Princeton University Releases AI Controller to Predict Plasma Tearing Risk 300 Milliseconds in Advance
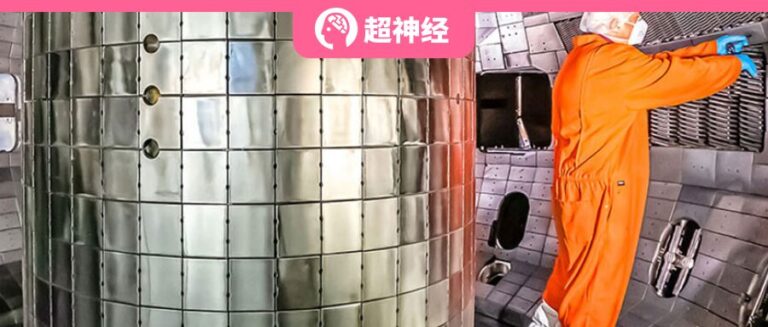
Under the dual pressures of growing energy demand and the approaching deadline for the "carbon neutrality" target, the application of clean energy with low or even zero carbon emissions is the general trend. In recent years, nuclear fusion, which has the potential for zero carbon emission power generation, has received more widespread attention, and more and more scholars and experts have begun to conduct in-depth research.
Despite the remarkable success of Tokamak fusion experiments, obstacles still exist, among which plasma interruption is one of the most critical problems that must be solved for the successful long-pulse operation of ITER. The main reason is that plasma is very easy to "tear" and escape the strong magnetic field used to confine it, thus causing the interruption of the fusion reaction.
In previous studies, it has been possible to maintain fusion energy for a short period of time. Now, as deep reinforcement learning (DRL) technology has shown high performance in nonlinear and high-dimensional driving problems, people have begun to explore its introduction into nuclear fusion research.Not long ago, researchers at Princeton University developed an AI controller for adaptive prediction and control, which can predict the potential risk of plasma tearing 300 milliseconds in advance and intervene in time. The relevant results have been published in "Nature".

Paper address:
https://www.nature.com/articles/s41586-024-07024-9
Follow the official account and reply "nuclear fusion" to download the full paper
Combining traditional physics methods with AI
As shown in the figure below, researchers integrated traditional physics-based methods with advanced AI techniques to improve the control and understanding of plasma behavior.

Figure a:Diagnostic system, showing the main diagnostic tools used in this study to monitor and analyze the plasma inside the DIII-D tokamak.
These include magnetics to measure magnetic fields, Thomson scattering (TS) to measure density and temperature distributions, and charge exchange recombination (CER) spectroscopy to measure ion temperature and flow rates. The specific tearing instability mode m/n =2/1 is highlighted in orange in the figure to highlight its importance.
Figure b:Heating, Current Driving, and Controlling Actuators, showing systems for heating plasma, driving current through it, and controlling its behavior.
These include equipment for injecting particle beams, applying magnetic fields, and using microwave or radio frequency waves for heating and current driving. Control actuators play a key role in manipulating the plasma to achieve the desired conditions while combating instabilities such as the m/n = 2/1 tearing mode.
Figure c:A control system for avoiding tearing, showing a control system for preventing or mitigating tearing instabilities.
In its preprocessing step, the signals from the diagnostic system are processed into structured data of the same dimension and spatial resolution through profile reconstruction and equilibrium fitting (EFIT), and then input into the deep neural network (DNN) model.
Figure d:The AI controller based on deep neural network determines the overall beam power and plasma shape.
An equilibrium fitting (EFIT) algorithm handles low-level control tasks, adjusting the magnetic coil current and beam power to satisfy the AI's control commands and user-preset constraints, such as maintaining a specific safety factor (q95) and beam torque.
Reinforcement Learning Algorithms: Anti-tearing Control
In a fusion reactor, the state of plasma is shown in the following figure:

The black line in Figure a shows that as the plasma pressure is increased by external heating (such as a neutral particle beam), a stability limit is eventually reached. When this limit is exceeded, tearing instabilities are induced. Figures b and c show that once tearing instabilities are induced, the plasma will be rapidly destroyed, which can lead to serious consequences in practical operations.
Based on deep neural networks and reinforcement learning, the researchers developed an intelligent control system that can respond to changes in plasma states in real time, predict the future state of plasma, and adjust control actions accordingly, so that the tokamak operation follows the ideal path and avoids tearing instabilities while maintaining high pressure.

