then performed computer database-based dereplication through molDiscovery.
Step 3.The researchers used the similarity of MS/MS spectral features to generate a t-SNE network and chemically classified the compounds at each node to accurately locate and replicate specific compound categories distributed in the self-circulating network.

Usability Assessment: Exploring Isoquinoline Analogs to Rapidly Identify Specific Compound Clusters in Molecular Networks
To evaluate the performance and advantages of the IMN4NPD workflow, the study reanalyzed the ethanol extract of lotus seed core, which is the embryonic part of the lotus pod and is a traditional Chinese medicinal plant rich in various alkaloids such as dibenzylisoquinoline, monobenzylisoquinoline, and aporphine, and can be used to treat insomnia, spermatorrhea, heart rhythm disorders, hypertension, and other symptoms.
Based on the experimental MS/MS spectral database, the study initially chemically classified individual nodes in the molecular network, thereby quickly identifying specific compound clusters in the molecular network to explore new isoquinoline analogs. After reviewing the chemical classification results of each feature mapping in the molecular network, the researchers found that it was easy to find certain compound clusters corresponding to isoquinoline analogs, and at the same time, isoquinoline compounds were mainly distributed in four clusters in the molecular network.

The study also found that only a limited number of features in large clusters could be successfully replicated using experimental MS/MS spectral databases such as the GNPS database.This study used the state-of-the-art in silico fragment algorithm molDiscovery for structural database matching.This de-duplication approach based on experimental and in silico MS/MS spectral databases enhances the ability to annotate material structures in molecular networks, especially in large clusters, in a timely and convenient manner.
Taking cluster A in monobenzylisoquinoline alkaloids as an example, this cluster consists of 36 nodes, of which only 7 nodes are annotated by the MS database, 35 nodes are annotated by the Structure database, and 8 nodes are annotated by both the MS and Structure databases. It is worth noting that there is a node with m/z 344.1855 (tR=7.6329) was fully annotated by the MS structure database, which indicated that the candidate structure was 3′-O-methyl-4′-methoxy-N-methylcoclaurine (as shown above).
Through further analysis, the node has lost NH3CH3. CH3OH and H2O, followed by ring fragmentation, α fragmentation, and β fragmentation, producing fragment ions at m/z 107.0496, 137.0597, 151.0757, 175.0750, 205.1098, 235.0752, 267.1017, 299.1271, and 312.1590, respectively.
Identified by Structure database, m/z 448.1963 (tR = 1.6287) is N-methylnorcoclaurine 7-O-glucoside. Another m/z 312.1593 (tR = 7.3621) node shows four candidate structures including one monobenzylisoquinoline.R=7.6329) compared to the node at m/z 190.0862 (C11H12NO2) indicates that this is a methylenedioxy group.
Research results: Based on deep neural networks, comparing the three research algorithms from the perspective of t-SNE networks
Compared with MolNetEnhancer,IMN4NPD uses NPClassifier, a deep neural network-based NP classification tool, to individually classify each feature in the molecular network.Rather than the entire cluster or molecular family. This study used an improved cosine similarity to calculate the similarity matrix and used it to generate a t-SNE network. At the same time, the study also classified each node based on its MS/MS spectral data through NPClassifier and mapped these classifications into the t-SNE network.
In the traditional molecular network view, isoquinoline is generally composed of three large clusters (clusters AC) and one small cluster (cluster D). From the perspective of the t-SNE network, it is obvious that the four cluster nodes of isoquinoline are tightly grouped to form different cluster areas. But it is worth noting that from the perspective of the t-SNE network, cluster A in the molecular network can be further divided into two smaller clusters. In addition,t-SNE can effectively locate isoquinoline nodes, thus greatly reducing the structural analysis work of related nodes.

The modified cosine similarity method has limitations in the face of spectra of various chemically modified compounds. This study also selected similarity algorithms such as Spec2Vec and MS2DeepScore to generate t-SNE networks. Based on Spec2Vec, isoquinoline still forms four major clusters in the molecular network.
However, based on MS2DeepScore, the nodes of large clusters A and B of isoquinoline are closely spaced, forming several clustering regions, but the nodes in large cluster C are scattered throughout the graph, which poses a challenge for subsequent analysis.