The reinforcement learning model uses a reward function to implement this obstacle-avoidance problem. The dynamics model used to predict future tearing instabilities integrates with the OpenAI Gym library, allowing it to interact with the controller as a training environment. The tearing-avoidance controller is trained using a deep deterministic policy gradient method implemented in Keras-RL.
The advantage of reinforcement learning is the ability to enter the higher βN region through multi-actuator (beam and shape) and multi-objective (low tearing and high βN) controllers while maintaining a tolerable tearing.
Tear control in DIII-D: Traditional vs. AI
Limitations of Traditional Feedback Control
We attempted to maintain the normalized plasma pressure (βN = 2.3) by conventional feedback control methods. However, in experiment No. 193273 (black line in the figure below), when the time reached 2.6 seconds, a large tearing instability occurred, resulting in an irreversible degradation of βN.Finally, plasma interruption occurred at 3.1 seconds.
Advantages of AI control
In experiment No. 193280 (blue line in the figure below), AI control was used to adaptively control the beam power and the three plasma angles to ensure that the predicted tearing degree did not exceed the threshold of 0.5.
The experimental results show that the estimated tearing degree can be successfully maintained below a given threshold through AI control, and a lower tearing degree can be achieved compared to the 193273 experiment.

Control strategies under different tearing thresholds

The researchers compared the control strategies under different tearing thresholds. Experiment numbers 193277 (gray line in the above figure), 193280 (blue line in the above figure) and 193281 (red line in the above figure) correspond to control experiments with tearing thresholds of 0.2, 0.5 and 0.7 respectively.
In the cases of k = 0.5 and k = 0.7, the plasma exhibits good stability before the end of the predetermined plateau period. The controller with a higher threshold (k = 0.7) tends to increase βN more aggressively in the early stages of the experiment (t < 3.5 s), but this causes the plasma to enter a more unstable region later.
The lower threshold controller with k = 0.2 was too conservative early in the experiment and overly suppressed the possibility of instability. The AI maintained a very low tearing degree (less than 0.2) until t = 5 seconds, but suddenly showed unavoidable instability at t = 5.5 seconds.
In contrast, the controller with a medium threshold (k = 0.5) is able to maintain the plasma stability until the end of the plateau period and eventually restore βN again.This suggests that an optimal threshold is required to maintain a stable plasma for a long time.
Chasing the Holy Grail of Clean Energy Still Faces Many Challenges
The realization of controlled nuclear fusion is one of the most challenging goals in modern science and engineering, and is considered the holy grail of clean energy. When will humans be able to hold this holy grail, and what role does AI play in it?
This study successfully demonstrated the potential of AI in effectively controlling fusion reactions. In addition, Jaemin, the first author of this paper, also innovatively used reinforcement learning methods while pursuing a doctorate degree in the Department of Nuclear Engineering at Seoul National University to design a new plasma control algorithm for KSTAR (Korea Superconducting Tokamak Advanced Research Facility).

Although more and more researchers have begun to conduct in-depth research on nuclear fusion and are getting closer to this holy grail step by step, there are still many technical barriers that need to be solved:
1. Plasma stability:
Controlling the stability of high-temperature plasma is one of the key challenges in achieving controlled nuclear fusion. Plasma must be kept at a sufficiently high temperature and pressure to maintain the necessary fusion reaction rate, which requires extremely precise magnetic field control technology.
2. Material issues:
Currently, there is no material that can withstand long-term high-temperature, high-neutron flux irradiation without significant degradation. Neutron irradiation causes the material to become brittle and its performance to degrade, which is a big problem for the long-term operation and economics of the reactor.
3. Energy capture and conversion:
How to efficiently capture energy from fusion reactions and convert it into electrical energy is also a focus of current research. A highly efficient thermoelectric conversion system is essential to achieving economically viable fusion energy.
4. Fusion fuel generation and supply:
Tritium and deuterium can undergo nuclear fusion reactions and are used as controlled nuclear fusion fuel. Although deuterium is relatively abundant in nature, tritium is extremely scarce. Because it is extremely difficult to prepare artificially, it needs to be produced inside the reactor through neutron capture or other methods. One kilogram of tritium is worth hundreds of millions of dollars.
In recent years, AI has brought too many surprises in scientific research, from AlphaFold's efficient prediction of protein structure, to rendering black hole images based on machine learning, to predicting weather changes based on neural networks...
Today, the problem of plasma stability has been solved by AI, which has accumulated experience for the application of AI in the research process of controlled nuclear fusion. I believe that more of the above challenges will also be overcome with the continuous exploration of researchers!