An interesting phenomenon is that the m/z 296.1646 node (tR = 11.54) In the t-SNE diagrams of corrected cosine similarity and MS2DeepScore similarity, it is far away from the node clustering area related to isoquinoline, but in the t-SNE diagram based on Spec2Vec spectral similarity, this node is adjacent to the clustering area of large cluster A. This type of self-exchange node may represent a class of isoquinoline compounds, and after further comparison, it can be confirmed that the node is an aporphine alkaloid.
therefore,The chemical classification of compounds and t-SNE network can provide different information about the features, respectively, which reduces the occurrence of false negatives to a certain extent.
In addition, based on the t-SNE network of Spec2Vec spectral similarity, there is a m/z 298.1438 (tR = 7.02) and m/z 298.1438 (tR = 7.60) are two nodes, which are the self-exchange node and the pair node in the molecular network. Although they are not classified as isoquinoline compounds, they are similar to the isoquinoline cluster A. Further analysis shows that m/z 298.1438 (tR = 7.02) is a known aporphine alkaloid, nornuciferidine, m/z 298.1438 (tR = 7.60) also showed aporphine alkaloids similar to nuciferine and nornuciferidine.
Through the study of the above three nodes, it was found that they all belong to aporphine alkaloids, which is different from monobenzylisoquinoline alkaloids. When using modified cosine similarity and MS2DeepScore similarity, these three nodes are far away from the cluster A of the clustering area of monobenzylisoquinoline alkaloid-related nodes, but based on Spec2Vec, these three nodes can be found near cluster A.
This difference demonstrates the superior ability of Spec2Vec spectral similarity in accurately capturing similar structures of isoquinoline compounds.
The application of artificial intelligence in natural product research is accelerating
In recent years, benefiting from the rapid development of various modern technologies, a large number of new strategies and methods based on LC-MS/MS and NMR technologies have emerged in the research of natural bioactive molecules, integrating multidisciplinary technical means such as bioinformatics, metabolomics, and computer science. In particular, as artificial intelligence and machine learning algorithms begin to be integrated into natural product research, a new round of productivity revolution has been further brought to researchers.
Initially, the application of artificial intelligence focused on the digitization of organic molecules and mapping the NP chemical space using dimensionality reduction techniques. Later, researchers developed machine learning binary classifiers to predict the biological functions of NPs. Today, neural network architectures are beginning to be used for genome mining and molecular design, and deep learning algorithms are becoming increasingly popular in the fields of drug discovery and molecular informatics.
Therefore, we can see that the industry, academia and research have accelerated the pace of related research in recent years. In 2022, the National Supercomputing Guangzhou Center, together with Sun Yat-sen University, Star Pharmaceutical Technology, Massachusetts Institute of Technology and Georgia Institute of Technology, based on the powerful computing and storage capabilities of Tianhe-2,A deep learning-driven bioretrosynthetic pathway navigation tool BioNavi-NP is proposed.
In the corporate world, research on natural products is also accelerating. In 2023, Tasly Pharmaceutical Group and Huawei Cloud reached a cooperation agreement. The two parties will combine the modern research data of natural products,Co-build a vertical big model in the field of traditional Chinese medicine.
However, natural product databases are still a major challenge in the scientific research process. At present, the mainstream natural product data repositories in the world include the Minimal Information of Biosynthetic Gene Clusters (MIBiG), Natural Product Maps (NP Maps), Global Natural Product Molecular Network (GNPS), Natural Product Magnetic Resonance Database (NP-MRD), etc., but these databases have low coverage and common data errors, which hinder the progress of artificial intelligence in natural product drug discovery.
In recent years, Chinese scientist Tu Youyou, Japanese scientist Omura Satoshi, and Irish scientist William C. Campbell have been nominated for the Nobel Prize in Chemistry for their achievements in the total synthesis of natural products. There is no doubt that as the importance of natural products continues to increase, the integration of artificial intelligence in natural product research is about to accelerate.